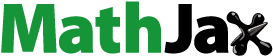
Abstract
The development of green finance and social low-carbon transformation is an essential concern for academia and industry. Based on Chinese provincial panel data spanning the period 2005–2019, we introduce the Cobb-Douglas production function and spatial Durbin and dynamic panel threshold models to deeply analyse the impact of green finance on the low-carbon economy. The mechanism test demonstrates that the scale, technique, and structural effects of green finance play a significant role in the low-carbon economy: they correct capital mismatch, promote green technology innovation, and optimise industrial structure. Meanwhile, green finance not only promotes the local low-carbon economy construction process, but also generates spatial spillover effects on neighbouring regions; however, there is regional heterogeneity in the impact of the transmission mechanism. Furthermore, only when capital mismatch is severe, and the low-end industrial structure poor is the positive impact of green finance on the low-carbon economy highlighted based on scale and structural effects; the ability of green finance to contribute to the low-carbon economy through the technique effect has been more stable and significant. This emphasises that green technology innovation is key to supporting low-carbon development in the long run.
1. Introduction
In 2021, China was ranked the second largest economy in the world, with its total economic output approaching US $17.73 trillion (Han et al., Citation2018). However, along with economic prosperity, a series of ecological damage problems have emerged, leading not only to a natural ecological imbalance, but also to serious threats to public health (Liu et al., Citation2020; Wang & Wang, Citation2019). Global warming is among the most serious ecological problems (Hu et al., Citation2020; Su & Yu, Citation2020). According to the International Energy Agency (IEA), global CO2 emissions from energy combustion and industrial processes will increase by 6%, year-on-year, to 36.3 billion tons in 2021. China emits more than 11.9 billion tons of CO2, accounting for 33% of the annual global total. The ‘Chinese Climate Bulletin’ shows that extreme weather climate events were frequent in 2021, while the average temperature was the highest on record in 70 years. The growing greenhouse effect and ecological damage have made the Chinese government realise that an economic rebound from the COVID-19 crisis is not sustainable. To avoid a global environmental disaster, the Chinese government has not only made a solemn commitment to the international community to reach a carbon peak and carbon neutralisation (Xi, Citation2017), but has also demonstrated its determination to achieve the sustainable development goals by promoting the construction of a low-carbon economy (Li et al., Citation2018). However, there remain some severe challenges in the process of the transformation and upgrading of China's low-carbon economy. Some scholars believe that, influenced by the era of a planned economy, limited resources preferentially flow or are allocated to the heavy industry sector, making it profitable in the short term (Abbasi et al., Citation2021b, Citation2021c; Sun et al., Citation2020). The resulting capital mismatch not only hinders the equalisation of marginal output values across sectors and structural progress of the economy, but also creates an inefficient and costly production model that induces excessive CO2 emissions (Bian et al., Citation2019; Hirakata & Sunakawa, Citation2019). In addition, due to a reliance on its advantage of energy resource endowment, China is overly dependent on the high-carbon development path, resulting in insufficient motivation for clean energy R&D and green process design (Cheng et al., Citation2018; Lv et al., Citation2021). Therefore, the weak foundation of green innovation and technical shortcomings are the second challenge faced. The low-end locking of industrial structure is another bottleneck that must be broken. China's economic development model is dominated by heavy industry, with most industries concentrated in the middle and low end of the industrial value chain with low added value; this directly leads to low energy efficiency and high pollution output (Abbasi et al., Citation2020, Citation2021f; Seth et al., Citation2018).
In summary, to comprehensively address the greenhouse gas emissions associated with economic development, the Chinese government has adopted a variety of financial incentives that consider the potential environmental rewards and risks. Green finance, which emerged as an innovative economic model against this background (Liu et al., Citation2019), emphasises the efficient allocation of resources and environmental risk transfer through interest transmission mechanisms; these mechanisms include financial investment and financing activities on the premise of respect for the concept of sustainable development (Lee et al., Citation2021). Economically, the green finance capital guarantee can avoid the investment risk associated with the green industry and optimise the quality and efficiency of the supply system, thereby accelerating the process of a low-carbon circular economy (Lee et al., Citation2022). From the perspective of the environment, the ‘green concept’ can guide a low-carbon transformation of industrial structures and the progress of green technology towards a sustainable development of the environment (Abbasi et al., Citation2021d, Citation2021e; Wang et al., Citation2022). In 2021, the ‘Green Finance Evaluation Scheme for Banking Financial Institutions’ was formulated by the PBoC, and became the programmatic document for China's green financial system. The ‘14th Five-Year’ plan further points out that under the guidance of a dual-carbon strategy, the ability of green financial services to promote low-carbon circular development should be promoted; this indicates that green finance is not only gradually attracting the attention of both the government and enterprises, but is also regarded as a significant incentive and leverage tool to promote the development of a low-carbon economy. Especially in the post-epidemic era, exploring the relationship between financial system innovation and low-carbon development is not only consistent with the significance of a future community of humanity, but also reflects the important role of China in global sustainable development (Iqbal et al., Citation2021; Zhou et al., Citation2022).
2. Literature review and theoretical analysis
2.1. The development of green finance
The development of green finance has increasingly been explored in the literature, which provides three main perspectives for discussion. The first view considers it as a concept of marketisation of ecological compensation, a kind of financial innovation mode, which gradually internalises the external costs or benefits of green technology to achieve an optimal disposition of financial resources in environmental and economic sectors (Han et al., Citation2017). The second refers to low-carbon finance, also called ecological finance, which is a general name for various financial institutions’ businesses and activities that are aimed at reducing carbon emissions (Wang et al., Citation2011). The third perspective considers green finance as a new standpoint from which sustainable economic and social development can be achieved through green credit, insurance, securities, and investment (Hu et al., Citation2021). For example, green credit and insurance provide investment and financing as well as risk sharing services for green technology innovation, respectively. Pollution control investments are used to compensate for environmental costs to guarantee the smooth development of a low-carbon economy (Lee & Wang, Citation2021; Wen et al., Citation2021).
Against this background, quantitative studies on green finance have been conducted. Thomas et al. (Citation2007) incorporated the external costs due to environmental pollution into economic benefits, providing an adequate basis for financial institutions to assess project risks and loan issuance. Employing the idea of game theory, Sheu and Chen (Citation2012) verified that the government should incentivise manufacturers to adopt green production through the implementation of green tax and subsidy policies. Su and Lian (Citation2018) pointed out that a green credit policy based on the restraint and punishment mechanism effectively reduced commercial banks’ cost efficiencies, especially for the investment and financing businesses of China's heavily polluting enterprises. In summary, reverse indicators such as green credit and green investment, or highly polluting enterprises’ interest expenses and fines, are now widely used to represent the development level of green finance (He et al., Citation2019b; Xie et al., Citation2020); the use of these indicators make it challenging to reveal green finance in its entirety, as the indicators can only describe the development of green finance from a single perspective. Green finance seems to be more aligned with a comprehensive system that comprises a coordinated operation of multiple systems such as markets, tools, and supervision. Referring to the design of green financial development established by Lee and Lee (Citation2022), they propose an index system via four levels (i.e., green credit, securities, insurance, and investment) to concretise the collaborative relationship between ecology and finance; this is undoubtedly conducive to the joint development of financial reform and low-carbon economic development (Lee et al., Citation2020). In addition, existing studies also address the direct contribution of green finance to the economy and society. Lee and Lee (Citation2022) argue that green finance can drive sustained economic growth. Based on Chinese provincial panel data, Bai et al. (Citation2022) confirm that green finance is the main means through which carbon emissions can be reduced, especially in the eastern region where the suppression effect is particularly pronounced.
H1. Green finance is positively associated with the construction of a low-carbon economy.
2.2. Green finance and a low-carbon economy
As discussed by Grossman (Citation1995), economic activities play a guiding role in environmental development through scale, technique, and structural effects. These effects provide theoretical support for exploring the function of green finance in optimising capital allocation, enhancing green technological innovation, and promoting industrial upgrading.
Based on Marshall's externality theory, the scale effect refers to the fact that green finance stimulates market competitiveness and alleviates the distortion of capital allocation among regions and industries through a centralised allocation of green funds, with a view to achieving a Pareto optimal state of capital in each sector. On the one hand, green finance, through policy guidance, may facilitate the expansion of corporate financing in the operation of green low-carbon projects, supporting a dynamic adjustment of the scales of enterprises and improvement of energy allocation efficiency. On the other hand, green finance compensates for the gap between the private and social costs of polluting enterprises by internalising environmental costs. This process not only constrains inefficient enterprises living spaces in polluting industries, but also guides the optimal allocation of resources between polluting and green industries. Yuan et al. (Citation2020) pointed out that the development of green financial resources at scale optimised the efficiency of inter-period capital flows and inter-regional transfers, which helped to reduce environmental pollution. Lee and Lee (Citation2022) contended that green finance alleviated distortions in capital allocation between regions and industries by improving the quality of the capital supply system.
H2: Green finance plays a positive role in promoting a low-carbon economy by correcting capital mismatch.
As a financial incentive-based environmental regulation instrument, the technique effect of green finance relies on Romer's theory of endogenous growth, which is reflected in the support for clean energy and the progress activities of the green process by prioritising the allocation of green R&D funds. On the one hand, green finance spreads technology R&D investment risk by facilitating the financing of corporate green progress. Green finance not only prioritises the concept of green development at the social level, but also promotes green technology innovation and iteration, contributing to the low-carbon transition. On the other hand, green financial services accelerate the flow and interaction of information, which alleviates information constraints and reduces the uncertainty of green technology innovation, thus ensuring that technology research has stable R&D space and long-term environmental benefits. He et al. (Citation2019a) and Abbasi et al. (Citation2021a) noted that green finance controlled the output of carbon emissions by supporting technological advances in renewable energy. Ren et al. (Citation2022) and Abbasi and Adedoyin (Citation2021) argued that green finance prioritised support for polluting enterprises and environmental preference enterprises in implementing green technology innovation activities through the technique effect. Thus, green finance is likely to alleviate the dilemma faced by China in the choice between energy demand and carbon emission reduction targets.
H3: Green finance positively impacts the building of a low-carbon economy by supporting green technology innovation.
Based on transaction cost theory and Porter's value chain theory, the structural effect refers to the fact that green finance stimulates industrial structural upgrading by guiding financial institutions to allocate low-cost capital to green and low-carbon industries. On the one hand, green finance differentially supports industries according to environmental policies, relying on structural effects to preferentially drive social funds into high value-added tertiary industries rather than high-energy-consuming and heavy-polluting enterprises. This provides a policy guarantee to curb the disorderly expansion of low- and medium-end manufacturing industries and optimise industrial structure. On the other hand, by breaking regional and industrial restrictions on existing sectors, green finance recombines production factors to maximise utility, which facilitates the realisation of the dominant advantage of green low-carbon sectors. Based on quasi-natural experiments and micro data, Wen et al. (Citation2021) confirm that green credit policies effectively address energy-intensive enterprises’ difficulties in transforming and upgrading under credit constraints. Long et al. emphasised that green finance accelerated the construction of a low-carbon circular economy by promoting the application of green production resources and sustainable production.
H4: Green finance has a positive role in low-carbon economy development by promoting industrial upgrading.
Although the causal relationships between green finance, CO2 emissions, and economic growth have been assessed (Charfeddine & Kahia, Citation2019; Jin et al., Citation2021), existing studies have failed to integrate economic growth and carbon emissions into a unified framework with a sustainable development perspective. In particular, a gap remains in the analysis of the transmission mechanism of green finance to a low-carbon economy. In light of these gaps, we first expand the framework by including green finance policies as an important factor in a low-carbon economy, and establish a transmission mechanism hypothesis on how green finance contributes to a low-carbon economy through capital allocation optimisation, green technology innovation, and industrial structural upgrading. Second, considering that low-carbon economic development typically exhibits a strong spatial correlation in geography, a spatial economic model that incorporates the characteristics of geographical location, combined with the moderating and spatial spillover effects, is adopted to explore the transmission mechanism of green finance to the development of a low-carbon economy through capital allocation, green technology innovation, and industrial structure. The model avoids biased and inconsistent results as a result of neglecting spatial layout and spatial dependence. Third, to address the endogenous problems and nonlinear relationships between the variables in the model, a dynamic panel threshold model is considered, which provides a theoretical cornerstone for examining the stage-specific characteristics of the effect of green finance on a low-carbon economy.
3. Research design
3.1. Model specification
3.1.1. Low-carbon economy function
A low-carbon economy is mainly moderated and affected by green finance through the scale, technique, and structural effects. Specifically, green finance plays a supporting role in the construction of a low-carbon economy by correcting capital mismatch, strengthening green technology innovation, and optimising industrial structure. The economic output (GDP) per unit of carbon emissions, carbon productivity (CP), that was first proposed by McKinsey consultancy in 2008 is considered an essential indicator in quantifying the low-carbon development of an economy and society (Hu & Liu, Citation2016; Liu & Zhang, Citation2021). Thus, CP is employed as the dependent variable to measure low-carbon economic development (see ). The low-carbon economy is expressed as follows:
(1)
(1)
Here, GF denotes green finance, and is employed as the independent variable. Following Liu et al. (Citation2019), and Lee et al. (Citation2022), we use the four indicators of green credit, insurance, investment, and government support to construct a comprehensive index for green finance, which is measured by the entropy weight method (see ). The related indicators and attributes in the evaluation system are described in . The average values for green finance and the low-carbon economy during the period 2005–2019 are presented in .
Figure 3. The average value of green finance and low-carbon economy during 2005–2019. Source: Authors.
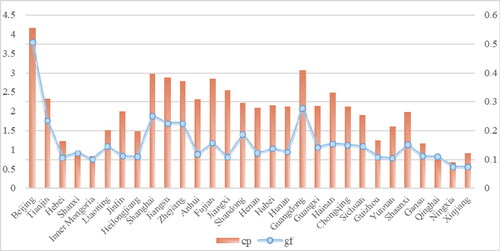
Table 1. Comprehensive evaluation system of green finance.
Here, the scale effect of green finance on the low-carbon economy is reflected in the optimisation of capital allocation, which can be characterised by its ability to correct capital misallocation. Therefore, based on the theoretical framework of scholars such as Hsieh and Klenow (Citation2009), we assume a Cobb-Douglas production function with a constant return to scale and take the logarithm of both sides, as in EquationEq. (2)(2)
(2) :
(2)
(2)
is the output variable, expressed by the real GDP when 2005 is the base period,
is the labour force input, defined as a province’s average annual employment.
is the capital factor input, expressed in terms of a province’s fixed capital stock and calculated using Zhang and Zhang’s (Citation2004) perpetual inventory method based on 2005 constant prices. An interaction term between the individual dummy and explanatory variables, whose coefficient is the capital-output elasticity
(Bai & Liu, Citation2018). is introduced into the regression equation. The index data are then brought into EquationEq. (3)
(3)
(3) to obtain the capital misallocation index.
(3)
(3)
where
represents the share of output,
of region
in total output,
refers to the weighted capital contribution.
is considered to be the actual proportion of the capital used by region
in the total capital, while
is the theoretical proportion of the capital used by region
when the capital is effectively allocated. Subsequently, the capital misallocation index (cmi) is computed as follows:
(4)
(4)
The World Intellectual Property Organization (WIPO) focuses on a sustainable development strategy and call for a wave of green technology innovation to address ecological issues. Therefore, the technique effect of green finance on the low-carbon economy is reflected in the promotion of sustainable progress, measured by the number of green invention patent applications (gti). The structural effect indicates the structural change of economic sectors related to carbon emissions. Compared with the industrial sector, the service sector is a prominent low-emission industry that plays a positive role in improving the overall growth rate of low-carbon economic development (Huang et al., Citation2020). Therefore, the proportion of the service industry’s added value in industrial added value (chg) is applied to denote the structural effect of green finance.
3.1.2. Control variables
For human capital (HC) is represented by per capita education level. Openness (OPEN) is measured in terms of foreign direct investment (Hao et al., Citation2020b). Infrastructure level (FUND) is measured by the ratio of fixed asset investment to GDP, while economic development level (ECO) is expressed in terms of per capita GDP. Environmental regulation (ER) is comprehensively measured using the entropy method with emissions of soot, SO2, and wastewater (Yang et al., Citation2015).
3.1.3. Data sources
The sample for this study comprises 30 Chinese provinces during the period 2005–2019. Primary data are obtained from the China Statistical Yearbook, China Statistical Yearbook on Science and Technology, and the official website of the National Bureau of Statistics. Data on green invention patents are derived from the national intellectual property database and matched with the green list of the WIPO’s international patent classification. The definitions and descriptive statistics of the data are shown in . In addition, the variance expansion factor and average value of each explanatory variable in the model are much lower than 10, which indicates that there is no multicollinearity problem ().
Table 2. The definitions of variables.
Table 3. Correlation coefficient of each variable and multicollinearity test.
3.2. Spatial econometric methods
3.2.1. Spatial econometrics model
Goodchild (Citation1992) pointed out that the spatial distribution of resources was not random, but often exhibited a certain spatial correlation due to geographical location or natural climate. Compared with the spatial lag and spatial error models (SEM), the spatial Durbin model (SDM) considers the spatial spillover effect between variables and the influence of the error term, which is more suitable for this study (Anselin & Florax, Citation1995). The SDM is formulated as follows:
(5)
(5)
(6)
(6)
where
and
denote province and time.
denotes a low-carbon economy and
denotes green finance.
represents human capital, openness, infrastructure level, economic development level, and environmental regulation.
and
are the random error terms assumed to be normally distributed.
3.2.2. Transmission mechanisms test
In this study, to detect the channel effects of the three transmission mechanisms of scale, technique, and structure between green finance and low-carbon economy, we first examine the impacts of green finance on the low-carbon economy (see EquationEq. (5)(5)
(5) ) based on the augmented Cobb-Douglas production function model. The capital mismatch index (cmi), green technology innovation (gti), and industrial structure change (chg) are then regarded as moderating variables and included in the regression model together with the interaction terms (gf*cmi, gf*gti, and gf*chg). Considering the possibility of increasing covariance, the interaction terms were decentralised (See Models 7, 8, and 9 for details).
(7)
(7)
(8)
(8)
(9)
(9)
The parameter, is the spatial autoregressive coefficient, which indicates the influence of the low-carbon economies of nearby provinces on a province.
represents the spatial spillover effects of the dependent variable,
and
is the regression coefficient of the independent variable.
is a vector of spatial autocorrelation coefficients of independent and control variables. Moreover,
and
indicate capital misallocation, green technology innovation, and industrial structural upgrading, respectively.
refers to the spatial weight matrix established based on the road distances between provincial capitals.
3.3. Dynamic generalized method of moments (GMM) threshold panel regression model
The GMM approach can effectively deal with endogenous problems, including those caused by missing variables and the bidirectional causality between green finance and the low-carbon economy. Meanwhile, it should be noted that, given the different degrees of capital mismatch, green technology innovation, and industrial structure, the absolute impact of green finance on the low-carbon economy based on scale, technique, and structural effects maybe not be the same in both the intense and even periods. Based on Hao et al. (Citation2020a), the dynamic threshold panel model settings are shown in EquationEqs. (10)(10)
(10) , Equation(11)
(11)
(11) , and Equation(12)
(12)
(12) , respectively.
(10)
(10)
(11)
(11)
(12)
(12)
is a lagging term of the low-carbon economy.
represents the index function, while
is the specific threshold value.
and
denote regime-dependent variables, which depend on the thresholds,
and
4. Empirical research and analysis
4.1. Spatial econometric regression results
Considering the spatial lag between the dependent and independent variables in the model, the interpretation of the parameters becomes more complex (LeSage & Pace, Citation2010). We report the direct and indirect effects of independent variables on the dependent variable based on a partial differential decomposition of EquationEqs. (5)(5)
(5) and Equation(7)–(9), to effectively avoid the errors of the SDM point estimation method (Elhorst, Citation2014). As shown at the bottom of , Moran’s index and spatial auto-correlation parameter,
are statistically significant at the 1% level in all types of model specifications. This not only emphasises the improvement of the low-carbon economies in adjacent regions, which leads to the same rising trends of low-carbon economies in the region, but also highlights the strong positive spatial spillover effects of the low-carbon economy.
Table 4. Regression results of spatial Durbin model for the whole sample.
Model (5) in shows that, based on the entire sample, green finance significantly promotes the development of the low-carbon economy at the 1% significance level, demonstrating that green finance is among the core drivers for accelerating the upgrading of low-carbon economic processes. H1 is thus supported. Next, to further explore the transmission mechanisms and impact of green finance on the low-carbon economy, the interaction terms, gf*cmi, gf*gti, and gf*chg, are included in the model to verify how green finance can correct capital mismatch, strengthen green technology innovation, and optimise industrial structural change based on the scale, technique, and structural effects.
According to the results of Model (7) in , capital mismatch has a blocking effect on the development of the low-carbon economy at the 5% significance level. Considering that environmental pollution has the characteristics of a negative externality, low-end production enterprises have the opportunity to obtain a market position at a lower cost, resulting in the distortion of resource allocation due to adverse selection, which finally hinders the improvement of the low-carbon economy. The coefficient of the interaction term, gf*cmi, is positive and statistically significant at the 1% level. Thus, a 1% rise in the interaction term leads to a 2.090% increase in the low-carbon economy’s growth, indicating that green finance achieves the goal of optimal capital allocation and pollution reduction through scale development, constituting a regulatory effect on the construction of the low-carbon economy. Thus, H2 is supported.
Model (8) in shows that the coefficient of the interaction term, gf*gti, is positive and statistically significant at the 1% level. When the interaction term increases by 1%, the CP will be raised by 1.501%, which is much higher than the direct effect of green technology innovation on the low-carbon economy. Regarding the contribution of green technology innovation to clean energy development and production technology progress, green finance emphasises persistent economic assistance to green technology innovation to provide continuous financial support and risk protection for economic construction and carbon emission reduction targets. H3 is thus supported.
Model (9) in presents the results concerning the impacts of green finance, industrial structural change, and the interaction term, gf*chg, on the low-carbon economy. The coefficient of industrial structural change is 0.073, positive but not significant, while the interaction term, gf*chg, is significant at the 10% level. In terms of its role, for a 1% increase in the interaction term, gf*chg, the development of the low-carbon economy will increase by 0.300%, which demonstrates that green finance supports the construction of the low-carbon economy through the low-carbon development of industrial structural upgrading. H4 is thus supported.
Furthermore, from the perspective of the regression coefficients, the scale effect of green finance is greatest on the low-carbon economy, followed by the technique and structural effects. A probable reason is the influence of the planned economic system, which led to capital mismatch that constrained economic transformation. Green finance, as a policy orientation, undoubtedly exerts pressure on high energy-consuming and inefficient enterprises and fundamentally eliminates reliance on high-carbon development paths. Therefore, green finance considerably promotes the process of optimising capital allocation and building a low-carbon circular economic system through the scale effect. In addition, the significance of green technology innovation in promoting the low-carbon economy is self-evident. However, due to the speed of transformation and market application ability of green invention patents, it is restricted to playing a greater role in supporting a low-carbon economy in the short term. Regarding the new planning and pilot reform of industrial layout, green finance will inevitably encounter risk loopholes and incur trial-and-error costs in promoting industrial transformation and upgrading, resulting in large investments in the early stage and slow effectivity. This will limit the release of sufficient green finance to drive low-carbon construction, and will negatively impact its supporting role in the low-carbon economy through industrial transformation and upgrading.
4.2. Regional heterogeneity and mechanism analysis results
In terms of annual average development levels of the low-carbon economy and green finance, the observed values for provinces in the eastern region are significantly higher than those in the central and western regions (), indicating that hierarchical characteristics are prominent. Therefore, the total sample is divided into three groups, eastern, central, and western, to highlight the potential impact of terrain diversity and to analyse the regional heterogeneous impact of green finance on the low-carbon economy.
shows that in the eastern region, green finance preferentially plays a corrective role in capital mismatch through the scale effect. For the central region, green finance plays a positive and moderate role in the low-carbon economy through the scale and technology effects at the 1% and 5% significance levels, respectively. Regarding the western region, the moderating effect of green finance on the low-carbon economy based on the technique and structural effects is positive at the 1% significance level, while the moderate path based on the scale effect is negative. From a spatial perspective, the spatial spillover effect of green finance in the central region is significantly negative and larger than the direct effect, while the spillover effects in the eastern and western regions are positive and negative, respectively, but nonsignificant. A possible reason is that there are differences in factor endowments and economic development between regions: Economically prominent regions will take the lead in popularising green financial services by relying on the advantages of financial infrastructure, resulting in excessive competition for limited resources in local regions. In addition, when neighbouring regions develop green finance and carbon reduction models, a stronger demonstration effect is generated. Consequently, the two forces are in a constant state of play between the two sides as resources move between regions. The economic construction in the eastern region is outstanding, with a strong demonstration effect, but is limited by the fact that green finance policies remain in the initial pilot stage, which constrains the positive spatial spillover effect on the low-carbon economy in the short term. Not only are the levels of financial infrastructure in the central and western regions weak, but the harsh climate and lack of resources also mean that they remain at a stage of marginal growth, relying on factor accumulation to achieve output. As seen from the above studies, the impacts of the scale, technique, and structural effects of green finance on the low-carbon economy are not unified, still reflecting inconsistent action paths and effects based on geographical location.
Table 5. Regional heterogeneity results of spatial Durbin model.
4.3. Dynamic threshold panel regression
The study additionally employs a dynamic panel threshold method that calibrates a nonlinear hypothesis into its framework; this not only addresses the endogenous problems and ensures the robustness of the results, but also provides an empirical basis for reflecting the nonlinear characteristics between green finance and the low-carbon economy.
shows that the P values for the AR (1) and AR (2) tests in the regression results of each model suggest that there is no obvious evidence of second-order or higher-order sequence correlation in the residuals. Specifically, there is only first-order but no second-order sequence correlation in the residuals of the difference equation. Moreover, based on Sargan’s test, the original hypothesis is supported at the 10% significance level, which confirms that all instrumental variables are influential, with no over-identification problem in the model.
Table 6. Dynamic threshold regression results.
As reported in Model 10 in , it can be deduced that the estimated threshold value for capital mismatch (cmi) based on two-stage least squares techniques is 0.148; the lower limit for the threshold value is 0.114, while the upper limit is 0.459, based on a 95% confidence interval. The coefficients, and
are −20.550 and 3.814, respectively. These values imply that a higher degree of regional capital mismatch may stimulate the role of green finance in promoting the low-carbon economy, rather than inhibit it. The heavier the capital distortion, the greater the room for the optimisation of social capital allocation efficiency, leading to a greater tendency for green finance to play a corrective function through the scale effect.
Model 6 shows the estimated green technology innovation (gti) threshold level and the corresponding 95% confidence interval ([4.771–8.878]). The coefficients, and
are 1.658 and 1.196, respectively, (both are regime-dependent coefficients of green finance) and are significant and similar. Green finance is conducive to actively promoting the growth of the low-carbon economy when green technology innovation is less than the threshold of 8.296 (
=1.658), while the support effect is slightly lower for higher green technology innovation (
=1.196). This notwithstanding, the above results confirm that the optimal path for green technology innovation-based technology effects is less influenced by macroeconomic factors. Thus, the marginal impact of green finance through the technique effect is significantly stable and sustainable, strongly supporting the development of China's low-carbon economic goals.
In Model 6, the lower limit of the threshold value is 0.744, while the upper limit is 1.575, based on a 95% confidence interval. When industrial structural change (chg) is less than the threshold value of 1.322, the coefficient is 4.390, which is significant at the 1% significance level. When the level of industrial structural change crosses the threshold value of 1.322, the coefficient is 0.145, which is not only significantly weaker than that in the first stage, but also fails to pass the significance level test. This suggests that the threshold value is at 1.322, below which green finance impacts the low-carbon economy, and beyond which the impact of green finance on the low-carbon economy weakens. Regarding the poor state of the low-end development of industrial structure, green finance plays a strong supporting role in the low-carbon economy through industrial transformation and upgrading. However, with the improvement of industrial upgrading construction, the marginal contribution of the structural effect of green finance to the low-carbon economy reaches a maximum, and the supporting role is gradually reduced.
5. Conclusions and policy implications
Based on a panel dataset that includes China’s 30 provinces and spans the period 2005–2019, we examine the influences and transmission mechanisms of green finance on the low-carbon economy through a combination of the spatial Durbin model and dynamic threshold panel. The main conclusions are as follows:
The scale, technique, and structural effects of green finance have a guiding and moderating effect on the low-carbon economy; the scale effect is the largest of the three, followed by the technique and structural effects. This indicates that green finance preferentially affects economic output and carbon emission through three paths: optimising capital allocation, strengthening green technology innovation, and improving industrial structure. Meanwhile, green finance not only promotes the local low-carbon economy construction process, but also generates spatial spillover effects on neighbouring regions; however, the significance of the spillover effects is weaker than that of the direct effects, indicating that the breadth and depth of green finance needs to be further popularised. There is regional heterogeneity in the interactive relationship between green finance and the low-carbon economy. The dynamic panel threshold model confirms that only when capital mismatch and low-end industrial structure are located within a specific threshold interval can green finance support the low-carbon economy through the path optimisation of the scale and structural effects. Meanwhile, the ability of green technology innovation to contribute to the low-carbon economy through the technique effect is not interval-bound and remains positive and stable in the long run.
Three policy implications for developing a low-carbon economy follow from this study:
First, the technique and structural effects of green finance are objectively slightly lower than the scale effect. This not only requires institutional openness to realise a market-oriented allocation of factors to consolidate the scale effect advantage of green finance; it is also necessary to establish a systematic market-oriented transformation mechanism of green technology by simplifying the green patent application procedure and strengthening intellectual property protection. Meanwhile, green technology and industrialisation feedback mechanisms must be established and relied on to guide effective innovation, optimise the suitability of the industrial upgrading path, and improve the support from the technique and structural effects of green finance on the low-carbon economy by reducing transformation costs and cycles.
Second, the leading role of green finance in the low-carbon economy should be based on local conditions. For the eastern region, a positive radiation effect will be released by accelerating the pilot construction work of green finance. For the central and western regions, it is necessary not only to continue to undertake industrial transfer from the eastern regions, but also to accelerate the learning and application of core technologies. Furthermore, green finance must be promoted to empower traditional resource factors, realise factor-driven efficiency and technology-driven transformation, and contribute to carbon peaking and neutrality.
Third, considering the obvious threshold interval selection effect of green finance based on the scale, technique, and structural effects, each province should follow the multi-level market evolution law of green finance. Meanwhile, according to the policy enactment and macroeconomic environmental changes, the local financial elements must be timely matched with the spillover model to support the development of the low-carbon economy. In particular, it is necessary to strengthen the coordination and complementarity between the application of green technology innovation and the carbon emission reduction market-based system to release the long-term potential of green technology innovation in the low-carbon economy construction process.
However, some questions remain unanswered in the empirical test, offering directions for future research. For instance, due to data limitations and the stage of green financial development, the selected measurement indicators are not comprehensive and specific, thus leading to deviations in the findings. Moreover, we can also refine the empirical data to the industry or enterprise level, such that the more intuitive impact of the green financial system on industrial development or enterprise operation can be investigated from a micro point of view.
Credit author statement
Yue Zhu: Methodology, Software, Writing—Original draft preparation. Juntao Zhang: Writing—Review and Editing, Supervision. Caiquan Duan: Data curation, Validation, Visualization. All authors have read and agreed to the published version of the manuscript.
Acknowledgements
The authors wish to acknowledge the financial support from the Philosophy and Social Science Research Planning Project of Heilongjiang Province (Grant No. 21GLC184).
Disclosure statement
The authors declare that they have no known competing financial interests or personal relationships that could have appeared to influence the work reported in this paper.
References
- Abbasi, K. R., Adedoyin, F. F., Abbas, J., & Hussain, K. (2021a). The impact of energy depletion and renewable energy on CO2 emissions in Thailand: Fresh evidence from the novel dynamic ARDL simulation. Renewable Energy. 180, 1439–1450. https://doi.org/10.1016/j.renene.2021.08.078
- Abbasi, K. R., Hussain, K., Abbas, J., Fatai Adedoyin, F., Ahmed Shaikh, P., Yousaf, H., & Muhammad, F. (2021b). Analyzing the role of industrial sector's electricity consumption, prices, and GDP: A modified empirical evidence from Pakistan. AIMS Energy, 9(1), 29–49. https://doi.org/10.3934/energy.2021003
- Abbasi, K. R., Abbas, J., & Tufail, M. (2021c). Revisiting electricity consumption, price, and real GDP: A modified sectoral level analysis from Pakistan. Energy Policy, 149, 112087. https://doi.org/10.1016/j.enpol.2020.112087
- Abbasi, K. R., & Adedoyin, F. F. (2021). Do energy use and economic policy uncertainty affect CO2 emissions in China? Empirical evidence from the dynamic ARDL simulation approach. Environmental Science and Pollution Research International, 28(18), 23323–23335. https://doi.org/10.1007/s11356-020-12217-6
- Abbasi, K. R., Adedoyin, F. F., Radulescu, M., Hussain, K., & Salem, S. (2021d). The role of forest and agriculture towards environmental fortification: designing a sustainable policy framework for top forested countries. Environment, Development and Sustainability, 24(6), 8639–8666. https://doi.org/10.1007/s10668-021-01803-4
- Abbasi, K. R., Jiao, Z., Shahbaz, M., & Khan, A. (2020). Asymmetric impact of renewable and non-renewable energy on economic growth in Pakistan: new evidence from a nonlinear analysis. Energy Exploration & Exploitation, 38(5), 1946–1967. https://doi.org/10.1177/0144598720946496
- Abbasi, K. R., Lv, K., Radulescu, M., & Shaikh, P. A. (2021e). Economic complexity, tourism, energy prices, and environmental degradation in the top economic complexity countries: fresh panel evidence. Environmental Science and Pollution Research International, 28(48), 68717–68731. https://doi.org/10.1007/s11356-021-15312-4
- Abbasi, K. R., Shahbaz, M., Jiao, Z., & Tufail, M. (2021f). How energy consumption, industrial growth, urbanization, and CO2 emissions affect economic growth in Pakistan? A novel dynamic ARDL simulations approach. Energy, 221, 119793. https://doi.org/10.1016/j.energy.2021.119793
- Anselin, L., & Florax, R. (1995). Introduction new direction in spatial econometrics (pp. 3–18). Springer-Verlag.
- Bai, J. C., Chen, Z. l., Yan, X., & Zhang, Y. Y. (2022). Research on the impact of green finance on carbon emissions: evidence from China. Economic Research-Ekonomska Istraživanja. Advance Online Publication. https://doi.org/10.1080/1331677X.2022.2054455
- Bai, J. H., & Liu, Y. Y. (2018). Can outward foreign direct investment improve the resource misallocation of China. China Industrial Economics, 1, 60–78.
- Bian, Y., Song, K., & Bai, J. (2019). Market segmentation, resource misallocation and environmental pollution. Journal of Cleaner Production, 228, 376–387. https://doi.org/10.1016/j.jclepro.2019.04.286
- Charfeddine, L., & Kahia, M. (2019). Impact of renewable energy consumption and financial development on CO2 emissions and economic growth in the MENA region: A panel vector autoregressive (PVAR) analysis. Renewable Energy. 139, 198–213. https://doi.org/10.1016/j.renene.2019.01.010
- Cheng, Z. H., Li, L. S., & Liu, J. (2018). Industrial structure, technical progress and carbon intensity in China's provinces. Renewable and Sustainable Energy Reviews, 81(2), 2935–2946. https://doi.org/10.1016/j.rser.2017.06.103
- Elhorst, J. P. (2014). Matlab software for spatial panels. International Regional Science Review, 37(3), 389–405. https://doi.org/10.1177/0160017612452429
- Goodchild, M. F. (1992). Geographical information science. International Journal of Geographical Information Systems, 6(1), 31–45. https://doi.org/10.1080/02693799208901893
- Grossman, G. M. (1995). Pollution and growth: What do we know? The economics of sustainable development. Cambridge University Press.
- Han, C., Zhang, W. G., & Feng, Z. X. (2017). How does environmental regulation remove resource misallocation—An analysis of the first obligatory pollution control in China. China Industrial Economics, 4, 115–134.
- Han, F., Xie, R., & Fang, J. Y. (2018). Urban agglomeration economies and industrial energy efficiency. Energy, 162, 45–59. https://doi.org/10.1016/j.energy.2018.07.163
- Hao, Y., Gai, Z., & Wu, H. (2020a). How do resource misallocation and government corruption affect green total factor energy efficiency? Evidence from China. Energy Policy, 143, 111562. https://doi.org/10.1016/j.enpol.2020.111562
- Hao, Y., Wu, Y., Wu, H., & Ren, S. (2020b). How do FDI and technical innovation affect environmental quality? Evidence from China. Environmental Science and Pollution Research International, 27(8), 7835–7850. https://doi.org/10.1007/s11356-019-07411-0
- He, L., Liu, R., Zhong, Z., Wang, D., & Xia, Y. (2019a). Can green financial development promote renewable energy investment efficiency? A consideration of bank credit. Renewable Energy. 143, 974–984. https://doi.org/10.1016/j.renene.2019.05.059
- He, L. Y., Zhang, L. H., Zhong, Z. Q., Wang, D. Q., & Wang, F. (2019b). Green credit, renewable energy investment and green economy development: Empirical analysis based on 150 listed companies of China. Journal of Cleaner Production, 208, 363–372. https://doi.org/10.1016/j.jclepro.2018.10.119
- Hirakata, N., & Sunakawa, T. (2019). Financial frictions, capital misallocation and structural change. Journal of Macroeconomics, 61, 103127. https://doi.org/10.1016/j.jmacro.2019.103127
- Hsieh, C. T., & Klenow, P. J. (2009). Misallocation and manufacturing Tfp in China and India. Quarterly Journal of Economics, 124(4), 1403–1448. https://doi.org/10.1162/qjec.2009.124.4.1403
- Hu, G., Wang, X., & Wang, Y. (2021). Can the green credit policy stimulate green innovation in heavily polluting enterprises? Evidence from a quasi-natural experiment in China. Energy Economics, 98, 105134. https://doi.org/10.1016/j.eneco.2021.105134
- Hu, M., Li, R., You, W., Liu, Y., & Lee, C. C. (2020). Spatiotemporal evolution of decoupling and driving forces of CO2 emissions on economic growth along the Belt and Road. Journal of Cleaner Production, 277, 123272. https://doi.org/10.1016/j.jclepro.2020.123272
- Hu, X. C., & Liu, C. L. (2016). Carbon productivity: a case study in the Australian construction industry. Journal of Cleaner Production, 112, 2354–2362. https://doi.org/10.1016/j.jclepro.2015.10.042
- Huang, J., Wu, J., Tang, Y., & Hao, Y. (2020). The influences of openness on China's industrial CO2 intensity. Environmental Science and Pollution Research International, 27(13), 15743–15757. https://doi.org/10.1007/s11356-020-08086-8
- Iqbal, N., Abbasi, K. R., Shinwari, R., Guangcai, W., Ahmad, M., & Tang, K. (2021). Does exports diversification and environmental innovation achieve carbon neutrality target of OECD economies? Journal of Environmental Management, 291, 112648. https://doi.org/10.1016/j.jenvman.2021.112648
- Jin, Y., Gao, X., & Wang, M. (2021). The financing efficiency of listed energy conservation and environmental protection firms: Evidence and implications for green finance in China. Energy Policy, 153, 112254. https://doi.org/10.1016/j.enpol.2021.112254
- Lee, C. C., & Lee, C. C. (2022). How does green finance affect green total factor productivity? Evidence from China. Energy Economics, 107, 105863. https://doi.org/10.1016/j.eneco.2022.105863
- Lee, C. C., & Wang, C. W. (2021). Firms' cash reserve, financial constraint, and geopolitical risk. Pacific-Basin Finance Journal, 65, 101480. https://doi.org/10.1016/j.pacfin.2020.101480
- Lee, C. C., Lee, C. C., & Li, Y. Y. (2021). Oil price shocks, geopolitical risks, and green bond market dynamics. The North American Journal of Economics and Finance, 55, 101309. https://doi.org/10.1016/j.najef.2020.101309
- Lee, C. C., Wang, C. W., & Ho, S. J. (2020). Financial innovation and bank growth: The role of institutional environments. The North American Journal of Economics and Finance, 53, 101195. https://doi.org/10.1016/j.najef.2020.101195
- Lee, C. C., Wang, C. W., & Ho, S. J. (2022). Financial aid and financial inclusion: Does risk uncertainty matter? Pacific-Basin Finance Journal, 71, 101700. https://doi.org/10.1016/j.pacfin.2021.101700
- LeSage, J. P., & Pace, R. K. (2010). Spatial econometric models. In Handbook of applied spatial analysis (pp. 355–376). Springer.
- Li, Z. H., Liao, G. K., Wang, Z. Z., & Huang, Z. H. (2018). Green loan and subsidy for promoting clean production innovation. Journal of Cleaner Production, 187, 421–431. https://doi.org/10.1016/j.jclepro.2018.03.066
- Liu, G. C., Liu, Y. Y., & Lee, C. C. (2020). Growth sources of green economy and energy consumption in China: New evidence accounting for heterogeneous regimes. Energy Journal, 41, 33–63. https://doi.org/10.5547/01956574.41.6.gliu
- Liu, R., Wang, D., Zhang, L., & Zhang, L. (2019). Can green financial development promote regional ecological efficiency? A case study of China. Natural Hazards, 95(1–2), 325–341. https://doi.org/10.1007/s11069-018-3502-x
- Liu, X. P., & Zhang, X. L. (2021). Industrial agglomeration, technological innovation and carbon productivity: Evidence from China. Resources, Conservation and Recycling, 166, 105330. https://doi.org/10.1016/j.resconrec.2020.105330
- Lv, C. C., Shao, C. H., & Lee, C. C. (2021). Green technology innovation and financial development: do environmental regulation and innovation output matter? Energy Economics, 98, 105237. https://doi.org/10.1016/j.eneco.2021.105237
- Ren, S. Y., Hao, Y., & Wu, H. T. (2022). How does green investment affect environmental pollution? Evidence from China. Environmental and Resource Economics, 81(1), 25–51. https://doi.org/10.1007/s10640-021-00615-4
- Seth, D., Rehman, M. A. A., & Shrivastava, R. L. (2018). Green manufacturing drivers and their relationships for small and medium (SME) and large industries. Journal of Cleaner Production, 198, 1381–1405. https://doi.org/10.1016/j.jclepro.2018.07.106
- Sheu, J. B., & Chen, Y. M. J. (2012). Impact of government financial intervention on competition among green supply chains. International Journal of Production Economics, 138(1), 201–213. https://doi.org/10.1016/j.ijpe.2012.03.024
- Su, D. W., & Lian, L. L. (2018). Does green credit policy affect corporate financing and investment? Evidence from publicly listed firms in pollution-intensive industries. Journal of Financial Research, 462, 123–137.
- Su, Y., & Yu, Y. Q. (2020). Spatial agglomeration of new energy industries on the performance of regional pollution control through spatial econometric analysis. The Science of the Total Environment, 704, 135261. https://doi.org/10.1016/j.scitotenv.2019.135261
- Sun, C., Zhan, Y., & Du, G. (2020). Can value-added tax incentives of new energy industry increase firm’s profitability? Evidence from financial data of China’s listed companies. Energy Economics, 86, 104654. https://doi.org/10.1016/j.eneco.2019.104654
- Thomas, S., Repetto, R., & Dias, D. (2007). Integrated environmental and financial performance metrics for investment analysis and portfolio management. Corporate Governance: An International Review, 15(3), 421–426. https://doi.org/10.1111/j.1467-8683.2007.00575.x
- Wang, R., Zhao, X., & Zhang, L. (2022). Research on the impact of green finance and abundance of natural resources on China's regional eco-efficiency. Resources Policy, 76, 102579. https://doi.org/10.1016/j.resourpol.2022.102579
- Wang, Y. L., Ma, Y., Wang, S. C., Liu, Y. T., & Ye, M. (2011). Establishment and development of green financial system of China. Finance & Trade Economics, 10, 38–46. https://doi.org/10.19795/j.cnki.cn11-1166/f.2011.10.006
- Wang, Y., & Wang, J. (2019). Does industrial agglomeration facilitate environmental performance: New evidence from urban China? Journal of Environmental Management, 248, 109244. https://doi.org/10.1016/j.jenvman.2019.07.015
- Wen, H. W., Lee, C. C., & Zhou, F. X. (2021). Green credit policy, credit allocation efficiency and upgrade of energy-intensive enterprises. Energy Economics, 94, 105099. https://doi.org/10.1016/j.eneco.2021.105099
- Xi, J. (2017). Full text of Xi Jinping's report at 19th CPC National Congress.
- Xie, H. L., Ouyang, Z. Y., & Choi, Y. (2020). Characteristics and influencing factors of green finance development in the Yangtze River Delta of China: Analysis based on the spatial Durbin model. Sustainability, 12(22), 9753. https://doi.org/10.3390/su12229753
- Yang, Z. B., Shao, S., & Zhang, C. (2015). Production comparative advantage, ratchet effect and technical capital deviation of Chinese industry. Journal of Quantitative and Technical Economics, 32, 39–55.
- Yuan, H. X., Feng, Y. D., Lee, J., Liu, H. M., & Li, R. Z. (2020). The spatial threshold effect and its regional boundary of financial agglomeration on green development: A case study in China. Journal of Cleaner Production, 244, 118670. https://doi.org/10.1016/j.jclepro.2019.118670
- Zhang, J. W., & G. Y., Zhang, J. P. (2004). Estimates of China’s inter-provincial physical capital stock. Economic Research, 10, 1952–2000.
- Zhou, R., Abbasi, K. R., Salem, S., Almulhim, A. I., & Alvarado, R. (2022). Do natural resources, economic growth, human capital, and urbanization affect the ecological footprint? A modified dynamic ARDL and KRLS approach. Resources Policy, 78, 102782. https://doi.org/10.1016/j.resourpol.2022.102782