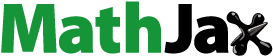
Abstract
The study analyzes the dynamic influences of composite fiscal decentralization index (CFD), human development, and research and development (R&D) expenditures on green innovations in G7 countries from 1990 to 2018. For empirical estimation, the study applies the cross-section autoregressive distributed lag method to resolve the issues of cross-section dependency and slope heterogeneity in the panel data. The results exhibit that CFD, human capital development, and R&D spending encourage green technologies in the long run. The short-run findings are also compatible with the long-run; however, their magnitude is smaller than the long-run except for CFD. In addition, the error correction term also indicates a negative and significant coefficient value, endorsing the conversion towards the long-run equilibrium position with a 25.3% annual adjustment rate in case of any shock in the short run. The robustness of the estimates is confirmed through the augmented mean group and common correlated effect mean group. These findings recommend that G7 countries should encourage human resources and R&D expenditures through education and renewable energy investment, respectively. In addition, local governments’ allocation of resources to promote green technologies must be monitored and regulated by a strong institutional framework.
JEL CODES:
1. Introduction
Over the years, the world has been facing the significant threat of environmental degradation, which raises the attention of policymakers and governments toward green growth. Achieving sustainable economic growth is the objective of every economy, and it is possible with the encouragement of green technologies. Green innovation is considered the best technological strategy for improving green growth and maintaining high environmental regulation (Wang et al., Citation2022; Liu et al., Citation2022; Ozturk et al., Citation2022). Climate-friendly innovation stands for technology that saves natural resources and decreases the harmful impacts of human-related activities through the gain in energy efficiency. These technologies include hybrid electric motors, renewable and clean energy sources, waste disposal management, circular economy, low-carbon pollution technology, and battery storage (Razzaq et al., Citation2021; Xuefeng et al., Citation2022; Ozturk & Ullah, Citation2022). Thus, there is a need to explore the factors that help stimulate ecological technologies.
Human capital is crucial in boosting green production through energy saving as it is a substantial source of knowledge accumulation and technical innovation. Human resource comprises not just human knowledge, degrees of education and training, labor skills, strong behavioral habits, and physical and mental health (Huang et al., Citation2021). Many studies have discussed the positive influence of human resources on eco-friendly innovation (Scarpellini et al., Citation2017; Ang et al., Citation2011), in which authors explained how managers’ and employees’ tangible and intangible skills, knowledge, and abilities improve eco-innovative entrepreneurship and green product design. Additionally, sustainable human capital can proactively develop new ideas and transform them into environmentally friendly solutions (Aboelmaged & Hashem, Citation2019; Khan & Ozturk, Citation2021).
Skilled human capital has a potential for absorptive capacity for green innovation because it encourages technology accumulation in businesses that improve energy efficiency, optimizes the allocation of energy resources under the energy market mechanism, and positively affects clean production. More educated people have an awareness of the benefits of green products. Thus, they consume eco-friendly goods for their livelihood. In addition, human capital is integrated with the industrial structure in industries, which boosts technical advancement in energy efficiency. However, a few studies found that human resources have less and even no influence on technology and innovation (Danquah & Amankwah-Amoah, Citation2017; Seeck & Diehl, Citation2017). Lack of investment in education and training does not improve human resources’ knowledge, abilities and skills. Thus, low and unskilled labor forces do not contribute to the technical assistance in making more productive green products and applications.
Besides human resources, R&D expenditure is essential for acquiring technological progress in a nation. Several studies have investigated the positive nexus between R&D investment and green technology (Orlando et al., Citation2022; Lee et al., Citation2015). Higher research and development in renewable energy not only support cleaner energy but also expands employment possibilities and support the growth of new industries that use eco-friendly materials and the latest technology called nanotechnology in their production process. Moreover, through R&D, there is a collaboration among different institutions, including academia, government, and industry, which help in sharing ideas and knowledge related to clean technologies. It is necessary to support R&D to increase energy efficiency and lower costs; thus, R&D is crucial to maximize investment return and search for new technological advancements. Therefore, public R&D in renewable energy is crucial since it influences future innovation processes and produces knowledge spillover (Irfan et al., Citation2022).
Additionally, research and development in renewable energy promotes competition among various energy technologies and guarantees the delivery of more sustainable and cleaner energy. Similarly, the increased innovation can help reduce the usage of fossil fuels and promote the use of cleaner energy sources like renewable energy. Moving the economy away from conventional energy-intensive production processes to ones that consume less energy and thus gain efficiency in energy usage (Su et al., Citation2021). However, the nonlinear association is explored in a study by (Hammar & Belarbi, Citation2021), who found that the effects of R&D on innovation are mixed due to different economic conditions and development. Hence, the spillover effects of R&D expenses on technology depend on the income level. Therefore, empirical studies show unclear results about concerning factors.
Along with human capital and R&D spending, fiscal decentralization is another determinant that plays an imperative role in advancing the level of green energy. Fiscal decentralization refers to the transfer of authority over the amount of income and expenditures from the central government to lower administrative units (provincial or municipal governments) to enhance public service delivery (Oates, Citation2008). In general, local government performance efficiency, inclusive growth, and better equity are benefits of this resource distribution at the lowest administrative level and revenue generation.
Prior empirical studies examined the positive relationship between fiscal decentralization and ecological technology (Chi et al., Citation2021; Acemoglu et al., 2007), which explained that the local government has some advantages in managing regional growth. For instance, the local government is better positioned to gather information about citizen preferences for green development because of its accessibility to service receivers. It also has the flexibility to act quickly in response to residents’ expanding requirements. Moreover, regional governments have greater authority and autonomy over the revenues and expenditures, which create competition and accountability regarding the facilitation and regulation of green energy services among these small administrative units. These features of provincial governments increase the resource efficiency gains in ecological energy. Thus, they allocate more investments toward renewable energy sources, green R&D projects, low-carbon capture technology, and ecological-related technologies.
Few studies argued that fiscal decentralization does not play a substantial role in providing sufficient technological goods and services (Chen et al., Citation2018; Song et al., Citation2018b). The close connection of political leaders in regions and greater authority reduces the positive gains of decentralization in the presence of corruption and a weak monitoring and institutional framework, which arises in inefficiencies. Moreover, the local government’s incentives are sometimes more interested in developing and infrastructure-related projects to increase regional growth. It reduces the attention on investment in green-related projects, which decreases the advancement in renewable technology. Therefore, there are mixed results regarding the association between the concerned variables.
Although many past research studies used either revenue decentralization (RD) or expenditure decentralization (ED) as a proxy for representing the influence of fiscal decentralization, they cannot analyze the full effect of fiscal decentralization. Thus, these single variables produce biased results due to their partial impacts. The balance of RD and ED is essential to produce efficiency gains in resource allocation from fiscal decentralization at the regional level (Lingyan et al., Citation2021). Unlike the previous studies, this article constructs a composite index of fiscal decentralization (CFD) by following the method of Martinez-Vazquez and Timofeev that includes both ED and RD features (Martinez-Vazquez & McNab, Citation2003).Thus, CFD is a multidimensional and reliable measure for showing the local government’s performance and efficiency in revenue generation and expenditure spending. In addition, most studies about the linkage between human capital and innovation are based on firm-level and country-level data. Thus, less attention has been given to the panel data for analyzing the relationship between these two concerning factors. Similarly, many empirical studies explore the association between R&D spending and technology or innovation in the literature. Very few studies are found the impact of R&D investment on green innovation. For the empirical analysis, G7 countries are chosen because they are highly decentralized economies. Moreover, these countries are advanced at the technological level due to having skilled human resources and increased R&D activities. Also, these countries are engaged in the development and innovation of clean or renewable energy for maintaining sustainable growth.
The study aims to determine the dynamic impacts of composite fiscal decentralization, human capital, and R&D expenditures on eco-friendly innovation in G7 nations by using the data from 1990 to 2018. Moreover, the study employed a multidimensional fiscal decentralization index (CFD) that incorporates the elements of RD and ED into a single cumulative index. CFD is a reliable measure to analyze the effects of decentralization suggested by Martinez-Vazquez and Timofeev’s approach. In addition, a cross-sectional autoregressive distributed lag (CS-ARDL) estimator is applied for the empirical analysis of panel data. Since CS-ARDL is superior to the other panel data estimators (POLS, FMOLS, PARDL) in the presence of cross-section dependency and slope heterogeneity, therefore, this method produces consistent results to ascertain the dynamic short and long-run relationship of the variables in the model. Finally, Augmented mean group (AMG) and common correlated effect mean group (CCEMG) approaches are used to confirm the robustness of the results estimated in the model from the CS-ARDL method.
The structure of the remaining part of this article is as follows: the literature review is presented in Section 2, followed by data and methodology in Section 3. Section 4 describes the results and discussion, and Section 5 shows the conclusion and policy implications.
2. Literature review
2.1. Human capital and green innovation
Human capital is the labor force’s accumulation of knowledge and skills from investments in health care, education, training, experience, and migration. Similarly, sustainable human capital can indicate a key capacity expressed in an employee’s behaviors, attitudes, skills, experience, commitments, and environmental knowledge (Huang and Kung, 2011). Human capital sustainability may also be a strategic instrument for the adoption of green innovation. Several studies found that human resource positively and significantly affects technological and product innovation. Scarpellini et al. (Citation2017) analyzed the impact of human capital in eight eco-innovative firms in Spain through qualitative comparative analysis (QCA). The study concludes that human capital is the most relevant factor in developing eco-innovation. Companies committing specialized human capital to eco-innovation activities are at least ten years old. Moreover, firms with R&D and innovation departments and a separate department for environmental management have been certified through some environmental certification standards. In addition, they have human resources dedicated to product design, support entrepreneurship for innovation among their staff, and are dependent on government funds.
Using the panel data of 28 manufacturing sectors in China from 2003 to 2014, Yuan and Zhang (Citation2017) determined that HC can boost green production through energy-saving and technical innovation because it is an important source of knowledge accumulation and technological innovation. Ang et al. (Citation2011) investigated the impact of human capital on innovation by utilizing the panel data of 87 countries from 1970 to 2004. The results conclude that when high and middle-income countries get closer to the technological frontier, the enhancing impacts of higher education attainment or skilled human capital increase. In other words, economies focusing more on innovation than imitation and investing more in higher education can increase TFP growth when their technology gap narrows. Wei et al. (Citation2011) conducted the study using the sample data of 223 Chinese firms to investigate the relation of human resources with product innovation. The findings indicate the positive effect of human capital on product innovation with development and firm structure. Jiménez-Jiménez and Sanz-Valle, (Citation2008) used data from 173 Spanish firms to evaluate the association between human resources and innovation. The results show that innovation is direct positively influenced by human resources. Verburg et al. (Citation2007) utilized sample data from 175 companies that the strategies of human resource management that focus on promoting a commitment culture rather than compliance have a favorable impact on a company’s innovative approach.
However, in a review of previous studies on the nexus between innovation and human capital, a study by Seeck and Diehl (Citation2017) found that compared to product and technological innovation, human resource has less influence on administrative and process innovation. Similarly, Danquah and Amankwah-Amoah (Citation2017) used the data from nineteen Sub-Saharan countries to find the role of human capital on innovation, technology adoption (efficiency change) and productivity growth from 1960 to 2003. The study’s empirical results reveal that human capital does not significantly impact innovation and productivity growth while positively affecting technological adoption. Therefore, the earlier empirical studies have provided inconclusive results on the linkage between human capital and technological innovation.
2.2. R&D and green technology
Scholars generally agreed about the widespread advantages of R&D expenditures in improving technology and innovation. Many research studies have examined that R&D is another important factor in introducing green innovation at the firm and country levels. Castellacci and Lie (Citation2017) explored that green innovations in the carbon dioxide and waste reduction sectors in South Korea are driven mainly by internal R&D competence and strong relationships with public research organizations and universities. Horbach (Citation2016) stated that internal expenditures of R&D are critical for environmentally beneficial technologies in the areas of material and energy savings in the context of firms from 19 European countries. Jaumotte and Pain (Citation2005) found evidence that earlier R&D and patenting activities, as well as increased collaboration between enterprises and government research organizations and universities, are major drivers of green inventions in a cross-country study. Cainelli et al. (Citation2015) used a panel dataset of Italian enterprises from 2005 to 2010 to conclude that R&D activity, collaboration with suppliers and universities, and adoption of external knowledge and equipment are more important for green innovations than for other innovations.
Lee et al. (Citation2015) analyzed the positive and significant correlation between the R&D factors and information technology innovation in 40 countries from 1999 to 2013. The study suggests that acquiring knowledge capital through R&D encourages ICT innovation and industry competitiveness because R&D can enhance triple-helical collaboration for ICT innovation among academia, industry, and government. Ramadani et al. (Citation2017) studied Balkan countries from 2013-2014. The study discovered that the R&D investment coefficient is statistically significant and positive. Businesses that invest in R&D are 38% more likely than those that do not engage in innovation-related activities. Orlando et al. (Citation2022) examined the influence of R&D investment on green technology in 28 European members from 2008 to 2017. The findings highlight that government spending on R&D positively impacts the eco-innovation index more than the firm’s spending. In addition, there has also been found that R&D spending and innovations have a nonlinear association. Hammar and Belarbi, (Citation2021) investigated the nonlinear relationship between innovation and R&D expenditures in 36 countries from 2002 to 2014. The results indicate that R&D has an inverse effect on innovation at the lower development stage while positively impacting innovation at a higher degree of development. Therefore, ambiguity exists in the nexus between R&D investment and green innovation.
2.3. Fiscal decentralization and green technology
Samuelson (Citation1954) is the pioneer who established the foundation for the new fiscal decentralization when he distributed the levels of service delivery to all individuals at a constant level, which promotes efficiency. Fiscal decentralization refers to the transfer of authority over the amount of income and expenditures to lower administrative units to enhance the delivery of public services (Oates, Citation2008). In the same way, fiscal decentralization plays a vital role in promoting and advancing the technological level of a country.
In the literature, many studies explored the effects of decentralization in enhancing environmental quality. Some studies found a positive nexus between fiscal decentralization and green innovation. Chi et al. (Citation2021) utilized the panel data of seven decentralized countries to find fiscal decentralization’s effects on innovation from 1996 to 2017. The study’s outcomes show that a positive and significant coefficient of fiscal decentralization implies that an increase in it leads to better the countries’ performance in innovation and technology. Additionally, Fagerberg & Srholec (Citation2008) studied the significance of political institutions from a macro perspective about technology development. Acemoglu et al. (2007) focused on the linkage between the decentralization of firms and the latest technologies by using the three micro-level data sets of French and British firms. The results indicate that the firms that choose decentralization are closer to technological advancements. Drezner Drezner, Drezner, (Citation2001) argued that progressive technological innovation is better suited to a decentralized governmental structure. Therefore, a decentralized governmental structure is required for nations to maintain themselves at the leading edge of innovation.
Song et al. (Citation2018a) analyzed the impact of fiscal decentralization on green total factor productivity (GTFP) in 11 provinces of China. The findings demonstrate that fiscal decentralization increases GTFP while strong fiscal decentralization reduces GTFP when the quantile level rises. Su et al., Citation2021 concluded that fiscal decentralization and environmental innovation increase the share of renewable energy consumption and reduce dependence on non-renewable sources in the OECD sample countries from 1990 to 2018. Sustainable energy alternatives were made possible by decentralization, and regional environmental taxes discourage the use of fossil or non-renewable fuels. To increase energy efficiency and the proportion of sustainable energy sources in the energy mix, Fiscal decentralization is linked to increasing R&D spending and sustainable energy solutions. The effect of fiscal decentralization on the effectiveness of public service delivery was studied by Sow and Razafimahefa (Citation2015) who discovered that, under some circumstances, decentralization could result in efficiency gain using the stochastic frontier model. First, there must be sufficient RD in addition to ED. Second, a workable institutional governance framework is a requirement for the benefits of decentralization to continue. Third, a significant level of ED is required for fiscal decentralization to produce fruitful results. Negative impacts of decentralization could result from the absence of these traits.
Elheddad et al. (Citation2020) offered a different viewpoint, showing that decentralization initially increases energy consumption in China but that over time, energy use declines as more funding is allocated for sustainable technology. Song et al. (Citation2018b) conducted a theoretical analysis of the relationship between technological advancement, environmental regulation, and industrial land-use efficiency. They discovered a negligible impact of environmental protection regulation on technological advancement based on the environment. Thus, the influences of fiscal decentralization on technology are mixed and need to explore.
From the above extensive literature, it has been discovered that most of the studies used RD and ED as the proxy of fiscal decentralization, which exhibits the partial impact of the variable. Unlike the previous studies, the current study includes CFD, which covers both aspects of RD and ED in fiscal decentralization. Moreover, most studies discussed the impact of R&D, human capital, and Fiscal decentralization at the firm level and gave less focus to the country level. Therefore, this article examines the dynamic impact of human capital, R&D expenditures, and CFD on eco-friendly technology in the context of G7 countries.
3. Data and methodology
3.1. Data sources
The data of G7 countries, namely France, Canada, Italy, German, Japan, the United States, and the United Kingdom, are selected to perform the empirical analysis, and the annual data is used from 1990 to 2018. The data of green innovation GI is measured as Eco patents % of total patents, human capital (HC) is measured as human development index, and research and development expenditures (R&D) are represented as a percentage share in total GDP. The GI and R&D data are taken from OECD statistics (Citation2021), while HC is taken from Penn World Table 10. All the data of variables are transformed into logarithms except CFD by following (Lingyan et al., Citation2021; Razzaq et al., Citation2022). The definitions, measurement units, and sources of variables are mentioned in . CFD is a multidimensional measure of fiscal decentralization, which is empirically calculated by adopting the Martinez-Vazquez and Timofeev (2009) method. It comprises revenue decentralization (RD) and expenditure decentralization (ED). While RD is expressed as a percentage of provincial government revenue to the overall federal government revenue (Oates, Citation2005)
(1)
(1)
Table 1. Variables of the study.
In EquationEquation (1)(1)
(1) , PR and FR refer to Provincial and Federal revenue.
While ED is calculated using the same methodology described above, it represents the proportion of provincial government expenditures to total federal government spending in the country (Oates, Citation2005).
(2)
(2)
In EquationEquation (2)(2)
(2) , PE and FE are provincial and federal expenditures. Thus, the formula for the composite index of fiscal decentralization (CFD) can be written below, and the range of the CFD index lies between zero and one.
(3)
(3)
3.2. Theoretical framework and model specification
The current study investigates the dynamic influence of the composite fiscal decentralization index and human capital on green innovation; thus, for this study, we follow the models of Chi et al. (Citation2021) and Sun and Razzaq, (Citation2022) and modify them in the context of high decentralized G7 economies. Thus, the model specification can be written as:
(4)
(4)
In EquationEquation (1)(1)
(1) , GI is the dependent variable, whereas CFD, HC, and R&D denote the independent variables. However,
is the intercept term, while slope coefficients are represented from
to
The cross-section identities are shown as i, and the time period is shown as t.
Following Lingyan et al. (Citation2021) and Wang et al. (Citation2022), the composite fiscal decentralization index is used as a proxy for fiscal decentralization. Fiscal decentralization is assigned to positive outcomes with eco-innovation mostly based on two theories. The first theory of decentralization argues that people who live in dispersed areas have different desires. Thus, local government, due to the closeness, has a greater understanding and information of residents in providing the public goods on their priorities and concerns. In this context, regional governments have greater technological awareness regarding green growth than the national government. Therefore, they are more efficient in allocating their regional expenditures and incomes to renewable technology and replacing conventional technology with green technology to provide a long-term viable energy supply. At the same time, the second theory of decentralization is based on community best practices that promote inter-jurisdictional competition and restrict the government’s capability to overtax residents and business firms.
Similarly, due to increasing environmental risks and their implications in their communities, local residents’ social consciousness is growing, placing pressure on governments to implement ecologically friendly technology such as renewable energy, carbon capture, and reduction technology. On the contrary, local governments typically have considerable incentives to do all possible to support their respective provinces’ rates of GDP growth. Thus they spend all the resources on growth-related infrastructure projects, which consume material and energy resources. Thus, they increase regional growth at the cost of ignoring the green energy projects and thus give less consideration to developing ecological technologies and innovations. As a result, FD can be a double-edged sword and affect innovation and technology levels depending on the government’s area of interest.
Moreover, CFD is a multidimensional aspect of RD and ED because the balance between revenue collection and expanding assignment maximizes the efficiency gains at the local level (Oates, Citation2008). Therefore, this article includes the CFD that includes both elements of ED and RD. Research and development are crucial in promoting technological advancement in the empirical literature. Thus, increased R&D expenditures improve green ecological technology by lowering energy consumption and pollution control expenses. Hence, this study expects a positive association of R&D with green innovation by following (Lee et al., Citation2015). In addition, human capital plays an essential role in upgrading technology through investing in education, training, and experiences. Thus, the long-term sustainability of human capital could be a strategic tool for promoting green innovation adoption through the skills and knowledge concerning the environment. Therefore, the study follows (Alawamleh et al., Citation2019) that human capital may positively impact environmental technology.
3.3. Econometric estimations
The whole econometric procedure of this article consists of six steps. At the initial stage of the empirical analysis of panel data, examining the cross-section dependency (CSD) in the variables is indispensable. The ignorance of CSD provides misleading results because all economies have variant economic and financial structures and social and demographic conditions. Therefore, the CSD test is applied in the study developed by (Pesaran et al., Citation2004).
The next step is to find the heterogeneity of the variables involved in the model because heterogeneity in slope coefficients yields biased results. Thus, it is essential to ascertain slope homogeneity by employing the heterogeneity test (Pesaran & Yamagata, Citation2008). After investigating the CSD and slope heterogeneity, the study finds the presence of unit root in the variables, unlike the previous empirical studies, which mostly used the first-generation unit root test. The study applied cross-section augmented IPS and Cross-sectional augmented Dickey-Fuller (CADF) second-generation unit root tests developed by Pesaran (Citation2007) that can handle the CSD and slope heterogeneity issues.
Before estimating the dynamic short and long-run relationship, the study finds the long-run cointegration among the variables using Westerlund’s (Citation2007) cointegration test. This cointegration test is appropriate compared to Kao and Pedroni and provides unbiased results on the existence of CSD and heterogeneous slopes. After examining long-run cointegration, the study estimates the short and long-run findings. For this, the study applied the cross-section autoregressive distributed lag (CS-ARDL) method, which was developed by Chudik and Pesaran (Citation2013). CS-ARDL estimators have benefits over other estimation methods, for example, PARDL, POLS, and FMOLS, because they cannot resolve the problems of CSD, heterogeneity, and endogeneity of the variables. Moreover, this estimator also handles the common and significant unobserved factors; thus, the estimation without considering these factors may produce inconsistent results (Rehman & Sohag, Citation2022). In the last step, the study checks the robustness and stability of results through the AMG and CCEMG presented by Eberhardt and Teal (Citation2010) and Pesaran (Citation2006).
4. Empirical results & discussion
4.1. CSD and slope homogeneity
The results of CSD and slope heterogeneity are presented in . The findings of the CSD test show rejection of the null hypothesis of no CSD. It means showing the presence of CSD in all the variables across the different countries at a 1% significance level. Further, the outcomes of the heterogeneity test indicate that all the slope parameters of the variables are heterogeneous, which indicates the differences in economic, social, and financial conditions of the cross-section identities included in the model.
Table 2. CSD and slope homogeneity test.
4.2. Panel unit root test
The study applied the CIPS and CADF unit root tests to examine the explanatory variables’ stationary level after confirming the existence of CSD and slope heterogeneity. Both unit root tests of CIPS and CADF are enabled to produce efficient results of the panel data in the case of CSD and heterogeneous slopes. The results are shown in , which shows that all the variables are not stationary at a level while stationary at first. Thus, there may be a long-run cointegration relationship among the variables.
Table 3. CIPS & CADF unit root tests.
4.3. Cointegration test
The existence of CSD and slope heterogeneity suggests the study uses Westerlund’s cointegration test to produce unbiased results. Thus after determining the stationary condition of the variables, the study checks the long-run cointegration association. The test findings are mentioned in , which demonstrates that all four statistics are significant and show that all the model variables are co-integrated in the long run.
Table 4. Cointegration outcomes.
4.4. Short and long-run estimations of the CS-ARDL test
The long-run relationship of all the variables allows the study to estimate the short and long-run results of the model using the CS-ARDL method. The outcomes of the long-run estimates are shown in , in which CFD is positively and significantly connected to promoting ecological green technology at a 5% significance level. It shows that a 1% increase in CFD encourages environmental technology by 0.126%. Since fiscal decentralization is a process of delegating authority and resources to the lower units, this phenomenon gives the local or provincial governments more autonomy over public funds or revenues and expenditures for regional green development. Moreover, these small administrative units are more accountable for pubic-related green projects and their performance. It would create competition among these lower units and efficiency in providing sustainable products and technologies as per the local inhabitants’ priorities.
Table 5. Cross-sectional ARDL results.
Due to the regional closeness, the local governments have the information advantage about the need for green energy projects such as installing solar panels, wind turbines, green buildings, and carbon capture technology. In this context, in the long-run, regional governments, through fiscal decentralization, foster the environment of green innovation in a country by investing more resources in renewable energy sources such as solar, tidal, wind, geothermal, or biomass energy to provide sustainable green technology. In addition, due to enough power over the resources, provincial governments replace traditional technology with green or renewable technology (Lingyan et al., Citation2021). Also, the legislative authority of the local government, because of the higher level of CFD, effectively supervises and controls the technological system for a green environment. This diminishes the energy usage and cost incurred on pollution control and thus generates positive spillover effects through promoting green efficiency. The findings of CFD are consistent with the results of (Chi et al., Citation2021; Fagerberg & Srholec, Citation2008), who found that decentralization is the best strategy to encourage technological development.
Our results are contrary to (Song et al., Citation2018b; Bahl & Bird, Citation2013), who discovered that indirect benefits of fiscal decentralization include increased infrastructure development like the construction of roads, buildings and, economic and industrial zones, etc., all of which need resource-intensive energy. Thus, rising infrastructure facilities neutralize the benefits of decentralization in terms of green efficiency. Moreover, regional governments are sometimes interested in high provincial GDP growth at the cost of environmental destruction. Therefore, less attention has been given to the advancement of green innovation and growth (Chen et al., Citation2018). In addition, local politicians’ autonomy, power and closeness raise the corruption problem, who argue that leakages and corruption are the potential demerits of decentralization (Lingyan et al., Citation2021).
Similarly, the result of the second variable, HD, is positive and significant at a 5% significance level. It exhibits that 1% increase in HD promotes the GI by 0.265% while other factors remain constant. Investing in human capital through education, training, and experiences enhances people’s skills and knowledge, directly affecting their lifestyle consumption. Thus, educated people are more likely to adopt green energy products in their consumption patterns, promoting green advancement. Similarly, as the input of the labor force, human capital with abilities and skills produces a spillover effect of knowledge and encourages the diffusion of low-carbon technology. Skillful human resource plays a role in absorptive capacity for green technologies because it promotes technology accumulation in enterprises that enhance energy efficiency, optimizes energy resources regarding the energy market mechanism, and produces beneficial effects in clean production. Moreover, during regional industrial production, human resource works with the industrial structure and, thus, efficiently uses the energy. This increased the technical progress in energy saving and improved the efficiency of technology in energy usage (Huang et al., Citation2021). This outcome is opposite to the results of (Danquah & Amankwah-Amoah, Citation2017), which found the insignificant influence of human capital on innovation because low or unskilled human capital is an obstacle in the innovation process of Sub Sharan African countries.
The third variable of the model, R&D, also has a positive and significant influence on renewable technologies, which shows that a 1% rise in R&D stimulates GI by 0.365%. Higher allocation of R&D expending on renewable energy sources and products comprises the potential to promote the quality of green technology. This investment also improves and substitutes the existing conventional technologies with clean technologies and develops new directions for green markets that produce eco-friendly materials. These advanced and improved materials provide the foundation for new industries that use the latest technology, such as nanotechnology (Lee et al., Citation2015). The industries of electric vehicles, LED products, low-carbon, green energy products (solar panels, wind turbines, bioreactors, motion sensors etc.), and recycled products have gained more attention for investment. Moreover, the R&D collaboration of universities, government and industries strengthens green development by sharing ideas and knowledge, which further helps in technological accumulation. These findings are identical to (Horbach, Citation2016) for nineteen European economies where R&D expenditures are essential for energy-saving and material use innovation. In contrast, these outcomes are opposite to those (Hammar & Belarbi, Citation2021), who argued that the impacts of R&D on innovation are based on the level of economic development.
Among the influencing factors for the advancement of green technologies, the coefficients of R&D and HD are larger in the long run, while the CFD has little impact in the long run. R&D investment in clean energy sources and HD through education potentially enhances environmental innovation. However, the CFD’s coefficient is larger in the short-run compared to the long run, which suggests that for sustainable green innovation, there is a need for more policy implications related to fiscal decentralization. Thus, the reason behind the lower impacts of CFD in the long term is that in G7 countries, Canada and United States are large geographical areas that show weak regulation control and accountability which may reduce the advantages of decentralization. Further, both economies demand heavy infrastructure facilities, and developments consume energy resources; therefore, the gains of CFD diminish in terms of high-energy costs.
The short-run estimations of the model are also mentioned in . The signs and coefficients of all the factors are similar to long-run results. Thus, CFD is positively associated with GI by 0.131%. Similarly, the other two factors, HD ad R&D, also significantly affected GI by 0.218% and 0.343%, respectively. However, the magnitude of the variable’s coefficients, except the CFD is low in the short-run compared to the long-run coefficients. It shows that the factors are more substantial and profound in the long run to promote green technologies. Therefore, the long-run policy is suggested for a sustainable technological level. In addition, the coefficient of error correction term (ECT) is 0.253, which is significantly negative at a 1% significance level. Thus, any shock in the short-run converges the model toward the steady-state equilibrium with a 25.3% speed of adjustment per annum. Thus, visualizes the outcome of estimators.
4.5. Robustness analysis
The study used AMG and CCEMG methods to check the robustness analysis, and the results of both estimators are represented in . The outcomes of CCEMG reveal that CFD, R&D, and HD increase GI by 0.98%, 0.312%, and 0.239%, respectively. Likewise, the AMG estimator reports that CFD increases GI by 0.106%, R&D by 0.338%, and HD by 26.6%. Thus, robustness tests’ outcomes confirm the CS-ARDL method’s positive findings.
Table 6. Robustness estimators.
5. Conclusion & policy recommendations
G7 economies are highly attributed to fiscal decentralization and human resources, which are essential determinants for boosting clean technology. In this regard, this study examines the dynamic effects of composite fiscal decentralization, human resources, and R&D expenses on environmental technologies in G7 countries. The study used the annual panel data from 1990 to 2018 for estimations. Firstly, CSD and slope heterogeneity tests are employed, which show that parameters are heterogeneous and CSD. Then, the study applied unit root tests of CIPS and CADF, which confirmed that all the variables are stationary at I (I) and thus integrated into order one. After conducting the unit root tests, long-run cointegration is determined by applying Westerlund’s test, which shows the existence of long-run association among the variables. The CS-ARDL method is used to estimate the short and long-run relationship.
The long-run outcomes of the CS-ARDL approach reveal that CFD, R&D, and HD are positively and significantly associated with GI and led to a change of 0.126%, 0.365%, and 0.265%, respectively. In the short run, the coefficients of all the explanatory variables are identical to long-run outcomes, having the same signs and directions of coefficients. However, the magnitude of all the variables is smaller in the short run than in the long run. Short-run estimates indicate that CFD, HD, and R&D raise GI by 0.131%, 0.218%, and 0.343%, respectively. The error correction term is negative and significant, with a value of 0.253 at a 1% significance level. Thus, in case of any deviation in the short run, the model will reach a long-run stable equilibrium with a 25.3% rate yearly. Lastly, the study confirms the findings from the CS-ARDL method by utilizing the AMG and CCEMG.
Local government should be more powerful in terms of authority and autonomy and bring institutional reforms to implement a clean and green growth agenda. Therefore, local governments should enforce environmental regulations to encourage new consumer influxes and employ technology-based environmental innovation, i.e., ecological energy, as their primary energy source. In large geographical regions of G7 countries, a proper institutional framework should be developed to monitor the local government’s financial and development activities. These measures will make the small local units more accountable and eliminate the corruption which may arise due to the self-interested priorities of regional governments and authorities.
Revenue and expenditure decentralization must balance for decentralization to be effective and should adjust the regulation and implementation of environmentally friendly requirements. To ensure environmental stewardship, RD encourages the sub-national governments to monitor their industry closely and collect carbon-related levies. In contrast, ED encourages spending on eco-friendly technologies to create dependable and long-lasting infrastructure for the economy and society. The provincial governments of G7 nations should make R&D spending on renewable energy sources, green-growth projects, and social awareness programs through public-private partnerships, which produce the spillover technology effects and thus, promote innovation-based clean and environment-friendly products. These energy-efficient and cost-saving products provide possible green solutions for sustainable economic growth. The policymakers of G7 economies should invest in sustainable human capital through education, training, and experiences to create positive knowledge-based effects, which help provide new ideas for developing new green technology-based commodities. Through education, skilled people prefer environmental-friendly goods in their consumption patterns, which boosts green production and innovations in these countries.
Lastly, this study includes only seven advanced countries for analysis, and the study should incorporate more developed and developing economies by increasing the size of the panel data. Moreover, the other influential factors should also add to estimations of the model, like green financing, financial development, trade openness and renewable energy consumption, to provide more comprehensive and meaningful policy implications for encouraging eco-friendly innovations and technologies.
Disclosure statement
No conflict of interest has been reported by the authors.
Additional information
Funding
References
- Aboelmaged, M., & Hashem, G. (2019). Absorptive capacity and green innovation adoption in SMEs: The mediating effects of sustainable organisational capabilities. Journal of Cleaner Production, 220, 853–863. https://doi.org/10.1016/j.jclepro.2019.02.150
- Acemoglu, D., Aghion, P., Lelarge, C., Van Reenen, J., & Zilibotti, F. (2007). Technology, information, and the decentralization of the firm. The Quarterly Journal of Economics, 122(4), 1759–1799.http://www.jstor.org/stable/25098888
- Alawamleh, M., Ismail, L. B., Aqeel, D., & Alawamleh, K. J. (2019). The bilateral relationship between human capital investment and innovation in Jordan. Journal of Innovation and Entrepreneurship, 8(1), 6. https://doi.org/10.1186/s13731-019-0101-3
- Ang, J. B., Madsen, J. B., & Rabiul Islam, M. (2011). The effects of human capital composition on technological convergence. Journal of Macroeconomics, 33(3), 465–476. https://doi.org/10.1016/j.jmacro.2011.03.001
- Bahl, R., & Bird, R. (2013). Decentralization and infrastructure in developing countries: Reconciling principles and practice. IMFG Papers on Municipal Finance and Governance. (Issue No. 16).
- Cainelli, G., De Marchi, V., & Grandinetti, R. (2015). Does the development of environmental innovation require different resources? Evidence from Spanish manufacturing firms. Journal of Cleaner Production, 94, 211–220. https://doi.org/10.1016/j.jclepro.2015.02.008
- Castellacci, F., & Lie, C. M. (2017). A taxonomy of green innovators: Empirical evidence from South Korea. Journal of Cleaner Production, 143, 1036–1047. https://doi.org/10.1016/j.jclepro.2016.12.016
- Chen, H., Hao, Y., Li, J., & Song, X. (2018). The impact of environmental regulation, shadow economy, and corruption on environmental quality: Theory and empirical evidence from China. Journal of Cleaner Production, 195, 200–214. https://doi.org/10.1016/j.jclepro.2018.05.206
- Chi, M., Muhammad, S., Khan, Z., Ali, S., & Li, R. Y. M. (2021). Is centralization killing innovation? The success story of technological innovation in fiscally decentralized countries. Technological Forecasting and Social Change, 168, 120731. https://doi.org/10.1016/j.techfore.2021.120731
- Chudik, A., & Pesaran, M. H. (2013). Federal Reserve Bank of Dallas Globalization and Monetary Policy Institute large panel data models with cross-sectional dependence. :153.
- Danquah, M., & Amankwah-Amoah, J. (2017). Assessing the relationships between human capital, innovation and technology adoption: Evidence from sub-Saharan Africa. Technological Forecasting and Social Change, 122, 24–33. https://doi.org/10.1016/j.techfore.2017.04.021
- Drezner, D. (2001). State structure, technological leadership and the maintenance of hegemony. Review of International Studies, 27(1), 3–25. https://doi.org/10.1017/S0260210501000031
- Eberhardt, M., & Teal, F. (2010). Department of economics discussion paper series productivity analysis in global manufacturing production productivity analysis in. Working Paper, 515, 1–32.
- Elheddad, M., Djellouli, N., Tiwari, A. K., & Hammoudeh, S. (2020). The relationship between energy consumption and fiscal decentralization and the importance of urbanization: Evidence from Chinese provinces. Journal of Environmental Management, 264, 110474. https://doi.org/10.1016/j.jenvman.2020.110474
- Fagerberg, J., & Srholec, M. (2008). National innovation systems, capabilities and economic development. Research Policy, 37(9), 1417–1435.
- Rehman, F. U., & Sohag, K. (2022). Does transport infrastructure spur export diversification and sophistication in the G-20 economies? An application of CS-ARDL. Applied Economics Letters, 1–5.
- Hammar, N., & Belarbi, Y. (2021). R&D, innovation and productivity relationships: Evidence from threshold panel model. International Journal of Innovation Studies, 5(3), 113–126. https://doi.org/10.1016/j.ijis.2021.06.002
- Pesaran, M. H., & Yamagata, T. (2008). Testing slope homogeneity in large panels. Journal of Econometrics, 142(1), 50–93. https://doi.org/10.1016/j.jeconom.2007.05.010
- Hinojosa, K. (2022). Determinants of eco-innovation in the change towards a circular economy: An empirical analysis of Spanish firms. Journal of Innovation Economics & Management, 39, 105–139. https://doi.org/10.3917/jie.pr1.0119
- Horbach, J. (2016). Empirical determinants of eco-innovation in European countries using the community innovation survey. Environmental Innovation and Societal Transitions, 19, 1–14. https://doi.org/10.1016/j.eist.2015.09.005
- Huang, C., & Kung, F. (2011). Environmental consciousness and intellectual capital management: Evidence from Taiwan’s manufacturing industry. Management Decision.
- Huang, C., Zhang, X., & Liu, K. (2021). Effects of human capital structural evolution on carbon emissions intensity in China: A dual perspective of spatial heterogeneity and nonlinear linkages. Renewable and Sustainable Energy Reviews, 135, 110258. https://doi.org/10.1016/j.rser.2020.110258
- Irfan, M., Razzaq, A., Sharif, A., & Yang, X. (2022). Influence mechanism between green finance and green innovation: Exploring regional policy intervention effects in China. Technological Forecasting and Social Change, 182, 121882. https://doi.org/10.1016/j.techfore.2022.121882
- Jaumotte, F., & Pain, N. (2005). From Ideas to Development: The Determinants of R&D and Patenting. OECD Economics Department Working Papers, No. 457, OECD Publishing. https://doi.org/10.1787/702226422387
- Jiménez-Jiménez, D., & Sanz-Valle, R. (2008). Could HRM support organizational innovation? The International Journal of Human Resource Management, 19(7), 1208–1221. https://doi.org/10.1080/09585190802109952
- Jin, C., Razzaq, A., Saleem, F., & Sinha, A. (2021). Asymmetric effects of eco-innovation and human capital development in realizing environmental sustainability in China: evidence from quantile ARDL framework. Economic Research-Ekonomska Istrazivanja, 35, 111922. https://doi.org/10.1080/1331677X.2021.2019598
- Khan, M., & Ozturk, I. (2021). Examining the direct and indirect effects of financial development on CO2 emissions for 88 developing countries. Journal of Environmental Management, 293, 112812.
- Liu, X., Razzaq, A., Shahzad, M., & Irfan, M. (2022). Technological changes, financial development and ecological consequences: A comparative study of developed and developing economies. Technological Forecasting and Social Change, 184, 122004. https://doi.org/10.1016/j.techfore.2022.122004
- Lee, S. H., Park, S., & Kim, T. (2015). Review on investment direction of green technology R&D in Korea. Renewable and Sustainable Energy Reviews, 50, 186–193. https://doi.org/10.1016/j.rser.2015.04.158
- Lingyan, M., Zhao, Z., Malik, H. A., Razzaq, A., An, H., & Hassan, M. (2021). Asymmetric impact of fiscal decentralization and environmental innovation on carbon emissions: Evidence from highly decentralized countries. Energy and Environment, 33(4), 752–782. https://doi.org/10.1177/0958305X211018453
- Danquah, M., & Ouattara, B. (2014). Productivity growth, human capital and distance to frontier in sub-Saharan Africa. Journal of Economic Development, 39(4), 27–48. https://doi.org/10.35866/caujed.2014.39.4.002
- Martinez-Vazquez, J., & McNab, R. M. (2003). Fiscal decentralization and economic growth. World Development, 31(9), 1597–1616. https://doi.org/10.1016/S0305-750X(03)00109-8
- Moyar, D. (2017). The oxford handbook of hegel. In the Oxford Handbook of Hegel. https://doi.org/10.1093/oxfordhb/9780199355228.001.0001
- Oates, W. E. (2005). Toward a second-generation theory of fiscal federalism. International Tax and Public Finance, 12(4), 349–373. https://doi.org/10.1007/s10797-005-1619-9
- Oates, W. E. (2008). National tax association on the evolution of fiscal federalism: Theory and institutions on the evolution of fiscal federalism: Theory and institutions. Source: National Tax Journal, 61(2), 313–334.
- Ozturk, I., & Ullah, S. (2022). Does digital financial inclusion matter for economic growth and environmental sustainability in OBRI economies? An empirical analysis. Resources, Conservation and Recycling, 185, 106489. https://doi.org/10.1016/j.resconrec.2022.106489
- Ozturk, I., Aslan, A., & Altinoz, B. (2022). Investigating the nexus between CO2 emissions, economic growth, energy consumption and pilgrimage tourism in Saudi Arabia. Economic Research-Ekonomska Istraživanja, 35(1), 3083–3098. https://doi.org/10.1080/1331677X.2021.1985577
- OECD Statistics. (2021). https://stats.oecd.org/
- Orlando, B., Ballestra, L. V., Scuotto, V., Pironti, M., & Giudice, M. D. (2022). The impact of R&D investments on eco-innovation: A cross-cultural perspective of green technology management. IEEE Transactions on Engineering Management, 69(5), 2275–2284. https://doi.org/10.1109/TEM.2020.3005525
- Pesaran, M. (2007). A simple panel unit root test in the presence of cross-section dependence. Journal of Applied Econometrics, 22(2), 265–312. https://doi.org/10.1002/jae.951
- Pesaran, M. H. (2006). Estimation and inference in large heterogeneous panels with a multifactor error structure. Econometrica, 74(4), 967–1012. https://doi.org/10.1111/j.1468-0262.2006.00692.x
- Pesaran, M. H. (2014). An empirical growth model for major oil exporters. Journal of Applied Econometrics, 29(1), 1–21. https://doi.org/10.1002/jae.2294
- Pesaran, M. H., Schuermann, T., & Weiner, S. M. (2004). Modeling regional interdependences using a global error-correcting macroeconometric model. Journal of Business & Economic Statistics, 22(2), 129–162. https://doi.org/10.1198/073500104000000019
- Ramadani, V., Abazi-Alili, H., Dana, L. P., Rexhepi, G., & Ibraimi, S. (2017). The impact of knowledge spillovers and innovation on firm-performance: Findings from the Balkans countries. International Entrepreneurship and Management Journal, 13(1), 299–325. https://doi.org/10.1007/s11365-016-0393-8
- Rani, T. (2021). Does fiscal decentralization and green innovation mitigate the environmental pollution with the help of Institutional governance? Evidence from Asian countries using novel (MMQR). Research Square, https://doi.org/10.21203/rs.3.rs-291426/v1
- Razzaq, A., Sharif, A., Najmi, A., Tseng, M. L., & Lim, M. K. (2021). Dynamic and causality interrelationships from municipal solid waste recycling to economic growth, carbon emissions and energy efficiency using a novel bootstrapping autoregressive distributed lag. Resources, Conservation and Recycling, 166, 105372. https://doi.org/10.1016/j.resconrec.2020.105372
- Razzaq, A., Wang, S., Adebayo, T. S., & Al-Faryan, M. A. S. (2022). The potency of natural resources on ecological sustainability in PIIGS economies. Resources Policy, 79, 102941. https://doi.org/10.1016/j.resourpol.2022.102941
- Samuelson, P. A. (1954). The pure theory of public expenditure. The Review of Economics and Statistics, 36(4), 387. https://doi.org/10.2307/1925895
- Scarpellini, S., Ortega-Lapiedra, R., Marco-Fondevila, M., & Aranda-Usón, A. (2017). Human capital in the eco-innovative firms: A case study of eco-innovation projects. International Journal of Entrepreneurial Behavior & Research, 23(6), 919–933. https://doi.org/10.1108/IJEBR-07-2017-0219
- Seeck, H., & Diehl, M. R. (2017). A literature review on HRM and innovation–taking stock and future directions. The International Journal of Human Resource Management, 28(6), 913–944. https://doi.org/10.1080/09585192.2016.1143862
- Song, M., Du, J., & Tan, K. H. (2018a). Impact of fiscal decentralization on green total factor productivity. International Journal of Production Economics, 205, 359–367. https://doi.org/10.1016/j.ijpe.2018.09.019
- Song, M., Wang, S., & Wu, K. (2018b). Environment-biased technological progress and industrial land-use efficiency in China’s new normal. Annals of Operations Research, 268(1–2), 425–440. https://doi.org/10.1007/s10479-016-2307-0
- Sow, M., & Razafimahefa, I. (2015). Fiscal decentralization and the efficiency of public service delivery. IMF Working Papers, 15(59), 1. https://doi.org/10.5089/9781484351116.001
- Su, C. W., Umar, M., & Khan, Z. (2021). Does fiscal decentralization and eco-innovation promote renewable energy consumption? Analyzing the role of political risk. The Science of the Total Environment, 751, 142220. https://doi.org/10.1016/j.scitotenv.2020.142220
- Sun, Y., & Razzaq, A. (2022). Composite fiscal decentralisation and green innovation: Imperative strategy for institutional reforms and sustainable development in OECD countries. Sustainable Development, 30(5), 944–957. https://doi.org/10.1002/sd.2292
- Verburg, R. M., Hartog, D. N. D., & Koopman, P. L. (2007). Configurations of human resource management practices: A model and test of internal fit. The International Journal of Human Resource Management, 18(2), 184–208. https://doi.org/10.1080/09585190601102349
- Wang, F., Rani, T., & Razzaq, A. (2022). Environmental impact of fiscal decentralization, green technology innovation and institution’s efficiency in developed countries using advance panel modelling. Energy & Environment, 958305X2210747. https://doi.org/10.1177/0958305X221074727
- Wei, L. Q., Liu, J., & Herndon, N. C. (2011). Shrm and product innovation: Testing the moderating effects of organizational culture and structure in Chinese firms. The International Journal of Human Resource Management, 22(1), 19–33. https://doi.org/10.1080/09585192.2011.538965
- Westerlund, J. (2007). Testing for error correction in panel data. Oxford Bulletin of Economics and Statistics, 69(6), 709–748. https://doi.org/10.1111/j.1468-0084.2007.00477.x
- Xuefeng, Z., Razzaq, A., Gokmenoglu, K. K., & Rehman, F. U. (2022). Time varying interdependency between COVID-19, tourism market, oil prices, and sustainable climate in United States: Evidence from advance wavelet coherence approach. Economic Research-Ekonomska Istraživanja, 35(1), 3337–3359. https://doi.org/10.1080/1331677X.2021.1992642
- Yuan, B., & Zhang, K. (2017). Can environmental regulation promote industrial innovation and productivity? Based on the strong and weak Porter hypothesis. Chinese Journal of Population Resources and Environment, 15(4), 322–336. https://doi.org/10.1080/10042857.2017.1416042