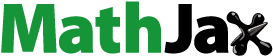
Abstract
Urban development relies heavily on labour, while the change in urban housing prices, in turn, has a crucial impact on labour. Therefore, the regulatory policies of housing prices have become a critical content of urban management worldwide for a long time. Based on the theoretical analysis of urban housing prices and labour mobility, we use fixed-effect panel model with the panel data of 281 cities in China from 2002 to 2018. We study the impact of rising urban housing prices on labour and identify the housing price jump as an instrumental variable in solving the endogenous problem. The results are as follows. First, the increase in urban housing prices has a crowding-out effect on labour mobility. Second, there is a moderating effect on different levels of education, urban construction, and income under the impact of urban housing prices on labour mobility. Third, the heterogeneity of the impact is reflected in the housing price-to-income ratios and industrial structures. These conclusions are supposed to have significant enlightenment for controlling the urban housing prices during high-quality economic growth and guiding labour flow more rationally.
1. Introduction
1.1. Motivation
The modern city is the main space where labourers gather, providing them with housing, employment opportunities, and related social welfare. At the same time, labourers make corresponding choices for their activity space based on internal constraints and external circumstances. Labour mobility has a far-reaching impact on optimising the spatial allocation of resources. It gradually replaces industry as the core element determining the success or failure of regional economic development. Over the past decade, many economies have experienced a phase of rapidly rising housing prices. The prosperity of the real estate market has also had a specific impact on the local labour market. According to the Organisation for Economic Cooperation and Development (O.E.C.D.), the real value added to the real estate of 27 economies averaged 9.78% from 2002 to 2018. The prosperity of the real estate market and competition of among real estate companies with large-scale strategies have driven the soaring prices.
The rising housing prices increase the actual costs of enterprises and affect the living quality of the labourers. It impacts the agglomeration and diffusion of urban labour, and further changes the local industry clustering and structure. The rising urban housing prices have hindered labour influx and decreased the spatial allocation efficiency of labourers (Hsieh & Moretti, Citation2019). The housing prices in the U.K. have a distributional effect on labour supply (Disney & Gathergood, Citation2018). The spatial changes of housing prices in Norway reflect the characteristics in the local labour market (Osland & Thorsen, Citation2013). Cities with higher housing prices in the Netherlands generally have superior working locations and better urban infrastructural facilities (Garretsen & Marlet, Citation2017). At the same time, the wealth effect of housing prices will further expand the polarisation of social resource allocation. With the persistently high housing prices, any small change will magnify several times to affect the relevant interest groups. Since China’s reform and opening-up, urbanisation has accelerated, and it has brought substantial demographic dividends and a corresponding housing problem. The labour migration in China reflects an outbreak trend. It is outstanding research to improve urban competitiveness and attract high-quality labour through reasonable housing price planning policies. The inflow of high-quality labour can accelerate economic transition and upgrading and achieve high-quality development. In this context, we study the impact of rising housing prices on labour mobility from an actual perspective in China. We further refine the relevant conclusions about the exact impact of urban housing prices on labour mobility under the background of the different social and cultural systems.
1.2. Literature review and contribution
The difference in urban housing prices profoundly affects labour mobility. The most critical macroscopic theory in demography is the ‘push–pull’ one in the fundamental theory of labour migration. The current research on the impact of urban housing prices on labour mobility falls into two categories. The rise in urban housing prices has a crowding-out effect on labour mobility. There is a spatial interaction between the real estate and housing market (Hincks, Citation2012). Further research finds that housing value is closely related to fundamental economic variables such as labour (Han et al., Citation2018; Li & Zhong, Citation2020). Peng et al. empirically study the positive correlation between housing prices and population migration and find that the impact in the short-term is not as significant as in the long-term (Peng & Tsai, Citation2019). The housing market seems to form the primary mechanism that slows down the workers’ mobility (Hämäläinen & Böckerman, Citation2004). The rate of population inflowing in high-income areas has decreased because the rising housing prices prevent migrants with low skills (Ganong & Shoag, Citation2017). Plantinga et al. believe that higher housing costs decrease the possibility of labourers entering a particular area (Plantinga et al., Citation2013). The higher housing prices will push the local labourers to the surrounding area with low (Guerrieri et al., Citation2013; Jeanty et al., Citation2010). The other one is that the rise in urban housing prices has a clustering effect on labour. Cities with higher housing costs will attract more labourers with more advanced job skills and higher education because they can reap rich rewards in those areas (Moretti, Citation2013; Zhou et al., Citation2020). The expected wealth effect of rising housing prices promotes the willingness of workers to live in immigrant areas (Li, Zou, & Mo, Citation2021; Zang et al., Citation2015). Rising housing price has a positive wealth effect and a negative lock-in effect on labour migration (Foote, Citation2016). Although different scholars have different conclusions about the impact of urban housing prices on labour mobility, these conclusions are not contradictory. The impact of housing prices on labour mobility presents an inverted U-shaped relationship (Zhou et al., Citation2020). The crowding-out effect is reflected on the right side of the inverted U-shaped curve, and the clustering effect is reflected on the curve’s left side. Meanwhile, according to the labour market segmentation theory, the labour market is divided into a primary market dominated by mental workers and a secondary market dominated by manual labour. The impact of urban housing prices rising on these two types of labour markets is also significantly different.
The impact of urban housing prices on different labourers varies due to the external environments. The decision-making factor of urban education level has the most significant impact on selecting family location (Bayoh et al., Citation2006). People are willing to afford higher housing prices to obtain better educational resources (Albouy, Citation2016; Bayer et al., Citation2007; Muellbauer & Murphy, Citation2008). Therefore, the proportion of the higher education population increases continually in areas with higher housing prices (Sorens, Citation2018). This is because the education gap often results in an income gap (Diamond, Citation2016; Li et al., Citation2017; Moretti, Citation2013). If the average duration of education increases by one year, personal income will increase by 11–13% (Liu, Citation2007). Higher educated labourers usually have access to more income, and their affordability of housing prices will increase accordingly. They are more concerned about the welfare effect of human capital clustering (Haviernikova & Kordos, Citation2019; Yang & Pan, Citation2020). The changes in salary, rent, and construction will exacerbate the inequalities among employees from different educational backgrounds, with effect beyond education itself (Diamond, Citation2016). Urban housing prices is inseparable from natural and artificial facilities (Carruthers & Mulligan, Citation2012). Urban dwellers are willing to pay higher housing costs for high-quality urban facilities (Carmona et al., Citation2017; Zheng et al., Citation2009). While enjoying the dividend of population growth, those developing countries will also face the challenges that municipal infrastructure cannot keep up (Gorelick & Walmsley, Citation2020; Li et al., Citation2019). Cities with superior levels of urban construction have significantly higher housing prices (Garretsen & Marlet, Citation2017). The protection zone in historic blocks led to a rise in housing prices under a specified salary (Waights, Citation2019). Environmental pollution poses a significant threat to public health that can affect labour migration (Kwakwa et al., Citation2021; Yang et al., Citation2021). The housing prices and income trends are driven by aggregate demand, not just by changes in local factors such as productivity or facilities (Gyourko et al., Citation2013; Jia et al., Citation2021). The income gap significantly affects the migration decision of labourers, and this impact is heterogeneous in gender (Zhu, Citation2002). An equal increase of wage in the labour market will lead labour to move from rural to urban, while the equal increase of housing prices results in labour moving to rural areas (So et al., Citation2001). Although relevant studies are affluent, there is limited heterogeneity in the impact of urban housing prices on labour mobility. Most generally starts from gender, urban and rural areas, and primary and secondary labour markets. The study subjects are based on the whole country or an individual city. Therefore, it is necessary to study further the impact of urban housing prices on labour mobility.
The main contributions of this paper are as follows. First, we study the impact of housing prices on labour mobility in China’s prefecture-level cities and compare the distinction between the short- and long-term impacts. To address the endogeneity problem in this paper, we innovatively construct housing price jump as the instrumental variable. Second, we analyse the moderating effect of urban housing prices on labour mobility. The level of education, urban construction and income can alleviate the crowding-out effect of rising urban housing prices on labour mobility, effectively supplementing existing studies. Third, we analyse the heterogeneity of the impact of urban housing prices on labour mobility. On the one hand, the impact of housing prices on labour mobility is heterogeneous in the housing price-to-income ratio. The intensity of the crowding-out effect of rising housing prices on the labour first strengthens and then weakens with the increase in house price-to-income ratio. On the other hand, the impact of urban housing prices on labour mobility is heterogeneous in different industry structures. The crowding-out effect of rising urban housing prices is weaker for the primary industry and more significant for the tertiary industry.
The remainder of the article is as follows (see for the logical framework).
2. Research design
2.1. Theoretical analysis and research hypothesis
The rise of urban housing prices, mainly commercial housing prices, will have a specific crowding-out effect on labour mobility. Labour mobility is essentially a process of re-selection of living space. According to the labour push–pull theory, the factors that promotes the improvement of living conditions in labour flow is ‘pull’. In contrast, the factors that are not conducive to improving living conditions in labour flowing out are ‘push’. ‘Pull forward and push backward’ factors affect labour mobility collectively (Benjamin, Citation1970). In China, the current restrictions on the free movement of labour are relatively weak, and labour can move and migrate freely across regions. The problem is not the labour barriers but how to actually keep the labour here. According to the labour market segmentation theory, China’s secondary labour market currently dominates, and the impact of urban housing prices on labour is shown as a crowding-out effect on the right side of the inverted U-shaped curve. The rapid rise of high housing prices will increase the living cost of labourers without home ownership, reduce the city’s attractiveness, and hinder the city’s introduction of talents. At the same time, labourers’ expectations are linked to urban housing prices. Suppose the rising rate of housing prices is higher than labour’s inner expectation or the tolerable range. Under this scenario, the labourer will become increasingly pessimistic about their future living standard and tend to reselect the employment area. Based on this, we propose the following hypothesis H1:
H1: Urban housing prices have a significant crowding-out effect on labour mobility.
H2: The level of education, urban constructions, and income respectively play a moderating role in the impact of urban housing prices on labour mobility.
H3: The impact of urban housing prices on labour mobility is heterogeneous in various cities with different housing price-to-income ratios or industrial structures.
2.2. Methods
2.2.1. Housing prices and labour mobility
In this article, the benchmark models of the impact of urban housing prices on labour mobility are set as the following model (1) and model (2):
(1)
(1)
where, the subscript
represents the sample city, the t represents the sample time. The
represents labour force, the
represents urban housing price, the
represents the control variables at the city level, including the economic development level
the industrial structure upgrading
the postal development level
the green area
the medical treatment
the cultural construction
the employment situation
the living costs
and the natural growth rate
To further investigate the short- and long-term distinction in the impact of labour mobility on urban housing prices, we introduce the P.M.G. estimator. The P.M.G. estimator could provide a good estimate of the coefficients both for short- and long-run relationship (Salim et al., Citation2020). Nested the model (1) into a general A.R.D.L. framework as follows:
(2)
(2)
where,
is coefficient of lagged dependent variables;
is a
vector of control variables and
is a
vector of control coefficients. Model (2) can be further reparametrised into an error-correction form as follows:
(3)
(3)
The parameter vector and
contains the long-run relationship between labour mobility and urban housing price. The parameter
is the error correction term. It measures the speed of adjustment of
towards its long-run equilibrium value following a change in
If
is 0, then there is no long-run equilibrium relationship between the dependent and independent variables. The parameter vector
and
contains the short-run relationship between labour mobility and urban housing price.
2.2.2. Test for moderating role
The previous analysis shows that there are moderating effects of education level, urban construction level, and income level in the effect on the impact of urban housing prices on labour mobility. To verify these three moderating mechanisms, the model is specifically set as follows.
(4)
(4)
where, the
is moderating role, including education level (
), urban construction level (
) and income level (
). In model (4), the coefficients
reflects the moderating effect.
2.3. Instrumental variable: housing price jump
The bilateral relationship between the variables mainly characterises the endogeneity of the rise in urban housing prices and labour mobility. We refer to the method of Charles et al. (Citation2018) and use the regression discontinuity design (R.D.D.) method that can effectively analyse the causal relationship between urban housing prices and labour mobility. The essence of R.D.D. is to estimate the ordinary least squares (O.L.S.) on both sides of the specific time and detect the changes of the two fitting lines at the discontinuity. The reasons for adopting the R.D.D. to identify the housing price jump are as follows. First, this paper has the advantage of a large sample size to analyse the housing price jump comprehensively. Second, identifying provincial housing price jump helps to eliminate the impact of a single policy in a city. Third, as a classic policy evaluation model, using R.D.D. to identify the housing price jump can effectively eliminate the endogeneity caused by neglected variables related to time trends, which is not possible with a single structural breakthrough. Fourth, the single structural break will be close to 0, which loses much data to analyse where the house price changes linearly over time. The R.D.D. can get more data on housing price jump to make the estimation more precise.
The housing price jump can estimate the causal relationship between them. The economic logic of this instrumental variable is that the economic fundamentals of a city will not change suddenly in a short period. What’s more, short-term structural changes in housing prices mainly from short-term shocks within the real estate market, rather than from traditional fundamentals. Such as speculative investment behaviour in real estate, changes in the adjustable interest rate collateral loans, or the downregulation of the housing loan rate (Chiang, Citation2020; Di Maggio et al., Citation2017; Favilukis et al., Citation2017; Matei, Citation2020; Mayer, Citation2011). The housing price jump can be used as an instrumental variable to explain changes in house price due to external shocks that are independent of other economic fundamentals. This type of shock will not affect labour’s employment city selection through channels other than housing prices. Therefore, it is a reasonable instrumental variable. The accuracy of the R.D.D. results is affected by the model set, of which the bandwidth is the key. As for the bandwidth selection, the I.K. method is used to calculate the Optimal Bandwidth (Imbens & Kalyanaraman, Citation2012; Li, Yang, et al., Citation2021). According to the research of Lee and Lemieux (Citation2010), the following model (9) is constructed:
(9)
(9)
Among them, the is the processing variable. That is, the
takes 1 after the housing price jump, otherwise takes the value 0. The
is the execution variable, that is,
minus the difference of the time when housing price jump. The
is the error term. In the provinces where 281 cities are located divided, the overall housing price jump in different cities within each province is identified.
2.4. Variables description and data sources
This article selected 281 prefecture-level cities in China from 2002 to 2018 as the initial sample considering the data availability. The dependent variable is labour represented by the population mobility ratio through the population mobility formula. The population mobility = (resident population
registered population)/resident population. The value is positive if the labour force flows into the city and negative when the labour force flows out of the city. It should be noted that the population mobility may include the mobility of the non-working population, which is larger than labour mobility, but does not constitute a substantive impact. Because the increase in urban housing prices, especially commodity housing prices, creates a certain extrusion effect on labour, the dependent variable is commodity housing prices
Referring to the practices of existing kinds of literature (Shen, Citation2012), the control variables are: economic development level
industrial structure upgrading
postal development level
the green area
the medical treatment
cultural construction
the employment situation
the living costs
and natural growth rate
Based on the research objectives, three indicators including education level, urban construction level, and income level, are selected as moderator variables in this article. The education level is calculated by the per capita local general public budget education expenditure. Since China’s higher education resources are mainly public, education has strong external economic benefits and the market will fail in the education sector. The urban construction level
represented by the built-up area of land. The per capita income measures the income level
Data on all variables are obtained from the China Urban Statistical Yearbook, except for house price data, which are obtained from the website of Guoxin Real Estate Information. reports the descriptive statistical results of all variables.
Table 1. Descriptive statistics.
Again, we employ the widely used panel unit root of the Levin-Lin-Chu test, the Breitung Test, the Im-Pesaran-Shin test and the Hadri Lagrange multiplier stationarity test. As shown in . Because the data set contains both I(0) and I(1) variables, model (3) opts to apply pooled mean group (P.M.G.) for estimation, which is robust to this type of panel data set.
3. Empirical results
3.1. Analysis of the impact of urban housing price on labour mobility
According to the setting models, the parameter estimation results are reported in . reports the fixed-effect model’s regression results of the impact of urban housing prices rising on labour mobility. According to the estimation results in columns (1) and (2), the rising urban housing prices have a significant crowding-out effect on labour mobility. Column (3) is the regression results obtained by the 2SLS estimation model. The results obtained by instrumental variables are consistent with the regression results of the fixed-effect model. That is, the urban housing price rising has a crowding-out effect on labour mobility. According to the labour push–pull theory, the crowding-out effect of urban rising housing prices on labour mobility is ‘push’. The rise of housing prices can increase the living cost of labourers and decrease their ability to pay. High housing prices will lead to lower life expectancy and a redirection of employment fields. The regression coefficient of the instrumental variable is significantly larger than the O.L.S. estimation, mainly because the instrumental variable solves the endogeneity of homogeneous change of urban housing prices rise and labour mobility. Some unobservable economic factors may cause urban housing prices and labour mobility to change in the same direction. The above conclusion is consistent with hypothesis H1.
Table 2. Panel unit root test.
There are three specific estimation methods for model (3) of P.M.G., M.G. (mean group) and dynamic fixed effects (D.F.E.). The D.F.E. estimator allows the intercept term to vary between cross-sectional units with a constant slope coefficient. The M.G. estimator accepts the intercept term and slopes to vary between cross-sectional units. However, the P.M.G. estimator lies in between the above two extreme estimators. According to , the P.M.G. estimator is preferred over the M.G. estimator ().
Table 3. Housing price and labour mobility.
Table 4. Housing price and labour mobility.
The error correction term is significantly negative, indicating that labour mobility is cointegrated with urban housing prices. The coefficient of error correction term implies that in the short run, the annual adjustment deviates from the long-run equilibrium relationship by about 29.7%. The empirical results show that rising housing prices promote labour mobility in the short run, but inhibit it in the long run. The reason may be that workers have a certain lagged response to the fluctuation of house prices. Rising house prices will cause an increasing cost of living for workers, lower savings, and cause labour outflow.
3.2. Robustness test
3.2.1. Robustness test of bootstrap
This section uses Bootstrap to perform re-sampling to obtain more gradual and effective estimators. We use 2000 repeated samplings to obtain the regression coefficients, and the results are reported in .
Table 5. Results of robustness test of bootstrap.
3.2.2. Robustness test of adjusted variables
We conduct the robustness analysis by altering the dependent variables in this part. The dependent variable labour is alternated by the proportion of urban unit employees in the total urban population. The regression results are reported in .
Table 6. Results of robustness test of adjusted variables.
The results in are still the same as the above results. That is, the conclusions are still robust. It suggests that the conclusions drawn in this article remain unchanged when the indicator measurement method changes. The conclusions are still valid when using similar data.
4. The moderating effect on the impact of urban housing prices on labour mobility
4.1. The moderating effect of education level
The regression results are reported in . Here we mainly focus on the cross-term coefficients between housing prices and education level.
Table 7. Estimations of the moderating effect of education level.
shows that education can alleviate the crowding-out effect of rising house prices on labour mobility. The empirical result verifies that the education level has a moderating effect on the impact of urban housing prices on labour mobility. In other words, the labourers are willing to undertake higher housing prices to have better educational resources. Generally, more educated labourers can earn higher income, thus offsetting the crowding-out effect caused by higher housing prices. Cities with abundant education resources can reduce the likelihood of local labourers’ outflow. With more education, labourers can improve their ability to pay housing prices.
4.2. The moderating effect of urban construction level
The regression results are reported in . Here we mainly focus on the cross-term coefficients of housing prices and the urban construction level.
Table 8. Estimations of the moderating effect of urban construction level.
According to , the urban construction level can alleviate the crowding-out effect of rising housing prices on labour mobility. The empirical result verifies that the urban construction level has a moderating effect on the impact of urban housing prices on labour mobility. With the improvement of urban construction, labourers are willing to bear higher housing prices for high-quality living needs. When the urban is better built, labourers can enjoy more social welfare and offset the crowding-out effect.
4.3. The moderating effect of income level
The regression results are reported in . Here we mainly focus on the cross-term coefficients of housing prices and income levels.
Table 9. Estimations of the moderating effect of urban income level.
According to , income level can alleviate the crowding-out effect of rising urban housing prices on labour mobility. The empirical result verifies that the income level has a moderating effect on the impact of urban housing prices on labour mobility. The income level is directly related to the labourers' affordability to housing prices. As the income level increases, the affordability increases too. At the same time, the living standard of labourers increases due to higher income levels, while housing prices reflect the level of social development to a certain extent. High-income labourers can accept higher housing prices.
Based on the above conclusions, hypothesis H2 is accepted.
5. Heterogeneity analysis of the impact of housing price on the labour mobility
5.1. Heterogeneity analysis based on the housing price-to-income ratio differences
The housing price-to-income ratio roughly reflects the relationship between urban income and housing price, suggesting the affordability of urban residents and the pressure of the real estate market. We sort the total samples according to the different levels of housing price-to-income ratio into five groups. To analyse the impact of rising urban housing prices on labour mobility under different levels of housing price-to-income ratio. The estimated results are reported in .
Table 10. Heterogeneity based on the housing price-to-income ratio differences.
reports the impact of urban housing prices on labour mobility. The research indicates an inverted U-shaped curve between the intensity of the crowding-out effect of urban housing prices on labour and the housing price-to-income ratio. The main reasons may be as follows. First, the real estate market bubble is relatively tiny in cities with relatively low housing price-to-income ratio, and the labourers' housing affordability is relatively high. Second, cities with higher housing price-income ratio are generally the first-tier or super first-tier cities in China. They always have rich education resources, leading urban construction levels, broader employment opportunities, good job prospects, and strong attractiveness to labour. At this point, despite the huge real estate bubble, the attractiveness of urban infrastructure to the labour largely mitigates the crowding-out effect of housing prices on the labour. Third, cities of the middle group are often too choosy to fulfil labourers’ wishes. In the embarrassing situation, labourers are sensitive to changes in urban housing prices.
5.2. Heterogeneity analysis based on the industrial structure differences
The industrial structure is the main component of the socio-economic system. The historical development sequence of social production activities can be divided into three parts. We analyse the heterogeneity of rising urban housing prices on labour mobility in different industry structures. The estimated results are reported in .
Table 11. Heterogeneity based on the industrial structure differences.
reports the impact of urban housing prices on labour mobility and heterogeneity in different industry structures. The impact of rising urban housing prices on labour intensity is lower in the primary industry and higher in the tertiary industry. It shows that urban housing prices have different labour crowding-out effect intensity in different industrial structures. The majority of the primary industry labourers who own their own homes have a low demand for commercial housing. The tertiary industry labourers are more flexible in their choice of space, have greater population mobility, and are more sensitive to changes in housing prices than those who are secondary industry labourers.
Based on the above analysis, the last hypothesis, H3 is accepted too.
6. Conclusions and implications
On the basis of the existing theories and literature, we empirically test the impact of rising urban housing prices on labour mobility and its moderating effect. In addition, we further examine whether this effect is heterogeneous across cities with different housing price-to-income ratio and industrial structure. This article addresses endogeneity using a panel model with fixed-effect model based on 2002–2018 panel data in 281 cities in China and identifies housing price jumps as instrumental variables.
First, the rising urban housing prices has a significant crowding-out effect on labour mobility. Considering that both urban housing prices and labour mobility reflect the upward trend of the economy. The endogeneity is solved by constructing instrumental variables to identify the housing prices increase in the provinces where the sample cities are located.
Second, the three variables of education level, urban construction level, and income level respectively play moderating effect of urban housing prices on labour mobility. The improvement of the three variables can alleviate the crowding-out effect. When labourers make employment decisions, the influence of urban ‘comfort’ characteristics such as education resources, urban construction level and income level becomes increasingly significant.
Third, the heterogeneity of the impact of urban housing prices on labour mobility is reflected in both housing price-to-income ratio and the industry structure. On the one hand, the research indicates an inverted U-shaped curve between the intensity of the crowding-out effect of urban housing prices on labour and the housing price-to-income ratio. On the other hand, rising urban housing prices on labour intensity are lower in the primary industry and higher in the tertiary industry. At present, few studies have been conducted from the perspective of housing price-to-income ratio. Therefore, it is essential to formulate a scientific and sound management policy based on different housing price-to-income ratio and industry structure.
Disclosure statement
No potential conflict of interest was declared by the authors.
Additional information
Funding
References
- Albouy, D. (2016). What are cities worth? Land rents, local productivity, and the total value of amenities. Review of Economics and Statistics, 98(3), 477–487. https://doi.org/10.1162/REST_a_00550
- Bayer, P., Ferreira, F., & McMillan, R. (2007). A unified framework for measuring preferences for schools and neighborhoods. Journal of Political Economy, 115(4), 588–638. https://doi.org/10.1086/522381
- Bayoh, I., Irwin, E. G., & Haab, T. (2006). Determinants of residential location choice: How important are local public goods in attracting homeowners to central city locations? Journal of Regional Science, 46(1), 97–120. https://doi.org/10.1111/j.0022-4146.2006.00434.x
- Benjamin, B. (1970). Principles of demography, by Donald J. Bogue. Journal of the Royal Statistical Society. Series D (the Statistician), 19(4), 410–411. https://doi.org/10.2307/2987376[Mismatch
- Carmona, J., Lampe, M., & Roses, J. (2017). Housing affordability during the urban transition in Spain. The Economic History Review, 70(2), 632–658. https://doi.org/10.1111/ehr.12418
- Carruthers, J., & Mulligan, G. F. (2012). The plane of living and the precrisis evolution of housing values in the USA. Journal of Economic Geography, 12(4), 739–773. https://doi.org/10.1093/jeg/lbr045
- Charles, K. K., Hurst, E., & Notowidigdo, M. J. (2018). Housing booms and busts, labor market opportunities, and college attendance. American Economic Review, 108(10), 2947–2994. https://doi.org/10.1257/aer.20151604
- Chiang, T. C. (2020). Economic policy uncertainty and stock returns – Evidence from the Japanese market. Quantitative Finance and Economics, 4(3), 430–458. https://doi.org/10.3934/qfe.2020020
- Di Maggio, M., Kermani, A., Keys, B. J., Piskorski, T., Ramcharan, R., Seru, A., & Yao, V. (2017). Interest rate pass-through: Mortgage rates, household consumption, and voluntary deleveraging. American Economic Review, 107(11), 3550–3588. https://doi.org/10.1257/aer.20141313
- Diamond, R. (2016). The determinants and welfare implications of US workers' diverging location choices by skill: 1980-2000. American Economic Review, 106(3), 479–524. https://doi.org/10.1257/aer.20131706
- Disney, R., & Gathergood, J. (2018). House prices, wealth effects and labour supply. Economica, 85(339), 449–478. https://doi.org/10.1111/ecca.12253
- Favilukis, J., Ludvigson, S. C., & Van Nieuwerburgh, S. (2017). The macroeconomic effects of housing wealth, housing finance, and limited risk sharing in general equilibrium. Journal of Political Economy, 125(1), 140–223. https://doi.org/10.1086/689606
- Foote, A. (2016). The effects of negative house price changes on migration: Evidence across US housing downturns. Regional Science and Urban Economics, 60, 292–299. https://doi.org/10.1016/j.regsciurbeco.2016.08.001
- Ganong, P., & Shoag, D. (2017). Why has regional income convergence in the US declined? Journal of Urban Economics, 102, 76–90. https://doi.org/10.1016/j.jue.2017.07.002
- Garretsen, H., & Marlet, G. (2017). Amenities and the attraction of Dutch cities. Regional Studies, 51(5), 724–736. https://doi.org/10.1080/00343404.2015.1135239
- Gorelick, J., & Walmsley, N. (2020). The greening of municipal infrastructure investments: Technical assistance, instruments, and city champions. Green Finance, 2(2), 114–134. https://doi.org/10.3934/GF.2020007
- Guerrieri, V., Hartley, D., & Hurst, E. (2013). Endogenous gentrification and housing price dynamics. Journal of Public Economics, 100, 45–60. https://doi.org/10.1016/j.jpubeco.2013.02.001
- Gyourko, J., Mayer, C., & Sinai, T. (2013). Superstar cities. American Economic Journal: Economic Policy, 5(4), 167–199. https://doi.org/10.1257/pol.5.4.167
- Hämäläinen, K., & Böckerman, P. (2004). Regional labor market dynamics, housing, and migration. Journal of Regional Science, 44(3), 543–568. https://doi.org/10.1111/j.0022-4146.2004.00348.x
- Han, B., Han, L., & Zhu, G. Z. (2018). Housing price and fundamentals in a transition economy: The case of the Beijing market. International Economic Review, 59(3), 1653–1677. https://doi.org/10.1111/iere.12316
- Haviernikova, K., & Kordos, M. (2019). The SMEs' perception of financial risks in the context of cluster cooperation. Quantitative Finance and Economics, 3(3), 586–607. https://doi.org/10.3934/qfe.2019.3.586
- Hincks, S. (2012). Daily interaction of housing and labour markets in North West England. Regional Studies, 46(1), 83–104. https://doi.org/10.1080/00343404.2010.486782
- Hsieh, C. T., & Moretti, E. (2019). Housing constraints and spatial misallocation. American Economic Journal: Macroeconomics, 11(2), 1–39. https://doi.org/10.1257/mac.20170388
- Imbens, G., & Kalyanaraman, K. (2012). Optimal bandwidth choice for the regression discontinuity estimator. The Review of Economic Studies, 79(3), 933–959. https://doi.org/10.1093/restud/rdr043
- Jeanty, P. W., Partridge, M., & Irwin, E. (2010). Estimation of a spatial simultaneous equation model of population migration and housing price dynamics. Regional Science and Urban Economics, 40(5), 343–352. https://doi.org/10.1016/j.regsciurbeco.2010.01.002
- Jia, S. S., Qiu, Y. S., & Yang, C. Y. (2021). Sustainable development goals, financial inclusion, and grain security efficiency. Agronomy, 11(12), 2542. https://doi.org/10.3390/agronomy11122542
- Kwakwa, P. A., Adusah-Poku, F., & Adjei-Mantey, K. (2021). Towards the attainment of sustainable development goal 7: What determines clean energy accessibility in sub-Saharan Africa? Green Finance, 3(3), 268–286. https://doi.org/10.3934/GF.2021014
- Lee, D. S., & Lemieux, T. (2010). Regression discontinuity designs in economics. Journal of Economic Literature, 48(2), 281–355. https://doi.org/10.1257/jel.48.2.281
- Li, F., Yang, C., Li, Z., & Failler, P. (2021). Does geopolitics have an impact on energy trade? Empirical research on emerging countries. Sustainability, 13(9), 5199. https://doi.org/10.3390/su13095199
- Li, L. H., Wu, F., Dai, M. J., Gao, Y. M., & Pan, J. Y. (2017). Housing affordability of university graduates in Guangzhou. Habitat International, 67, 137–147. https://doi.org/10.1016/j.habitatint.2017.07.007
- Li, Z. H., Huang, Z. H., & Dong, H. (2019). The influential factors on outward foreign direct investment: Evidence from the “The Belt and Road”. Emerging Markets Finance and Trade, 55(14), 3211–3226. https://doi.org/10.1080/1540496X.2019.1569512
- Li, Z. H., & Zhong, J. H. (2020). Impact of economic policy uncertainty shocks on China's financial conditions. Finance Research Letters, 35, 101303. https://doi.org/10.1016/j.frl.2019.101303
- Li, Z. H., Zou, F. Q., & Mo, B. (2021). Does mandatory CSR disclosure affect enterprise total factor productivity? Economic Research-Ekonomska Istraživanja, 34, 1–20. https://doi.org/10.1080/1331677X.2021.2019596
- Li, Z. H., Zou, F. Q., Tan, Y., & Zhu, J. H. (2021). Does financial excess support land urbanization – An empirical study of cities in China. Land, 10(6), 635. https://doi.org/10.3390/land10060635
- Liu, Z. Q. (2007). The external returns to education: Evidence from Chinese cities. Journal of Urban Economics, 61(3), 542–564. https://doi.org/10.1016/j.jue.2006.08.007
- Matei, I. (2020). Is financial development good for economic growth? Empirical insights from emerging European countries. Quantitative Finance and Economics, 4(4), 653–678. https://doi.org/10.3934/QFE.2020030
- Mayer, C. (2011). Housing bubbles: A survey. Annual Review of Economics, 3, 559–577. https://doi.org/10.1146/annurev.economics.012809.103822
- Moretti, E. (2013). Real wage inequality. American Economic Journal-Applied Economics, 5(1), 65–103. https://doi.org/10.1257/app.5.1.65
- Muellbauer, J., & Murphy, A. (2008). Housing markets and the economy: The assessment. Oxford Review of Economic Policy, 24(1), 1–33. https://doi.org/10.1093/oxrep/grn011
- Osland, L., & Thorsen, I. (2013). Spatial impacts, local labour market characteristics and housing prices. Urban Studies, 50(10), 2063–2083. https://doi.org/10.1177/0042098012474699
- Peng, C. W., & Tsai, I. C. (2019). The long- and short-run influences of housing prices on migration. Cities, 93, 253–262. https://doi.org/10.1016/j.cities.2019.05.011
- Plantinga, A. J., Detang-Dessendre, C., Hunt, G. L., & Piguet, V. (2013). Housing prices and inter-urban migration. Regional Science and Urban Economics, 43(2), 296–306. https://doi.org/10.1016/j.regsciurbeco.2012.07.009
- Salim, R., Hassan, K., & Rahman, S. (2020). Impact of R&D expenditures, rainfall and temperature variations in agricultural productivity: Empirical evidence from Bangladesh. Applied Economics, 52(27), 2977–2990. https://doi.org/10.1080/00036846.2019.1697422
- Shen, J. F. (2012). Changing patterns and determinants of interprovincial migration in China 1985-2000. Population, Space and Place, 18(3), 384–402. https://doi.org/10.1002/psp.668
- So, K. S., Orazem, P. F., & Otto, D. M. (2001). The effects of housing prices, wages, and commuting time on joint residential and job location choices. American Journal of Agricultural Economics, 83(4), 1036–1048. https://doi.org/10.1111/0002-9092.00228
- Sorens, J. (2018). The effects of housing supply restrictions on partisan geography. Political Geography, 66, 44–56. https://doi.org/10.1016/j.polgeo.2018.07.007
- Su, Y., Li, Z., & Yang, C. (2021). Spatial interaction spillover effects between digital financial technology and urban ecological efficiency in China: An empirical study based on spatial simultaneous equations. International Journal of Environmental Research and Public Health, 18(16), 8535. https://doi.org/10.3390/ijerph18168535
- Waights, S. (2019). The preservation of historic districtsis it worth it? Journal of Economic Geography, 19(2), 433–464. https://doi.org/10.1093/jeg/lby002
- Yang, C., Li, T., & Albitar, K. (2021). Does energy efficiency affect ambient PM2.5? The moderating role of energy investment. Frontiers in Environmental Science, 9(210), Article no. 707751. https://doi.org/10.3389/fenvs.2021.707751
- Yang, K., Yi, J. Z., Chen, A. B., Liu, J. Q., & Chen, W. J. (2022). ConDinet++: Full-scale fusion network based on conditional dilated convolution to extract roads from remote sensing images. IEEE Geoscience and Remote Sensing Letters, 19, 1–5. https://doi.org/10.1109/LGRS.2021.3093101
- Yang, Z. S., & Pan, Y. H. (2020). Human capital, housing prices, and regional economic development: Will "vying for talent" through policy succeed? Cities, 98, 102577. https://doi.org/10.1016/j.cities.2019.102577
- Yao, Y., Hu, D., Yang, C., & Tan, Y. (2021). The impact and mechanism of fintech on green total factor productivity. Green Finance, 3(2), 198–221. https://doi.org/10.3934/GF.2021011
- Zang, B., Lv, P., & Warren, C. M. J. (2015). Housing prices, rural-urban migrants' settlement decisions and their regional differences in China. Habitat International, 50, 149–159. https://doi.org/10.1016/j.habitatint.2015.08.003
- Zheng, S. Q., Fu, Y. M., & Liu, H. Y. (2009). Demand for urban quality of living in China: Evolution in compensating land-rent and wage-rate differentials. The Journal of Real Estate Finance and Economics, 38(3), 194–213. https://doi.org/10.1007/s11146-008-9152-0
- Zhou, Q., Shao, Q. L., Zhang, X. L., & Chen, J. (2020). Do housing prices promote total factor productivity? Evidence from spatial panel data models in explaining the mediating role of population density. Land Use Policy, 91, 104410. https://doi.org/10.1016/j.landusepol.2019.104410
- Zhu, N. (2002). The impacts of income gaps on migration decisions in China. China Economic Review, 13(2-3), 213–230. https://doi.org/10.1016/S1043-951X(02)00074-3