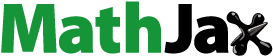
Abstract
This study examines the impact of the COVID-19 pandemic on the livelihood and economic survival of Thai citizen workers, using The Asia Foundation’s survey data which were conducted in May 2020 (first round), August 2020 (second round) and November 2020 (third round). We adopt the Cox proportional-hazards regression with lasso estimation to estimate the coefficients and perform variable selection simultaneously. The model allows us to identify the vulnerable groups with risks of consumption inadequacy. The empirical results show that those workers characterized as low-educated, unemployed, unskilled, working in the tourism sector and living in the northeastern or southern regions are less likely to sustain their consumption. However, our study highlights that higher education is a crucial factor influencing the survivability of Thai workers. Regarding the role of government schemes, the result shows that that a set of cash assistance programs is less likely to increase the survivability of the non-agricultural workers.
1. Introduction
The presence of the COVID-19 pandemic has contributed to a substantial negative impact on employment in many countries (Posel et al., Citation2021). Thailand is no exception. The service sector experienced the employment shock earlier than other sectors and experienced a sudden drop in the number of workers. International tourism demand decreased significantly, causing a severe management crisis in the service sector.Moreover, the government-imposed lockdown measures and the consumer behavioural changes have caused a sizable slowdown in economic activity. As a result, millions of tourism-related and manufacturing workers in Thailand became unemployed (Komin et al., Citation2020). The International Labour Organisation (2020) confirmed that 2.2 million workers lost their jobs from January to March 2020, following the sizeable decrease in tourist inbounds, the slowdown in the flow of goods and services in global supply chains, the wide-scale suspension of economic activity, as well as the lockdown policy. Since the Thai government declared a state of emergency, the several COVID-19 lockdowns and partial lockdown events caused unemployment to rise to 745,176 people in the second quarter of 2020. This doubled the number of unemployed people in the previous quarter. The National Statistical Office of Thailand reported that the unemployment rate has increased to 2 percent in the second quarter of 2020, which is more than three times the unemployment rate for the last decade. The Bank of Thailand (Citation2020) reported that the number of hours worked in Thailand declined by 6 percent, and 5,411,407 people worked less than 4 hours per day in this second quarter. This high unemployment phenomenon has been significantly exacerbated by the consumption problem and thereby leading to the low survivability of workers (Antipova, Citation2021).
As COVID-19 has directly affected workers’ career and financial situations, some workers made adjustments by moving back to their hometown, borrowing more money, and participating in career/professional skill training aiming to alleviate the immediate and medium-term difficulties. On the other hand, the Thai government has also launched a set of COVID-19 relief measures to alleviate the impact of COVID-19 on workers and stimulate the economy. There is however substantial heterogeneity in the survivability across workers. This is due to the COVID-19 impact differs depending on worker and job characteristics, and partly due to government schemes to mitigate the impact may benefit some but not others (Crossley et al., Citation2021).
The spread of COVID-19 has created a survival problem for most workers regardless of the types of their jobs and characteristics (Kikuchi et al., Citation2021). Therefore, determining the survival factors of workers is vital as it provides the basis for formulating policies and strategies that improve the survivability of workers. However, there are few comprehensive studies, particularly survival analysis, that identify the factors influencing the survival of worker, especially in Thailand. Thus, the existed knowledge gap and the focus for the survival of worker against COVID-10 motivated us to empirically investigate the factors associated with the risk of survivability of the worker in Thailand.
In this regard, this study considers the survival analysis of workers and adopts the Cox proportional-hazards regression model (Cox, Citation1992, together with Kaplane Meier estimator, to identify the vulnerable workers who cannot sustain their consumption in the one year after the COVID-19 outbreak. This model is used to analyze this study’s survey data by focusing on the factors that supported or hindered the survivability of the Thai workers during this COVID-19 crisis. Our results will assist the government in exploring the correct treatments and devising an appropriate policy to increase the strength and survival of Thai workers. We want to note that his/her self-assessment measures the survivability of individual worker. Each worker is asked to answer the question of ‘If Thailand faces COVID-19 for another year, how long do you think you can last, given your income, savings, and all the food that you have now?’ To answer this question, each worker is given a range of choices from one day to one year, and this answer is used to determine the respondent’s survivability. Also note that, this study involves an econometrics model, and the term ‘survivability’ does not concern health or mortality resulted from the COVID-19 pandemic.
This study is novel and contributes to the existing literature in two ways: firstly, this is the first attempt of providing the empirical evidence regarding the risk factors of the worker’s survivability in Thailand. secondly, Cox proportional hazards model is relatively standard in the literature on survival analysis and has been applied in many fields such as medical study (e.g., Salinas-Escudero et al., Citation2020), financial study (e.g., Gunsel, Citation2010; Shih & Giles, Citation2009), and economic study (e.g., Danacica & Babucea, Citation2010; Puttachai et al., Citation2019), it has been rarely applied to worker’s survivability. Thus, this study supplements existing literature on the value of survival analysis by applying the Cox proportional hazards model and Kaplane Meier estimator to estimate the probability of worker’s survivability in each period.
This paper is organized to contain five parts. After the introduction, Section 2, begins with the relevant literature. The methodology and the detail about our data and population inferences from survey data are introduced in Section 3. Section 4 presents the results and discussion. Finally, the conclusion is discussed in the last section.
2. Literature review
The effects of COVID-19 on the survivability of workers are hardly uniform across heterogenous workers (Crossley et al., Citation2021). As recently revealed by Crossley et al. (Citation2021), the economic impact of the COVID-19 crisis is very different for different workers depending on individual worker’s characteristics and government subsidies. Al-Youbi et al. (Citation2020) mentioned that the impact of this crisis on workers does not have the same pattern, as knowledge and flexibility to work from each worker’s home play an essential role in their employment opportunities. Therefore, the impact of COVID-19 on Thai workers may differ across socio-economic groups of workers, and careful analysis should be undertaken to define who are the most immune to COVID-19 shocks (high survivability) and the most vulnerable to COVID-19 (low survivability).
This study contributes to the fast-growing literature focusing on the impacts of COVID-19 on workers and the roles of government’s relief schemes to alleviate the impacts of COVID-19 on workers’ survival ability. In the literature review, it is evident that numerous studies have been carried out all over the world to determine the impacts of COVID-19 on the workers. For example, Adams-Prassl et al. (Citation2020) studied the impact of COVID-19 shock on workers of Germany, the USA, and the UK. They reported that workers with temporary contracts and less-educated workers are more affected by the crisis. Belot et al. (Citation2021) reported large effects of COVID-19 on young people in China, South Korea, Japan, Italy, the UK, and the USA. Aum et al. (Citation2021) investigated the impact of COVID-19 on local employment in Korea and revealed that the workers, who are less educated, young, in low-wage occupations, and on temporary contracts, experienced a highly vulnerability to the adverse economic effect of COVID-19. Similar findings are obtained for the case of Japan’s workers. Kikuchi et al. (Citation2021) stated that temporary workers are hit harder than permanent workers, females than males, younger than older, and workers engaged in social and non-flexible jobs than those in ordinary and flexible jobs. Furthermore, Crossley et al. (Citation2021) ascertained that the COVID-19 impact is bigger for the minority ethnic groups. These studies have shown which socio-economic characteristics of workers made them more vulnerable to the shocks from crisis. Another aspect that lacks clarity is technology adoption. Narayanamurthy and Tortorella (Citation2021) examined the role of COVID-19’s work implications on the performance of Indian workers and found that home office work environment, job insecurity, and virtual connection contribute to moderate enhancement in workers. The impact of COVID-19 shocks on the workers has also been investigated in Saudi Arabia Al-Youbi et al. (Citation2020), Korea (Jung et al., Citation2021), Bangladesh (Karim et al., Citation2020) and United Kingdom (Jones, Citation2022), and OECD (Picatoste et al., Citation2021).
From the above literature, we can observe that research on COVID-19’s impact on workers has been conducted in many countries. However, the investigation of the COVID-19’s impact on workers in Thailand is quite limited. Our study is closely related to that of Komin et al. (Citation2020), who studied the Covid-19 impact on informal workers in Thailand. However, there is an essential difference in that we emphasize both formal and informal workers during this crisis. Besides the scope issue, this study also highlights how socio-economic and government scheme impacts survival probability of Thai workers during this crisis. Acquiring important insights from workers enhances the applicability of the present study and provides more reliability to its finding in assessing the survivability of workers at any time and defining the risk factors to suggest suitable government relief schemes. Note again that the term ‘survivability’ applies to how long an individual worker believes his/her resources will last if the pandemic continues. In other words, the survivability of a worker is an estimate of how much longer he/she could keep going, under present conditions, before running out of money and having to cut back on consumption.
3. Methodology
3.1. Model
To define what factors may affect the survival of workers, the basic concept of the Cox model is described. The model takes the form as
(1)
(1)
or, equivalently
(2)
(2)
where
is the survival time,
is an unspecified baseline hazard function,
is the vector of unknown coefficients, and
is the vector of factors affecting the survival probability of the Thai workers. In this equation,
is the hazard function, which assesses the probability that the event of interest (in this case, consumption failure or non-survival) occurred before time
given
thus, if
the hazard function increases, which means that the survival duration of Thai workers will decrease.
We use the Lasso method of Tibshirani (Citation1997), that allows variable selection and parameter estimation to be performed simultaneously, to estimate the Cox model. This is because our study considered a multitude of socio-economic and government relief scheme variables and the Lasso technique is reliable for estimating high-dimensional survival data. By using this estimator, not all the factors may contribute to the prediction of the survival of workers: as some coefficients may be zero in our survival model result.
Following Tibshirani (Citation1997) and Wang et al. (Citation2019), we can maximize the penalized log partial likelihood function to obtain the estimated parameters This function can be written as
(3)
(3)
where
is the censoring indicator, whereas
and
are, respectively, the failure time and censoring time of worker
(in this study,
days).
is the tuning parameter.
To have a better understanding of the event of interest, we illustrate the logic behind our survival analysis in which shows the features of variables for the survival data in this study. When the study ends, the individual I still had not been affected by the event yet, or it is the case of survival from the COVID-19 crisis without consumption failure, while the others (II and II) experienced the non-survival in this crisis with consumption failure.
3.2. Data collection
This study uses the survey data collected as a part of the Revisiting the Pandemic Project of the Asia Foundation to investigate the impact of COVID-19 on the livelihoods of Thai workers. The survey examines the COVID-19 impacts on the employment, economic situations, and behavioural adjustments of Thai workers. The Asia Foundation collected two main sets of survey of the COVID-19 impacts, which are (1) impacts on Thai workers and (2) impacts on Thai micro and small enterprises. This study uses the Thai workers dataset. The survey is a panel-data survey (the same participants are tracked), collecting three rounds of data in June (Round 1), September (Round 2), and November (Round 3) of 2020. These three surveys cover a total of 3,181 individuals. This sample was collected from Bangkok and all four major regions in Thailand, consisting of the North, Northeast, Central, and South. Over the three survey rounds, the sampling methodology closely matched the population’s distribution in Thailand, with an average sample of 13.84% in Bangkok, 31.07% in the Central Region, 16.4% in the North, 25% in the Northeast, and 13.72% in the South. The data are collected from Thai citizen aged 15 years and over who reported having engaged in any paid work during the previous 12 months. The sample might be employed, unemployed and outside of the labor force at the time of survey. In addition, both formal and informal workers were considered in this survey.
For the issue of economic survival duration of Thai workers, the survey asked, ‘If Thai society still faces the COVID-19 situation for another year, how long do you think you can last, given your income, savings or all the food you have now?’. The choices provided are that the respondents would (1) have enough for living for a year or more with no trouble and (2) be in difficulty. If the respondents indicated that they had difficulty, they are then asked how many months or many days that they can support their consumption. To identify factors relating to the economic survival duration, the explanatory variables considered include workers’ demographic background, work characteristics, behavioural adjustments, and policy variables. The details of the variables are shown in . This table shows continuous variables using average and standard deviation and categorical variables as numbers and percentage across the three rounds.
Table 1. Data description for three rounds.
We observe that Workers’ demographics, work characteristics, and behavioural adjustments during COVID-19. Demographic variables remained unchanged because the same respondents were asked in all three rounds, except for the income variable. For our outcome variables, consumption failure and economic survival duration are quite difference across the three rounds. From round 1 to round 3, the percentage of workers who face consumption failure or non-survival from this crisis decreases from 62.4% to 52.0%, corresponding to the economic survival duration or the number of survival days that increases from 186.76 days to 221.85 days. For the case of social protection policies categorical variables, we found that most of the workers do not receive any assistance from the government. This means that the social protection policies are quite limited in some groups of workers in Thailand.
In sum, five categories: demographics, characteristics, behavioural adjustments, and social protection policies related to Thai workers are considered as the factors affecting the survival probability of workers. Note that the survival probability of workers is the outcome variable, which is time to consumption failure (non-survival) constructed as the time between the first day that participant is asked and consumption failure with censoring on day 365.
4. Empirical results
4.1. Lasso-Cox regression analysis
We then estimate the Lasso-Cox regression model to identify factors associated with the hazard ratio or, in this case, the probability that a worker would face consumption failure in a given time. It should be emphasized that the model only identifies the association of the factors and does not imply causation (Salinas-Escudero et al., Citation2020).
displays the results for the statistically significant variables selected by the lasso estimation. Note that these estimated coefficients cannot be directly interpreted as Cox being a non-linear model; thus, the effect of independent variables on consumption failure differs from individual to individual. Furthermore, the estimated coefficients can be interpreted as the effect of independent variables on the log-odd of consumption failure, not on the probability. Therefore, we consider the hazard ratio, for our interpretation. If the
> 1, it indicates that the worker has a shorter survival time than the control or reference group, and if it is < 1, it indicates that the worker is likely to have a longer time to survive than the reference group. The ratio does not quantify the magnitude of the difference (Kavkler et al., Citation2009).
Table 2. Estimation results in the three rounds.
In all three rounds, the selected variables are gender, edu_bachelor, edu_hbachelor, occu_unskilled, inc_ch_dec, borrow, indicating that these variables are the key risk factors affecting the survival of Thai workers. Overall, we observe that gender, occu_unskilled, inc_ch_dec, and borrow coefficients are positive indicating that these factors worsen the survival probability, while edu_bachelor and edu_hbachelor are positive meaning that workers with higher education are less affected than those with low education level. Furthermore, compared to those with primary education or lower, workers with a Bachelor’s degree or higher face a significantly lower likelihood of having consumption failure in all three rounds. In contrast, workers with secondary education or diploma had similar levels of hazard ratio to those with primary education or lower or even had a slightly higher hazard ratio in some periods. This result is consistent with the findings of Al-Youbi et al. (Citation2020), who mentioned that the higher-educated workers (Bachelor’s degree or above), have quickly adjusted themselves from traditional to remote work, which is the most important skill required by the business during this COVID-19 crisis.
Moreover, we explore heterogeneity in consumption failure with respect to gender, age, and region. We find that the younger workers seem more likely to face consumption failure in the third round, while the older workers are likely to face consumption failure in the first two rounds. In terms of region, we observe that northeastern and southern workers are less likely to survive in the second and third rounds. This result is not surprising for the case of Thailand, since the pre-COVID period, the southern and northeastern regions have the highest and second-highest poverty rates in Thailand. In 2019, the poverty rate in the southern region is 11.27% and the rate in the northeastern region is 8.37%, which are relatively high comparing to the national rate of 6.24% (NESDC., Citation2019). With the COVID-19 situation, workers in these two regions might be more vulnerable. Finally, we find strong evidence that male workers are more likely to survive than females in all three rounds. Thus, female workers are more vulnerable than male workers. We observe that the hazard ratio of gender is 1.084 (exp(0.081), 1.066 (exp(0.081), and 1.152 (exp(0.142), for rounds 1-3, respectively. This means that female workers are 1.084, 1.066, and 1.152 times more likely to have the consumption failure in rounds 1-3, respectively. The possible reason is that female has more care responsibilities compared to male. Adams-Prassl et al. (Citation2020) suggested that women workers have to take care of their children and spend more time homeschooling and caring for children than males working from home. Thus, it is not easy for a female to have more survivability during this crisis. Besides, we also suggest that female workers appeared to be more vulnerable than male workers as the tourism and services sectors, which are the important segments of Thailand’s economy, hire more female workers than males; and the pandemic has hit women in these sectors harder than those in the agricultural and manufacturing sectors. International Labour Organisation (2020) reported that these two sectors are among the most vulnerable in the face of the demand and supply-side shocks associated with the COVID-19 crisis, thus bringing about millions of tourism-related and service-related jobs loss.
Next, we explore whether workers’ characteristics relate to differences in survival; we find that indus_publicsector and occu_govt play an important role in increasing the survival probability of workers in round 1; and rounds 2 and 3, respectively. This means that government workers are less likely to face a consumption failure in the second and third round compared to the first round, while public sector workers (responsible for providing all public services) are more likely to survive their livelihood only in the first round.
We also explore whether government protection policies relate to differences in survivability. We learn that government protection policy contributes a weak mitigation to Thai workers as there is only sp_type3 that shows a negative coefficient of −0.107 (hazard ratio is exp(-0.107)= 0.898) in the first round, indicating a protective effect of this variable on the survivability of Thai farmworkers. Specifically, receiving THB 5,000/month for 3 months reduces the hazard by a factor of 0.898, or 11.2%.
4.2. Factors associated with the economic survivability of Thai workers during COVID-19
To analyse the factors associated with economic survivability, we use the Kaplan-Meier estimator, which analyses the survival probability over time (Puttachai et al., 2019).
To illustrate the overview of the survival situation in 2020, we provide to show the economic survival probability or the ability for an individual to sustain his/her consumption in June (Round 1), September (Round 2), and November (Round 3). From the graph, most individuals can sustain their consumption for the first 30 days as the survival probabilities from the 1st day to the 30th day are close to 1. The survival probabilities then dropped every 30 days. Less than 50% of the sample (39.3% in June, 44.1% in September, and 49.8% in November 2020) had enough income or savings to sustain their consumption longer than six months. However, if they can last for six months, most can last longer than a year. In June 2020, people were less prepared, and 2.7% could afford to sustain consumption for less than a week. This phenomenon disappeared in September and November indicating that the most vulnerable groups became better prepared as the COVID-19 situation prolonged. Across the three rounds, Thai workers in the third round have been significantly more likely to be surviving rather than failing to survive when compared to the first two rounds. This is to be expected given that most of the workers are not allowed to do any work for their employers under the lockdown from mid-March until June 2020. Thus, the survival expectation of workers during the first two round surveys is low. However, in the last round of surveys during November 2020, workers have become immune to the COVID-shocks. It should be noted that the improvement of the survival situation in November (Round 3) could be due to the timing of the survey collecting period, which was right before the second wave of the COVID-19 pandemic starting in December 2020. Therefore, the improvement of the survival probability in November may not reflect the situation from December 2020 onward.
Figure 2. The overall economic survival probability of Thai workers over the three survey periods.
Source: authors' calculations.
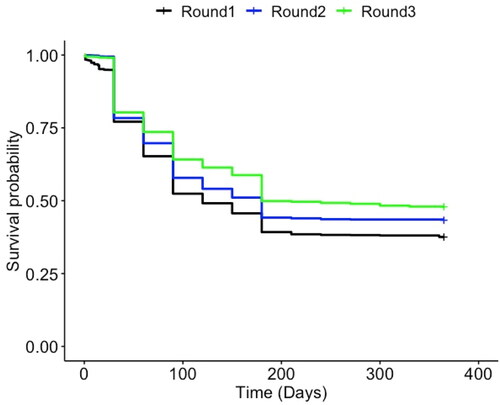
4.2.1. Economic survivability of workers with different demographics
For survivability across different demographics, the education of workers is the most significant factor. In , the survival function has a step or stair-shaped downward slope. We observe that the survival probability is 100% on the first day and then drops only slightly on the first ten-day. This indicates that the risk of the crisis is low in those periods; however, the graphs dropped significantly afterward for all education levels, which means that the risk increases over time. After day 180, the survival function ends in a horizontal line in all rounds, indicating that the survival probability turns constant after 180 days. The survival probabilities of workers differ across workers with different education levels. It is observed that the workers with higher education (Bachelor’s degree or higher) have higher survival rates. However, even workers with a master’s degree or Ph.D. have a survival probability lower than 75%. This indicates that COVID-19 affects all groups, and some workers with higher education also can have difficulty sustaining their consumption. Workers with primary, secondary, and diploma education face lower survival probability. In the first round, workers with a diploma have a survival rate of approximately 50%, which is higher than 30% of workers with high school or lower. In the second and third rounds, the survival rate is quite similar for workers with a diploma, primary school, or lower, with a survival probability below 50% after day 180.
Figure 3. Survival probability of workers in different education levels over the three survey periods.
Source: authors' calculations.
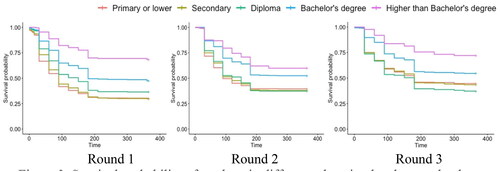
In addition to education, female workers and adult workers aged 15 to 59 faced a higher consumption risk. shows that female workers have a significantly lower survival probability than male workers in all three rounds of the survey. This may be because female workers are more likely to be in a less stable job, such as a part-time job. In addition to the potential differences in job situations of male and female workers, this result could also partially be due to family factors, as females are more likely to have more family responsibilities. We can observe that the survival path of female workers is lower than that of the male workers in all three periods; however, the survival probabilities of males and females seem to be stable after day 180 with the probability around 45-50%, indicating that almost 50% of male and female workers can survive more than one year during this crisis period.
Figure 4. Survival probability of workers in different gender over the three survey periods.
Source: authors' calculations.
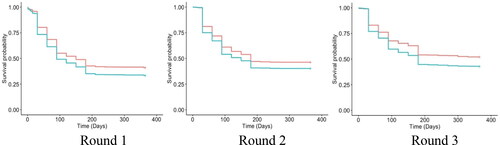
In the case of a vulnerable older worker, shows that he/she presents a higher chance of survival compared to the middle-aged and young workers as most older workers are not the head of the household and do not need to take responsibility for their family after transferring it to their child. Also, the older worker has received an old-age pension since 60 years old, and we expect that old-age pension is an effective vehicle for older worker emergency relief during the COVID-19 pandemic. Our result is consistent to Koczan (Citation2022) who revealed that job losses also appear to be more concentrated among the young than the older workers in both emerging and advanced economies. However, the survival probability of older workers will drop if COVID-19 remains going on. We observe the survival probability among workers decreases rapidly after day 20 and 180, but the probability remains constant after that.
Figure 5. Survival probability of workers in different age groups over the three survey periods.
Source: authors' calculations.
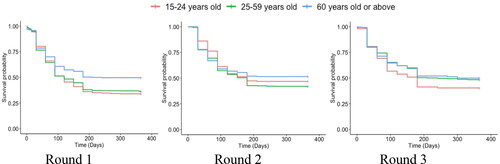
For regions, shows that the probability of survival in the third round is higher than the other two rounds. Besides, we observe that Bangkok worker has the highest survival probability compared to other regions. In the northeastern and southern regions, on the other hand, workers are less likely to sustain their consumption. We also observe an interesting result obtained from the survival paths of Bangkok workers during these three rounds. The survival probability among Bangkok, central and northern workers has increased along the passage of time from rounds 1 − 3 of the survey, implying that these workers have become more confident of surviving from this crisis. In contrast, the survival probabilities of southern and northeastern workers are likely to be stable throughout the three rounds.
4.2.2. Economic survivability of workers with different work characteristics
By work characteristics, temporary employees, unskilled workers, and those whose income is reduced due to COVID-19 faced a significantly higher hazard ratio in all three rounds. In terms of occupational type, shows that the survival probabilities are quite different across occupations. It is observed that the retired-workers with pension have higher survival rates most of the time. General contractors or unskilled laborers are significantly more likely to have consumption failure than people outside the labor force in all rounds. Government officials, government employees, state enterprise employees, and conscripts are the least affected by COVID-19. The unemployed individuals are also at the highest risk, although the coefficient is not statistically significant in round 1. This is due to the small sample of the unemployed individuals (0.16% of all respondents) in round 1. The unemployed became more prevalent in rounds 2 and 3 (5.02% and 4.10% of all respondents).
Figure 7. Survival probability of workers in different occupational groups over the three survey periods.
Source: authors' calculations.
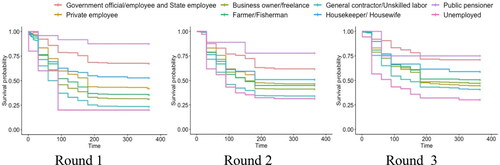
The industry in which the workers are employed also has something to do with the risk of consumption failure. Specifically, workers in the tourism and other service sectors are more likely to face consumption failure. The insignificance of the tourism coefficients in several rounds is potentially due to the heterogeneity in the sector. Workers in the tourism sector range from employees in large hotels to small street vendors and, thus, faced different levels of risk. Nevertheless, shows that workers in the tourism sector are affected by COVID-19 the most on average. This result is expected as the COVID-19 situation stopped international tourists and lowered the number of domestic tourists. For other industries, workers in the public sector are least affected by COVID-19. Finally, workers in the agricultural and industrial sectors’ consumption risks are not significantly different from those outside the labor force. In addition, we can see that the survival probability of workers in the tourism sector started to be significantly lower than that of individuals outside the labor force on the 60th day, and the gap continues increasing over the survival time. On the other hand, workers in the public sector have the highest survival probability from the 30th day onward in all survey rounds.
Figure 8. Survival probability of workers in different industries over the three survey periods.
Source: authors' calculations.
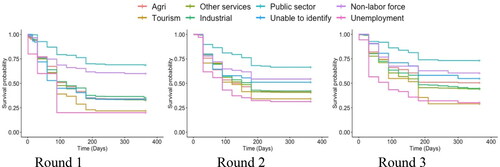
In addition to the factors of occupation and industry, the pre-COVID income is also a matter associated with consumption risk. Workers with a higher pre-COVID income are less likely to have consumption failure. Moreover, workers whose income was reduced due to COVID-19 faced a significantly higher hazard ratio. presents the impact of income status on the survival probability of workers. If the income level is stable during this COVID-19 crisis, it is evident that the survival probability is high, especially in the first round. Not surprisingly, when the income of worker decreases, the survival probability decreases. However, we can see a 25-30% chance that all workers can survive.
4.2.3. Behavioral adjustment during COVID-19
On the behavioral adjustments during the COVID-19 crisis, this study found 85.8% of workers borrowed additional loans, 2.5% of workers went back to their hometown, and 6.3% of workers participated in at least one career/professional skill training. Borrowing additional loans is significantly associated with the consumption risk. This indicates that most workers went deeper into debt during COVID-19. It also implies that Thai workers had some access to loans during the pandemic. In , we observe that the worker, who does not borrow money, has a higher chance of survival than the worker who makes borrowing in all rounds. This indicates that the loan does not assist Thai workers during the COVID-19 crisis. Going back to hometown and participating in training do not have a significant association with the consumption risk. This does not imply that the workers did not benefit from moving back or the training. The insignificance may be due to the selection bias, as workers who lost employment are more likely to move back or join the training. Moreover, the benefits of the adjustments may also be realized in a longer-term period.
4.2.4. Social protection policies
During the beginning stage of the COVID-19 crisis, the government launched three main social protection policies targeting three different groups of workers. Type 1 policy is the THB 5,000/month (3 months) cash assistance for non-government workers not covered by social security fund and not registered as a farmer, which is designed to help informal workers. Type 2 policy is the severance pay from laying off or business closure from Social Security Fund, which is designed to help formal private employees. Type 3 policy is the THB 5,000/month (3 months) cash assistance for farmers, which is designed to help farmers. Note that all three policies were available only for rounds 1 and 2 of the survey but not for round 3. presents that all policies cannot eliminate all workers’ risks to achieve sustain consumption as the survival function has a stair-shaped downward slope.
4.3. Discussion
The overall results indicate that the impacts of the COVID-19 crisis on Thai workers (both informal and formal) vary across workers of different genders, age groups, occupations, education levels, and industries.These results coincide with the recent observations made by Kikuchi et al. (Citation2021) that there is a heterogeneous response in workers to the COVID-19 economic impact in Japan. Moreover, the causal patterns we obtained across the three rounds of survey tell us that the unskilled workers with low socio-economic status and income were more likely to face consumption failure within 365 days. We also discovered that female workers had a lower chance of survival than male workers in all rounds. This result is consistent with the findings of Adams-Prassl et al. (Citation2020); Aum et al. (Citation2021); and Crossley et al. (Citation2021), who reported that increased childcare needs at home contributed to a rise in unemployment of females and thereby reducing the probability of surviving during the crisis. Moreover, in Thailand’s case, most female workers are employed in the tourism and services sectors, which are directly hit by the pandemic. In terms of age, we found that middle-aged workers were less likely to survive during the first two rounds, while the young workers were significantly more impacted in the last survey period. This is due to the part-time and full-time jobs for young workers were more limited in the last round of the survey. However, when we compared all age groups (including the elderly group aged 60 or above) using the survival analysis path, as shown in , the elderly workers had a higher survival probability in all three periods. This is surprising, so we assume that it is because the elderly workers have a reliable stream of income from non-work-related sources, such as a civil service pension, a social security pension, or the universal old-age allowance. Furthermore, our analysis revealed that workers in the Northeast and the South suffered more than those in other regions. This is not surprising due to the fact that the Northeast is the poorest region and the South is the most tourism-dependent region in Thailand.
Apart from socio-economic risks, the study also considers the role of the Thai government’s relief schemes during the pandemic. Our results show that the government relief schemes contributed a weak impact to workers’ survivability. The result shows that only the cash assistance for farmers (THB 5,000/month for 3 months) was effective in the first round. The significant impact of this program on Thai farmers is in line with Varshney et al. (Citation2021), who investigated the impact of India’s COVID-19 social assistance package on the agricultural sector. They revealed that the government transfer package was significant in alleviating liquidity constraints and resulted in increased spending on agricultural activities.
Nevertheless, our results related to the government assistance schemes are quite surprising since, to our expectation, the additional cash assistance from the program should have relaxed workers’ liquidity and consumption burdens and increased their survivability. The possible explanation is that the results from our analysis are the net of two major effects. The first effect is that of selection bias, which means that workers who were eligible to receive the government support were more vulnerable, to begin with, which makes them less likely to survive. The second effect is the government support programs themselves, which are supposed to be positive. The net effect is the result of the interaction between these two effects, which also considers the effects of other variables in the model. Janssens et al. (Citation2021), who studied the economic effects of COVID-19 in Kenya, suggested that households can maintain food spending during the initial weeks after the first assistance program. However, it is quite challenging to maintain it as time passes. Therefore, this could be another reason for the weak contribution of Thai government relief schemes.
Finally, when we compared the survival paths of workers in all three rounds, workers were relatively confident that they would survive in the short term, but the longer the pandemic continued, the less confident workers were about the likelihood of their survival because their resources had diminished over time (see ). However, we can observe a significant difference between the three survey rounds in that shows slight increases in survivability in the second and third surveys. In this regard, we expect that the impact of the pandemic may vary over time. Akkermans et al. (Citation2020) argued that job loss might initially be viewed as a negative impact. However, over time, it may allow workers to shift their skills and explore new jobs. Hopefully, Thai workers would be able to adjust eventually to survive the pandemic.
5. Conclusion
This study investigates the risk factors affecting the survival duration of Thai workers and assesses the role of the Thai government in increasing the economic survival probability of Thai workers during the recent COVID-19 crisis from May to November 2020. To achieve this research goal, we use The Asia Foundation’s survey data conducted in May 2020 (first round), August 2020 (second round) and November 2020 (third round) and adopt the Cox proportional-hazards regression with lasso estimation to quantitatively examine the effects of worker characteristics, worker behavior and government protection policies. The results from the Cox proportional-hazards regression reveal that workers characterized as low-educated, unemployed, unskilled, working in the tourism sector and living in the northeastern or southern regions are less likely to survive against COVID-19 crisis. On the other hand, the empirical results suggest that higher education significantly improves the survivability of Thai workers. As for the government policies, the results show that most of the policies have only a weak impact on workers’ survivability.
This study has important implications and recommendations for Thai government. Firstly, although the programs may have successfully targeted the vulnerable groups, there is not enough evidence that the social assistance programs were sufficient for Thai workers’ economic survivability. Looking forward, it is crucial to create a system to encourage more savings for workers, especially the informal low-skilled workers, to both sustain their lifestyle after retirement and be less vulnerable during future crises. Secondly, the government has put efforts in assisting the more vulnerable groups. However, the evidence showed that it was not yet enough. In addition to the low-skilled informal workers, the vulnerable groups to be targeted for additional assistances are females working in the tourism sector and living in regions with the highest poverty rates, which are north-eastern and southern regions. For female workers, they were more vulnerable partially due to their family responsibility. Additional cash supports for children was given through the child support grant. However, female workers might have also struggled with time allocation for work and childcare as schools were closed in many periods during the pandemic. Therefore, childcare may also be necessary. Moreover, the government could provide more direct supports to workers in the tourism and relevant services sectors, as well as people under poverty. Finally, approximately half of the workers were still at risk as of the end of 2020. If the COVID-19 situation has not improved, more assistance would be needed for Thai workers.
Few limitations of this study are worth mentioning. The first limitation is related to the focus on Thailand citizens; thus, our results could not be used as a reference for other countries or migrant workers living in Thailand. This is especially because the economic survival duration was partially improved by the government’s social protection packages as COVID-19 relief measures for Thai citizens. The benefits were highly limited for non-Thai citizens. Secondly, as the COVID-19 pandemic is a recent crisis, perceptions related to survivability of worker are ambiguous and may lead to false conclusion on impacts of a COVID-19. Although this is a relevant limitation of our study, we curbed such issue by restricting the scope of analysis to worker’s duration to consumption failure. This allows respondents to have a clearer opinion about their own survivability results during the pandemic. It should be noted that the duration to consumption failure was self-reported and can be biased due to respondents’ ability to evaluate their own economic situation, which was particularly difficult under the pandemic shock. Additionally, there could have been differences between workers who chose to participate in the survey and those who chose not to participate. Finally, the scope of the study is to evaluate short-run impacts of COVID-19 in the survivability of worker, between May 2020 and November 2020, and there may be other effects triggered by the crisis, such as dynamic changes in the labor market over the medium and long-run. Hence, the future work should cover a longer time horizon.
Disclosure statement
No potential conflict of interest was reported by the authors.
Correction Statement
This article has been republished with minor changes. These changes do not impact the academic content of the article.
References
- Adams-Prassl, A., Boneva, T., Golin, M., & Rauh, C. (2020). Inequality in the impact of the coronavirus shock: Evidence from real time surveys. Journal of Public Economics, 189, 104245. https://doi.org/10.1016/j.jpubeco.2020.104245
- Akkermans, J., Richardson, J., & Kraimer, M. (2020). The Covid-19 crisis as a career shock: Implications for careers and vocational behavior. Journal of Vocational Behavior, 119, 103434. https://doi.org/10.1016/j.jvb.2020.103434
- Al-Youbi, A. O., Al-Hayani, A., Rizwan, A., & Choudhry, H. (2020). Implications of COVID-19 on the labor market of Saudi Arabia: The role of universities for a sustainable workforce. Sustainability, 12(17), 7090. https://doi.org/10.3390/su12177090
- Antipova, A. (2021). Analysis of the COVID-19 impacts on employment and unemployment across the multi-dimensional social disadvantaged areas. Social Sciences & Humanities Open, 4(1), 100224. https://doi.org/10.1016/j.ssaho.2021.100224
- Aum, S., Lee, S. Y. T., & Shin, Y. (2021). Covid-19 doesn’t need lockdowns to destroy jobs: The effect of local outbreaks in Korea. Labour Economics, 70, 101993. https://doi.org/10.1016/j.labeco.2021.101993
- Bank of Thailand. (2020). Effects of COVID-19 on Thailand’s labor market. Bank of Thailand. https://www.bot.or.th/Thai/ResearchAndPublications/articles/Pages/Article_12Oct2020.aspx
- Belot, M., Choi, S., Tripodi, E., Van den Broek-Altenburg, E., Jamison, J. C., & Papageorge, N. W. (2021). Unequal consequences of Covid 19: Representative evidence from six countries. Review of Economics of the Household, 19(3), 769–783.
- Cox, D. R. (1992). Regression models and life-tables. Breakthroughs in statistics. Stat. Soc, 372, 527–541.
- Crossley, T. F., Fisher, P., & Low, H. (2021). The heterogeneous and regressive consequences of COVID-19: Evidence from high quality panel data. Journal of Public Economics, 193, 104334. https://doi.org/10.1016/j.jpubeco.2020.104334
- Danacica, D. E., & Babucea, A. G. (2010). Using survival analysis in economics. Survival, 11, 15.
- Gunsel, N. (2010). Determinants of the timing of bank failure in North Cyprus. The Journal of Risk Finance, 11(1), 89–106. https://doi.org/10.1108/15265941011012705
- International Labour Organization. (2020). COVID-19 employment and labour market impact in Thailand. ILO. https://www.ilo.org/asia/publications/labour-markets/WCMS_747944/lang–en/index.htm
- Janssens, W., Pradhan, M., de Groot, R., Sidze, E., Donfouet, H. P. P., & Abajobir, A. (2021). The short-term economic effects of COVID-19 on low-income households in rural Kenya: An analysis using weekly financial household data. World Development, 138, 105280. https://doi.org/10.1016/j.worlddev.2020.105280
- Jones, M. (2022). COVID-19 and the labour market outcomes of disabled people in the UK. Social Science & Medicine (1982), 292, 114637. https://doi.org/10.1016/j.socscimed.2021.114637
- Jung, H. S., Jung, Y. S., & Yoon, H. H. (2021). COVID-19: The effects of job insecurity on the job engagement and turnover intent of deluxe hotel employees and the moderating role of generational characteristics. International Journal of Hospitality Management, 92, 102703. https://doi.org/10.1016/j.ijhm.2020.102703
- Karim, M. R., Islam, M. T., & Talukder, B. (2020). COVID-19′ s impacts on migrant workers from Bangladesh: In search of policy intervention. World Development, 136, 105123. https://doi.org/10.1016/j.worlddev.2020.105123
- Kavkler, A., Danacica, D. E., Babucea, A. G., Bicanic, I., Bohm, B., Tevdovski, D., Tosevska, K., & Borsic, D. (2009). Cox regression models for unemployment duration in Romania, Austria, Slovenia, Croatia, and Macedonia. Romanian Journal of Economic Forecasting, 10(2), 81–104.
- Kikuchi, S., Kitao, S., & Mikoshiba, M. (2021). Who suffers from the COVID-19 shocks? Labor market heterogeneity and welfare consequences in Japan. Journal of the Japanese and International Economies, 59, 101117. https://doi.org/10.1016/j.jjie.2020.101117
- Koczan, Z. (2022). Not all in this together? Early estimates of the unequal labour market effects of Covid-19. Applied Economics, 54(44), 5021–5034. https://doi.org/10.1080/00036846.2022.2035311
- Komin, W., Thepparp, R., Subsing, B., & Engstrom, D. (2020). Covid-19 and its impact on informal sector workers: A case study of Thailand. Asia Pacific Journal of Social Work and Development, 31(1–2), 80–88.
- Narayanamurthy, G., & Tortorella, G. (2021). Impact of COVID-19 outbreak on employee performance–moderating role of industry 4.0 base technologies. International Journal of Production Economics, 234, 108075. https://doi.org/10.1016/j.ijpe.2021.108075
- NESDC. (2019). The 2019 poverty and inequality report. National Economic and Social Development Council. http://social.nesdc.go.th/social/Default.aspx?tabid=126&articleType=ArticleView&articleId=243
- Picatoste, X., Aceleanu, M. I., & Șerban, A. C. (2021). Job quality and well-being in OECD countries. Technological and Economic Development of Economy, 27(3), 681–703. https://doi.org/10.3846/tede.2021.14731
- Posel, D., Oyenubi, A., & Kollamparambil, U. (2021). Job loss and mental health during the COVID-19 lockdown: Evidence from South Africa. PloS One, 16(3), e0249352. https://doi.org/10.1371/journal.pone.0249352
- Puttachai, W., Yamaka, W., Maneejuk, P., & Sriboonchitta, S. (2019). Analysis of the global economic crisis using the cox proportional hazards model. In International Econometric Conference of Vietnam (pp. 863–872). Springer.
- Salinas-Escudero, G., Carrillo-Vega, M. F., Granados-García, V., Martínez-Valverde, S., Toledano-Toledano, F., & Garduño-Espinosa, J. (2020). A survival analysis of COVID-19 in the Mexican population. BMC Public Health, 20(1), 1–8.
- Shih, R., & Giles, D. E. (2009). Modelling the duration of interest rate spells under inflation targeting in Canada. Applied Economics, 41(10), 1229–1239. https://doi.org/10.1080/00036840701721232
- Tibshirani, R. (1997). The lasso method for variable selection in the Cox model. Statistics in Medicine, 16(4), 385–395. https://doi.org/10.1002/(SICI)1097-0258(19970228)16:4<385::AID-SIM380>3.0.CO;2-3
- Varshney, D., Kumar, A., Mishra, A. K., Rashid, S., & Joshi, P. K. (2021). India’s COVID-19 social assistance package and its impact on the agriculture sector. Agricultural Systems, 189, 103049. https://doi.org/10.1016/j.agsy.2021.103049
- Wang, D., Wu, T. T., & Zhao, Y. (2019). Penalized empirical likelihood for the sparse Cox regression model. Journal of Statistical Planning and Inference, 201, 71–85. https://doi.org/10.1016/j.jspi.2018.12.001