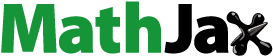
Abstract
Through a production process based on low carbon emissions, achieving sustainable development is the desired goal of the various social and political actors around the world. However, achieving this requires energy policies focused on the factors that determine green economic growth (GEG). Consequently, the current study investigates the long-run nexus between GEG and some factors that determine its behaviour using annual data from 1990 to 2020 for the Organization for Economic Co-operation and Development. The empirical evidence relies on second-generation estimators comprising cross-sectional dependence tests, Westerlund tests, and method of moment quantile regression. Feedbacks from the empirical analyses uncover the pertinence of energy efficiency, renewable energy, and technology as positive predictors of GEG. Complementarily, the findings reveal that the Integrity of the Government plays an essential role in implementing more efficient and environmentally friendly production processes. From the results found, several policy suggestions are derived from being considered to develop an energy policy aimed at mitigating environmental degradation.
1. Introduction
For time immemorial, energy resources have been widely recognized as fundamental factors that drive economic growth in both developed and developing economies (Nathaniel et al., Citation2021; Shahbaz et al., Citation2021). The pertinence of these resources is more evident in the various ways they are utilized across all facets of the economy, including production of goods and services, construction and building activities, provision of infrastructure facilities, and domestic usages such as lighting, cooking, and heating, among others (Cotton et al., Citation2021; Roy et al., Citation2021). All the preceding activities emphasize that energy resources are inevitable and inseparable from economic growth and development. That notwithstanding, the utilization of energy resources for the benefit of the economy is never without their costs that are more apparent on the environment (Mohsin et al., Citation2021; Musa et al., Citation2021; Yu, Khan, et al., Citation2022). Among many other consequences, the environmental impacts of energy consumption have been extensively discussed in the literature for at least three reasons. First, it is widely established that energy consumption from fossil fuels constitutes the most substantial contributor to global greenhouse gas (GHG) emissions, with over 70% (Sadik-Zada & Gatto, Citation2021). Second, scholars have realized that combating global climate change is impossible without significantly reducing fossil fuel consumption and embracing a pathway that promotes cleaner energy consumption and transition to renewable energy (Luo et al., Citation2022; Semieniuk et al., Citation2021). Third, delivering on the targets of sustainable development goals (SDGs) by 2030 will remain a mirage if carbon-intensive energy sources are not substantially reduced or eliminated (Awan, Citation2021; Bao et al., Citation2022; Yu, Ridwan, et al. 2022). The above instances suggest that mitigating the continuous surge in carbon emissions is at the core of numerous empirical research and development agendas pursued by policymakers, international organizations, and research pundits alike.
The emerging efforts towards addressing the challenges posed by carbon emissions and other related variants of GHG have seen the advent of numerous initiatives and advocacies in recent times, among which carbon-neutrality (CO2N) and green economic growth (GEG) are not hard to notice. The concept of CO2N posits the process of maintaining equilibrium between carbon emitted and absorbed in a way that promotes a carbon-free environment (Ponce & Khan, Citation2021; Shen et al., Citation2021). The pathway to attaining CO2N cannot do without adopting the green growth philosophy for four reasons. First, GEG is a concept that advocates embracing a development path that is environmentally friendly and sustainable (Hao et al., Citation2021; Zhao et al., Citation2022). Second, GEG is based on a widely held view that since economic growth constitutes an overall goal for every country, recording fewer environmental pollutants becomes a fundamental condition that is not negligible (Khan et al., Citation2022; Meirun et al., Citation2021). Third, GEG promotes low-carbon emission content and enhances sustainable development (Hao et al., Citation2021; Martinez & Poveda, Citation2021). Fourth, a well-structured and implemented GEG policy holds the possibility to improve employment opportunities through the promotion of activities in selected sectors like green agriculture, renewable energy, and sustainable forestry (Baş, Citation2021; Dordmond et al., Citation2021). Globally, GEG remains a recent policy priority for international organizations like World Bank, Organisation for Economic Co-operation and Development (OECD), and United Nations (Allan & Meckling, Citation2021).
Worthy of note from the preceding assertions on GEG is the fact that despite the centrality of the concept to achieving CO2N and sustainable development, the idea remains an evolving research area in the growth and development empirics with a limited understanding of its intricacies (Hao et al., Citation2021; Herman, Citation2021). Besides, GEG cannot be attained by coincidence; instead with an appropriate policy focus on certain driving factors, including energy efficiency, renewable energy, technology innovation, financial freedom, and Integrity of Government. These factors play significant roles in promoting green growth initiatives across developing and developed economies. For instance, energy efficiency (EEF), which involves the utilization of less energy in performing similar tasks in a way that eliminates energy wastes and moderates carbon emissions, has been empirically advanced as a critical factor that promotes GEG (Akram et al., Citation2022; Lei et al., Citation2022; Mahapatra & Irfan, Citation2021). The assertion is based on the ground that with a reduced volume of energy consumption compared with an increased growth rate, the associated carbon-embedded growth outcomes are lessened through the process called decoupling (Wang & Su, Citation2020; Wu et al., Citation2019). These processes all promote GEG and sustainable development.
Furthermore, it is pertinent to note that the pursuit of GEG also requires that an economy thread a path of transition to renewable energy (REN). The reason is that RE remains one of the most effective and efficient ways of achieving a green economy because of its significant impact on moderating environmental pollution (Chen et al., Citation2022; He et al., Citation2019; Naqvi et al., Citation2021). Besides, REN being a low-carbon content energy resource, is clean, cheap, affordable, and accessible for multipurpose utilization comprising production activities, agriculture and non-agriculture activities, domestic utilization, services, and other productive use (Croutzet & Dabbous, Citation2021; Maksoud et al., Citation2021). The associated benefits of RE enhance the achievement of increasing economic growth rate that is eco-friendly and equally secures future generations’ sustainability without undermining the present’s interest (Oke et al., Citation2021). Some notable studies have empirically provided evidence to support the GEG-inducing effects of REN (Hao et al., Citation2021; Sohag et al., Citation2021). Elucidating on the technology innovation, it is instructive to note that the role of technology in the growth and environment empirics can hardly be overemphasized. Theoretically, the augmented exogenous and endogenous growth theories postulated the critical role of technology in achieving sustained economic growth (Alam & Ullah, Citation2021; Tadele & Sirany, Citation2021). Moreover, in promoting GEG, technology innovation remains a significant force to be reckoned with (Ji et al., Citation2021; Nosheen et al., Citation2021). The importance of technology innovation cuts across all facets of the green economy and remains a crucial factor that promotes their drivers. For instance, factors such as energy efficiency (EEF), clean energy (CE), renewable energy (REN), and nuclear energy (NE) all required an interplay of a well-sophisticated technology to thrive (Chen et al., Citation2021; Ibrahim & Ajide, Citation2021).
The issue of green growth financing is another critical concept reverberating at the centerpiece of academic discussion on the green economy, environmental sustainability, and sustainable environment, among others. Recognizing this fact, international organizations such as the United Nations climate objectives agreed upon at the ‘Paris Climate Agreement (COP 21, 2015)’ set a benchmark investment plan of US$100 Billion in combating climate issues (Liu et al., Citation2021). Besides, an estimated US$1.6 trillion annually has been projected as an energy system requirement for years spanning 2020 through 2050 (Schumacher et al., Citation2020). It is pertinent to note that while the achievement of a green economy requires massive investment funds from both public and private investors, the latter has increasingly constituted essential stakeholders in recent times (Semieniuk & Mazzucato, Citation2019). For private investors to continuously promote investment in green economy, they have to be financially independent. Hence, the role of financial freedom becomes highly sacrosanct in the finance-led GEG (Akinci et al., Citation2015). The achievement of green growth and the environmental agenda of any form lies in the game’s rules governed by institutions (Egbetokun et al., Citation2020). Consequently, this study argues that the Government, which is at the frontline of policy implementation, requires some significant level of confidence attainable through Integrity of high pedigree. The reason is that Integrity remains a crucial factor in earning public trust and confidence (Huberts, Citation2018; Liu et al., Citation2020). In order words, government integrity implies that the Government must exhibit some levels of loyalty, truthfulness, and kindness (Du et al., Citation2018). Based on the initial submissions, this study posits that government integrity (GIN) remains an essential factor that should be given the utmost attention in any policy initiative to achieve GEG.
Following the preceding narratives, this study avers that despite the criticality of the highlighted factors of GEG drivers, empirical evidence advancing their pertinence is budding, thus suggesting that research studies into them are in the early stage. This makes the debate broader for the present study to further examine the highlighted issues of concern to enhance a more comprehensive understanding and extend the frontier of knowledge accordingly.
1.2. Research objective/contributions
The primary objective of this study is to examine how energy efficiency (EEF), renewable energy (REN), technology innovation (TEC), financial freedom (FFR), industrialization (IND), and government integrity (GIN) promote the GEG of the OECD countries by relying on empirical data straddling 1990–2020. A series of econometric methods is applied to examine the study variables with precedence given to the novel method of moments quantile regression (MMQR) approach created and developed by Machado and Silva (Citation2019). The contribution of this study to the extant literature is four-fold. These are enlisted thus;
The study contributes to understanding the crucial roles of energy efficiency and renewable energy in OECD’s roadmap to green economy. These countries have been at the frontline of supporting and advocating efforts towards promoting sustainable development (Palit & Hussain, Citation2021).
Second-generation estimators robust for heterogeneous slope coefficient and cross-sectional dependence are employed. Further, the median of the conditional distribution of GEG is examined at different quantile points, allowing to explore green growth’s asymmetric behaviour.
The quantile analysis allows suggesting the definition of policies according to the asymmetric behavior of GEG.
The study employs factors related to technology, financial freedom, and government integrity on GEG, which lead to increased GEG.
To actualise the stated objective and bring the potential contributions to reality, eight hypotheses are tested and verified thus;
H1. Capital formation is positively related to green economic growth.
H2. The labour force is positively related to green economic growth.
H3. Energy efficiency increases green economic growth.
H4. Renewable energy consumption encourages green economic growth.
H5. Increased technology innovation increases green economic growth.
H6. Financial freedom increases green economic growth.
H7. Industrialization is positively related to green economic growth.
H8. Governance integrity drives green economic growth.
Finally, following the introduction section, the current study is structured thus. Section 2 reviews relevant extant studies. Section 3 entails the methodological pathway adopted in modelling the empirical nexuses among the variables of interest. Section 4 presents and discusses the empirical outcomes. Furthermore, Section 5 concludes and provides insightful polices towards achieving green growth in OECD economies.
2. Literature review
The devastating threat posed by GHG emissions remains one of the drivers of research interest that keeps empirical investigations on determinants of economic growth and sustainable environment ongoing. This is so as the problem of climate change remains pervasive relative to the growing economy despite government efforts and the vast number of studies that have emerged to halt it (Gao et al., Citation2022; Khan et al., Citation2021; Nukusheva et al., Citation2021). Contemporary world economies seek to achieve desirable growth without compromising the stake of future generations (Oke et al., Citation2021). Hence, green growth remains an outstanding option among many other identified practical paths to achieving sustainable development (Alola et al., Citation2021; Ibrahim et al., Citation2022; Islam & Managi, Citation2019). As such, research attention is profusely focusing on identifying the drivers of GEG. Following these lines of arguments, this section reviews studies based on determinants of GEG with a preferential focus on energy efficiency, renewable energy, technology innovation, financial development and inclusion, and institutional quality. While the highlighted drivers are favoured due to the research interest of this study, other relevant drivers are equally considered.
Lin and Zhou (Citation2022) probe the functional impacts of energy efficiency on China’s economic growth quality. The study’s analysis relies on provincial data collected from 2000 to 2017, subject to the entropy weight method. The empirical outcomes show that the impacts of energy efficiency are not significant enough to drive economic growth. However, a U-shaped connection is established between the two indicators. Considering the effects from regional perspectives, the study confirms empirical support for the positive and significant impact of energy efficiency on economic growth in the eastern region. Contra-wise, the effects are insignificant on the Eastern region’s economic growth. Similarly, the U-shaped nexus is evident in the Western and Central regions. Razzaq et al. (Citation2021) explore empirical research on the nexuses among economic growth, energy efficiency, municipal solid waste recycling (MSWR), and carbon emissions in the United States by employing the bootstrapping autoregressive distributed lag modelling on quarterly data for the period 1990–2017. The findings reveal MSWR adversely negatively impacts carbon emissions in the short and long runs. Similarly, energy efficiency positively and negatively impacts economic growth and carbon emissions, respectively. Thus, suggesting that energy efficiency supports the path towards a green economy. Akram et al. (Citation2021) examine the disaggregated effects of energy efficiency on economic growth in BRICS economies from 1990 to 2014 using a quantile regression estimator. The results show that energy efficiency positively and significantly affects economic growth across all estimated quantiles. Similarly, the study provides empirically backed evidence for validating the feedback hypothesis in the energy efficiency-economic growth nexus.
With motivations stemming from European Union’s target on decarbonisation, Dell’Anna (Citation2021) examines the investment prospect in the energy sector on economic growth and carbon emissions reduction in Italy. The study employs the Input-Output analysis to analyze the proposed power system installation plan between 2020 and 2040, focusing on selected renewable energy (RE) components such as photovoltaic, wind, geothermal infrastructures, and hydroelectric. The outcomes reveal that RE exerts both positive and negative effects on economic growth in Italy. Irfan (Citation2021) evaluates the extent to which low-carbon intensive energy drives economic growth in selected twenty-four advanced and thirty-four emerging economies from 1990 to 2017. Feedbacks from the long-run analyses show that energy efficiency enhances significant improvements in economic growth for all the sample economies. However, in terms of energy diversity, positive and significant impacts are observed in developing countries. In terms of causality, unidirectional causal nexus is supported, running from economic growth to energy efficiency in developed economies, while bidirectional causality is reported for developing countries. Zhang et al. (Citation2021) investigate the connectivity between energy efficiency, public spending on research and development, and economic growth in Brazil, Russia, and India by employing the generalized method of moments and data envelopment analysis (DEA) on annual data spanning 2008–2018. The study reveals support for both composition and technique effects on the BRI economies. Besides, the study finds that public spending supports green growth through human capital development and research and development.
Go et al. (Citation2020) estimate the functional relationship between energy efficiency, foreign direct investment, carbon emissions, exports, and economic growth from both homogenous and heterogeneous angles in Malaysia. The empirical study relies on ARDL estimator for the period between 1971 and 2013. The feedback from the analyses reveals that bidirectional causality runs from energy efficiency to economic growth. However, the reported causal effects differ across the various production stages comprising primary, secondary, and tertiary. Similarly, the growth hypothesis is evident in the causality between export and economic growth. By implies, exports granger causes economic growth in Malaysia. The empirical verification of the EKC hypothesis interests Zhang et al. (Citation2020) in a panel of fifteen developing countries by employing ARDL and DEA estimators on annual data straddling 1990-2013 periods. The latter estimator is mainly used to assess energy efficiency, energy intensity, and environment in the selected countries. The empirical results based on estimates produced by the ARDL technique validates the proposition of EKC for the selected economies considering the direct and indirect GDP-CO2 emissions nexuses recorded. The feedback from the DEA reveals the presence of low-level pollutions in the developing economies due to high and low degrees of energy intensity and energy efficiency, respectively. Ohene-Asare et al. (Citation2020) evaluate the nexus between energy efficiency and economic development in forty-six economies in the African continent by employing the three-stage least square estimator. On average, a 56% energy efficiency score is assigned to the continent’s performance for the study period. Besides, a bidirectional causal impact is reported between energy efficiency and economic development.
Another driver of GEG under consideration in the present study is renewable energy. For this reason, empirical studies illustrating the link between renewable energy and GEG are reviewed below.
Sohag et al. (Citation2021) evaluate the effects of renewable energy, technological innovation, and militarisation on green economic in OECD economies. The study finds empirical support for the positive and significant impact of renewable energy and technological innovation on the green economy while militarization negatively affects it. Hao et al. (Citation2021) assess the nexuses among renewable energy, human capital, green growth, and carbon emissions in G7 economies from 1991 through 2017. The empirical evidence, anchored on Cross-Sectionally Augmented Auto-regressive Distributive lag (CS-ARDL), reveals that green growth’s linear and non-linear dimensions significantly reduce carbon emissions. Similarly, the carbon-abating effects of human capital, renewable energy, and environmental tax are established. Probing the pathway to a green economy, Mohsin et al. (Citation2021) examine the impacts of non-renewable and renewable energy and economic growth on greenhouse gas emissions in 25 Asian economies for the period stretching 2000–2016. The findings reveal that energy consumption from both sources promotes economic growth. More so, renewable energy is highly effective in achieving a green economy, moderating carbon emissions. Similarly, Doytch and Narayan (Citation2021) confirm the contribution of renewable energy in promoting economic growth with fewer carbon emissions.
The environmental impacts of economic growth and energy resources (non-renewable energy and renewable energy) are the core of research interest in Rahman and Velayutham (Citation2020). The empirical analysis focuses on five selected countries in the Southern Asian region from 1990 to 2014 by utilizing Fully Modified OLS and Dynamic OLS estimators. The study reveals that both energy sources significantly drive economic growth. Destek and Sinha (Citation2020) verify the applicability of EKC in a model comprising trade openness and renewable and non-renewable energy towards decreasing the ecological footprint of twenty-four selected OECD economies by employing yearly data spanning 1980 to 2014. Feedbacks from the empirical analyses support the existence of U-shaped EKC in the sample economies. Further, renewable energy promotes a green environment through its mitigating effects on ecological footprint. Fan and Hao (Citation2020) estimate the functional connection between renewable energy, FDI, and economic growth in thirty-one provinces in China based on annual data covering 2000–2015. The results establish affirm significantly positive impacts of renewable energy and FDI on economic growth. Khan et al. (Citation2020) estimate the possible effects of renewable energy, public health expenditure, and logistics performance on environmental sustainability in ASEAN economies. Empirical outcomes from the study provide practical support to advance the enhancing role of renewable energy on ecological sustainability as a pathway towards attaining green growth. Khan et al. (Citation2020) evaluate the drivers of green growth via environmental sustainability assessment effects of renewable energy, natural resource use, and regulatory pressure from 2005 to 2017 in the South Asian Association for Regional Cooperation (SAARC). The empirical outcomes reveal that renewable energy, among others, promotes environmental sustainability. The role of renewable energy in fostering green growth finds practical support in Ulucak’s (Citation2020) study or the BRICS economies.
Some studies focus on the technological impacts of green growth, advancing how technological advancement enhances carbonless growth. For instance, Fernandes et al. (Citation2021) probe the significant effects of sustainable technology and innovations on green growth in thirty-two OECD countries based on yearly data from 1990 to 2013. Empirical fallouts from the study show that both technologies promote green growth. Nosheen et al. (Citation2021) analyze the effects of technological innovations on green growth in the European economies and selected regions (Eastern and Western) spanning 2000–2017. The empirical evidence relies on the STIRPAT and IPAT model with estimation technique based on fully modified ordinary least square (FMOLS) approach. The estimated model supports green growth-induced technology impacts. Similarly, the results from the regional analysis on Eastern and Western Europe are robust for the primary empirical outcomes. Likewise, Sohag et al. (Citation2021) provide sturdy evidence to justify the pro-green growth impacts of innovation in a panel of selected economies from OECD. Cao et al. (Citation2021) analyze the effects of technology and financial development on green growth in thirty Chinese provinces based on annual data covering 2011 to 2018. Considering the data structure, the study employs a dynamic system GMM estimator to estimate the empirical models. The findings reveal that financial development exacerbates green growth volatility while the intervention of technology reduces it.
The effect of information computer technology (ICT) has equally gained extensive recognition in green growth empirics in recent times. Among the recent strides in this regard, Sharma et al. (Citation2021) examine the relationship between ICT, energy use, and economic growth in ten selected emergent economies of the Asian region. The analysis covers a span of seventeen years running from 2000 to 2017. The proxies of ICT involving the internet and mobile cellular subscriptions significantly promote economic growth while energy consumption impedes. Ulucak (Citation2020) investigates the role of environmental technologies in attaining green growth within the framework of energy consumption (renewable and non-renewable energy) in BRICS economies. Findings from the analyses reveal empirical support for green growth-induced effects of environmental-based technologies. Concerning energy consumption, evidence affirms the driving and dragging effects of renewable and non-renewable energy, respectively. The growth effects of technological innovation constitute the core of research interest in Meirun et al. (Citation2021) in Singapore b employing bootstrap-ARDL using annual time data series covering 1990–2018. Empirical results from the study show that green technology innovation drives economic progress in the short-run and long-run.
The emerging research studies on the concept of green growth have witnessed the emergence of empirical evidence from financial development and policy implementations on environmental regulations, regulatory pressures, and other outreach of government tools. To this end, further studies related to growth financing, financial deepening, and regulatory pressures are explored. Clear evidence of the role of financial development in promoting green growth Hen et al. (2021b) examines the nexuses among financial development, natural resource rent, energy consumption, green investment, and carbon emissions in thirty Chinese provinces from 1995 to 2017. The study employs a second-generation estimation technique based on CS-ARDL to ascertain the long-run and short-run effects of the empirical model. The feedback from the study reveals that financial development constitutes one of the militating factors of the green economy owing to its enhancing effects on carbon emissions. The role of financial development in the promotion of green economy was evaluated by Peng et al. (Citation2021) for a panel of thirty-seven provinces in China. Findings from the study have it that financial development significantly and positively promote green growth. The empirical fallouts in Hsu et al. (Citation2021) support the green growth inducing impacts of financial development.
From the perspective of policy effects on green economy, Qian et al. (Citation2021) employ yearly data from 2004 to 2017 to assess the impacts of smart city policy on GEG. The study affirms that smart cities significantly drive green growth, which is achieved by promoting economic growth, reducing waste emissions, and decreasing GDP-induced energy consumption. By and large, smart city policy is empirically supported as a driving factor of green growth. Similarly, Qiu et al. (Citation2021) examine the role of environmental regulations and FDI on green total factor productivity in a panel of thirty Chinese provinces. The empirical findings reveal that environmental regulations promote a green economy and equally moderate the hindering effects of FDI on the green economy. Ahmed et al. (Citation2022) explore the impacts of financial deepening and institutional quality in realizing green growth objectives in a panel of selected South Asian countries from 2000 to 2008. The empirical results based on both FMOL and DOLS estimators reveal a long term contributive roles of both regressors on green growth. Abid et al. (Citation2021) estimate the level at the government indicators (ISO 14001) promote or deter green growth in Pakistan by employing a time series data spanning 2000-2017. The study finds ISO 14001 to be an effective tool that drives green growth. Specifically, the rule of law emerges as the most efficient indicator that improves green growth in Pakistan. Tawiah et al. (Citation2021) assess the driving factors of green growth from a range of one-hundred and twenty-three developed and developing economies. Significant findings provide empirical support for the driving impacts of renewable energy and economic development on green growth. In contrast, trade openness and non-renewable energy consumption deter green growth
The appraisal of the existing studies on green growth reveals some remarkable research gaps that this study seeks to fill. First, the prior studies on the drivers of green growth are just evolving. Reference can be made to research focusing on Asian regions, G7 economies, BRICS, Chinese provinces, and Africa. Little is known about the factors driving green growth in the OECD economies, notwithstanding the group’s substantial contributions towards promoting green growth. The few available studies focused on OECD countries are limited by narrow consideration of drivers or focus more on the environment. For instance, Sohag et al. (Citation2021) only focused on innovation, renewable energy, and militarization, while Fernandes et al. (Citation2021) considered only technology and innovation. Other factors were estimated on the environment and not on green growth, as evident in Destek and Sinha (Citation2020). Consequently, this study will constitute the first all-inclusive analysis considering a wide range of green growth drivers covering energy efficiency, renewable energy, technology innovation, financial freedom, and government integrity for the OECD countries. Besides, considering financial freedom and government integrity is a novel contribution to extending knowledge frontier is the first globally and for the OECD economies. Other contributions to the extant literature have been exposited in the introductory section.
3. Data and econometric approach
3.1. Data
This research aims to examine the role played by the determinants of the GEG in the OECD countries. The OECD countries analyzed are 37 out of a total of 38 countries. Costa Rica was excluded from the analysis due to the availability of the information. The countries examined are listed in of Appendix A. The analysis period corresponds from the year 1990 to the year 2020; the frequency of the data of the variables is annual. Therefore, databases obtained from secondary information sources from World Bank databases (WDI, Citation2020) and BP Statistical Review of World Energy (BP, Citation2020), Heritage Foundation (Citation2021), and OECD (2020) are used. Once the information was collected from the different databases, it was refined, and a data panel was compiled to comply with the econometric processing.
The dependent variable for this study is GEG, and the explanatory variables are namely: capital formation (CAF), the labour force (LAF), energy efficiency (EEF), renewable energy (REN), technological innovation (TEC), financial freedom (FFR), industrialization (IND) and Integrity of Government (GIN). The original variables were converted to natural logarithms to eliminate the heterogeneity of the measurement unit and homogenize the econometric analysis. The description of the empirical variables are provided in .
Table 1. Description of variables.
A detailed summary of the variables of interest to the current study is presented in . Descriptive statistics are mean, standard deviation (St. Dev.), Minimum value, maximum value, kurtosis, skewness, Jarque – Bera, and correlation. Of which, the skewness and kurtosis statistics preliminarily indicate the distribution of each variable. While the Jarque – Bera statistic allows confirming that the variables do not follow a normal distribution. Therefore, this generates an advantage when using quantile regression in econometric analysis. In addition, the last row of indicates the correlation coefficient between the explanatory variables and the dependent variable, which allows a preliminary check of the association between the study variables.
Table 2. Descriptive statistics.
On the other hand, graphically displays the correlation between the study variables, which preliminarily indicates their association.
3.2. Methodology
After the specification and description of the variables of interest, the next step is to describe the steps of the econometric strategy to be used in this study. EquationEquation (1)(1)
(1) describes the econometric model to estimate, which is written as:
(1)
(1)
where GEG represents GEG, CAF represents capital formation, LAF represents the workforce, REN denotes renewable energy, TEC represents technological innovation, FFR represents financial freedom, IND indicates industrialization, and GIN is the Integrity of Government. ε, represent the error term of the econometric equation. The subscripts
and
describe the examined countries
in the analysis period
To satisfactorily develop the estimation of the long-term elasticities of the model, it is necessary to ensure the properties of the variables used in the study (Santos Pereira & Cardoso Marques, Citation2020). Therefore, preliminary tests must be developed to guarantee its quality. The tests to be developed are (i) assessment of presence of multicollinearity between the variables, using the variance inflation factor (VIF) (Belsley et al., Citation1980); (ii) Examine the cross-sectional dependence (CD) in the data panel (Breusch & Pagan, Citation1980), for which the Pesaran (Citation2015) CD test is used. (iii) Evaluate the unit-roots of the model variables (Zafar et al., 2019). Therefore, the Cross-sectional Im-Pesaran-Shin (CIPS) second test developed by (Pesaran, Citation2007) and Herwartz and Siedenburg (Citation2008) is applied. Both control for DC issues between the model variables. (iv) The long-term causal link between the study variables should be evaluated. Thus, the second-generation Westerlund (Citation2007) test controls CD issues (Agresti, Citation1990).
After ensuring the quality of the model variables, the long-term elasticities will be examined. In view of this, most of the existing estimators provide estimates for the mean effects of independent variables on the outcome variables limiting the empirical evidence. Focus on the average of the dependent variable, which becomes a limitation for economic analysis (Kao & Chiang, Citation2001). Consequently, the panel quantile regression (PQR), introduced by Koenker and Bassett, offers several advantages compared to linear econometric methods (Zhu et al., Citation2016). One of the main advantages is that PQR examines the effects of the explanatory variables on various points of the conditional distribution of the dependent variable (Chen & Lei, Citation2018). Despite the multiple advantages that PQR provides, it fails to address the potential impacts of fixed effects of individuals in the panel.
Further, this anomaly can be resolved with the MMQR approach created and developed by Machado and Silva (Citation2019). MMQR has the particularity of considering the effect of the conditional heterogeneous variance of the factors that lead to the explained variable (Alhassan et al., Citation2020). That is, this approach allows capturing the individual effects of the panel members; it allows estimating models with endogenous variables (Koengkan & Fuinhas, Citation2021). Additionally, PQR allows calculating the coefficients of the regressor variables that affect the entire conditional distribution of the dependent variable (Machado & Silva, Citation2019). Consequently, MMQR is a method that is fully adapted to this study. Further, the MMQR model can be defined according to the following function:
(2)
(2)
where, with a probability,
considering the individuals in panel
on the time periods examined. The individual
fixed effects is determined by the parameter
Z denotes a k-vector of known modules of
with elements
that is determined by:
(2)
(2)
and
are similarly distributed across individuals
and time-period
is statistically independent of
to satisfy the moment condition of Machado and Silva (Citation2019).
Subsequently, in order to compare the MMQR results, linear complementary estimates are made, called fully modified ordinary least squares (FMOLS), the dynamic ordinary least squares (DOLS), and the fixed effects ordinary least squares OLS (FE-OLS). Finally, Dumitrescu and Hurlin Granger non-causality in the heterogeneous panel is applied to examine the causal relationship between the model variables, which is usable with the presence of CD.
4. Empirical results and discussion
In order to achieve the objective of the investigation, the possible presence of multicollinearity problems in the data panel must first be verified through the VIF test. The results in indicate the statistic VIFs below the generally accepted benchmark of ten.
Table 3. VIF Statistic.
Second, after verifying the absence of multicollinearity problems, the presence of CD should be tested in the data panel. The CD test verifies the null hypothesis of independence of the data, so the p-values of the test allow accepting the null hypothesis of CD among the study variables. Third, after examining the presence of DC in the data panel, unit root tests are used to examine the stationarity of the model series. The second generation tests used are CIPS (Pesaran, Citation2007) and Herwartz and Siedenburg (Citation2008), which control DC issues in the data panel. The outcomes of the Pesaran (Citation2015) CD test are presented in . Consequently, the variables become stationary when obtaining their first difference; their order of integration is I (1).
Table 4. CD test and first-generation unit root test.
Afterward, controlling for CD-related issues in the model series, examine the long-term causal link between the analysis variables. Consequently, provides the outcomes of Westerlund (Citation2007) test. The results suggest the null hypothesis of longrun nexus is accepted. In other words, a long-term relationship between GEG and CAF, LAF, EEF, REN, TEC, FFR, IND and GIN is evidenced in the OECD countries during the period examined.
Table 5. Westerlund panel cointegration.
Subsequently, the presence of long-run association has been validated, and the elasticities of the explanatory variables on the dependent variable MMQR are examined. The usefulness of the MMQR approach can be observed due to the variety of results across all quantiles of GEG. Thus, presents the regression analysis results by MMQR in the various quantiles, named (1st–9th). Furthermore, it is visualized that the coefficients are heterogeneous across the quantiles and differ in statistical significance. Consequently, it is observed that CAF maintains a positive and significant association with GEG across 1st–4th quantiles. A 1% increase in CAF represents an increase in GEG between 0.627% and 0.032%, which indicates that CAF contributes to GEG. This finding is related to the fundamental concepts of economic growth developed by Solow (Citation1956) and Swan (Citation1956). These authors indicate that economic growth can be made more dynamic by accumulating intermediate goods (CAF), generating added value, which contributes to economic expansion. However, it is also evidenced that CAF contributes to the GEG, which could be associated with the fact that CAF allows the development of more efficient processes. These results contribute to Zubair et al. (Citation2020), who affirm that CAF contributes to sustainable production.
Table 6. Results of MMQR.
Then, it is seen that LAF is positively related to GEG in the upper quantiles (7th–9th). A 1% increase in LAF is associated with an increase in GEG between 0.202% and 0.234%. Similarly, LAF is consistent with views held by Solow (Citation1956) and Swan (Citation1956). The intuition behind this finding is that the intensification in the use of labour contributes to higher production levels, contributing to a more efficient production process. At this production level, the labour to be required may be of higher qualification as opposed to the initial phase of production, in which no qualified labour is required. This synergy contributes to generating more sustainable processes due to the capabilities of the workers, which makes the production process more efficient, contributing to the GEG. The findings coincide with the study by Wang and Su (Citation2020), who argue that the most qualified LAF contributes to green factor productivity.
On the other hand, EEF positively impacts GEG across all quantiles of the conditional distribution of GEG. The magnitude of EEF elasticities from the lowest to the highest quantiles. The variation of 1% of EEF is associated with the variation of GEG between 0.861% and 1.037%, as corresponds to each quantile. The argument associated with this finding is that, through EEF, the production performance increases concerning the use of the energy factor, which leads to factor productivity based on fewer carbon emissions. In other words, the production process requires less energy to moderate the negative externalities on the environment since the production process will generate fewer carbon emissions. These empirical findings strongly support the study by Lin and Zhou (Citation2022), who affirm that FES can contribute significantly to promoting GEG and sustainable development (Abbasi et al., Citation2021; Wu et al., Citation2019).
Likewise, REN exerts a positively significant impact on GEG across all quantiles, except in the 9th quantile. A 1% increase in REN is associated with GEG increase between 0.015% and 0.034%. It shows that the magnitude of REN decreases when the quantiles are higher. The behaviour of REN allows it to infer that higher production levels demand more energy; however, most of the energy demand comes from fossil fuels. However, REN is a catalyst for GEG because RENs do not emit carbon dioxide and most often exhibit a decreasing level of carbon emissions to productivity. Conclusively, REN has a significant impact on reducing carbon emissions. These findings follow what was found by Sohag et al. (Citation2021) and Hao et al. (Citation2021) in OECD countries and G7 economies, who find that green productivity can be achieved through REN.
Otherwise, TEC positively predicts GEG across all quantiles, showing that the magnitude of TEC elasticities is ascending between quantiles. This fact shows that the variation of 1% of TEC represents a variation of TEC between 0.033% and 0.060%. TEC stimulates the economic process efficiently, which allows economic growth to be sustained due to its efficiency in the production process since it allows increasing factor productivity (Alam & Ullah, Citation2021). The role of TEC is not new since TEC is a promoter of the EEF and REN, which contributes to profitable growth with less use of productive and energy resources. TEC represents the new emerging technologies, which directly contribute to production efficiency because it promotes productivity based on less energy use. In this way, production processes become sustained and more profitable (Upadhyay et al., Citation2021). These findings corroborate the submissions in Nosheen et al. (Citation2021) in European countries, which evidences the positive effects on production and the environment through TEC promotion.
Further, FFR shows a positive and significant association with GEG in the upper quantiles of the distribution. The 1% increase in FFR represents an increase in GEG between 0.008% and 0.019%, descending across the upper quantiles. This behaviour elucidates the importance of the FFR in the achievement of green development since to generate greater GEG in the upper levels of economic growth; greater efforts are required to achieve this objective. With this, FFR contributes to the economy with more financing options in projects or activities of sustainable productivity. This aspect allows for greater financing options, especially for GEG-related activities. The results align with Hen et al. (2021b), who find that FFR is a driver for achieving sustainable production framed in the GEG.
Next, IND presents statistically significant and positive associativity with GEG throughout the quantile distribution. A 1% increase in IND means an increase in GEG between 0.09% and 0.169%. The intuition behind this finding is that IND is a value-adding process, which employs efficient production processes to maximize economic benefit. Therefore, it uses efficient production processes based on factor productivity, which improves efficiency and lower production costs. The IND modernizes production processes and aims to improve productivity, which leads to the impulse of GEG (Yang, Citation2017). These findings support the claim of Zhang et al. (Citation2021), who attributes that the GEG can be promoted through the IND, TEC and green finances in the Belt and Road Initiative (BRI) member countries.
In addition, GIN shows a positive and significant association with GEG at the initial quantiles of the examined distribution (1st–3rd). The variation of 1% of GIN contemplates GEG variation between 0.071% and 0.274%, as appropriate. GIN is associated with the State’s capacity to influence the economy’s decisions, that is, its hegemony to govern. Therefore, when regulatory laws are more efficient, the State constitution can design measures aimed at sustainable development. In other words, GI can lead to the design and implementation of government policies to mitigate environmental degradation and promote environmental awareness on the use of productive resources, contributing to GEG. In other words, the GIN improves the governance scenario and contributes to adopting sustainability-oriented scenarios (Du et al., Citation2018). However, the results of the GIN study have an impact on the first quantiles, which may elucidate that to achieve the GEG at higher levels of production, more efficient policies with greater coercion are required.
Consequently, to the results achieved, the scale and location effect coefficients validate the use of the MMQR approach. Further, these two elements significantly support the coefficients found for the explanatory variables across the quantiles examined, 1st - 9th. First, the location effect suggests statistical significance concerning the distribution of the GEG observations across the quantiles. Second, the scale effect is significant for the heterogeneity and magnitude of the coefficients of the explanatory variables. Therefore, the location and scale effect validate the magnitude of the coefficients in different quantiles, allowing an efficient examination of the behavior of the GEG conditional distributor (Machado & Silva, Citation2019).
In order to compare the quantile regressions using MMQR, the results are compared with linear approaches that focus on the average of the explanatory variable. Therefore, presents the estimates made by FMOLS, DOLS, and FE-OLS. The results support the sign and significance of the coefficients similar to those presented in . CAF, LAF, EEF, REN, TEC, FFR, IND and GIN maintain a positive and significant relationship with GEG, showing the importance of the factors determining the GEG. Next, presents the coefficients of the MMQR, FMOLS, DOLS and FE-OLS regressions, in which the heterogeneity of these can be appreciated and the importance of examining the behavior of GEG using linear and non-linear econometric methods.
Figure 2. Graphic representation of the MMQR, FMOLS, DOLS and FE-OLS coefficients.
Source: Authors own estimations.
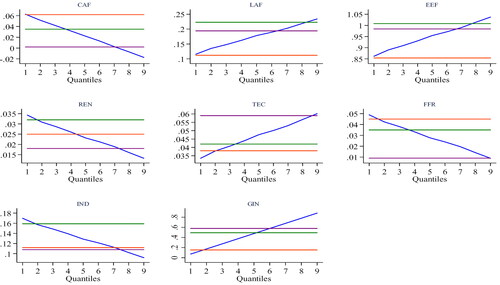
Consequently, as discussed above, it is essential to mention that the scope of sustainable development of the planet can be achieved by taking appropriate and efficient measures. On the contrary, if the policy definitions used to take the wrong path, it would become a waste of time, due to the urgency to counteract environmental degradation (Hao et al., Citation2021). Therefore, the impulse of the GEG is an alternative to generate a sustainable production with low-carbon emission content, which are actions directed under the SGD12.
However, GEG is an exogenous variable determined by the factors identified in this study, which are possible public policy instruments to achieve the objective of interest, in this case, to energize the GEG. Consequently, the causal relationship between the GEG and the explanatory variables of the model is carried out using the test of Dumitrescu-Hurlin. describes the results of the causality test, which indicate the presence of a bidirectional causality relationship between the study variables at 1% significance.
Table 7. The results of Dumitrescu–Hurlin panel causality tests.
This fact allows us to infer that CAF, LAF, EEF, REN, TEC, FFR, IND and GIN are energy policy instruments to be considered for the achievement of GEG. Consequently, any policy measure in these instruments will have an impact on GEG. Similarly, any policy measure in GEG will generate an impact on the explanatory variables of this model. These findings become valuable inputs for the correct definition of policy measures to set the path of sustainable development in the study countries.
5. Conclusions and policy implications
The degradation of the environment has become a particular reason to rethink how economic activities are being developed. These new forms seek to improve productivity through the efficient use of factors and generate less impact on the environment. One of these forms is the GEG, which contributes to clean production aimed at the planet’s sustainable development. Therefore, this study examines the long-term causal link between GEG and CAF, LAF, EEF, REN, TEC, FFR, IND and GIN in 37 OECD countries from 1990 to 2020. Second-generation econometric techniques are used to test the long-run equilibrium relationship. Subsequently, the non-linear approach MMQR is applied to examine the coefficients of the explanatory variables at different points of the GEG variable.
The results show a long-term equilibrium relationship between the variables of the model. Likewise, the coefficients of the explanatory variables show a series of heterogeneous results according to the quantile examined. It is revealing that the explanatory variables are positively and significantly associated with GEG. However, the coefficients are heterogeneous according to the quantile examined. It fact denotes the importance of this type of approach that focuses on various points of the conditional distribution of the GEG. Likewise, it was verified that GEG follows a different behaviour than the approaches that focus on the average of the GEG distribution—evidencing the MMQR approach’s congruence according to the study’s GEG scope.
Finally, the factors that drive the GEG in the OECD countries were tested to evaluate the causal relationship of the model, which evidenced a bidirectional causal relationship between the explanatory variables and the GEG variable. This action makes it possible to define instruments that are useful in defining energy policies to promote sustainable development through the dynamism of GEG. Therefore, the following study derives profound policy implications aimed at ensuring compliance with SDG12 by government authorities. First, the EEF is a clear determinant of the momentum of the GEG. However, most of the energy in OECD countries comes from nonrenewable energy sources, which limits its potential effect. Therefore, government mechanisms must be created that oblige industries to use energy with low carbon content. Likewise, it is evidenced that REN contributes significantly to GEG due to its null effect on carbon emissions. Therefore, the imposition of REN in industries should be promoted through fiscal or tax incentives to those companies that obtain their production through clean energy.
Second, the momentum of TEC in the industrial sector is a crucial element when the rise of the GEG is on the horizon since the TEC drives the effect of EEF and REN on GEG. Therefore, its implementation in production activities should be promoted, which can be made effective with an incentive program by the Government in which advice and public financing are provided to create productive projects based on modern technology. However, this action does not limit the private financial sector’s participation, which should help offer green financing programs using modern technology. Both financing edges provide greater openness and inclusion for projects oriented towards sustainable development. Third, Government actions to achieve a GEG generate a better effect when the Government entirely operates its laws. Therefore, constitutional laws and regulations are required to generate sustainable production in the economic sector, which should consider promoting clean production processes to achieve sustainable economic development. Finally, one of the study’s main limitations is the lack of information to know the green productivity factor according to each type of fuel. This procedure could help to target policy measures according to each fuel. Furthermore, this limitation would be considered an extension for future research.
Acknowledgments
This research is supported by the National Natural Science Foundation of China (72250410375). Furthermore, the authors would like to thank Prince Sultan University for paying the APC and the support through TAS research lab and Open Research Fund of NJIT Institute of Industrial Economy and Innovation Management.
Disclosure statement
No potential conflict of interest was reported by the authors.
Additional information
Funding
References
- Abbasi, K. R., Hussain, K., Redulescu, M., & Ozturk, I. (2021). Does natural resources depletion and economic growth achieve the carbon neutrality target of the UK? A way forward towards sustainable development. Resources Policy, 74, 102341. https://doi.org/10.1016/j.resourpol.2021.102341
- Abid, N., Ikram, M., Wu, J., & Ferasso, M. (2021). Towards environmental sustainability: exploring the nexus among ISO 14001, governance indicators and green economy in Pakistan. Sustainable Production and Consumption, 27, 653–666. https://doi.org/10.1016/j.spc.2021.01.024
- Agresti, A. (1990). Categorical data analysis. John Wiley and Sons. ISBN 0-471-36093-7
- Ahmed, F., Kousar, S., Pervaiz, A., & Shabbir, A. (2022). Do institutional quality and financial development affect sustainable economic growth? Evidence from South Asian countries. Borsa Istanbul Review, 22(1), 189–196. https://doi.org/10.1016/j.bir.2021.03.005
- Akinci, M., Akinci, G. Y., & Yilmaz, Ö. (2015). The relationship between central Bank independence, financial freedom, and economic growth: a panel ARDL bounds testing approach. Central Bank Review, 15(3), 1.
- Akram, R., Chen, F., Khalid, F., Huang, G., & Irfan, M. (2021). Heterogeneous effects of energy efficiency and renewable energy on economic growth of BRICS countries: a fixed effect panel quantile regression analysis. Energy, 215, 119019. https://doi.org/10.1016/j.energy.2020.119019
- Akram, R., Umar, M., Xiaoli, G., & Chen, F. (2022). Dynamic linkages between energy efficiency, renewable energy along with economic growth and carbon emission. A case of MINT countries an asymmetric analysis. Energy Reports, 8, 2119–2130. https://doi.org/10.1016/j.egyr.2022.01.153
- Alam, B., & Ullah, M. N. (2021). Do human resources run economic growth? Empirical evidence from Pakistan. Review of Economics and Development Studies, 7(2), 205–213. https://doi.org/10.47067/reads.v7i2.351
- Alhassan, A., Usman, O., Ike, G. N., & Sarkodie, S. A. (2020). Impact assessment of trade on environmental performance: accounting for the role of government integrity and economic development in 79 countries. Heliyon, 6(9), e05046. https://doi.org/10.1016/j.heliyon.2020.e05046
- Allan, B. B., & Meckling, J. O. (2021). Creative learning and policy ideas: The global rise of green growth. Perspectives on Politics, 1–19. https://doi.org/10.1017/S1537592721000037
- Alola, A. A., Ozturk, I., & Bekun, F. V. (2021). Is clean energy prosperity and technological innovation rapidly mitigating sustainable energy-development deficit in selected sub-Saharan Africa? A myth or reality. Energy Policy, 158, 112520. https://doi.org/10.1016/j.enpol.2021.112520
- Awan, U. (2021). Steering for sustainable development goals: a typology of sustainable innovation. Industry, Innovation and Infrastructure, 1026–1036.
- Bao, Z., Ferraz, D., & Rebelatto, D. (2022). Energy efficiency and China’s sustainable carbon neutrality target: evidence from novel research methods quantile on quantile regression approach. Economic Research-Ekonomska Istraživanja, 35(1), 6985–7007. https://doi.org/10.1080/1331677X.2022.2054456
- Baş, H. (2021). Green growth and green jobs in Turkey: An opportunity for youth employment in competitive markets. In Financial strategies in competitive markets (pp. 95–108). Springer.
- Belsley, D. A., Kuh, E., & Welsch, R. E. (1980). Regression diagnostics: Identifying influential dataand source of collinearity. Wiley. https://doi.org/10.1002/0471725153
- BP. (2020). BP statistical review of world energy. https://www.bp.com/.
- Breusch, T. S., & Pagan, A. R. (1980). Lagrange multiplier test and to model applications specification in econometrics. Rev. Econ. Stud, 47(1), 239–253.
- Cao, J., Law, S. H., Samad, A. R. B. A., Mohamad, W. N. B. W., Wang, J., & Yang, X. (2021). Impact of financial development and technological innovation on the volatility of green growth—evidence from. China. Environmental Science and Pollution Research, 28, 1–17.
- Chen, M., Sinha, A., Hu, K., & Shah, M. I. (2021). Impact of technological innovation on energy efficiency in industry 4.0 era: Moderation of shadow economy in sustainable development. Technological Forecasting and Social Change, 164, 120521. https://doi.org/10.1016/j.techfore.2020.120521
- Chen, Y., Shao, S., Fan, M., Tian, Z., & Yang, L. (2022). One man’s loss is another’s gain: Does clean energy development reduce CO2 emissions in China? Evidence based on the spatial Durbin model. Energy Economics, 107, 105852. https://doi.org/10.1016/j.eneco.2022.105852
- Chen, W., & Lei, Y. (2018). The impacts of renewable energy and technological innovation on environment-energy-growth nexus: New evidence from a panel quantile regression. Renewable Energy, 123, 1–14. https://doi.org/10.1016/j.renene.2018.02.026
- Cotton, M., Kirshner, J., & Salite, D. (2021). The politics of electricity access and environmental security in Mozambique. Energy and Environmental Security in Developing Countries, 279–302.
- Croutzet, A., & Dabbous, A. (2021). Do FinTech trigger renewable energy use? Evidence from OECD countries. Renewable Energy. 179, 1608–1617. https://doi.org/10.1016/j.renene.2021.07.144
- Dell’Anna, F. (2021). Green jobs and energy efficiency as strategies for economic growth and the reduction of environmental impacts. Energy Policy, 149, 112031. https://doi.org/10.1016/j.enpol.2020.112031
- Destek, M. A., & Sinha, A. (2020). Renewable, nonrenewable energy consumption, economic growth, trade openness and ecological footprint: Evidence from organisation for economic Co-operation and development countries. Journal of Cleaner Production, 242, 118537. https://doi.org/10.1016/j.jclepro.2019.118537
- Dordmond, G., de Oliveira, H. C., Silva, I. R., & Swart, J. (2021). The complexity of green job creation: An analysis of green job development in Brazil. Environment, Development and Sustainability, 23(1), 723–746. https://doi.org/10.1007/s10668-020-00605-4
- Doytch, N., & Narayan, S. (2021). Does transitioning towards renewable energy accelerate economic growth? An analysis of sectoral growth for a dynamic panel of countries. Energy, 235, 121290. https://doi.org/10.1016/j.energy.2021.121290
- Du, J., Li, W., Lin, B., & Wang, Y. (2018). Government integrity and corporate investment efficiency. China Journal of Accounting Research, 11(3), 213–232. https://doi.org/10.1016/j.cjar.2017.03.002
- Egbetokun, S., Osabuohien, E., Akinbobola, T., Onanuga, O. T., Gershon, O., & Okafor, V. (2020). Environmental pollution, economic growth and institutional quality: Exploring the nexus in Nigeria. Management of Environmental Quality: An International Journal, 31(1), 18–31. https://doi.org/10.1108/MEQ-02-2019-0050
- Fan, W., & Hao, Y. (2020). An empirical research on the relationship amongst renewable energy consumption, economic growth and foreign direct investment in China. Renewable Energy. 146, 598–609. https://doi.org/10.1016/j.renene.2019.06.170
- Fernandes, C. I., Veiga, P. M., Ferreira, J. J., & Hughes, M. (2021). Green growth versus economic growth: Do sustainable technology transfer and innovations lead to an imperfect choice? Business Strategy and the Environment, 30(4), 2021–2037. https://doi.org/10.1002/bse.2730
- Gao, B., Ozturk, I., & Ullah, S. (2022). A new framework to the green economy: asymmetric role of public-private partnership investment on environment in selected Asian economies. Economic Research-Ekonomska Istraživanja, 31(6), 1–12. https://doi.org/10.1080/1331677X.2022.2094441
- Go, Y. H., Lau, L. S., Yii, K. J., & Lau, W. Y. (2020). Does energy efficiency affect economic growth? Evidence from aggregate and disaggregate levels. Energy & Environment, 31(6), 983–1006. https://doi.org/10.1177/0958305X19882395
- Hao, L. N., Umar, M., Khan, Z., & Ali, W. (2021). Green growth and low carbon emission in G7 countries: how critical the network of environmental taxes, renewable energy and human capital is? Science of the Total Environment, 752, 141853. https://doi.org/10.1016/j.scitotenv.2020.141853
- He, L., Zhang, L., Zhong, Z., Wang, D., & Wang, F. (2019). Green credit, renewable energy investment and green economy development: Empirical analysis based on 150 listed companies of China. Journal of Cleaner Production, 208, 363–372. https://doi.org/10.1016/j.jclepro.2018.10.119
- Heritage Foundation. (2021). Index of economic freedom. https://www.heritage.org/
- Herman, K. S. (2021). Green growth and innovation in the Global South: a systematic literature review. Innovation and Development, 1–27. https://doi.org/10.1080/2157930X.2021.1909821
- Herwartz, H., & Siedenburg, F. (2008). Homogenous panel unit root tests under cross sectional dependence: Finite sample modifications and the wild bootstrap. Computational Statistics & Data Analysis, 53(1), 137–150. https://doi.org/10.1016/j.csda.2008.07.008
- Hsu, C. C., Quang-Thanh, N., Chien, F., Li, L., & Mohsin, M. (2021). Evaluating green innovation and performance of financial development: mediating concerns of environmental regulation. Environmental Science and Pollution Research, 28, 1–12.
- Huberts, L. W. (2018). Integrity: What it is and Why it is Important. Public Integrity, 20(sup1), S18–S32. https://doi.org/10.1080/10999922.2018.1477404
- Ibrahim, R. L., & Ajide, K. B. (2021). Disaggregated environmental impacts of nonrenewable energy and trade openness in selected G-20 countries: the conditioning role of technological innovation. Environmental Science and Pollution Research, 28, 1–15.
- Ibrahim, R. L., Al-Mulali, U., Ozturk, I., Bello, A. K., & Raimi, L. (2022). On the criticality of renewable energy to sustainable development: Do green financial development, technological innovation, and economic complexity matter for China? Renewable Energy, 199, 262–277. https://doi.org/10.1016/j.renene.2022.08.101
- Irfan, M. (2021). Low-carbon energy strategies and economic growth in developed and developing economies: the case of energy efficiency and energy diversity. Environmental Science and Pollution Research, 28(39), 54608–54620. https://doi.org/10.1007/s11356-021-14070-7
- Islam, M., & Managi, S. (2019). Green growth and pro-environmental behavior: Sustainable resource management using natural capital accounting in India. Resources, Conservation and Recycling, 145, 126–138. https://doi.org/10.1016/j.resconrec.2019.02.027
- Ji, X., Umar, M., Ali, S., Ali, W., Tang, K., & Khan, Z. (2021). Does fiscal decentralization and eco‐innovation promote sustainable environment? A case study of selected fiscally decentralized countries. Sustainable Development, 29(1), 79–88. https://doi.org/10.1002/sd.2132
- Kao, C., & Chiang, M. H. (2001). On the estimation and inference of a cointegrated regression in panel data. In Nonstationary panels, panel cointegration, and dynamic panels. Emerald Group Publishing Limited.
- Khan, S. A. R., Yu, Z., Sharif, A., & Golpîra, H. (2020). Determinants of economic growth and environmental sustainability in South Asian Association for Regional Cooperation: evidence from panel ARDL. Environmental Science and Pollution Research, 27(36), 45675–45687. https://doi.org/10.1007/s11356-020-10410-1
- Khan, S. A. R., Zhang, Y., Kumar, A., Zavadskas, E., & Streimikiene, D. (2020). Measuring the impact of renewable energy, public health expenditure, logistics, and environmental performance on sustainable economic growth. Sustainable Development, 28(4), 833–843. https://doi.org/10.1002/sd.2034
- Khan, S. A. R., Godil, D. I., Quddoos, M. U., Yu, Z., Akhtar, M. H., & Liang, Z. (2021). Investigating the nexus between energy, economic growth, and environmental quality: A road map for the sustainable development. Sustainable Development, 29(5), 835–846. https://doi.org/10.1002/sd.2178
- Khan, S. A. R., Ponce, P., Yu, Z., & Ponce, K. (2022). Investigating economic growth and natural resource dependence: An asymmetric approach in developed and developing economies. Resources Policy, 77, 102672. https://doi.org/10.1016/j.resourpol.2022.102672
- Koengkan, M., & Fuinhas, A. (2021). Does the overweight epidemic cause energy consumption ? A piece of empirical evidence from the European region. Energy, 216, 119297. https://doi.org/10.1016/j.energy.2020.119297
- Lei, W., Xie, Y., Hafeez, M., & Ullah, S. (2022). Assessing the dynamic linkage between energy efficiency, renewable energy consumption, and CO2 emissions in China. Environmental Science and Pollution Research International, 29(13), 19540–19552. https://doi.org/10.1007/s11356-021-17145-7
- Lin, B., & Zhou, Y. (2022). Does energy efficiency make sense in China? Based on the perspective of economic growth quality. The Science of the Total Environment, 804, 149895.
- Liu, H., Yao, P., Latif, S., Aslam, S., & Iqbal, N. (2021). Impact of green financing, FinTech, and financial inclusion on energy efficiency. Environmental Science and Pollution Research, 29, 1–12.
- Liu, L., Bouman, T., Perlaviciute, G., & Steg, L. (2020). Effects of competence-and integrity-based trust on public acceptability of renewable energy projects in China and the Netherlands. Journal of Environmental Psychology, 67, 101390. https://doi.org/10.1016/j.jenvp.2020.101390
- Luo, B., Khan, A. A., Safi, A., & Yu, J. (2022). Research methods in economics to evaluate the role of energy efficiency and financial inclusion in achieving China’s carbon neutrality target. Economic Research-Ekonomska Istraživanja, 1–29. https://doi.org/10.1080/1331677X.2022.2093245
- Machado, J. A., & Silva, J. S. (2019). Quantiles via moments. Journal of Econometrics, 213(1), 145–173. https://doi.org/10.1016/j.jeconom.2019.04.009
- Mahapatra, B., & Irfan, M. (2021). Asymmetric impacts of energy efficiency on carbon emissions: A comparative analysis between developed and developing economies. Energy, 227, 120485. https://doi.org/10.1016/j.energy.2021.120485
- Maksoud, M. I. A., Fahim, R. A., Shalan, A. E., Abd Elkodous, M., Olojede, S. O., Osman, A. I., Farrell, C., Al-Muhtaseb, A. H., Awed, A. S., Ashour, A. H., & Rooney, D. W. (2021). Advanced materials and technologies for supercapacitors used in energy conversion and storage: a review. Environmental Chemistry Letters, 19(1), 375–439. https://doi.org/10.1007/s10311-020-01075-w
- Martinez, C. I. P., & Poveda, A. C. (2021). The importance of science, technology and innovation in the green growth and sustainable development goals of Colombia. Environmental and Climate Technologies, 25(1), 29.
- Meirun, T., Mihardjo, L. W., Haseeb, M., Khan, S., A., R., & Jermsittiparsert, K. (2021). The dynamics effect of green technology innovation on economic growth and CO2 emission in Singapore: New evidence from bootstrap ARDL approach. Environmental Science and Pollution Research, 28(4), 4184–4194. https://doi.org/10.1007/s11356-020-10760-w
- Mohsin, M., Kamran, H. W., Nawaz, M. A., Hussain, M. S., & Dahri, A. S. (2021). Assessing the impact of transition from nonrenewable to renewable energy consumption on economic growth-environmental nexus from developing Asian economies. Journal of Environmental Management, 284, 111999.
- Musa, M. S., Jelilov, G., Iorember, P. T., & Usman, O. (2021). Effects of tourism, financial development, and renewable energy on environmental performance in EU-28: does institutional quality matter? Environmental Science and Pollution Research, 29, 1–12.
- Naqvi, S., Wang, J., & Ali, R. (2021). Towards a green economy in Europe: Does renewable energy production has asymmetric effects on unemployment? Environmental Science and Pollution Research, 29, 1–8.
- Nathaniel, S. P., Murshed, M., & Bassim, M. (2021). The nexus between economic growth, energy use, international trade and ecological footprints: the role of environmental regulations in N11 countries. Energy, Ecology and Environment, 6(6), 496–512. https://doi.org/10.1007/s40974-020-00205-y
- Nosheen, M., Iqbal, J., & Abbasi, M. A. (2021). Do technological innovations promote green growth in the European Union? Environmental Science and Pollution Research International, 28(17), 21717–21729.
- Nukusheva, A., Ilyassova, G., Rustembekova, D., Zhamiyeva, R., & Arenova, L. (2021). Global warming problem faced by the international community: International legal aspect. International Environmental Agreements: Politics, Law and Economics, 21(2), 219–233. https://doi.org/10.1007/s10784-020-09500-9
- OECD. (2020). Organization of economic co-operation and development. https://data.oecd.org/
- Ohene-Asare, K., Tetteh, E. N., & Asuah, E. L. (2020). Total factor energy efficiency and economic development in. Energy Efficiency, 13(6), 1177–1194. https://doi.org/10.1007/s12053-020-09877-1
- Oke, D. M., Ibrahim, R. L., & Bokana, K. G. (2021). Can renewable energy deliver African quests for sustainable development? The Journal of Developing Areas, 55(1), 1–16. https://doi.org/10.1353/jda.2021.0022
- Palit, S., & Hussain, C. M. (2021). Minerals and metal industry in the global scenario and environmental sustainability. In Sustainable resource management (pp. 163–177). Elsevier.
- Peng, B., Zhang, X., Elahi, E., & Wan, A. (2021). Evolution of spatial–temporal characteristics and financial development as an influencing factor of green ecology. Environment, Development and Sustainability, 24, 1–21.
- Pesaran, M. H. (2007). A simple panel unit root test in the presence of cross‐section dependence. Journal of Applied Econometrics, 22(2), 265–312. https://doi.org/10.1002/jae.951
- Pesaran, M. H. (2015). Testing weak cross-sectional dependence in large panels. Econometric Reviews, 34(6-10), 1089–1117. https://doi.org/10.1080/07474938.2014.956623
- Ponce, P Khan,., & S., A., R. (2021). A causal link between renewable energy, energy efficiency, property rights, and CO2 emissions in developed countries: A road map for environmental sustainability. Environmental Science and Pollution Research International, 28(28), 37804–37817. https://doi.org/10.1007/s11356-021-12465-0
- Qian, Y., Liu, J., Cheng, Z., & Forrest, J. Y. L. (2021). Does the smart city policy promote the green growth of the urban economy? Evidence from China. Environmental Science and Pollution Research, 28, 1–15.
- Qiu, S., Wang, Z., & Geng, S. (2021). How do environmental regulation and foreign investment behavior affect green productivity growth in the industrial sector? An empirical test based on Chinese provincial panel data. Journal of Environmental Management, 287, 112282. https://doi.org/10.1016/j.jenvman.2021.112282
- Rahman, M. M., & Velayutham, E. (2020). Renewable and nonrenewable energy consumption-economic growth nexus: new evidence from. Renewable Energy, 147, 399–408. https://doi.org/10.1016/j.renene.2019.09.007
- Razzaq, A., Sharif, A., Najmi, A., Tseng, M. L., & Lim, M. K. (2021). Dynamic and causality interrelationships from municipal solid waste recycling to economic growth, carbon emissions and energy efficiency using a novel bootstrapping autoregressive distributed lag. Resources, Conservation and Recycling, 166, 105372. https://doi.org/10.1016/j.resconrec.2020.105372
- Roy, J., Some, S., Das, N., & Pathak, M. (2021). Demand side climate change mitigation actions and SDGs: literature review with systematic evidence search. Environmental Research Letters, 16(4), 043003. https://doi.org/10.1088/1748-9326/abd81a
- Sadik-Zada, E. R., & Gatto, A. (2021). The puzzle of greenhouse gas footprints of oil abundance. Socio-Economic Planning Sciences, 75, 100936. https://doi.org/10.1016/j.seps.2020.100936
- Santos Pereira, D., & Cardoso Marques, A. (2020). Could electricity demand contribute to diversifying the mix and mitigating CO2 emissions? A fresh daily analysis of the French electricity system. Energy Policy, 142, 111475. https://doi.org/10.1016/j.enpol.2020.111475
- Schumacher, K., Chenet, H., & Volz, U. (2020). Sustainable finance in Japan. Journal of Sustainable Finance & Investment, 10(2), 213–246. https://doi.org/10.1080/20430795.2020.1735219
- Semieniuk, G., & Mazzucato, M. (2019). Financing green growth. In Handbook on green growth. Edward Elgar Publishing.
- Semieniuk, G., Campiglio, E., Mercure, J. F., Volz, U., & Edwards, N. R. (2021). Low‐carbon transition risks for finance. Wiley Interdisciplinary Reviews: Climate Change, 12(1), e678.
- Shahbaz, M., Sharma, R., Sinha, A., & Jiao, Z. (2021). Analyzing non-linear impact of economic growth drivers on CO2 emissions: Designing an SDG framework for India. Energy Policy, 148, 111965. https://doi.org/10.1016/j.enpol.2020.111965
- Sharma, G. D., Rahman, M. M., Jain, M., & Chopra, R. (2021). Nexus between energy consumption, information and communications technology, and economic growth: An enquiry into emerging Asian countries. Journal of Public Affairs, 21(2), e2172. https://doi.org/10.1002/pa.2172
- Shen, Y., Li, X., & Hasnaoui, A. (2021). BRICS carbon neutrality target: Measuring the impact of electricity production from renewable energy sources and globalization. Journal of Environmental Management, 298, 113460. https://doi.org/10.1016/j.jenvman.2021.113460
- Sohag, K., Husain, S., Hammoudeh, S., & Omar, N. (2021). Innovation, militarization, and renewable energy and green growth in OECD countries. Environmental Science and Pollution Research, 28, 1–14.
- Solow, R. M. (1956). A contribution to the theory of economic growth. The Quarterly Journal of Economics, 70(1), 65–94. https://doi.org/10.2307/1884513
- Swan, T. W. (1956). Economic growth and capital accumulation. Economic Record, 32(2), 334–361. https://doi.org/10.1111/j.1475-4932.1956.tb00434.x
- Tadele, E., & Sirany, T. (2021). The relative choice over destiny in a country’s long-run economic growth and economic affluence. Cogent Economics & Finance, 9(1), 1949133. https://doi.org/10.1080/23322039.2021.1949133
- Tawiah, V., Zakari, A., & Adedoyin, F. F. (2021). Determinants of green growth in developed and developing countries. Environmental Science and Pollution Research, 28, 1–16.
- Ulucak, R. (2020). How do environmental technologies affect green growth? Evidence from BRICS economies. The Science of the Total Environment, 712, 136504. https://doi.org/10.1016/j.scitotenv.2020.136504
- Upadhyay, A., Mukhuty, S., Kumar, V., & Kazancoglu, Y. (2021). Blockchain technology and the circular economy: Implications for sustainability and social responsibility. Journal of Cleaner Production, 293, 126130. https://doi.org/10.1016/j.jclepro.2021.126130
- Wang, Q., & Su, M. (2020). Drivers of decoupling economic growth from carbon emission–an empirical analysis of 192 countries using decoupling model and decomposition method. Environmental Impact Assessment Review, 81, 106356. https://doi.org/10.1016/j.eiar.2019.106356
- WDI. (2020). World Bank Development indicators. https://www.worldbank.org/
- Westerlund, J. (2007). Testing for error correction in panel data. Oxford Bulletin of Economics and Statistics, 69(6), 709–748. https://doi.org/10.1111/j.1468-0084.2007.00477.x
- Wu, Y., Tam, V. W., Shuai, C., Shen, L., Zhang, Y., & Liao, S. (2019). Decoupling China’s economic growth from carbon emissions: Empirical studies from 30 Chinese provinces (2001–2015.) The Science of the Total Environment, 656, 576–588. https://doi.org/10.1016/j.scitotenv.2018.11.384
- Yang, J. S. (2017). The governance environment and innovative SMEs. Small Business Economics, 48(3), 525–541. https://doi.org/10.1007/s11187-016-9802-1
- Yu, Z., Khan, S. A. R., Ponce, P., de Sousa Jabbour, A. B. L., & Jabbour, C. J. C. (2022). Factors affecting carbon emissions in emerging economies in the context of a green recovery: Implications for sustainable development goals. Technological Forecasting and Social Change, 176, 121417. https://doi.org/10.1016/j.techfore.2021.121417
- Yu, Z., Ridwan, I., Irshad, A. U. R., Tanveer, M., & Khan, S. A. R. (2022). Investigating the nexuses between transportation infrastructure, renewable energy Sources, and economic Growth: Striving towards sustainable development. Ain Shams Engineering Journal, 34, 101843. https://doi.org/10.1016/j.asej.2022.101843
- Zafar, M. W., Haider Zaidi, S. A., Sinha, A., Gedikli, A., & Hou, F. (2019). The role of stock market and banking sector development, and renewable energy consumption in carbon emissions : Insights from G-7 and N-11 countries. Resources Policy, 62(April), 427–436. https://doi.org/10.1016/j.resourpol.2019.05.003
- Zhang, D., Mohsin, M., Rasheed, A. K., Chang, Y., & Taghizadeh-Hesary, F. (2021). Public spending and green economic growth in BRI region: Mediating role of green finance. Energy Policy, 153, 112256. https://doi.org/10.1016/j.enpol.2021.112256
- Zhang, J., Alharthi, M., Abbas, Q., Li, W., Mohsin, M., Jamal, K., & Taghizadeh-Hesary, F. (2020). Reassessing the environmental Kuznets curve in relation to energy efficiency and economic growth. Sustainability, 12(20), 8346. https://doi.org/10.3390/su12208346
- Zhao, J., Taghizadeh-Hesary, F., Dong, K., & Dong, X. (2022). How green growth affects carbon emissions in China: the role of green finance. Economic Research-Ekonomska Istraživanja, 1–22. https://doi.org/10.1080/1331677X.2022.2095522
- Zhu, H., Duan, L., Guo, Y., & Yu, K. (2016). The effects of FDI, economic growth and energy consumption on carbon emissions in ASEAN-5: evidence from panel quantile regression. Economic Modelling, 58, 237–248. https://doi.org/10.1016/j.econmod.2016.05.003
- Zubair, A. O., Samad, A. R. A., & Dankumo, A. M. (2020). Does gross domestic income, trade integration, FDI inflows, GDP, and capital reduces CO2 emissions? An empirical evidence from Nigeria. Current Research in Environmental Sustainability, 2, 100009. https://doi.org/10.1016/j.crsust.2020.100009
Appendix A
Table A1. Countries examined.
Table A2. Results panel estimation.