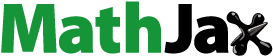
Abstract
This study distinguishes between two dimensions of firm process innovation, namely, quantity and quality, and uses data from the World Bank’s China Manufacturing Firm Survey to analyse the differential impact of Internet applications on the quantity and quality of process innovation and their mechanisms of action. Internet applications have a significant facilitating effect on the quantity and quality of process innovation. However, from the perspective of the average marginal effect, the facilitating effect of Internet applications on the quantity of process innovation is greater than that on the quality of process innovation. Further analysing firm size, industry, ownership, and regional heterogeneity shows that in terms of the quantity of process innovation, Internet applications have a greater impact on small- and medium-sized firms, labour-intensive firms, non-state-owned firms, and eastern firms. As for the quality of process innovation, Internet applications have a stronger promoting effect on large firms, technology-intensive firms, and state-owned firms. The mechanism test reveals that open innovation and informatisation capability play a mediating role in the influence of a firm’s Internet applications on process innovation. This study provides micro-empirical evidence for firms’ Internet applications to promote process innovation and policy insights into China’s manufacturing transformation and upgrading.
1. Introduction
The manufacturing industry is the fundamental source of China’s comprehensive strength and serves as a solid economics foundation. However, at present, China’s manufacturing industry is facing multiple pressures from developed countries’ strategies of ‘return of manufacturing’ and ‘re-industrialisation’ and the rapid rise of the manufacturing strength of emerging countries (Ciravegna & Michailova, Citation2022). Hence, China’s manufacturing cost advantage has become unsustainable, and the country now requires a new model to drive the transformation and upgrading of the manufacturing industry.
Process innovation is considered a major source of economic development (Terjesen & Patel, Citation2017) and a key factor in improving firm efficiency (Piening & Salge, Citation2015). Utterback and Abernathy (Citation1975) first introduced the concepts of product and process innovation, both of which are innovative activities in the field of technological innovation. Product innovation is market-focused, with new products or services introduced to meet external user needs, and it is embodied in a firm’s output and may lead to product differentiation or improved product quality (Aliasghar et al., Citation2019a). However, product innovation alone is not enough to sustain growth in the efficiency of manufacturing firms, and studies by US scholars have found that neglecting process innovation is an important reason why the US has lost its competitive advantage in the manufacturing sector (Maniaci, Citation1990). Process innovation is more systematic, complex, and costly to invest in than product innovation, but it is more likely to occur and has a higher efficiency (Lambertini, Citation2004). Unlike product innovation, process innovation emphasises the improvement of production methods and procedures in the production of a product so as to increase output levels and reduce production costs, thereby achieving economies of scale and sustained low-cost advantages (Martínez-Ros, Citation2019). Process innovation is mostly endogenous and is the result of cumulative learning over time, which is unique and creates greater value for the firm (James et al., Citation2013). Process innovation may be more effective than product innovation in terms of a firm’s competitive advantage as its intangibility and embeddedness make it more difficult for competitors to imitate (Gopalakrishnan et al., Citation1999). Thus, enhanced process innovation can help Chinese manufacturing firms regain their competitive advantage.
The use of Internet technologies in business has led to a shift in activities towards the use of Internet technologies to create new value for firms and their customers (Neirotti & Pesce, Citation2019). The Internet has enriched and expanded the innovation resources of manufacturing firms due to its ‘interconnectedness,’ information sharing, and reduced search and transaction costs (Bygstad & Aanby, Citation2010), thereby making various types of innovation possible. Early studies provide evidence that Internet applications in firms facilitate process innovation (Galati & Bigliardi, Citation2019; Neirotti & Pesce, Citation2019). However, few studies have examined the impact of Internet applications on firms’ process innovation quantity and quality and the mechanisms of such impact. Unlike previous studies, this work contributes to the existing literature in two ways: Firstly, it distinguishes between the quantitative and qualitative dimensions of firm process innovation. Specifically, the quantity of process innovation intuitively reflects the degree of firm process innovation, whereas the quality indicator of process innovation objectively measures the degree of market realisation of process innovation outcomes, that is, it reflects the value of use ultimately formed by process innovation. Secondly, by considering the mediating role of open innovation and firms’ informatisation capability, the differentiated impact of firms’ Internet application on the quantity and quality of process innovation is elucidated.
2. Literature review and research hypothesis
2.1. Literature review
Utterback and Abernathy (Citation1975) defined process innovation as the cumulative development of an entire (production) process, that is, ‘a system of process equipment, labour, task specifications, material inputs, and work and information flows employed to produce a product or service.’ Process innovation is centred within the firm and introduces new elements in the production or service process to reduce costs (Caldera, Citation2010; Lo Turco & Maggioni, Citation2015), shorten the design cycle of products (Gao & Bi, Citation2014), transition from customer-driven manufacturing (Hullova et al., Citation2016), improve product quality and production flexibility (Bulut et al., Citation2022; Hwang & Hsu, Citation2019; Reichstein & Salter, Citation2006), and promote business performance.
The quantity and quality of innovation are the two dimensions that measure a firm’s innovation output. Quality of innovation reflects an innovation’s technical level and market value (Zhao et al., Citation2020). Haner (Citation2002) defined innovation quality as the combined result of product or service quality, operational process quality, and management quality. An innovation has ‘quality’ if the firm creates a new product, improved process, or management model through innovation efforts, and the result satisfies the relevant stakeholders (Yang, Citation2013). Process innovation is an activity in which technology and markets are integrated. Therefore, technical and market information are indispensable to directly measure the ‘quality’ of innovation results through the market realisation of a firm’s product value. Therefore, in the current study, the quality of process innovation is defined as ‘the ratio of the correlation between the firm’s annual output and the newly introduced or newly improved process.’ Engelstätter (Citation2012) defined the number of process innovations as ‘the number of specific types of innovation activities that are beneficial to reducing marginal production costs.’
Process innovation represents an improvement in the processes and methods of production in a firm, and it is permeated by the way various factors of productivity are recombined and utilised (Chang, Citation2018). The widespread use of the Internet has accelerated the generation, acquisition, integration, and flow of various factors of production in manufacturing firms (Agostini et al., Citation2020), greatly optimising production business processes (Galati & Bigliardi, Citation2019). Firms increasingly tend to aggregate into inter-organisational ecosystems, collaborating extensively with partner firms, suppliers, customers, consultants, universities, and research institutions (Agostini & Nosella, Citation2019; Pereira et al., Citation2019). These ecosystems consist of interconnected and independent stakeholders that share factors of production, such as information, data, and technological knowledge. The widespread use of the Internet has accelerated the transfer and exchange of these factors of production within the ecosystem (Galati & Bigliardi, Citation2017), with a higher degree of recombination and reuse than would otherwise be the case; this condition, in turn, has led to innovation in business processes (Chang, Citation2018; Wareham et al., Citation2014).
Process innovation means that firms need to strengthen their information technology capabilities to enable them to access, assimilate, and utilise external knowledge and information promptly (Aliasghar & Haar, Citation2021; Aliasghar et al., Citation2019b; Guo et al., Citation2021). Internet-based supply chain management (SCM) systems and enterprise resource planning (ERP) can effectively forecast raw material and intermediate input demand, expand the scope of supplier search and matching, improve intrafirm collaboration, and facilitate business process improvement (Nwankpa et al., Citation2022). Customer relationship management (CRM) systems have become one of the most popular tools in firm information management (Gil-Gomez et al., Citation2020). CRM can positively impact business processes by increasing customer loyalty, improving marketing strategies, improving customer service and support, increasing efficiency, and reducing costs (Huang & Lin, Citation2005; Neirotti & Pesce, Citation2019).
2.2. Research hypothesis
Successful process innovation relies on a firm’s ability to identify, acquire, and utilise complex knowledge (Hullova et al., Citation2016; West & Bogers, Citation2014). The rapidly evolving Internet has improved how information is generated and disseminated between organisations, breaking through the time and space limitations of information transfer and rapidly enabling the penetration of knowledge and information (Bloom et al., Citation2014). The widespread application of the Internet by firms not only reduces the cost of external information search and acquisition and increases the stock of knowledge but also promotes the diffusion and absorption of internal knowledge with a knowledge accumulation amplification effect, which facilitates the transformation of knowledge into innovation (Bygstad & Aanby, Citation2010). In this way, firms can recognise the value of new external information; absorb, transform, and utilise the newly generated knowledge in the manufacturing process; and promote the development of process innovation (Chuks, Citation2022). As a general-purpose technology, Internet technology is embedded in process innovation activities and can exert the effect of technological progress embedded in itself. By contrast, the knowledge spillover and learning effects caused by network effects promote firm innovation efficiency and change in factor use. On the basis of this discussion, we hypothesise the following:
H1: Internet application promotes firm process innovation.
The Internet has enabled a significant reduction in collaboration time and search costs for technological innovation between firms. Moreover, it has minimised the efficiency loss due to information asymmetry and reconfigured pre-existing technological knowledge and learning effects, thus giving rise to new technological knowledge and accelerating the flow of innovation resources, such as knowledge across organisations. The search for new knowledge with commercial potential is key to firms’ innovation activities (Laursen & Salter, Citation2006; Qu & Li, Citation2019). Internet applications have greatly increased the willingness and ability to share technology, ideas, and information of manufacturing firms and innovation agents in different supply chain segments, thus expanding and enriching innovation sources (Battistella et al., Citation2018). Therefore, this study proposes the following:
H2: Open innovation mediates the impact of firms’ Internet applications on process innovation.
The widespread use of Internet-based SCM systems enables the effective forecasting of the demand for raw materials and intermediate inputs and improves collaboration within firms. Meanwhile, ERP systems expand the scope of supplier search and matching, provide real-time information on the inventory and use of various raw materials and intermediate goods within the firm, reduce order delivery times, and improve production collaboration, thereby facilitating the enhancement of process innovation (Hullova et al., Citation2016). Implementing SCM and ERP systems helps shorten delivery times, improve production efficiency, and enhance business processes (Neirotti & Pesce, Citation2019; Nwankpa et al., Citation2022).
CRM systems have become one of the most popular tools in business information management (Gil-Gomez et al., Citation2020). By implementing and using CRM in businesses, companies can better understand consumers and know more about their requirements, opinions, suggestions, and ideas (Lam et al., Citation2016). Moreover, mining and analysing CRM data can positively impact business profitability and improve business processes by increasing customer loyalty, improving marketing strategies, improving customer service and support, increasing efficiency, and reducing costs (Guo et al., Citation2021; Huang & Lin, Citation2005; Neirotti & Pesce, Citation2019). Meyer and Schwager (Citation2007) argued that the effective management of supplier- and customer-related information can bring about potential improvements in any given business relationship. This allows firms to achieve operational advantages by simultaneously achieving superior efficiency or organisational effectiveness across many market segments (Neirotti & Pesce, Citation2019). In summary, this study proposes the following hypotheses:
H3: Information technology capability mediates the impact of firms’ Internet applications on process innovation.
3. Data sources and model specification
3.1. Data sources
The data in this study come from a survey conducted by the World Bank between December 2011 and February 2013 on a sample of 2,700 private firms and 148 wholly state-owned firms in 25 major cities in eastern and western China, including Beijing, Shanghai, Guangzhou, Hefei, and Lanzhou. These firms belonged to 20 manufacturing categories, including precision instruments, chemical products, machinery, and equipment; and 7 services and retail categories, including transportation, motor vehicle services, and information technology. The questionnaire consisted of two parts. The first part investigates the business and investment environment in which the companies operate, including questions on background information, infrastructure and public services, customers and suppliers, competitive environment, innovation and technology, business-government relations, and barriers to business. In this study, we focus on the mechanism of the impact of Internet applications on process innovation in manufacturing firms. Hence, service and retail firms and manufacturing firms with missing core indicators are excluded from the study, thus resulting in data on 1,708 manufacturing firms.
3.2. Variable selection and description
3.2.1. Explained variables
The dependent variable is firm process innovation (PI). Innovation quantity and quality are two sides of the same coin, reflecting the scale and market value of innovation output, respectively. Focusing on innovation quantity or quality alone cannot guide firms to improve their technological innovation capabilities. Therefore, this study constructs process innovation indicators from the different innovation outcomes of process innovation quantity and quality to fully capture the difference between Internet applications and a firm’s process innovation. Drawing on Engelstätter’s (Citation2012) study, the quantity of process innovation (PIN) is measured by the ‘number of times a firm engages in specific types of innovation activities that contribute to reducing marginal production costs’ whilst companies engaging in process innovation activities are characterised according to the following: CNO14a: ‘introduction of new technologies and equipment to enhance products and business processes;’ CNO14b: ‘introduction of new quality control procedures in products and operations;’ CNO14c: ‘introduction of new management processes;’ CNO14d: ‘providing technical training for employees;’ and CNO14g: ‘taking measures to improve product resilience.’ Drawing on Haner (Citation2002) and Yang (Citation2013), the quality of process innovation (PIL) is measured by the ‘proportion of annual firm output correlated with newly introduced or newly improved processes.’
3.2.2. Explanatory variables
The core explanatory variable is Internet application (IA). Drawing on the studies of Wang et al. (Citation2020) and Zhang and Wei (Citation2019), the questionnaire (CNO.15) investigates the extent to which firms’ Internet application supported the five specific types of innovation activities in which they are engaged in (CNO14), assign a value of 0 to ‘never use’ and a value of 1 to ‘sometimes use’ and ‘often use,’ and sum up the dummy variables. The higher the value, the higher the degree to which the company uses the Internet to support process innovation. In addition, the five types of process innovation are assigned a value of 0 for ‘never use’ and ‘sometimes use’ and a value of 1 for ‘often use.’ The Internet dummy variable (IAdum) is constructed to further test whether Internet use exerts a process innovation promotion effect.
3.2.3. Mediating variables
Drawing on Wang et al. (Citation2020) measure of open innovation, this study assigns a value of 1 to the question ‘In what way do firms introduce or enhance business processes’ if the firms cooperate with suppliers in development and 0 otherwise. A value of 1 is also assigned ‘if firms cooperate with customer companies in the development;’ otherwise, a value of 0 is assigned. If a company cooperates with other companies in research and development (R&D), the value is 1; otherwise, it is 0. If the company’s R&D ideas come from external organisations, such as consultants, universities, and research institutes, the value is 1; otherwise, it is 0. These four dummy variables are summed to obtain the mediating variable of open innovation (OIB). For the question ‘What types of communication media and technologies are used to maintain and transact inter-firm relationships,’ the answer options of SCM, ERP, and CRM systems are assigned a value of 1 if they are used and 0 otherwise to obtain the mediating variable of information technology capability (IC).
3.2.4. Control variables
For firm size (Lnsize), Kim (Citation2022) found that firm size and age can significantly affect firm innovation. In this study, we use the natural logarithm of the number of employees as a proxy variable for firm size. This study controls for firm age (Age), measured as the difference between firm establishment and the year of the survey (2011). Drawing on Lin et al. (Citation2010) measure of firm age, this study uses 2011 minus the year of firm incorporation plus 1 and takes the natural logarithm of the result. As for whether or not to export, the ‘export learning effect’ induces firms with export experience to engage in technological innovation whilst technological innovation further improves firm productivity, thus making firms more likely to choose to export (Mai et al., Citation2019); this is coded as ‘1’ if the firm exports positively and ‘0’ otherwise.’ There is a significant correlation between a firm’s labour productivity (Productivity) and process innovation (Woltjer et al., Citation2021). In this study, we use the natural logarithm of the ratio of a firm’s sales revenue to its total employment, which is the firm’s sales revenue per capita, to control for the firm’s labour productivity. Whether or not foreign technology licencing (FTL) is obtained, China’s technological innovation benefits to a large extent from technology transfer from developed countries; this is coded as ‘1’ if the firm obtains technology licences from foreign companies other than office software and ‘0’ otherwise. Human capital (HR), a firm’s highly skilled employees, is a key factor in innovation (Agostini & Filippini, Citation2019). In this study, we use the ratio of the number of skilled employees to the total number of full-time employees to measure a firm’s human capital. Process innovation usually involves organisational and technological changes in business processes, such as the use of information and communication technologies (ICTs), the adoption of new management methods, and the introduction of new equipment; therefore, Internet infrastructure investment (Ict_invest) has a direct impact on innovation (Zhu et al., Citation2021; Chuks, Citation2022). In this study, the natural logarithm of the amount of infrastructure investment is used as a measure. As for informal_competition (Informal_com), the activity of informal firms puts pressure on formal firms to innovate (Pérez et al., Citation2019). Firms are coded as ‘1’ if they reported that they faced competition from informal firms and ‘0’ otherwise. Higher tax burdens can negatively affect the amount of innovation by firms (Akcigit et al., Citation2022); herein, Tax_burden ranges from ‘no effect’ (0) to ‘significant effect’ (4) according to the item ‘extent to which corporate tax rates affect business conditions.’ Differences in business ownership (state-owned enterprises, SOEs) can also have an impact on process innovation (Amin et al., Citation2019). This study identifies firms with government ownership greater than 50% as SOEs. Finally, we control for geographic location (city) because cities differ in terms of knowledge spillover, resources, and government support (Tsinopoulos et al., Citation2018). The descriptive statistics for each variable are shown in .
Table 1. Descriptive statistics of variables.
3.3. Model setting
3.3.1. Baseline model
The questionnaire measures the number of process innovations (PIN) using ‘the number of times a firm engages in a specific type of innovative activity that contributes to reducing marginal production costs.’ The dependent variable is a count variable that does not meet the basic assumptions of ordinary least squares estimation. Moreover, the mean (r(mean) = 3.0158) and variance (r(Var) = 3.1814) of the number of process innovations are approximately equal, which satisfies the assumptions of the Poisson regression model and successfully passes the goodness-of-fit test. Therefore, this study uses the Poisson regression model to estimate the impact of Internet application on the number of process innovations. The Tobit model is used to estimate the impact of Internet applications on the quality of process innovation. Drawing on the existing literature on the factors influencing firms’ innovation activities (Simonen & McCann, Citation2008), we set a baseline regression equation:
(1)
(1)
where the subscript i represents each firm; the dependent variable PIkji denotes the process innovation of firm i in industry j in city k, including the quantity of process innovation (PIN) and the quality of process innovation (PIL); the core explanatory variable IAkji denotes the Internet application of firm i in industry j in city k; CV denotes the set of control variables in the model; industryj and cityk are industry fixed effects and city fixed effects, respectively; and μkji is a random disturbance term. In the above model, α1 is the coefficient of interest. If it is statistically significantly positive, firms improve their Internet applications and enhance their process innovation. As this study uses cross-sectional data, heteroskedasticity-robust standard errors are used in the regression to avoid the effect of heteroskedasticity on the estimation results.
3.3.2. Mediating effect model
In this study, we draw on Wen and Ye (Citation2014) on the mediating effect test method to construct a sequential recursive model to test the mechanism of the impact of Internet application on process innovation. The baseline model is constructed without mediating the variables in the first step, that is, the baseline regression in EquationEq. (1)(1)
(1) . Then, in the second step, the effect of the core explanatory variable IAkji on the mediating variable is tested with the mediating variable Mkji as the explanatory variable, that is, EquationEq. (2)
(2)
(2) :
(2)
(2)
If the coefficient of β1 in EquationEq. (2)(2)
(2) and the coefficient of γ2 in EquationEq. (3)
(3)
(3) are significant, then an indirect effect exists. If the indirect effect holds, then the third step is to judge whether there is a direct effect according to the significance of the coefficient of γ1 in EquationEq. (3)
(3)
(3) . If the coefficient of γ1 is not insignificant, then only the indirect effect exists. If the coefficient of γ1 is significant, then there is a direct effect. In the case in which the direct impact holds, we compare β1 × γ2 with γ1 in terms of sign consistency, according to which we judge whether there is a partial mediating effect.
(3)
(3)
4. Empirical results and analysis
4.1. Baseline regression results and average marginal effect
The baseline regression results are presented in . A variance inflation factor test is performed on the regression model, and all independent variables are less than the standard critical value of 10 for multicollinearity. By contrast, the Poisson regression goodness-of-fit (poisgof) test for the number of process innovations shows that the bias goodness-of-fit and Pearson goodness-of-fit tests are statistically insignificant, thus confirming the appropriateness of using the Poisson distribution. Columns (1) and (2) present the test results for the relationship between whether firms apply the Internet and the number of process innovations. Poisson regression results indicate that with the inclusion of firm-level control variables, industry fixed effects, and city fixed effects, Internet application can significantly increase the number of process innovations relative to the case in which firms do not use the Internet. In terms of the incidence rate ratio (IRR), firms applying the Internet can enhance the number of process innovations by 114.0%. Columns (3) and (4) present the results of the relationship between Internet applications and the number of process innovations, which show that the regression results are significantly positively correlated. From the perspective of the IRR, an increase of one unit of Internet application by firms will increase the number of process innovations by11.05%. In this study, the Tobit model is used to regress the quality of process innovation. Column (5) shows that firms that apply the Internet can significantly improve the quality of process innovation relative to those that do not use the Internet. Meanwhile, column (6) shows that there is a significant positive correlation between Internet application and the quality of process innovation at the 1% statistical level. Hypothesis H1 of this study is thus tentatively confirmed. These results are largely consistent with those of earlier studies (Galati & Bigliardi, Citation2019; Neirotti & Pesce, Citation2019), but the current study distinguishes the impact of Internet applications on the quantity and quality of process innovation in more detail.
Table 2. Baseline regression results of Internet application affecting process innovation.
The Poisson and Tobit models are nonlinear regression models that cannot directly explain the effects on explanatory variables in terms of the coefficients of the independent variables; therefore, this study analyses the average marginal effect of Internet applications on the quantity and quality of process innovation. The regression results in columns (1) and (3) of show that the average marginal effect of Internet application on the quantity of process innovation is greater than the effect on the quality of process innovation from the perspective of whether or not the Internet is used (IAdum). Columns (2) and (4) of show that the average marginal effect of Internet application on the quantity of process innovation is greater than the effect on the quality of process innovation from the perspective of the degree of Internet application (IA). Thus, Internet applications promote process innovation but with heterogeneity, and the above analysis reinforces the basic hypothesis of this study (H1). The quantity of process innovation is primarily a reflection of the degree of innovation effort undertaken by firms to reduce marginal production costs (Engelstätter, Citation2012), whereas the quality of process innovation is a reflection of the degree of market realisation of the outcome of the process innovation effort undertaken, that is, the market value of process innovation (Haner, Citation2002; Yang, Citation2013). However, there is a time lag between process innovation and annual firm sales, and annual firm sales are subject to product innovation, organisational innovation, and so on (Neirotti & Pesce, Citation2019; Roberts et al., Citation2022). This may be the main reason why the impact of Internet applications on the quantity of process innovation is greater than the impact on the quality of process innovation.
Table 3. Average marginal effect estimation results.
4.2. Endogeneity analysis
This study attempts to mitigate the possible endogeneity problem between Internet adoption and process innovation using an instrumental variable approach. Drawing on Khalid et al. (Citation2022) treatment of the endogeneity problem, this study uses the average value of the information and communication technology (ICT) of other firms in the same city and industry as an instrumental variable for firms’ Internet adoption. It serves as an indicator that is related to the characteristics of each firm but is less likely to influence firms’ innovation decisions. In this study, model (1) is estimated using two-stage least squares regression. The results are presented in . The results of the one-stage regression in columns (1) and (3) indicate that the instrumental variable is significantly and positively correlated with the endogenous variable at the 1% level, thus satisfying the correlation hypothesis. The results of the two-stage regression in columns (2) and (4) show that the sign of the coefficient of the firm Internet application variable is positive at the 1% level, which is consistent with the baseline regression results. This study uses the Kleibergen and Paap Lagrange multiplier statistic for the instrumental variable unidentifiability test; the Cragg–Donald Wald F statistic and the Kleibergen–Paap rk Wald F statistic for the weak instrumental variable test; and the Anderson–Rubin Wald test for the weak identification robust test on the instrumental variables. The test results significantly reject the original hypothesis, indicating that there is a strong correlation between the instrumental variables selected in this study and the endogenous variables and that the instrumental variables are set reasonably and effectively. Furthermore, the regression results of the introduced instrumental variables are consistent with those of the baseline regression, and the estimation results are robust.
Table 4. Regression results of instrumental variables.
4.3. Heterogeneity analysis
4.3.1. Considering the impact of differences in firm size
There are significant differences in the innovation behaviour of firms of different sizes. According to the World Bank’s definition of surveyed firms, firms with more than 100 employees are defined herein as large firms, and those with fewer are regarded as small- and medium-sized enterprises (SMEs). Group regressions are then conducted, and the results are shown in . The results show that Internet application is significantly and positively correlated with the quantity and quality of process innovation and are consistent with the baseline regression results. From the analysis of the average marginal effect, the impact of Internet application on the quantity of process innovation in manufacturing firms is greater than the impact on the quality of process innovation. In terms of the quantity of process innovation, the marginal effect of the Internet application of SMEs is not significantly different from that of large firms. However, in terms of the quality of process innovation, the marginal effect of the Internet application of large firms is higher than that of SMEs This result indicates that large firms pay more attention to improving the quality of process innovation through the application of the Internet because of their focus on the construction of standardised processes, which make the annual output of firms more correlated with process innovation.
Table 5. Heterogeneity analysis for distinguishing firm size differences.
4.3.2. Considering the effect of industry differences
Drawing on the study of Lyu and Wang (Citation2015), we conduct a cluster analysis of industries on the basis of the proportion of R&D expenditures and the ratio of fixed assets to classify manufacturing industries into labour-intensive, capital-intensive, and technology-intensive categories. We further examine the impact of Internet applications on process innovation through a subsample regression. The regression results are shown in . The control variables no longer include industry factors. Consistent with the primary regression results, the regression outcomes show that Internet application positively impacts the quantity and quality of process innovation in different industries at the 1% level. However, the degree of impact has some variability. For example, in terms of the quantity of process innovation, the regression coefficient of Internet application is slightly higher for labour-intensive firms, followed by technology-intensive firms and capital-intensive firms. This result indicates that due to the scarcity of capital, labour-intensive and technology-intensive firms are more inclined to produce with labour and technology instead of wealth-utilising process innovation. Meanwhile, the regression results of process innovation quality are significantly different, as the regression coefficients of labour-intensive firms are substantially lower than those of capital- and technology-intensive firms. This indicates that capital- and technology-intensive firms pay more attention to the integration of process innovation and production operations.
Table 6. Heterogeneity analysis for distinguishing industry differences.
4.3.3. Considering the effect of firm ownership differences
The resources, objectives, and governance of SOEs are very different from those of non-SOEs, and differences in corporate ownership (SOEs) can also have an impact on process innovation (Amin et al., Citation2019). In this study, companies with government ownership greater than 50% are identified as SOEs whilst the rest are regarded as non-SOEs. The regression results are shown in . The control variables no longer include the ownership factor. The regression results show that Internet application has a positive effect on both the quantity and quality of process innovation in different industries at the 1% level, in line with the basic regression results. In terms of the quantity of process innovation, the marginal effect of Internet application is slightly higher for non-SOEs than for SOEs. As for the quality of process innovation, the marginal effect of Internet application is significantly higher for SOEs than for non-SOEs. On the one hand, SOEs have a stable market and financing environment and their production and innovation do not rely primarily on participation in Internet-enabled markets. Non-SOEs, on the other hand, operate to chase profits and focus more on using the Internet for internal and external information interaction and assistance in the Internet transformation process; in this way, they can reduce their innovation costs and risks.
Table 7. Heterogeneity analysis for distinguishing ownership differences.
4.3.4. Considering the effect of region differences
Regions differ in terms of knowledge spillover, resources, and government support (Tsinopoulos et al., Citation2018), and the differences can impact firms’ process innovation. The survey data used in this study cover 25 major cities in central and eastern China, including Beijing, Shanghai, Guangzhou, Hefei, and Lanzhou. The sample is divided into the midwest (Midwest) and east (East) regions according to the administrative regions of China, and the impact of Internet application on process innovation is further examined through subsample regression. The regression results are presented in . In line with the basic regression results, the empirical results indicate that Internet application exerts a positive impact on both the quantity and quality of process innovation in different regions at the 1% level. In terms of the quantity of process innovation, the marginal effect of Internet application is significantly higher in the eastern region than in the central and western regions probably because the eastern region of China has a more complete Internet infrastructure, a relatively high concentration of high-level universities and research institutions, and advantages in terms of knowledge spillover and government support (Chen et al., Citation2022). However, there are no significant regional differences in the quality of process innovation. This suggests that firms in all regions focus on the integration of process innovation and production operations and make good use of Internet conditions to streamline the sales process and reduce sales costs (Dan et al., Citation2014).
Table 8. Heterogeneity analysis for distinguishing regions’ differences.
4.4. Mediating effect test
In this study, the mediating effects are tested separately in terms of the quantity and quality of process innovation. Panel A of reports the results of the quantitative test of process innovation. To enhance the quantitative effect of process innovation caused by a firm’s Internet application, we present the test results in column (1), which indicate that a firm’s Internet application positively affects the quantity of process innovation at the 1% significance level, thus confirming the existence of a mediating effect of a firm’s Internet application on the quantity of process innovation. Columns (2) and (4) show the test results for EquationEq. (2)(2)
(2) and indicate that the coefficient of Internet application is significantly positive at the 1% significance level. This result implies that a firm’s Internet application significantly expands its open innovation and enhances its information technology capability. Columns (3) and (5) show the test results for EquationEq. (3)
(3)
(3) and indicate that the regression coefficients of the mediating variables are significantly positive. This result implies that open innovation and information technology capabilities also promote the number of process innovations in firms, thus confirming the existence of an indirect effect of the model. The consistency of the sign of the product (β1 × γ2) of the mediating variables in EquationEqs. (2)
(2)
(2) and Equation(3)
(3)
(3) with the coefficient γ1 of EquationEq. (2)
(2)
(2) in Panel A of confirms the existence of a partial mediating effect in the model. Hypotheses H2 and H3 are confirmed from the perspective of the quantity of process innovation. Similar to the analysis above, Panel B of reports the results of the test of the mediating effects in terms of the quality of process innovation in manufacturing. The results confirm hypotheses H2 and H3 from the perspective of the quality of process innovation.
Table 9. Results of intermediate effect test.
5. Conclusion
This study analysed the impact of Internet application on process innovation in manufacturing firms and its mechanism of action in terms of the quantity and quality of process innovation using microfirm survey data. The results show that Internet application has a significant promotion effect on the quantity and quality of process innovation. Nevertheless, from the perspective of average marginal effect, the promotion effect of Internet application on the quantity of process innovation is more significant than that on quality of process innovation. Heterogeneity analysis finds that in terms of the quantity of process innovation, Internet application has a strong promotion effect on small and medium-sized firms and labour-intensive firms; in terms of the quality of process innovation, Internet application exerts a more substantial promoting effect on large firms and technology-intensive firms. The mechanism test reveals that Internet application promotes process innovation by expanding open innovation and improving the level of information technology capability. This study finds that firms can broaden their external sources of information and knowledge to drive process innovation through open innovation. Process innovations are difficult to reverse engineer (James et al., Citation2013), take longer to develop (Damanpour & Gopalakrishnan, Citation2001), and are tacit and systematic in nature (Terjesen & Patel, Citation2017). Informational capabilities, by contrast, enhance firms’ ability to acquire, assimilate, and use external knowledge and information to improve manufacturing processes.
In the deep application of the Internet in the firm innovation process, process innovation serves as the driving force of ‘Internet + manufacturing’ and gives full play to its inherent role in technological progress. Internet application in the manufacturing industry helps the proliferation and recombination of ‘fragmented’ knowledge and the formation of a completely integrated knowledge system. Internet application has also prompted a change in the way firms innovate, emphasising the acquisition and reconfiguration of innovation resources. The government should strengthen multilateral dialogue and cooperation, establish a multilevel communication and dialogue mechanism with firms as the main body and with the government’s participation, strengthen the cross-border flow of innovation resources amongst different innovation bodies, and expand the open innovation of firms.
Our study has some limitations that may open up future research directions. Firstly, it analyses the differential impact of Internet application on the quantity and quality of process innovation and their mechanisms of action using data from a 2012 World Bank survey of Chinese manufacturing firms, which was conducted in a specific context that may limit the generalisability of the findings. It would be meaningful to explore the impact of Internet application on process innovation in other geographic contexts or industries. Secondly, this study does not consider the harmful effects of the COVID-19 pandemic on the global integration of production and supply, which may hinder open innovation in multinational firms (Ciravegna & Michailova, Citation2022). It would also be valuable to further explore the impact of Internet application on process innovation in this context.
Disclosure statement
The authors report there are no competing interests to declare.
Additional information
Funding
References
- Agostini, L., & Filippini, R. (2019). Organizational and managerial challenges in the path toward Industry 4.0. European Journal of Innovation Management, 22(3), 406–421. https://doi.org/10.1108/EJIM-02-2018-0030
- Agostini, L., Galati, F., & Gastaldi, L. (2020). Open innovation: Are absorptive and desorptive capabilities complementary. European Journal of Innovation Management, 23(1), 1–12. https://doi.org/10.1108/EJIM-11-2019-0330
- Agostini, L., & Nosella, A. (2019). Inter-organizational relationships involving SMEs: A bibliographic investigation into the state of the art. Long Range Planning, 52(1), 1–31. https://doi.org/10.1016/j.lrp.2017.12.003
- Akcigit, U., Grigsby, J., Nicholas, T., & Stantcheva, S. (2022). Taxation and innovation in the twentieth century. Quarterly Journal of Economics, 137(1), 329–385. https://doi.org/10.1093/qje/qjab022
- Aliasghar, O., & Haar, J. (2021). Open innovation: Are absorptive and desorptive capabilities complementary? International Business Review, 101865. https://doi.org/10.1016/j.ibusrev.2021.101865
- Aliasghar, O., Rose, E. L., & Chetty, S. (2019a). Where to search for process innovations? The mediating role of absorptive capacity and its impact on process innovation. Industrial Marketing Management, 82, 199–212. https://doi.org/10.1016/j.indmarman.2019.01.014
- Aliasghar, O., Rose, E. L., & Chetty, S. (2019b). Building absorptive capacity through firm openness in the context of a less-open country. Industrial Marketing Management, 83, 81–93. https://doi.org/10.1016/j.indmarman.2018.11.007
- Amin, M. Y., Besim, M., & Ul Haq, Z. (2019). Does state ownership really matter for capital structure in selected G-20 economies? Economic Research-Ekonomska Istraživanja, 32(1), 3150–3167. https://doi.org/10.1080/1331677X.2019.1661000
- Badrinarayanan, V., Ramachandran, I., & Madhavaram, S. (2019). Resource orchestration and dynamic managerial capabilities: Focusing on sales managers as effective resource orchestrators. Journal of Personal Selling & Sales Management, 39(1), 23–41. doi: https://doi.org/10.1080/08853134.2018.1466308
- Battistella, C., De Toni, A., & Pessot, E. (2018). Framing open innovation in start-ups’ incubators: A complexity theory perspective. Journal of Open Innovation: Technology, Market, and Complexity, 4(3), 33. doi: https://doi.org/10.3390/joitmc4030033.
- Bloom, N., Garicano, L., Sadun, R., & Van Reenen, J. (2014). The distinct effects of information technology and communication technology on firm organization. Management Science, 60(12), 2859–2885. https://doi.org/10.1287/mnsc.2014.2013
- Bulut, C., Kaya, T., Mehta, A. M., & Danish, R. Q. (2022). Linking incremental and radical creativity to product and process innovation with organisational knowledge. Journal of Manufacturing Technology Management, 33(4), 763–784. https://doi.org/10.1108/JMTM-01-2021-0037
- Bygstad, B., & Aanby, H. P. (2010). ICT infrastructure for innovation: A case study of the enterprise service bus approach. Information Systems Frontiers, 12(3), 257–265. https://doi.org/10.1007/s10796-009-9169-9
- Caldera, A. (2010). Innovation and exporting: Evidence from Spanish manufacturing firms. Review of World Economics, 146(4), 657–689. https://doi.org/10.1007/s10290-010-0065-7
- Chang, V. (2018). A proposed social network analysis platform for big data analytics. Technological Forecasting and Social Change, 130, 57–68. https://doi.org/10.1016/j.techfore.2017.11.002
- Chen, L., Hasan, R., & Jiang, Y. (2022). Urban agglomeration and firm innovation: Evidence from Asia. The World Bank Economic Review, 36(2), 533–558. https://doi.org/10.1093/wber/lhab022
- Chuks, O. (2022). IT capability, organisational learning and innovation performance of firms in Kenya. Journal of the Knowledge Economy, 1–29. https://doi.org/10.1007/s13132-021-00886-8
- Ciravegna, L., & Michailova, S. (2022). Why the world economy needs, but will not get, more globalization in the post-COVID-19 decade. Journal of International Business Studies, 53(1), 172–186. https://doi.org/10.1057/s41267-021-00467-6
- Damanpour, F., & Gopalakrishnan, S. (2001). The dynamics of the adoption of product and process innovations in organizations. Journal of Management Studies, 38(1), 45–65. https://doi.org/10.1111/1467-6486.00227
- Dan, B., Qu, Z. J., Liu, C., Zhang, X. M., & Zhang, H. Y. (2014). Price and service competition in the supply chain with both pure play internet and strong bricks-and-mortar retailers. Journal of Applied Research and Technology, 12(2), 212–222. https://doi.org/10.1016/S1665-6423(14)72337-5
- Engelstätter, B. (2012). It is not all about performance gains—Enterprise software and innovations. Economics of Innovation and New Technology, 21(3), 223–245. https://doi.org/10.1080/10438599.2011.562359
- Galati, F., & Bigliardi, B. (2017). Does different NPD project’s characteristics lead to the establishment of different NPD networks? A knowledge perspective. Technology Analysis & Strategic Management, 29(10), 1196–1209. https://doi.org/10.1080/09537325.2016.1277581
- Galati, F., & Bigliardi, B. (2019). Industry 4.0: Emerging themes and future research avenues using a text mining approach. Computers in Industry, 109, 100–113. https://doi.org/10.1016/j.compind.2019.04.018
- Gao, W., & Bi, K. X. (2014). An empirical study on the relationship between informatization level and process innovation capability in manufacturing enterprise. Science of Science and Management of S & T, 35(8), 96–103. Retrieved from https://doi.org/10.1145/3473714.3473795
- Gil-Gomez, H., Guerola-Navarro, V., Oltra-Badenes, R., & Lozano-Quilis, J. A. (2020). Customer relationship management: Digital transformation and sustainable business model innovation. Economic Research-Ekonomska Istraživanja, 33(1), 2733–2750. https://doi.org/10.1080/1331677X.2019.1676283
- Gopalakrishnan, S., Bierly, P., & Kessler, E. H. (1999). A reexamination of product and process innovations using a knowledge-based view. The Journal of High Technology Management Research, 10(1), 147–166. https://doi.org/10.1016/S1047-8310(99)80007-8
- Guo, J., Zhou, S., Chen, J., & Chen, Q. (2021). How information technology capability and knowledge integration capability interact to affect business model design: A polynomial regression with response surface analysis. Technological Forecasting and Social Change, 170, 120935. https://doi.org/10.1016/j.techfore.2021.120935
- Haner, U. E. (2002). Innovation quality—A conceptual framework. International Journal of Production Economics, 80(1), 31–37. https://doi.org/10.1016/S0925-5273(02)00240-2
- Huang, E. Y., & Lin, C. Y. (2005). Customer-oriented financial service personalization. Industrial Management & Data Systems, 105(1), 26–44. https://doi.org/10.1108/02635570510575171
- Hullova, D., Trott, P., & Simms, C. D. (2016). Uncovering the reciprocal complementarity between product and process innovation. Research Policy, 45(5), 929–940. https://doi.org/10.1016/j.respol.2016.01.012
- Hwang, B.-N., & Hsu, M.-Y. (2019). The impact of technological innovation upon servitization: Evidence from Taiwan community innovation survey. Journal of Manufacturing Technology Management, 30(7), 1097–1114. https://doi.org/10.1108/JMTM-08-2018-0242
- James, S. D., Leiblein, M. J., & Lu, S. (2013). How firms capture value from their innovations. Journal of Management, 39(5), 1123–1155. https://doi.org/10.1177/0149206313488211
- Khalid, F., Naveed, K., Nawaz, R., Sun, X., Wu, Y., & Ye, C. (2022). Does corporate green investment enhance profitability? An institutional perspective. Economic Research-Ekonomska Istraživanja, 1–24. https://doi.org/10.1080/1331677X.2022.2063919
- Kim, J. (2022). Innovation failure and firm growth: Dependence on firm size and age. Technology Analysis & Strategic Management, 34(2), 166–179. https://doi.org/10.1080/09537325.2021.1892622
- Lam, H. K. S., Yeung, A. C. L., & Cheng, T. C. E. (2016). The impact of firms’ social media initiatives on operational efficiency and innovativeness. Journal of Operations Management, 47-48(1), 28–43. https://doi.org/10.1016/j.jom.2016.06.001
- Lambertini, L. (2004). Process and product R&D by a multiproduct monopolist: A reply to Lin. Oxford Economic Papers, 56(4), 745–749. https://doi.org/10.1093/oep/gpf066
- Laursen, K., & Salter, A. (2006). Open for innovation: The role of openness in explaining innovation performance among UK manufacturing firms. Strategic Management Journal, 27(2), 131–150. https://doi.org/10.1002/smj.507
- Lin, C., Lin, P., & Song, F. (2010). Property rights protection and corporate R&D: Evidence from China. Journal of Development Economics, 93(1), 49–62. https://doi.org/10.1016/j.jdeveco.2009.04.006
- Lo Turco, A., & Maggioni, D. (2015). Dissecting the impact of innovation on exporting in Turkey. Economics of Innovation and New Technology, 24(4), 309–338. https://doi.org/10.1080/10438599.2014.946311
- Lyu, T., & Wang, H. C. (2015). Influence of labor market regulation on enterprise technical innovation: Analysis on data of World Bank’s survey on Chinese enterprises. Chinese Journal of Population Science, 04, 32–46. + 127.
- Mai, A. N., Van Vu, H. V., Bui, B. X., & Tran, T. Q. (2019). The lasting effects of innovation on firm profitability: Panel evidence from a transitional economy. Economic Research-Ekonomska Istraživanja, 32(1), 3417–3436. https://doi.org/10.1080/1331677X.2019.1660199
- Maniaci, N. D. (1990). Made in America: Regaining the productive edge. Business Economics, 25(4), 61–63.
- Martínez-Ros, E. (2019). Revisiting product and process innovations. International Journal of Business Environment, 10(3), 270. https://doi.org/10.1504/IJBE.2019.10019357
- Meyer, C., & Schwager, A. (2007). Understanding customer experience. Harvard Business Review, 85(2), 116–126, 157.
- Neirotti, P., & Pesce, D. (2019). ICT-based innovation and its competitive outcome: The role of information intensity. European Journal of Innovation Management, 22(2), 383–404. https://doi.org/10.1108/EJIM-02-2018-0039
- Nwankpa, J. K., Roumani, Y., & Datta, P. (2022). Process innovation in the digital age of business: The role of digital business intensity and knowledge management. Journal of Knowledge Management, 26(5), 1319–1341. https://doi.org/10.1108/JKM-04-2021-0277
- Pereira, R. M., Borini, F. M., & Oliveira, M. Jr., (2019). Interorganizational cooperation and process innovation. Journal of Manufacturing Technology Management, 31(2), 260–283. doi: https://doi.org/10.1108/jmtm-12-2018-0430
- Pérez, J. A. H., Yang, X., Bai, O., Flores, A., & Heredia, W. H. (2019). How does competition by informal firms affect the innovation in formal firms? International Studies of Management & Organization, 49(2), 173–190. https://doi.org/10.1080/00208825.2019.1608402
- Piening, E. P., & Salge, T. O. (2015). Understanding the antecedents, contingencies, and performance implications of process innovation: A dynamic capabilities perspective. Journal of Product Innovation Management, 32(1), 80–97. https://doi.org/10.1111/jpim.12225
- Qu, L., & Li, Y. (2019). Research on industrial policy from the perspective of demand-side open innovation—A case study of Shenzhen new energy vehicle industry. Journal of Open Innovation: Technology, Market, and Complexity, 5(2), 31. https://doi.org/10.3390/joitmc5020031
- Reichstein, T., & Salter, A. (2006). Investigating the sources of process innovation among UK manufacturing firms. Industrial and Corporate Change, 15(4), 653–682. https://doi.org/10.1093/icc/dtl014
- Roberts, D. L., Palmer, R., & Hughes, M. (2022). Innovating the product innovation process to enable co‐creation. R&D Management, 52(3), 484–497. https://doi.org/10.1111/radm.12492
- Simonen, J., & McCann, P. (2008). Innovation, R&D cooperation and labor recruitment: Evidence from Finland. Small Business Economics, 31(2), 181–194. https://doi.org/10.1007/s11187-007-9089-3
- Terjesen, S., & Patel, P. C. (2017). In search of process innovations: The role of search depth, search breadth, and the industry environment. Journal of Management, 43(5), 1421–1446. https://doi.org/10.1177/0149206315575710
- Tsinopoulos, C., Sousa, C. M. P., & Yan, J. (2018). Process innovation: Open innovation and the moderating role of the motivation to achieve legitimacy. Journal of Product Innovation Management, 35(1), 27–48. https://doi.org/10.1111/jpim.12374
- Utterback, J. M., & Abernathy, W. J. (1975). A dynamic model of process and product innovation. Omega, 3(6), 639–656. https://doi.org/10.1016/0305-0483(75)90068-7
- Wang, W. N., Liu, J. J., & Zhang, Z. K. (2020). R&D internalization, financial constraints and technological innovation of manufacturing enterprises. Business and Management, 42(09), 127–143. https://doi.org/10.19616/j.cnki.bmj.2020.09.008
- Wareham, J., Fox, P. B., & Cano Giner, J. L. (2014). Technology ecosystem governance. Organization Science, 25(4), 1195–1215. https://doi.org/10.1287/orsc.2014.0895
- Wen, Z., & Ye, B. (2014). Analyses of mediating effects: The development of methods and models. Advances in Psychological Science, 22(5), 731–745. https://doi.org/10.3724/SP.J.1042.2014.00731
- Wernerfelt, B. (1984). A resource-based view of the firm. Strategic Management Journal, 5(2), 171–180. doi: https://doi.org/10.1002/smj.4250050207
- West, J., & Bogers, M. (2014). Leveraging external sources of innovation: A review of research on open innovation. Journal of Product Innovation Management, 31(4), 814–831. https://doi.org/10.1111/jpim.12125
- Wittfoth, S., Berger, T., & Moehrle, M. G. (2022). Revisiting the innovation dynamics theory: How effectiveness- and efficiency-oriented process innovations accompany product innovations. Technovation, 112, 102410. https://doi.org/10.1016/j.technovation.2021.102410
- Woltjer, G., van Galen, M., & Logatcheva, K. (2021). Industrial innovation, labour productivity, sales and employment. International Journal of the Economics of Business, 28(1), 89–113. https://doi.org/10.1080/13571516.2019.1695448
- Yang, D. M., & Liu, Y. W. (2018). Why can Internet Plus increase performance. China Industrial Economics, 5, 80–98. https://doi.org/10.19581/j.cnki.ciejournal.2018.05.005-en
- Yang, Y. H. (2013). Theoretical framework of innovation quality: Concept, connotation and characteristics. Science Research Management, 2013(s1), 320–325.
- Yang, Z. N., & Zhao, H. (2020). Chinese enterprises’ open innovation: Institutional environment, co-opetition relationship and innovation performance. Management World, 36(02), 139–160. https://doi.org/10.19744/j.cnki.11-1235/f.2020.0027
- Zhang, S. F., & Wei, X. H. (2019). Does information and communication technology reduce enterprise’s energy consumption—Evidence from Chinese manufacturing enterprises survey. China Industrial Economics, 2, 155–173. https://doi.org/10.19581/j.cnki.ciejournal.2019.02.013
- Zhao, S. C., Zeng, D. M., & Luo, Z. (2020). The effect of scientific and technical cooperation on firms’ innovation performance: Based on the perspective of quantity and quality. Science of Science and Management of S & T, 41(1), 33–48. 10.3389/fpubh.2022.980845
- Zhu, F., Li, Q., Yang, S., & Balezentis, T. (2021). How ICT and R&D affect productivity? Firm level evidence for China. Economic Research-Ekonomska Istraživanja, 34(1), 3468–3486. https://doi.org/10.1080/1331677X.2021.1875861