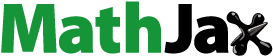
Abstract
The paper seeks to determine whether Bitcoin behaves differently from forex markets and Gold, and whether it offers any diversification, hedging, or safe-haven potential. A Markov regime-switching regression model is employed to determine the relationship between Bitcoin, the real economic activity, foreign exchange markets, financial markets, Energy, and Gold. The results indicate that, unlike USD/EUR and Gold, besides other variables, Bitcoin exhibits significant deviations in terms of its association with other financial and economic variables. Bitcoin appears to be strikingly positively associated with equity markets in both regimes. This may limit its potential to either act as a hedge or a safe-haven for US Equity markets. Bitcoin also deviates considerably from Gold and USD/EUR as it is not affected by the same set of variables as Gold or USD/EUR are under either regime. Moreover, while Gold appears to offer considerably weak safe-haven properties, particularly against equity, Bitcoin fails to be a safe-haven for any of the assets under study. The results, however, indicate that the properties of Bitcoin may range between a diversifier and a hedge, however, such potential of Bitcoin must be viewed with caution owing to the large volatility exhibited by Bitcoin.
1. Introduction
Nowadays, businesses have come to largely rely upon electronic transactions, almost entirely replacing check and cash-based transactions. The viability of electronic transactions is entirely based on trust, as these transactions are performed over platforms where financial institutions serve as trusted third parties. This system, however, is prone to fraud, disputes, mediation costs, hacking, and reversibility of transactions, thus raising the cost and risk of using such services.
Recent innovations in blockchain technology have changed the entire milieu of online transactions by introducing a disruptive mechanism that allows payments to be sent directly from one party to another without relying on a trusted third party. This mechanism involves using cryptographic proofing instead of third-party trust to conduct transactions, which is achieved through the use of cryptocurrencies. This system is highly secure as long as the network nodes control more CPU power than a group of hacking nodes together (Nakamoto, Citation2008). Digital money has been in existence since early 1980s, but to scale its operability as a fully distributed system took more than two decades. Cryptocurrencies, on the other hand, became viral in a short span of time by making the system practically decentralized, economical, and viable. After the introduction of Bitcoin in November 2008, it gained prominence in the financial markets, and investors started comparing it to assets like Gold and other precious metals, thus creating value in Bitcoin as a first-mover through a network effect large enough to maintain its dominance over other cryptocurrencies (Gandal & Halaburda, Citation2016).
Although the popularity of Bitcoin as a positive disruption in digital currencies is widely acknowledged, Bitcoin has many critics. Skeptics like ElBahrawy et al. (Citation2017) argue that Bitcoin is losing its popularity and market share to alternative cryptocurrencies (altcoins such as Ethereum, Litecoin, Ripple, Bitcoin Cash, etc.), which arose as ‘hardforks’ of Bitcoin. Other critics assert that Bitcoin should be outlawed altogether due to the lack of regulation thus giving it a fraudulent feel. As recently as 2017, the Governor of the Reserve Bank of Australia Philp Lowe stated, ‘when thought of purely as a payment instrument, it seems more likely to be attractive to those who want to make transactions in the black or illegal economy, rather than everyday transactions’. But the most prominent criticism that Bitcoin has received is that it is prone to fragile price bubbles. Former chief economist of the International Monetary Fund Kenneth Rogoff (Citation2018), for example, likens Bitcoin to a lottery ticket, and extremely vulnerable to bubble-like collapse. Klein et al. (Citation2018) also report that Bitcoin’s price innovations display a large chain of small to medium bubbles as well as explosive behavior, which demonstrates the highly volatile nature of cryptocurrencies. Cheah and Fry (Citation2015) argue that Bitcoin lacks any fundamental value and contains a considerable speculative element that makes it prone to bubble-like collapse. Baur et al. (Citation2018) tested the hedging capabilities of Bitcoin as compared to foreign exchange and stock markets throughout different periods in a dynamic framework and found that Bitcoin should be considered a speculative asset rather than a transaction medium. Recent volatility in the Bitcoin price and the restrictions on Bitcoin trading and mining (or its use in online transactions) in many countries (especially China) tend to reinforce this view. There are, however, cryptocurrency enthusiasts who are on the path to legalizing and formalizing selected cryptocurrencies. CME Group, for instance, listed Bitcoin futures in December 2017, while NASDAQ and other exchanges have been working for most of 2018 and 2019 to list Bitcoin futures on the exchange. In 2021, many recognized brands and platforms started accepting Bitcoin as a mode of payment; Payment giants—Mastercard, Visa, and Paypal have opened their platforms for transactions in Cryptocurrencies, Bitcoin in particularFootnote1; El Salvador has legalized the use of Bitcoin as a legal tender in June 2021Footnote2, encouraging other crypto-leaning countries to follow the same path. Interestingly, amid the recent Russia-Ukraine conflict and trade sanctions on Russia, Russia is also considering accepting cryptocurrencies, bitcoin in particular, for its oil and gas exports by allowing what it calls ‘friendly’ countries to pay in cryptocurrencies, despite the risks.Footnote3 Bitcoin has also emerged as one of the popular modes for crowdfunding and donations, for instance, the war efforts in Ukraine are being financed through anonymous bitcoin donations. As of March 3, 2022, almost 10 days after the onset of the Russia Ukraine War, more than $54 million have been received in donations for Ukraine’s war efforts.Footnote4 Finally, investment firms are also trying to obtain approval from the Securities Exchange Commission (SEC) to list a Bitcoin ETF on US stock exchanges.
Recent developments and technological innovations in payment systems and platforms have led to the popularization of blockchain technology in which cryptocurrencies are built, leading to the entire milieu of online transactions being disrupted. Cryptocurrencies in general, most notably Bitcoin, became a financial sensation over a very short period and have generated overwhelmingly large interest from both practitioners and academicians as an alternative mechanism for electronic payment systems. Amid the increasing popularity and public interest in Bitcoin, mainstream financial media and financial blogs are abuzz with discussions on the longevity, future, and sustainability of Bitcoin. The research community has largely focused on the fundamental, technical, legal, and security aspects of Bitcoin to the neglect of its possible links to the broader economic and financial determinants. While some argue that ‘Bitcoin works in practice, but not in theory’, others argue that Bitcoin’s stability depends on unknown variables in unknown combinations, and thus it is difficult if not impossible to model precisely, thereby raising doubts about the integrity of the system.
The present study seeks to determine the extent to which Bitcoin is affected, if at all, by changes in economic activity, forex markets, financial markets, energy, and Gold. Moreover, this paper seeks to evaluate whether or not cryptocurrencies can provide safe-haven, hedging, or diversification benefits. This study is expected to contribute to the existing literature by providing more evidence on the relationship between Bitcoin and other financial and economic variables. Furthermore, by analyzing the behavior of Bitcoin, this study provides a brief discussion on the diversification, hedging, or safe-haven potential of Bitcoin to global investors. The remainder of the paper is organized as follows. Section 2 provides a comprehensive review of the literature. Section 3 provides the description of the data. Section 4 describes the research methodology. Section 5 presents the analysis of the results, and section 6 sums up the conclusions derived from this study.
2. Review of the literature
The demand for cryptocurrencies, bitcoin, in particular, is mainly led by investors and users with a broad range of objectives, ranging from its utility as a medium of exchange to a safe-haven. The diversity among the investors in bitcoin has led to a disagreement among academicians and practitioners about the classification of bitcoin into a single category. The holding motives for bitcoin can be broadly classified as transactional, investment, and speculative. While the transactional motives for holding bitcoin are guided by peoples’ demand for money and a payment system, which in turn is a key function of interest rates, inflation, central bank policy, etc. The speculative demand for cryptocurrencies, on the other hand, is led largely due to herding behavior, heuristics, clustering illusion, prospect, and contrarianism (Jiang et al., Citation2022; Lee et al., Citation2020). Ma and Tanizaki (Citation2022), for instance, examined the intra-day price evolution and clustering behavior of bitcoin and discovered the existence of behavioral price clustering in bitcoin prices, oddly during the active trading hours in conventional markets. They attribute the anomalous pattern and price clustering in cryptocurrency exchanges to the domination of retail investors, strategic pricing, and the availability and influence of relevant active information during the daytime. Luis et al. (Citation2019) dismiss the demand for bitcoin as a safe-haven commodity or a medium of exchange while arguing that the short-term demand for bitcoin is mostly speculative and the long-term demand for bitcoin is driven by expectations towards the medium of exchange. Glaser et al. (Citation2014) suggest that the current interest in Bitcoin is not due to its currency aspect but due to its asset nature, and that the increasing transaction volumes are largely due to speculative investors rather than for transaction-related to purchases of goods and services. Schuh and Shy (2016) and Ciaian et al. (Citation2016) also argue that while transaction demand is a large component of cryptocurrency demand, there is also a substantial speculative demand for cryptocurrency. Yermack (Citation2014) further argues that Bitcoin fails on many accounts to qualify as a medium of exchange, and that the volatility in Bitcoin resembles more with that of a speculative asset than a currency. Kristoufek (Citation2013) finds a two-way relationship between Bitcoin users’ interest (Google Trends and Wikipedia) and the Bitcoin prices; they argue that while increases in Bitcoin prices may generate users’ interest, this interest also seems to generate speculative demand for Bitcoin. Huang (Citation2019) argues that the perceived risks and value of investing in bitcoin are not understood by the majority of the investors and that an increased understanding of the perceived risk and return dynamics in bitcoin will subsequently alter their transaction or speculation motive for investment in bitcoin. He further argues that the government intervention is less likely to impact the speculative or transaction demand for bitcoin, would the investors be aware of the risk and return prospect/perception of bitcoin. Sontakke and Ghaisas (Citation2017) also support a similar assertion that cryptocurrencies such as Bitcoin and Ethereum are an evolving asset class, identifying their high-risk prospect as characteristic of their early stage of evolution (similar to technology-based companies coming into existence in the late 90 s and early 2000s). Lewis (Citation2017) on the other hand, suggests that even though the current demand for Bitcoin is mainly driven by speculation, the parallel demand for Bitcoin as a medium of transaction is also growing simultaneously. He also suggests that as the speculative demand for Bitcoin declines, its potential as a transaction tool could further improve. Sahoo’s (Citation2017) study on the volatility of Bitcoin using a GARCH model attributes the growing interest in Bitcoin to its novel mechanism, and the speculative demand. The study also concludes that given Bitcoin may not yet be suitable as an alternative payment system due to its high volatility. However, if its volatility decreases considerably as more liquidity flows into the asset class, then the demand for Bitcoin might shift more towards transaction utility, and thus it may rise as a future digital currency. Nguyen et al. (Citation2018) in a similar study suggest that Bitcoin prices are currently primarily driven by unconventional factors such as media attention. They argue that Bitcoin behaves more like a speculative asset particularly due to the manipulation by ‘Bitcoin whales’, however, the volatility in Bitcoin prices has declined over time due to the introduction of Bitcoin futures and drastic changes in regulation. Similar results have been reported by Bouoiyour et al. (Citation2014), who assert that Bitcoin is being considered more like a speculative asset, rather than a medium of exchange.
Iwamura et al. (Citation2019) explore the potential of Bitcoin to replace or compete with central bank money, and conclude that even though Bitcoin is extremely efficient, secure and decentralized, and operates on a ‘proof of work’ system, it doesn’t have the potential to replace the existing monetary system that runs on central bank money. They further argue that cryptocurrencies are subject to extreme volatility and are thus subject to inflationary or deflationary tendencies. Gandal and Halaburda (Citation2016) argue that a decreasing hash rate limits the production rate of Bitcoins and puts deflationary pressure on the system. This being so, the belief that Bitcoin can operate as a currency without inflation doesn’t hold any water in its current legal and operational standing. Raymaekers (Citation2015) argue that Bitcoin and other cryptocurrencies are currently marred by critical regulatory and legal challenges, and thus don’t seem to offer a potential alternative to the existing payment systems. Furthermore, as cryptocurrencies are not linked to any monetary policy instruments, the US Commodity Futures Trading Commission (CFTC) clearly distinguishes cryptocurrencies from fiat money by classifying all digital virtual currencies as crypto-assetsFootnote5 that fall under commodities. Tiwari (Citation2018) supports the classification of cryptocurrencies as a commodity, and also provides a legal framework to remove various legal hurdles that stand in the way of Bitcoin’s operation, but he also recognizes that there is no general consensus or support for cryptocurrencies replacing the current payment system. In another study, Raskin and Yermack (Citation2016) explore the potential lessons for digital currencies and central banks to learn to digitize currencies. They argue that the blockchain mechanism has the potential to bring about a positive disruption that improves the payment and settlements system, and may also help central banks to issue their own centralized digital currency. The proponents of cryptocurrencies also argue that a more comprehensive and accommodative operational and legal framework is needed to integrate cryptocurrencies into the formal fold of payment systems (Harwick, Citation2016; Yermack, Citation2014). It is further argued that such integration would not only improve payment systems but would also bring about confidence and consistency in economic policies (Harwick, Citation2016; Raymaekers, Citation2015). Bonneau et al. (Citation2015) argue that although Bitcoin’s price is driven by a set of unknown economic variables, it nonetheless provides a mechanism for developing universal payment systems which are more decentralized, secure, and relatively less costly. They also argue that the relationship between Bitcoin and the variables affecting it must be more actively and assiduously examined by the research community to re-assuring this mechanism for payments.
Besides legal and regulatory challenges, as opposed to fiat currency, Bitcoin is largely used for speculative purposes rather than for transaction purposes. Cryptocurrencies in general, and Bitcoin in particular, have seen considerable turbulence during and towards the end of 2018 with Bitcoin breaching the USD 10,000 mark, touching USD 19,000, and falling back to USD 3,300. A similar pattern was once again witnessed in late 2020 when the Bitcoin touched USD 60,000 mark and then falling down to USD 30,000 in mid-2021. In April 2021, a flash crash in the cryptocurrency market wiped out about USD 300 Billion in less than 24 hours and about USD 1 trillion in one week, which is about more than 15% and 50% of the total cryptocurrency market, respectivelyFootnote6. This exponential volatility remains to be a cause of substantial concern among cryptocurrency enthusiasts as well as encouraging skeptics to pitch their dismal predictions and criticisms. Bouoiyour et al. (Citation2014) attribute the turbulent and speculative nature of Bitcoins to the Chinese Stock Market Index (Kristoufek, Citation2015) and the increasing hash rate. Yermack (Citation2014), by comparing the volatility of fiat currencies and Bitcoin, concludes that the current uncertainty and price volatility of Bitcoin undermines its ability to compete with fiat currency. Dehouche (Citation2022) examines the volatility of bitcoin by comparing it to Gold and the S&P 500 and concludes that the volatility of bitcoin shows a considerable diversion in terms of the concepts of volatility. He further argues that the volatility in bitcoin is an intra-day phenomenon that gets considerably reduced for longer time-frames (weekly or monthly), while the opposite is true for Gold. In a recent study by Jiang et al. (Citation2022), examining the dynamics and spillover among bitcoin and other financial markets, they conclude that bitcoin transmits volatility to other financial markets, therefore suggesting the movement of bitcoin towards the center of financial markets.
Although a significant portion of the literature about cryptocurrencies deals with the operational issues of the blockchain mechanism, considerable interest in the possible financial aspects has also been generated among researchers. A few studies addressing the volatility characteristics and other economic and financial aspects of cryptocurrencies are briefly summarized in this section. A few empirical studies suggest that there is a weak relationship between Bitcoin and conventional financial assets because each is driven by a different set of causal factors (Bouoiyour et al., Citation2014; Kristoufek, Citation2015). While financial assets are affected by economic and financial variables, Bitcoin depends on a unique variety of factors like its exotic appeal, illegal activity, security, and anonymity, among other factors which remain unknown (Kristoufek, Citation2015; Yelowitz & Wilson, 2015). Ciaian et al. (Citation2016) found that Bitcoin prices are mainly attributed to supply and demand generated mostly by investors departing from rationality (Bouri et al., Citation2019). The volatility in Bitcoin prices might be caused by Chinese stock indices (Kristoufek, Citation2015), Bitcoin volumes (growing interest in Bitcoin as a speculative asset), the Dow Jones Industrial Average Index, and hash rate (Bouoiyour and Selmi (Citation2014). Hernandez et al. (Citation2022) argue that the relationship of bitcoin with economic policy uncertainty (EPU) changes over different time horizons, they found that the EPU affects the bitcoin negatively in the short-run and positively in the long run. Mokni et al. (Citation2021) argue that while bitcoin does not act as a hedge against EPU as an aggregate measure, it provides considerable hedging potential against fiscal policy, trade policy, and other specific political and economic uncertainties. In a similar study, Yin et al. (Citation2021) argue that the oil price shocks may produce an effect similar to macroeconomic events, and therefore, oil prices may be a key factor/determinant in cryptocurrency uncertainty. Palombizio and Morris (Citation2012) assess the impact of global macroeconomic indicators on Bitcoin and report an indirect effect of oil prices on Bitcoin price evolution, whereas Ciaian et al. (2016) report no impact of oil prices on Bitcoin. Samah et al. (Citation2018), found that Bitcoin is sensitive to macroeconomic indicators, and thus behaved in a similar fashion to Gold and the US Dollar. They conclude that since the response of Bitcoin to macroeconomic indicators is more frequent and rapid, it has some hedging to diversification properties.
Research also suggests that Bitcoin is a good choice for portfolio diversification due to its anomalous behavior, its resemblance to Gold, as well as its relationship with unknown economic and behavioral factors (Bouri et al., Citation2017a; Dyhrberg, Citation2016a, Citation2016b). Pal and Mitra (Citation2019) show that Bitcoin can provide a hedge against volatility in equity markets, Gold, and commodities. They also indicate that the hedging effectiveness of Bitcoin is highest with Gold; a long position in Bitcoin provides a hedge with a short position in Gold. Dyhrberg (Citation2016a) and Palombizio and Morris (Citation2012) classify Bitcoin as an asset with price behavior resembling Gold and also bears some resemblance to the US Dollar. Tschorsch and Scheuermann (Citation2016) argue that while the investment potential of Bitcoin is huge due to its price behavior resembling that of Gold, its ability to transform the payment system (and to be one itself) must not be undermined. Conrad et al. (Citation2018) use a GARCH-MIDAS model to study the factors affecting Bitcoin volatility and conclude that Bitcoin volatility is positively associated with real economic variables and negatively related to volatility in the financial markets. Their results indicate that Bitcoin volatility is less during the ‘flight to safety’ periods and periods of high volatility in the US stock markets (Kristoufek, Citation2015), while the volatility has a positive and significant association with real economic activity. They further argue that although most research compares Bitcoin with precious metals like Gold (Baur et al., Citation2018), its behavior is unlike precious metals; precious metals are counter-cyclical whereas Bitcoin is pro-cyclical. Baur et al. (Citation2018) indicate that Bitcoin returns are not related to conventional assets like stocks or bonds, and thus can be a potential asset for portfolio diversification.
During periods of market turbulence, investors seek assets that are uncorrelated or negatively correlated with conventional financial assets and commodities. Unfortunately, conventional safe havens like Gold are dissipating fast (Gantori et al., Citation2017). Thus, some research has been conducted into the question of whether cryptocurrencies (such as Bitcoin) are capable of serving as either diversifiers, hedges, or safe-havens. Research suggests that due to the unique risk-return characteristics of Bitcoin and its uncorrelated nature with other assets, Bitcoin might serve as a safe-haven against global financial stress, commodities, and energy (Bouri et al., Citation2017b, Citation2017c, Citation2018), a hedge against equities, currencies, commodities, and VIX (Baur et al., Citation2018; Bouri et al., Citation2017a, Citation2017c; Chan et al., Citation2019; Dyhrberg, Citation2016a). Park (Citation2022), for instance, examines the trading behavior and the impact of market stress on the dynamics of the relationship among bitcoin futures and trading behavior variables and concludes that the trading volumes and volatility of bitcoin increased during the COVID-19 pandemic, while the returns decreased substantially. On the properties of bitcoin as a safe-haven during turbulent markets, owing to strong positive correlations between Bitcoin and Gold during periods of market turmoil, Jareño et al. (Citation2020) argue that Bitcoin may offer some safe-haven potential. However, Shahzad et al. (Citation2020) assert that there are considerable differences between Bitcoin and Gold in terms of safe-haven, hedging, and diversification properties, they argue that Gold is a better safe-haven, hedge, and diversifier compared to Bitcoin. Jiang et al. (Citation2022) in a similar study discovered that the volatility spillovers in bitcoin are significantly correlated with other financial markets, and therefore, its potential in acting as a safe-haven is rather limited. They further argue that bitcoin may provide considerable potential as a hedge against financial markets and commodities.
At this stage, however, the results are mixed. As defined by Baur and Lucey (Citation2010), unconventional assets positively correlated to conventional assets can act as diversifiers. An uncorrelated or a negatively correlated unconventional asset can provide a hedge for the conventional assets, while an unconventional asset that is uncorrelated or negatively correlated with conventional assets serves as a safe-haven only in ‘flight to safety’ periods. The existing literature supports that Bitcoin can provide diversification opportunities or act as a short-term hedge (Bouri et al., Citation2017b; Corbet et al., Citation2018; Dyhrberg, Citation2016a). By applying APARCH and FIAPARCH models to analyze the leverage effect and long memory, Klein et al. (Citation2018) show that although Bitcoin has a similar asymmetric response as observed for Gold and silver, the persistence in Bitcoin is fairly different. They further conclude that the connectedness of Bitcoin to markets is considerably different from that of Gold; there are, however, short-lived temporal similarities that dissipate after corrections. They further argue that in terms of being a safe-haven during the ‘flight to quality’ periods, although Gold’s safe-haven nature is established, Bitcoin shows a positive decoupling effect and fails to provide a hedge or a safe-haven against equity markets/instruments. Shahzad et al. (Citation2019), on the contrary, show that the safe haven nature of Bitcoin is market and context-dependent. By applying the rolling window approach, they find that while Bitcoin offers a hedge or safe-haven during extreme market movements in developing economies, it fails to offer a hedge against the developed markets where the only safe-haven is Gold. They also propose a new definition of safe-haven, and classify Bitcoin and Gold as ‘weak’ safe havens in some cases. On the other hand, Charfeddine et al. (Citation2020) found that the diversification or hedging potential of cryptocurrencies is dependent upon the economic and political scenarios, and therefore, they concluded that the cryptocurrencies can be considered for portfolio diversification, however, they are poor hedges, in most cases. Similar results were reported by Das et al. (Citation2020) for Bitcoin, they argue that Bitcoin does not offer superior hedging capabilities and that the hedging efficacy of Bitcoin is sensitive to market conditions, and therefore, must be viewed with caution, particularly during uncertain economic conditions (Hernandez et al., Citation2022). Similar results are reported by Shahzad et al. (Citation2022) during COVID for BRICS economies. They report that while bitcoin and gold may offer conditional diversification and hedging benefits for BRICS economies during the COVID-19 pandemic, gold offers the highest potential for diversification and hedging, while employing bitcoin as an alternative asset for diversification or hedging should be considered with extreme caution, despite its huge popularity among the institutional investors. Symitsi and Chalvatzis (2019) found that Bitcoin can offer substantial diversification benefits in regime-dependent (bearish and bullish) market conditions. Chemkha et al. (Citation2021) in their study on major stock indices and currencies during the COVID-19 crisis found that while Bitcoin and Gold can hedge risk in the international portfolios effectively, Gold only acts as a weak safe-haven and Bitcoin does not offer any safe-haven potential. Chkili et al. (Citation2021) examine the safe-haven potential of bitcoin for Islamic stock indices during the COVID-19 pandemic and found that while the diversification potential of bitcoin considerably increases during the pandemic, the hedging strategy involving bitcoin requires higher costs during the crisis.
Corbet et al. (Citation2020), on the other hand, in their study on Chinese financial markets during the COVID-19 pandemic assert that Bitcoin does not act either as a hedge or safe-haven. Choi and Shin (Citation2021) also found that bitcoin prices are correlated with the economic policy uncertainty, particularly during the COVID-19 pandemic, and therefore do not act as a hedge or a safe-haven. Similar results were reported by Conlon and McGee (Citation2020) and Karamti and Belhassine (2021) for US markets during the COVID-19 crisis. They found that Bitcoin and the S&P 500 index were strikingly positively correlated during the development of the COVID-19 crisis, therefore dissipating any potential of Bitcoin to act as a safe-haven for US Equity markets. In a similar study, Goodell and Goutte (Citation2021) report that the benefits of Bitcoin in portfolio diversification for an Equity portfolio are very thin during the COVID-19 crisis, they argue that the co-movements between Bitcoin and Equity markets gradually spiked as the crisis progressed, thereby reducing the benefits of Bitcoin to portfolio diversification. On the other hand, Su et al. (Citation2022) studied the dynamic relationship between Bitcoin and VIX and found that Bitcoin does not act as a safe haven during the high fear sentiment. They, however, found a striking signal transmission between BTC and VIX and concluded that the high fear sentiment can potentially provide a signal for investors to alter their investment strategies with regard to portfolio diversification with Bitcoin through portfolio rebalancing. Obeng (Citation2021) reported the existence of asymmetry in the cryptocurrency markets and concluded that the cryptocurrency markets are characterized by regimes of bad sentiment and good sentiment. While cryptocurrencies strongly respond to the negative market sentiment represented by a huge rise in volatility, the same is not reflected during the positive news regime.
3. Data description
The current study aims to examine as to what extent, if at all, is Bitcoin related to global economic activity, forex markets, equity markets, energy markets, fixed-income assets (corporate bonds), and commodities (Gold). The study further examines whether Bitcoin expresses any different behavior than currencies (proxied by USD-Euro) and Gold in terms of its potential to act as either a hedge, diversifier, or a safe-haven. The following proxies were employed to model the proposed relationship; Bitcoin (BTC) for Bitcoin (cryptocurrencies), the Baltic Dry Index (BALTICF) for real economic activity, USD to EURO (USEURSP) for Forex markets, S & P 500 for equity markets (S&P), the Dow Jones UBS Energy Spot Subindex (DJUBENS) for Energy Markets, the iShares Long-Term Corporate Bond ETF (U:IGLB) for Corporate Bonds, and the CMX-Gold 100 ounce (NGCC.01) for Gold. The data for all variables have been collected from the Refinitiv Eikon database for the period January 1st, 2014 to July 15th, 2021. The data is of daily frequency and each variable has been sorted and time-stamped for all variables with a total of 1,967 observations across all variables.
Bitcoin has remained the most prominent cryptocurrency in the decentralized digital payments ecosystem despite a phenomenal growth of altcoins in recent times. As of August 2021, the market capitalization of Bitcoin is approximately USD 920 billion which is roughly 45% of the total cryptocurrency ecosystemFootnote7. The Baltic Exchange Dry Index (BDI) provides insights into the global supply and demand trends and is considered an indicator of global economic activity. The index was first introduced in January 1985 by the London-based Baltic Exchange. BDI is a composite of Capesize, Panamax, Handysize, and Supramax sub-indices. It measures the changes in the cost of transporting raw materials across more than 20 different sea routes. USD/EUR exchange rate is considered the most important indicator across worldwide forex markets. The importance of the USD/EUR exchange rate is due to the investment and trade of these two large economic areas with each other. The trade and investment among the two regions is such that the prices in these economic regions are arbitraged against the exchange rateFootnote8. Introduced in 1957, the S&P 500 index is a widely followed indicator and is regarded as the best gauge of large-cap US equity markets. The index includes the top 500 leading companies making up around 80% of the available market capitalizationFootnote9. The Dow Jones UBS Energy Spot Subindex measures the price movements of energy included in the Bloomberg subindices - crude oil, heating oil, natural gas, and unleaded gasoline. The iShares Long-Term Corporate Bond ETF is an index of US-denominated investment-grade corporate bonds with remaining maturities greater than 10 yearsFootnote10. Finally, CMX-Gold 100 ounce is the price of 100 ounces of Gold for spot delivery.
4. Research methodology
As part of preliminary time series testing, we implement a unit root test. The study employs the Kapetanios and Shin (Citation2008) unit root test to confirm whether the data is stationary, as non-stationarity in time series data could lead to spurious innovations in the results. There is a growing consensus among researchers that the traditional unit root tests fail to consider nonlinearity, fractional integration, and other deterministic properties of time series (Bierens, Citation1997; Gil-Alana & Robinson, Citation1997; Kapetanios et al., Citation2003; Kapetanios & Shin, Citation2008; Leybourne et al., Citation1998). Since the Markov Regime Switching Regression (MRSR) model employed in this study requires the data to follow a non-linear structure, it is more appropriate to use the Kapetanios and Shin (Citation2008) unit root test as the traditional tests could lead to spurious results.
To confirm the non-linear structure of the series, the residuals of the estimated ARFIMA model are generated to perform the BDS (Brock, Dechert, and Scheinkman) test. The BDS test is employed to check for the stochastic linearity of returns. BDS is a portmanteau test for independence and identical distribution but has been extended to test for deviations from independence including non-linear dependence. After checking for non-linear dependence in the series, MRSR models are employed to analyze the asymmetric relationship between the regime-dependent variables. The model, thus, examines the impact of the analyzed variables on Bitcoin prices, and well as the switching properties of Bitcoin from bull to bear regime and vice-versa. The specification of the models is presented below.
4.1. The Kapetanios and Shin (K & S) unit root test
The Kapetanios and Shin (Citation2008) unit root model is given by the following analogous ESTAR specification:
(1)
(1)
where
is the demeaned or detrended time series of interest, and
is the exponential transition function to present the nonlinear adjustment. Given
contains a unit root when
while
is globally stationary when
Therefore, under the null hypothesis, the series follows a linear unit root process whereas, under the alternative hypothesis, the series is non-linear stationery.
To test the null hypothesis, reparameterization by computing a first-order Taylor series approximation of EquationEq.(1)(1)
(1) is suggested by KSS, to obtain the following auxiliary function:
(2)
(2)
Where the serially correlated errors are corrected with augmentations, and
with a t-test hypothesis for
against
the asymptotic critical value of t-statistics is computed as:
where
is the OLS estimate of
and
is its standard error. Kapetanios and Shin (Citation2008) argue that the OLS-based KSS test demonstrates low power, and therefore suggest a modified KSS test with GLS residuals. They confirm through a simulation procedure that the GLS detrending-based KSS test is superior in terms of power to the OLS-based test.
4.2. The ARFIMA model
Since most financial markets exhibit long memory properties, the systems working with short memory parameters fail to capture this long memory component (Liu et al., Citation2017; Sheng et al., Citation2011). Most financial time series exhibit long-range dependence that can be classified as I(0) and not I(1). To more accurately capture the long memory component for time series exhibiting fractional properties, a more flexible class of process known as autoregressive fractionally integrated moving averages (ARFIMA) model has been introduced by Granger and Joyeux (Citation1980), Granger (Citation1980), and Hosking (1981). The ARFIMA process of a time series of order (p, d, q) is given by:
(3)
(3)
where the fractional differencing parameter is denoted by d, and
is the white noise. The stochastic process
is both stationary and invertible if all roots of
and
lie outside the unit circle and
the process is nonstationary for
Granger and Joyeux (Citation1980). The autocorrelation function,
is proportional to
as
indicating the slow rate of hyperbolic decay (Hosking, 1981).
4.3. The Brock, Dechert and Scheinkman (BDS) test
The BDS test developed along with the chaos theory by Brock et al. (Citation1987) is one of the most popular tests for detecting general stochastic non-linearity. It is a nonparametric test originally designed to test for independence and identical distribution but has been extended to test for deviations from independence including non-linear dependence (or ‘chaos’). The test can be applied to a series of estimated residuals to check whether the residuals are independent and identically distributed. The correlation integral at the embedding dimension,
is estimated by the following:
(4)
(4)
where
denotes an indicator function and is given by:
The correlation integral estimates the probability that any two -dimensional points are within a distance of
of each other (Bisaglia & Gerolimetto, Citation2014):
If the are IID, the probability equals the following:
The BDS statistic as defined by Brock et al. (Citation1987) is given by:
where
denotes the consistent estimate of the asymptotic standard deviation.
4.4. The Markov Regime Switching Regression (MRSR) model
Hamilton (Citation1989) introduced a model of order p, where the time series can assume substantially different characteristics by forming regimes. The model is generally applicable to economic scenarios such as policy changes (e.g. expansionary vs contractionary fiscal policy stances), as well as to financial structures where the data can assume multiple structures such as bearish vs bullish regimes, as is relevant to this study. The distinguishing feature of this model is that it allows for switching between the bear-bull regimes and allows one to control this switching mechanism through the unobservable state variable which follows the first-order Markov chain (Kuan, Citation2002). For two regime data, the model can be presented as:
(5)
(5)
where each
is independent of each other and are normally distributed with a given mean and variance (0,
). The unobservable state or regime variable is governed by a first-order Markov chain with the transition probabilities:
A small value indicates that the model stays longer in state
with an expected duration
in state
5. Empirical analysis
As previously stated, this study examines the impact of several financial and economic indicators to understand the resemblance (if any) between Bitcoin and the conventional investment asset classes with particular attention given to determining whether it could contribute to portfolio diversification, acts as a hedge, or serve as a safe-haven asset. The study employs a Markov-regime regression model to ascertain whether or not bitcoin considerably resembles or differs from other assets in its relationship with other variables in a regime-dependent model, and whether it presents any potential safe-haven properties.
To carry out the analysis, it is important to employ the variables in their stationary state. Since the non-stationary time series data could lead to spurious innovations in the results, the unit root test is used to confirm whether the data is stationary. Conventional tests such as the Augmented Dickey-Fuller (ADF) Test and the Philips-Perron (PP) unit root test have largely been used in the existing literature to test for a unit root in the time series. However, these tests may fail to reject the unit root hypothesis in the presence of a structural break (Perron, Citation1989). Zivot and Andrews (Citation1992) have shown that one can check for unit root by allowing a single structural break. Moreover, Lumsdaine and Papell (Citation1997) and Kapetanios (Citation2005) have shown that one can extend the test by allowing anywhere between two and m breaks, respectively. However, when the time series of interest is non-linear, the traditional unit root tests fail to consider nonlinearity, fractional integration, and other deterministic properties of time series (Bierens, Citation1997; Gil-Alana & Robinson, Citation1997; Kapetanios et al., Citation2003; Leybourne et al., Citation1998). Therefore, this study has employed the Kapetanios and Shin (Citation2008) unit root test to capture the non-linear dynamics of time series. The test results are presented below:
The results presented in indicate that all the variables are stationary at level at the 99% confidence interval based on the SIC criteria, and stationary at level at 90% confidence level based on all criteria. Since the results confirm that all the variables chosen for the study are stationary at a level, we can proceed with the raw returns series at I(0) level. The further analysis is conducted by employing the Markov-Regime Switching (MRSR) to examine the nature of interactions and impact of the selected variables on Bitcoin prices, as well as the probability of transitioning from a bull to bear regime and vice-versa. The model requires the returns series to have a non-linear structure and to test that the BDS test is employed to check for the stochastic linearity of returns. Thus, the residuals of the estimated linear models (ARMA, ARIMA, or ARFIMA, for instance) are generated to perform the BDS test as demonstrated by Brock and Sayers (Citation1988). This analysis is based on the ARFIMA structure of the returns, which is presented in .
Table 1. Kapetanios and Shin (Citation2008) unit root test.
Table 2. ARFIMA structure of the returns series.
The ARFIMA (p,d,q) model considers the long-run dependence or persistence in the time series with a fractionally (non-integer) integrated differencing parameter. The concept of fractional integration often refers to defining the long-range dependence or long memory, while a pure ARIMA process represents a short memory, where the value of past disturbances follows a geometric decay and almost quickly dies out. Against the reference ranges of 0 < d < 0.5 for long memory (Baillie, Citation1996), Bitcoin and Energy autocorrelation function follows a long memory or hyperbolic decay, which indicates a deviation from the weak form of market efficiency, and thus non-random chaotic behavior. It may thus be concluded that Bitcoin exhibits different volatility characteristics in comparison to ordinary fiat currencies (USD/EUR exchange rate), Gold, and other selected variables as indicated by their d parameter in . The extracted residuals of the ARFIMA estimations are then used in the BDS independence test. This test checks whether there is any non-linear dependence in the series and if the residuals of the ARFIMA process (linear fitted model) follow an independent and identical distribution (i.i.d). Simply put, the observations of a pure i.i.d series have a constant probability of the distance between any two points being less than or equal to epsilon (ε). The model requires parameters ε and m; ε being the epsilon value assigned to the difference between the consecutive m-histories (the embedding dimension). For the residuals of ARFIMA, epsilon values between 1 and 2, and embedding dimensions 3, 5, and 7 have been employed (Hsieh & LeBaron, Citation1988). The BDS test results thus obtained are presented in .
Table 3. BDS test results.
The BDS test can be used to detect various possible deviations from independence (such as chaotic behavior) and can be employed to test against arbitrary dependence by using the correlation function—the asymptotic distribution of which is known under the assumption of the null hypothesis (Barnett et al., Citation1996). A BDS test statistic above the critical value is required for the null hypothesis to be rejected. As observed in , the BDS test statistic for all variables (except Gold at embedding dimension 3) is indeed above the recommended critical values of 1.960 and 2.575 at 95% and 99% confidence intervals, respectively (Broock et al., Citation1996). Therefore, the null hypothesis is rejected for all the variables at all epsilon values and embedding dimensions. The results indicate deviation from the i.i.d property, and a non-linear dependence in all variables, thus making it convenient to use for the MRSR model.
The MRSR model is useful when the parameters of a data generating process vary over a set of different observed states. The model can estimate the probability of each possible state, and the transitions thereof, and thus is useful in application to economic cycles (Recession/Expansion), financial markets (Low/High volatility), and interest-rate regimes (Expansionary/Contractionary), etc. An MRSR model thus enables the analysis of the asymmetric relationship between the regime-dependent variables. Since, financial markets, and more recently cryptocurrency markets, have witnessed increased volatility (switching between the bearish and bullish phases). The current study is an attempt to examine the behavior of Bitcoin across different phases of volatility by using the MRSR model in comparison to other variables, Gold and Forex, in particular. The MRSR results are presented in .
Table 4. Markov regime switching regression.
As the MRSR model does not itself label the regimes, the regimes are labeled on the basis of average returns represented by their constants. Therefore, Regime 1 represents a bullish regime and regime 2 represents a bearish regime for all variables. In Regime 1, Bitcoin is positively related to the S&P 500 and Gold, while negatively related to Energy (p-value < 0.1); whereas in Regime 2, Bitcoin is positively related to USD/EUR and S&P 500, while negatively related to Energy. Gold, on the contrary, is positively related to USD/EUR, Energy, and Bonds, and negatively related to S&P 500 in Regime 1, whereas, in Regime 2, positively related to Bitcoin (p-value < 0.1), S&P 500, and Bonds, and negatively related to BDI, USD/EUR (p-value < 0.05), and Energy. Similarly, USD/EUR is positively related to Bitcoin (p-value < 0.1) and Gold, and negatively related to Bonds (p-value < 0.1) in Regime 1, whereas, in Regime 2, it is positively related to Bonds and negatively related to S&P 500 at 90% and 95% confidence intervals, respectively. These results indicate that the behavior of Bitcoin is considerably different from both the USD/EUR and Gold in terms of its relationship with different variables under study in regime-dependent models. The results also suggest that while Gold and Bonds appear to be significantly positively related in both the regimes, Gold offers better hedging and safe-haven potential compared to both the bonds as well as the Bitcoin due to its substantial and negative relationship with variables across regimes. To further substantiate the argument, it is observed that Bitcoin is positively (though not considerably) related to USD/EUR (p-value < 0.1) S&P 500, and Gold (p-value < 0.1); and negatively related to Energy and Bonds in Regime 1 (Bullish Regime) of the underlying variables, whereas, unrelated to these any of those variables in Regime 2 (Bearish Regime) of those variables. Gold, on the contrary, is positively related to Bitcoin, USD/EUR, S&P 500, and Energy in Regime 1 of the underlying variables; whereas, negatively related to S&P 500 and positively related to Bonds, and not related to any other variables in Regime 2. This indicates that while Gold may offer considerable hedge to safe-haven potential (particularly for the equity markets), Bitcoin can at best offer potential as a diversifier or a hedge. These results are consistent with Charfeddine et al. (Citation2020), Das et al. (Citation2020), Shahzad et al. (Citation2020), Bakry et al. (Citation2021), Choi and Shin (Citation2021), Jiang et al. (Citation2022), and Shahzad et al. (Citation2022) who suggest that Bitcoin can, at best, act as a diversifier or a hedge.
Moreover, since Bitcoin demonstrates no resemblance with the properties of USD/EUR or Gold, as it is not affected by the same set of variables as USD/EUR or Gold are under either regime, it can be concluded that Bitcoin considerably deviates from both. However, owing to the nature of its relationship with other variables across regimes, it can be concluded that Bitcoin offers a considerable potential in portfolio diversification which may extend to hedging, but it would be going too far to classify ‘Bitcoin as the new Gold’. According to the definition of a diversifier, hedge, and safe-haven proposed by Baur and Lucey (Citation2010), Bitcoin may be classified as a potential diversifier, or at most, a hedge, particularly against energy and bonds. A safe-haven requires an asset to have a negative association with other assets, particularly during negative market movements. Based on this fundamental property of a safe-haven, Bitcoin does not seem to resemble these properties as it is not related to any variables in the bearish regime (Regime 2). To further explore the safe-haven, hedge, or diversifier properties of Bitcoin, we examined the dynamic conditional correlation of Bitcoin with all assets, which is presented in .
Figure 1. Dynamic conditional correlation graphs (DCC-GARCH). Source: Authors' estimations/calculations.
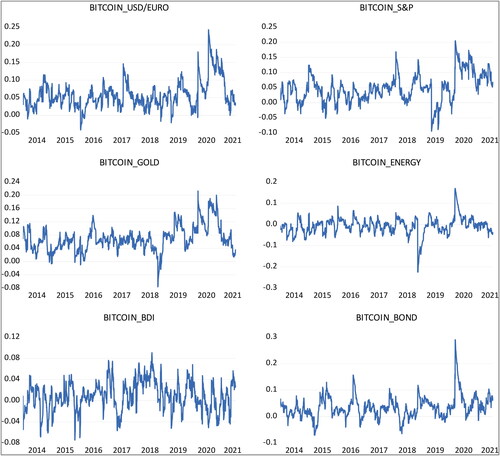
As depicted by the graphs above, we follow the approach followed by Ngene et al. (Citation2018) to investigate the time-invariant interactions in volatility among different assets. Specifically, we explored the dynamic conditional correlation of Bitcoin with all assets as shown in . The results indicate that, on average, Bitcoin exhibits a very low dynamic conditional correlation with USD/EUR, the S&P 500, Gold, and Bonds. However, it exhibits a negative dynamic conditional correlation with Energy and no discernible pattern of conditional correlation with BDI. These results suggest that Bitcoin may provide considerable potential for portfolio diversification, in general, as well as some hedging potential against Energy and global economic activity (proxied by BDI). Moreover, upon careful examination of the shift in the dynamic conditional correlations during the periods of market distress (marked by the COVID-19 crisis in early 2020), we find a sharp rise in the conditional correlation of Bitcoin with USD/EUR, Gold, S&P 500, and Bonds. These findings are consistent with our earlier analysis suggesting that Bitcoin, at best has the potential to act as a diversifier or a hedge, rather than a safe-haven. These results are consistent with Corbet et al. (Citation2020), Conlon and McGee (Citation2020), Karamti and Belhassine (2021), and Goodell and Goutte (Citation2021).
Moreover, it is often argued that Bitcoin may not act as a stable hedge or a safe-haven due to its extreme volatility, unknown set of determinants, absence of institutional investors, the dominance of retail investors, lack of proper regulation, and market mechanism, legal, and environmental concerns. With respect to the concern of extreme volatility, we compare the standard errors of Bitcoin and find that it has a considerably higher set of standard errors compared to other variables. This may suggest that Bitcoin price discovery is driven by some speculative components and thus is more volatile. Furthermore, since the standard errors for the bearish regime are considerably higher compared to the bullish regime, particularly for Bitcoin, it could be concluded that the volatility in Bitcoin is prone to a downward spiral due to the dominance of retail investors.
The transition probabilities are presented in below. It can be observed from the transition probabilities in and , that although most of the variables move considerably between bullish and bearish trends, for the most part, the Bitcoin price exhibits a bullish trend, and the probability of transition from bearish to bullish trend is quite large. This transition probability bears a striking resemblance with Gold. Specifically, the probability of staying in Regime 1 for Bitcoin and Gold is 0.97 and 0.99, respectively, while the probability of staying in Regime 2 for Bitcoin and Gold is 0.12 and 0.14, respectively. The corresponding probability of transition from Regime 2 to Regime 1 is 0.88 and 0.86 for Bitcoin and Gold, respectively. Nevertheless, this resemblance is not sufficient to argue that Bitcoin carries the same safe-haven properties as Gold, if not substantiated by other measures.
Figure 2. Filtered regime probabilities.
Note: The Figure represents the transition probabilities of all the assets under the study in a two-regime Markov Regime Switching Regression Model. The graphs indicate that Bitcoin and Gold mostly stay in Regime 1 (Bullish Regime) with a considerably low transition to Regime 2 (Bearish Regime). USD/EUR demonstrates a constant transition between the regimes and almost equal durations in either regime. BDI, likewise, exhibits a high probability for both regimes to exist with a considerable probability to transition between the regimes. On the other hand, S&P 500, Energy, and Bonds tend to mostly stay in regime in Regime 2 (Bearish Regime) with a considerable probability of transition from Regime 1 to Regime 1. Source: Authors' estimations/calculations.
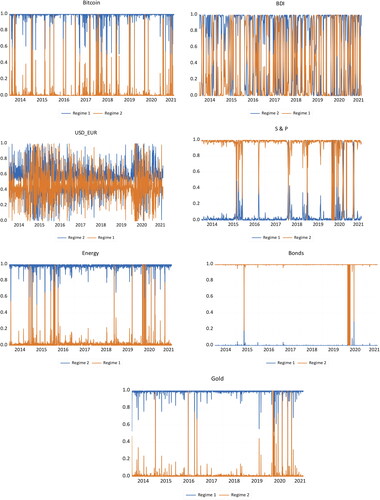
Table 5. Transition probabilities.
6. Conclusions
Bitcoin and other cryptocurrencies (altcoins) have attracted increasing attention over the last few years. Despite numerous controversies and substantial legal uncertainties, cryptocurrencies continue to gain interest and legitimacy, besides their entry into the derivatives market. The study aims to understand bitcoin from the perspectives of a medium of transaction, a speculative asset, a diversifier, a hedge, or a safe-haven, and therefore, compares it to currency, commodities, other financial assets, and gold. This study examines the relationship between Bitcoin, global economic activity, foreign exchange markets, equity markets, Energy markets, Bonds, and Gold, and explored the potential for Bitcoin to act as a safe-haven, a hedge, or a diversifier, with a particular focus on being classified as a medium of transaction and a hedge (or a safe-haven). The results indicate that Bitcoin behaves differently than both the USD/EUR and Gold vis-à-vis its relationship to different variables. Our study finds that Bitcoin exhibits a positive relationship with equity markets in both the bullish and bearish regimes, a positive relationship with the Forex markets and Gold in the bullish regime, and a negative relationship with Energy in both regimes. Moreover, the study suggests that Bitcoin differs considerably from USD/EUR and Gold in terms of its relationship with the examined variables under both regimes. The results also indicate that Bitcoin is related to all the variables except BDI in the bullish regime while not related to any of the variables in the bearish regime, contrary to the properties of Gold. It may, therefore, be concluded that Bitcoin fails to provide any potential safe-haven properties as characterized by Gold, rather it may act as a diversifier or a hedge, at best. Furthermore, while most of the variables frequently switch from one regime to another and have higher transition probabilities, Bitcoin’s transition probability is very similar to Gold. We also found that Bitcoin and Gold both largely tend to stay in a bullish regime with a considerably lower probability of transition to a bearish regime. While these results indicate that Bitcoin may offer some hedging to diversification potential in global portfolio investments, it must be viewed with caution due to the speculative component in Bitcoin which can cause excessive volatility in this evolving asset class.
Nevertheless, it must be noted that our results are based on a short sample period; and it will be worth examining how Bitcoin is related to yet other financial and real variables. Future research could also examine whether derivatives trading has affected the volatility and maturity of Bitcoin for larger sample periods. This could provide further insights into whether the diversification potential still exists in Bitcoin, or whether it tends to diminish in the long run. The results of this study could be further extended by examining the dynamics of bitcoin with other altcoins during the turbulent market conditions to examine its safe-haven/hedging potential. It would also be interesting to see how bitcoin differs from conventional safe-haven assets for different asset classes and portfolios by conducting event studies during turbulent market conditions. The recent research in the area of cryptocurrencies has established that the prominence and popularity of bitcoin are decreasing fast leading way to altcoins and Non-fungible tokens (NFTs) like Ethereum, Litecoin, Ripple, etc., it would, therefore, be interesting to study the evolutionary direction of the diversification and hedging dynamics of cryptocurrencies in the evolving NFT and metaverse landscape.
CRediT authorship contribution statement
All authors contributed equally in carrying out the study.
Disclosure statement
No potential conflict of interest was reported by the authors.
Notes
1 Reuters 2021
2 Forbes 2021
6 Forbes 2021
7 Data accessed from https://coinmarketcap.com/ on August 28th, 2021.
8 Forbes 2018
References
- Bouri, E., Gupta, R., & Roubaud, D. (2019). Herding behaviour in cryptocurrencies. Finance Research Letters, 29, 216–221. https://doi.org/10.1016/j.frl.2018.07.008
- Baillie, R. T. (1996). Long memory processes and fractional integration in econometrics. Journal of Econometrics, 73(1), 5–59. https://doi.org/10.1016/0304-4076(95)01732-1
- Bakry, W., Rashid, A., Al-Mohamad, S., & El-Kanj, N. (2021). Bitcoin and portfolio diversification: A portfolio optimization approach. Journal of Risk and Financial Management, 14(7), 282. https://doi.org/10.3390/jrfm14070282
- Barnett, W. A., Barnett, W. A., Kirman, A. P., & Salmon, M. (Eds.). (1996). Nonlinear dynamics and economics: Proceedings of the Tenth International Symposium in Economic Theory and Econometrics (Vol. 10). Cambridge University Press.
- Baur, D. G., & Lucey, B. M. (2010). Is gold a hedge or a safe haven? An analysis of stocks, bonds and gold. Financial Review, 45(2), 217–229. https://doi.org/10.1111/j.1540-6288.2010.00244.x
- Baur, D. G., Hong, K., & Lee, A. D. (2018). Bitcoin: Medium of exchange or speculative assets? Journal of International Financial Markets, Institutions and Money, 54, 177–189. https://doi.org/10.1016/j.intfin.2017.12.004
- Bierens, H. J. (1997). Testing the unit root with drift hypothesis against nonlinear trend stationarity, with an application to the US price level and interest rate. Journal of Econometrics, 81(1), 29–64. https://doi.org/10.1016/S0304-4076(97)00033-X
- Bisaglia, L., & Gerolimetto, M. (2014). Testing for (non) linearity in economic time series: A Monte Carlo comparison. Working Paper Series, Department of Statistical Sciences, pp. 1–24.
- Bonneau, J., Miller, A., Clark, J., Narayanan, A., Kroll, J. A., & Felten, E. W. (2015). Sok: Research perspectives and challenges for Bitcoin and cryptocurrencies. In 2015 IEEE Symposium on Security and Privacy (pp. 104–121). IEEE.
- Bouoiyour, J., & Selmi, R. (2014). What does crypto-currency look like? Gaining insight into Bitcoin phenomenon. MPRA Paper No. 58133. https://mpra.ub.uni-muenchen.de/id/eprint/58133
- Bouoiyour, J., Selmi, R., & Tiwari, A. (2014). Is Bitcoin business income or speculative bubble? Unconditional vs. conditional frequency domain analysis. MPRA Working Paper 59595. http://mpra.ub.uni-muenchen.de/59595
- Bouri, E., Gupta, R., Lau, C. K. M., Roubaud, D., & Wang, S. (2018). Bitcoin and global financial stress: A copula-based approach to dependence and causality in the quantiles. The Quarterly Review of Economics and Finance, 69, 297–307. https://doi.org/10.1016/j.qref.2018.04.003
- Bouri, E., Gupta, R., Tiwari, A. K., & Roubaud, D. (2017c). Does Bitcoin hedge global uncertainty? Evidence from wavelet-based quantile-in-quantile regressions. Finance Research Letters, 23, 87–95. https://doi.org/10.1016/j.frl.2017.02.009
- Bouri, E., Jalkh, N., Molnár, P., & Roubaud, D. (2017b). Bitcoin for energy commodities before and after the December 2013 crash: Diversifier, hedge or safe haven? Applied Economics, 49(50), 1–11. https://doi.org/10.1080/00036846.2017.1299102
- Bouri, E., Molnár, P., Azzi, G., Roubaud, D., & Hagfors, L. I. (2017a). On the hedge and safe haven properties of Bitcoin: Is it really more than a diversifier? Finance Research Letters, 20, 192–198. https://doi.org/10.1016/j.frl.2016.09.025
- Brock, W. A., & Sayers, C. L. (1988). Is the business cycle characterized by deterministic chaos? Journal of Monetary Economics, 22(1), 71–90. https://doi.org/10.1016/0304-3932(88)90170-5
- Brock, W., Dechert, W. D., & Scheinkman, J. (1987). A test for independence based on the correlation dimension. Economics Working Paper SSRI-8702. University of Wisconsin.
- Broock, W. A., Scheinkman, J. A., Dechert, W. D., & LeBaron, B. (1996). A test for independence based on the correlation dimension. Econometric Reviews, 15(3), 197–235. https://doi.org/10.1080/07474939608800353
- Catania, L., & Grassi, S. (2017). Modelling crypto-currencies financial time-series. https://ssrn.com/abstract=3028486 accessed on 21/03/2019.
- Chan, W. H., Le, M., & Wu, Y. W. (2019). Holding Bitcoin longer: The dynamic hedging abilities of Bitcoin. The Quarterly Review of Economics and Finance, 71, 107–113. https://doi.org/10.1016/j.qref.2018.07.004
- Charfeddine, L., Benlagha, N., & Maouchi, Y. (2020). Investigating the dynamic relationship between cryptocurrencies and conventional assets: Implications for financial investors. Economic Modelling, 85, 198–217. https://doi.org/10.1016/j.econmod.2019.05.016
- Cheah, E. T., & Fry, J. (2015). Speculative bubbles in Bitcoin markets? An empirical investigation into the fundamental value of Bitcoin. Economics Letters, 130, 32–36. https://doi.org/10.1016/j.econlet.2015.02.029
- Chemkha, R., BenSaïda, A., Ghorbel, A., & Tayachi, T. (2021). Hedge and safe haven properties during COVID-19: Evidence from Bitcoin and gold. The Quarterly Review of Economics and Finance, 82, 71–85. https://doi.org/10.1016/j.qref.2021.07.006
- Chkili, W., Rejeb, A. B., & Arfaoui, M. (2021). Does bitcoin provide hedge to Islamic stock markets for pre-and during COVID-19 outbreak? A comparative analysis with gold. Resources Policy, 74, 102407. https://doi.org/10.1016/j.resourpol.2021.102407
- Choi, S., & Shin, J. (2021). Bitcoin: An inflation hedge but not a safe haven. Finance Research Letters, 46, 102379.
- Ciaian, P., Rajcaniova, M., & Kancs, D. A. (2016). The economics of Bitcoin price formation. Applied Economics, 48(19), 1799–1815.
- Conlon, T., & McGee, R. (2020). Safe haven or risky hazard? Bitcoin during the COVID-19 bear market. Finance Research Letters, 35, 101607. https://doi.org/10.1016/j.frl.2020.101607
- Conrad, C., Custovic, A., & Ghysels, E. (2018). Long-and short-term cryptocurrency volatility components: A GARCH-MIDAS analysis. Journal of Risk and Financial Management, 11(2), 23–12. https://doi.org/10.3390/jrfm11020023
- Corbet, S., Larkin, C., & Lucey, B. (2020). The contagion effects of the COVID-19 pandemic: Evidence from gold and cryptocurrencies. Finance Research Letters, 35, 101554. https://doi.org/10.1016/j.frl.2020.101554
- Corbet, S., Meegan, A., Larkin, C., Lucey, B., & Yarovaya, L. (2018). Exploring the dynamic relationships between cryptocurrencies and other financial assets. Economics Letters, 165, 28–34. https://doi.org/10.1016/j.econlet.2018.01.004
- Das, D., Le Roux, C. L., Jana, R. K., & Dutta, A. (2020). Does Bitcoin hedge crude oil implied volatility and structural shocks? A comparison with gold, commodity and the US Dollar. Finance Research Letters, 36, 101335. https://doi.org/10.1016/j.frl.2019.101335
- Dehouche, N. (2022). Revisiting the volatility of bitcoin with approximate entropy. Cogent Economics & Finance, 10(1), 2013588. https://doi.org/10.1080/23322039.2021.2013588
- Dyhrberg, A. H. (2016a). Bitcoin, gold and the dollar–A GARCH volatility analysis. Finance Research Letters, 16, 85–92. https://doi.org/10.1016/j.frl.2015.10.008
- Dyhrberg, A. H. (2016b). Hedging capabilities of Bitcoin. Is it the virtual gold? Finance Research Letters, 16, 139–144. https://doi.org/10.1016/j.frl.2015.10.025
- ElBahrawy, A., Alessandretti, L., Kandler, A., Pastor-Satorras, R., & Baronchelli, A. (2017). Evolutionary dynamics of the cryptocurrency market. Royal Society Open Science, 4(11), 170623.
- Gandal, N., & Halaburda, H. (2016). Can we predict the winner in a market with network effects? Competition in cryptocurrency market. Games, 7(3), 16. https://doi.org/10.3390/g7030016
- Gantori, S., Donovan, P.Ganesh, K.DeMichiel, M.Dennean, K.Trussardi, F., & Klien, M. (2017). Cryptocurrencies Beneath the Bubble. UBS. https://www.ubs.com/content/dam/WealthManagementAmericas/cio-impact/cryptocurrencies.pdf
- Gil-Alana, L. A., & Robinson, P. M. (1997). Testing of unit root and other nonstationary hypotheses in macroeconomic time series. Journal of Econometrics, 80(2), 241–268. https://doi.org/10.1016/S0304-4076(97)00038-9
- Glaser, F., Zimmermann, K., Haferkorn, M., Weber, M. C., & Siering, M. (2014, April 15). Bitcoin-asset or currency? Revealing users’ hidden intentions. ECIS.
- Goodell, J. W., & Goutte, S. (2021). Diversifying equity with cryptocurrencies during COVID-19. International Review of Financial Analysis, 76, 101781. https://doi.org/10.1016/j.irfa.2021.101781
- Granger, C. W. (1980). Long memory relationships and the aggregation of dynamic models. Journal of Econometrics, 14(2), 227–238. https://doi.org/10.1016/0304-4076(80)90092-5
- Granger, C. W., & Joyeux, R. (1980). An introduction to long‐memory time series models and fractional differencing. Journal of Time Series Analysis, 1(1), 15–29. https://doi.org/10.1111/j.1467-9892.1980.tb00297.x
- Hamilton, J. D. (1989). A new approach to the economic analysis of nonstationary time series and the business cycle. Econometrica, 57(2), 357–384. https://doi.org/10.2307/1912559
- Harwick, C. (2016). Cryptocurrency and the problem of intermediation. The Independent Review, 20(4), 569–588.
- Hernandez, J. A., Hasan, M. Z., & McIver, R. P. (2022). Bitcoin, gold, and the VIX: Short-and long-term effects of economic policy uncertainty. Applied Economics Letters, 1–5.
- Hosking, J. R. M. (1981). Fractional differencing. Biometrika, 68(1), 165–176. https://doi.org/10.1093/biomet/68.1.165
- Hsieh, D., & LeBaron, B. (1988). Small sample properties of the BDS statistic, I. Graduate school of business. University of Chicago.
- Huang, W. (2019). The impact on people’s holding intention of bitcoin by their perceived risk and valuec. Economic Research-Ekonomska Istraživanja, 32(1), 3570–3585. https://doi.org/10.1080/1331677X.2019.1667257
- Iwamura, M., Kitamura, Y., Matsumoto, T., & Saito, K. (2019). Can we stabilize the price of a Cryptocurrency?: Understanding the design of Bitcoin and its potential to compete with Central Bank money. Hitotsubashi Journal of Economics, 60(1), 41–60.
- Jareño, F., de la, O., González, M., Tolentino, M., & Sierra, K. (2020). Bitcoin and Gold price returns: A quantile regression and NARDL analysis. Resources Policy, 67, 101666. https://doi.org/10.1016/j.resourpol.2020.101666
- Jiang, S., Li, Y., Lu, Q., Wang, S., & Wei, Y. (2022). Volatility communicator or receiver? Investigating volatility spillover mechanisms among Bitcoin and other financial markets. Research in International Business and Finance, 59, 101543. https://doi.org/10.1016/j.ribaf.2021.101543
- Kapetanios, G. (2005). Unit‐root testing against the alternative hypothesis of up to m structural breaks. Journal of Time Series Analysis, 26(1), 123–133. https://doi.org/10.1111/j.1467-9892.2005.00393.x
- Kapetanios, G., & Shin, Y. (2008). GLS detrending-based unit root tests in nonlinear STAR and SETAR models. Economics Letters, 100(3), 377–380. https://doi.org/10.1016/j.econlet.2008.02.033
- Kapetanios, G., Shin, Y., & Snell, A. (2003). Testing for a unit root in the nonlinear STAR framework. Journal of Econometrics, 112(2), 359–379. https://doi.org/10.1016/S0304-4076(02)00202-6
- Karamti, C., & Belhassine, O. (2021). COVID-19 pandemic waves and global financial markets: Evidence from wavelet coherence analysis. Finance Research Letters, 45, 1–9, 102136.
- Klein, T. (2017). Dynamic correlation of precious metals and flight-to-quality in developed markets. Finance Research Letters, 23, 283–290. https://doi.org/10.1016/j.frl.2017.05.002
- Klein, T., Thu, H. P., & Walther, T. (2018). Bitcoin is not the New Gold–A comparison of volatility, correlation, and portfolio performance. International Review of Financial Analysis, 59, 105–116. https://doi.org/10.1016/j.irfa.2018.07.010
- Kristoufek, L. (2013). Bitcoin meets Google Trends and Wikipedia: Quantifying the relationship between phenomena of the Internet era. Scientific Reports, 3, 3415. https://doi.org/10.1038/srep03415
- Kristoufek, L. (2015). What are the main drivers of the Bitcoin price? Evidence from wavelet coherence analysis. PloS One, 10(4), e0123923. https://doi.org/10.1371/journal.pone.0123923
- Kuan, C. M. (2002). Lecture on the Markov switching model. Institute of Economics Academia Sinica, 8(15), 1–30.
- Lee, A. D., Li, M., & Zheng, H. (2020). Bitcoin: Speculative asset or innovative technology? Journal of International Financial Markets, Institutions and Money, 67, 101209. https://doi.org/10.1016/j.intfin.2020.101209
- Lewis, N. (2017). What is the fundamental value of bitcoin? Retrieved February 2, 2019, from. https://www.forbes.com/sites/nathanlewis/2017/12/07/what-is-the-fundamental-value-ofBitcoin/2/#123d85343c08
- Leybourne, S., Newbold, P., & Vougas, D. (1998). Unit roots and smooth transitions. Journal of Time Series Analysis, 19(1), 83–97. https://doi.org/10.1111/1467-9892.00078
- Liu, K., Chen, Y., & Zhang, X. (2017). An evaluation of ARFIMA (autoregressive fractional integral moving average) programs. Axioms, 6(4), 16. https://doi.org/10.3390/axioms6020016
- Lowe, P. (2017, December 13). An eAUD? Address to the 2017 Australian Payment Summit Sydney. Reserve Bank of Australia. https://www.rba.gov.au/speeches/2017/sp-gov-2017-12-13.html
- Luis, P., de la Fuente, G., & Perote, J. (2019). The drivers of Bitcoin demand: A short and long-run analysis. International Review of Financial Analysis, 62, 21–34.
- Lumsdaine, R. L., & Papell, D. H. (1997). Multiple trend breaks and the unit-root hypothesis. Review of Economics and Statistics, 79(2), 212–218. https://doi.org/10.1162/003465397556791
- Ma, D., & Tanizaki, H. (2022). Intraday patterns of price clustering in Bitcoin. Financial Innovation, 8(1), 1–25. https://doi.org/10.1186/s40854-021-00307-4
- Mokni, K., Bouri, E., Ajmi, A. N., & Vo, X. V. (2021). Does bitcoin hedge categorical economic uncertainty? A quantile analysis. SAGE Open, 11(2), 215824402110163. https://doi.org/10.1177/21582440211016377
- Nakamoto, S. (2008). Bitcoin: A peer-to-peer electronic cash system. https://Bitcoin.org/Bitcoin.pdf
- Ngene, G., Post, J., & Mungai, A. (2018). Volatility and shock interactions and risk management implications: Evidence from the US and frontier markets. Emerging Markets Review, 37, 181–198.
- Nguyen, T., de Bodisco, C., & Thaver, R. (2018). Factors affecting Bitcoin price in the cryptocurrency market: An empirical study. International Journal of Business & Economics Perspectives, 13(1), 106–126.
- Obeng, C. (2021). Measuring value at risk using GARCH model - evidence from the cryptocurrency market. International Journal of Entrepreneurial Knowledge, 9(2), 63–84. https://doi.org/10.37335/ijek.v9i2.133
- Pal, D., & Mitra, S. K. (2019). Hedging Bitcoin with other financial assets. Finance Research Letters, 30, 30–36. https://doi.org/10.1016/j.frl.2019.03.034
- Palombizio, E., & Morris, I. (2012). Forecasting exchange rates using leading economic indicators. Open Access Scientific Reports, 1, 402. https://doi.org/10.4172/scientificreports.402
- Park, B. J. (2022). The COVID-19 pandemic, volatility, and trading behavior in the bitcoin futures market. Research in International Business and Finance, 59, 101519. https://doi.org/10.1016/j.ribaf.2021.101519
- Perron, P. (1989). The great crash, the oil price shock, and the unit root hypothesis. Econometrica, 57(6), 1361–1401. https://doi.org/10.2307/1913712
- Raskin, M., & Yermack, D. (2016). Digital currencies, decentralized ledgers, and the future of central banking (No. w22238). National Bureau of Economic Research.
- Raymaekers, W. (2015). Cryptocurrency Bitcoin: Disruption, challenges and opportunities. Journal of Payments Strategy & Systems, 9(1), 30–46.
- Rogoff, K. (2018, December 10). Cryptocurrencies are like lottery tickets that might pay off in future. The Guardian. https://www.theguardian.com/business/2018/dec/10/cryptocurrencies-Bitcoin-kenneth-rogoff
- Sahoo, P. K. (2017). Bitcoin as digital money: Its growth and future sustainability. Theoretical & Applied Economics, 24(4), 53–64.
- Samah, H., Wajdi, M., & Regaïeg, R. (2018). Dynamic linkages among Bitcoin, Gold prices and exchange rates of US Dollar in JPY, GBP and CNY: DCC EGARCH approach. Journal of Academic Research in Economics, 10(2), 239–247.
- Schuh, S., & Shy, O. (2016). US consumers’ adoption and use of Bitcoin and other virtual currencies. In DeNederlandsche Bank, Conference Entitled “Retail Payments: Mapping Out the Road Ahead.
- Shahzad, S. J. H., Bouri, E., Rehman, M. U., & Roubaud, D. (2022). The hedge asset for BRICS stock markets: Bitcoin, gold or VIX. The World Economy, 45(1), 292–316. https://doi.org/10.1111/twec.13138
- Shahzad, S. J. H., Bouri, E., Roubaud, D., & Kristoufek, L. (2020). Safe haven, hedge and diversification for G7 stock markets: Gold versus Bitcoin. Economic Modelling, 87, 212–224. https://doi.org/10.1016/j.econmod.2019.07.023
- Shahzad, S. J. H., Bouri, E., Roubaud, D., Kristoufek, L., & Lucey, B. (2019). Is Bitcoin a better safe-haven investment than Gold and commodities? International Review of Financial Analysis, 63, 322–330. https://doi.org/10.1016/j.irfa.2019.01.002
- Sheng, H., Chen, Y., & Qiu, T. (2011). Fractional processes and fractional-order signal processing: Techniques and applications. Springer Science & Business Media.
- Sontakke, K. A., & Ghaisas, A. (2017). Cryptocurrencies: A developing asset class. International Journal of Business Insights & Transformation, 10(2), 10–17.
- Su, C. W., Xi, Y., Tao, R., & Umar, M. (2022). Can Bitcoin be a safe haven in fear sentiment? Technological and Economic Development of Economy, 28(2), 268–289. https://doi.org/10.3846/tede.2022.15502
- Symitsi, E., & Chalvatzis, K. J. (2019). The economic value of Bitcoin: A portfolio analysis of currencies, Gold, oil and stocks. Research in International Business and Finance, 48, 97–110. https://doi.org/10.1016/j.ribaf.2018.12.001
- Tiwari, N. (2018). The commodification of cryptocurrency. Michigan Law Review, 117(3), 611. https://doi.org/10.36644/mlr.117.3.commodification
- Tschorsch, F., & Scheuermann, B. (2016). Bitcoin and beyond: A technical survey on decentralized digital currencies. IEEE Communications Surveys & Tutorials, 18(3), 2084–2123. https://doi.org/10.1109/COMST.2016.2535718
- Yelowitz, A., & Wilson, M. (2015). Characteristics of Bitcoin users: An analysis of Google search data. Applied Economics Letters, 22(13), 1030–1036. https://doi.org/10.1080/13504851.2014.995359
- Yermack, D. (2014). Is Bitcoin a real currency? An economic appraisal. In D. Lee Kuo Chuen (Ed.), The handbook of digital currency (pp. 31–44). Elsevier.
- Yin, L., Nie, J., & Han, L. (2021). Understanding cryptocurrency volatility: The role of oil market shocks. International Review of Economics & Finance, 72, 233–253. https://doi.org/10.1016/j.iref.2020.11.013
- Zivot, E., & Andrews, D. W. K. (1992). Further evidence on the great crash, the oil-price shock, and the unit-root hypothesis. Journal of Business & Economic Statistics, 10(3), 251–270. https://doi.org/10.2307/1391541