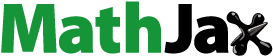
Abstract
Increasing renewable energy consumption is considered the panacea to tackle the issue of global warming and energy security. The digitalization of society can provide smart solutions for energy and environmental-related issues. Similarly, education can bring environmental awareness and encourage individuals and firms to rely more on renewable energy sources. We have tried to investigate the impact of education and digitalization on the renewable energy demand in Japan by applying the Quantile ARDL model. In the short and long run, the estimates attached to education are significantly positive almost all quantiles, which confirms that the higher the level of education in Japan, the higher the renewable energy demand. Likewise, the estimated coefficients of ICT, in both the short and long run, are positive and significant, confirming that increased digitalization help facilitate the renewable energy demand in Japan. Moreover, when we observe the Wald test, the asymmetric impact of education and digitalization are confirmed on the renewable energy demand in the short and long run.
1. Introduction
The survival of human society is largely dependent on energy consumption, which has a long-lasting effect on the stability and affluence of the nations (Brown et al., Citation1987; X. Guo et al., Citation2022). However, fossil fuel-based energy consumption has given rise to energy security problems, natural capital exhaustion, and environmental setbacks (Umar et al., Citation2022; Usman et al., Citation2022; Yousaf et al., Citation2022). The literature agrees that carbon emissions from burning fossil fuels are the primary reason behind global warming and climate change (W. Li & Ullah, Citation2022; Su et al., Citation2022). The UN agenda and (Paris Agreement Citation2015) have demanded that the world community reduce CO2 emissions to achieve a better environment (Lei et al., Citation2021). Empirics and environmentalists have presented several options to control CO2 emissions, but the most attractive solution is to increase renewable energy consumption (Akintande et al., Citation2020; Khribich et al., Citation2021). Despite all these efforts to improve the environmental quality through increasing renewable energy consumption, the actual deployment of renewable energy is still far from the target set during the Paris agreement (Su et al., Citation2022; Umar et al., Citation2021). Therefore, nations must increase renewable energy consumption as an essential part of sustainable development goals (Churchill et al., Citation2021; Ielasi et al., Citation2018; X. Ji et al., Citation2021).
Over the last two decades, the global energy market has witnessed a process of energy transition (Sohail et al., Citation2021; Yan et al., Citation2022), whereby many countries are trying to increase the generation of renewable energy (Y. Guo et al., Citation2021; Liang et al., Citation2022). Irrespective of the type of solar energy, such as solar, wind, and biomass, all are considered important strategic choices aimed at accomplishing sustainable economic development (Burke & Stephens, Citation2018; Cai & Menegaki, Citation2019; Khribich et al., Citation2021). Due to considerable policy support from all around the globe and a decline in related costs, renewable energy production is increasing globally with every passing year (Q. Ji & Zhang, Citation2019). Since the consumption of renewable is on the rise, the empirics have shifted their focus to investigating the factors of renewable energy (J. Wang et al., Citation2021). The most significant factors mentioned in the literature are macroeconomic factors such as economic growth and environmental factors (Naqvi et al., Citation2021; Uzar, Citation2020). For instance, (R. Li & Leung, Citation2021) have observed that renewable energy consumption is positively impacted by economic growth in the long run. However, (Usman et al., Citation2022) have given another view regarding it and tied the positive impact of economic growth on renewable energy demand when the economic activities are supported by renewable energy. Similarly, the erstwhile studies on the impact of environmental quality on renewable energy consumption did not provide conclusive results. Some past analyses have confirmed the positive impact of environmental degradation on renewable energy consumption (Nguyen & Kakinaka, Citation2019); conversely, some others confirmed the negative impact (Khan et al., Citation2020).
In addition, some empirics have also pointed out the significance of social factors in affecting renewable energy demand. In this regard, (Khribich et al., Citation2021 and Zhang et al., Citation2021) observed that social development promotes renewable energy. Similarly, the positive role of institutional quality is validated by (Dorfleitner & Grebler, Citation2022; Hmaittane et al., Citation2019; Uzar, Citation2020), whereas the negative role of income inequality is confirmed by (Churchill et al., Citation2021). Human capital is another important factor that can facilitate the generation of renewable energy consumption (Bansal & Kumar, Citation2021; Yao et al., Citation2019). Moreover, the literature suggests that raising awareness about environmental degradation is also crucial in increasing renewable energy production, and (Akerlof, Citation2017) provided three reasons behind such consciousness. Firstly, intervention is required through policy instruments to modify the personal behavior policy. Secondly, the institutions that drive the policies must follow democratic nor ms. Thirdly, education can directly influence environmental awareness (Hasnaoui et al., Citation2021). Environmental education is an important factor that can help improve environmental quality (W. Li & Ullah, Citation2022). The current literature also supports that raising environmental awareness has a strong role in shaping energy policies that protect the environment (Jian et al., Citation2021). The main cause of low renewable energy production in developing economies include low investment in research and development activities and a lack of environmental awareness and education (Berger, Citation2022; Ferrat et al., Citation2021; Gao et al., Citation2021). The research and development activities are directly linked to the level of formal education (Yarovaya et al., Citation2021). Hence, a low education level means low research and development activities which slow down the production of renewable energy and deteriorate the environmental quality (W. Li & Ullah, Citation2022).
The issue of climate change has become the most daunting challenge among all goals of sustainable development in the developed and developing world (Mirza et al., Citation2020; Wei et al., Citation2022). The last two decades have been impressive for expanding information and communication technologies (ICT) in emerging economies (IEA, 2020). This has led the economies toward the path of digitalization and dematerialization. Digitalization and dematerialization are critical in helping the economies to install innovative solutions as technologies that are slowly but surely becoming part and parcel of the socio-economic structure of such economies (Chen et al., Citation2022; Lobato et al., Citation2021). In the past, some empirics observed that ICT could promote energy efficiency and help the sectors like real estate, manufacturing, and transportation reduce their reliance on primary energy consumption by providing smart solutions (Usman et al., Citation2021). This is an era of digitalization where ICT is used to enhance energy efficiency with the help of data propagation, observing and administration of power grids, devolution of energy generation by assimilating clean energy projects, dispersed trading, and dematerialization.
From the above discussions, we conclude that renewable energy is a panacea to environmental degradation and depleting fossil fuel resources. Education and ICT are crucial factors in promoting renewable energy consumption. However, the empirics in the past have ignored the nexus between education, digitalization, and renewable energy consumption, particularly in the context of Japan. The top pollution emitters of the world are the USA, India, China, Russia, and Japan. Japan is determined to cut GHGs emissions from 26% to 46% from 2013 to 2030. Thus, Japan has adopted a renewable energy strategy to achieve this target by 2030 as the Japanese government aims to solve its climate change problem and wants to become a carbon-neutral economy by 2050. Renewable energy consumption is increasing rapidly in Japan as an alternative energy source to fossil fuel.
Against this background, our study determines to answer the following questions. Do education and digitalization help in enhancing the renewable energy demand in Japan? Therefore, our primary objective is to estimate digitalization and education’s impact on Japan’s renewable energy demand. The association between digitalization and education on renewable consumption in the literature is estimated through other approaches. But in this study, we have applied the latest and extended version of the ARDL model known as the Quantile ARDL model, which can estimate the short and long-run results across various quantiles. Additionally, this is the first-ever study in Japan that considers the impact of digitalization and education at different intensities of renewable energy demand. The study’s findings are valuable for policymakers, government, and educationists in understanding and evaluating how education and digitalization influence renewable energy consumption in both ways, i.e. negatively and positively.
2. Methodology and data
Following the standard literature of (Khan et al., Citation2020) and (Lv et al., Citation2022), we assume that the leading factors of renewable energy demand are education, the internet, GDP, and CO2. The QARDL method is used in this investigation. The QARDL approach seems more important and outperforms linear regression approaches (Cho et al., Citation2015). This study investigates the long-term relationship between renewable energy demand, education, internet, GDP, and CO2 across the quantile variations of the conditional distribution of various quantiles through the QARDL cointegration techniques (Shahbaz et al., Citation2018). Depending on these solid pieces of data, the QARDL approach is shown to be the best for explaining the nonlinear linkage between renewable energy demand, education, internet, GDP, and CO2. The simplest form looks like this:
(1)
(1)
In the above EquationEq. (1)(1)
(1)
represents the error term which is described through REDt-E[REDt/Ft − 1], where Ft − 1 is the smallest σ—field prepared by (REDt-1, Edut, Internett, GDPt, CO2t, REDt-1, Edut-1, Internett-1, GDPt-1, CO2t-1}, and p and n1….n4 signifies the lag orders of variables respectively. Moreover, from EquationEq. (1)
(1)
(1) , we confer that education, Internet, GDP, and CO2. are represented by Edut, Internett, GDPt, CO2t, respectively, while REDt represents renewable energy demand. Subsequent (Cho et al., Citation2015) studies have been presented in the format of QARDL as revealed below:
(2)
(2)
Where εt(τ) = REDt − QREDt(τ/Ft −1) and QREDt(τ/Ft − 1) and 0 > < 1 denote quantile. To avoid autocorrelation, we have rewritten EquationEq. (2)
(2)
(2) into generalized form:
(3)
(3)
With the help of EquationEq. (3)(3)
(3) , we can state that the possibility of a correlation between
and ΔEdut, ΔInternett, ΔGDPt, and ΔCO2t increase. Next, we have prolonged the EquationEq. (3)
(3)
(3) into the QARDL-ECM form, and revised model is revised as follows:
(4)
(4)
EquationEq. (4)(4)
(4) can derive the cumulative short-run effects through
Similarly, the cumulative short-run effects of Edut, Internett, GDPt, and CO2t are represented by
and
respectively. The cointegration among the long-run variables of education, Internet, GDP, and CO2 are described with the help of
correspondingly. The delta technique helps measure the various parameters, such as current and previous parameters and quantile cointegrating parameters (Chao et al., Citation2022). Finally, the nonlinear impact of education, the Internet, GDP, and CO2 on renewable energy demand can be measured by applying the wald test.
To evaluate the effects of education and digitalization on renewable energy demand, the present study adopts four variables, i.e. renewable energy consumption (REC), education (Edu), internet users, CO2 emissions, and GDP per capita. The data handling process is based on two steps. The annual data for all variables are collected in the first step over the period 1995 to 2020 for Japan. REC is measured as % of total final energy consumption. To assemble education data series, the study adopted secondary school enrolment. Digitalization is measured from the internet users as % of the total population. The data for CO2 emissions is gathered in terms of kilotons. Finally, GDP per capita is taken at 2015 constant US$. Data series for all these variables are collected from the WDI published by the World Bank. In the second step, the annual data series are transformed into quarterly data series using the match-sum method. The technique transforms the low-frequency to high-frequency data series.
3. Empirical results
The descriptive statistics for all concerned variables, i.e. REC, EDU, Internet, GDP, and CO2, are given in . The descriptive analysis confirms that all the mean values are positive. The mean score for REC is 4.850, with a maximum score of 7.824 and a minimum score of 3.487. The mean EDU score is 4.639, with maximum and minimum scores of 4.688 and 4.595, respectively. The average internet score is 3.820, and the maximum and minimum range is 4.536 and 0.029. The mean score for GDP is 10.40, with a maximum score of 10.50 and a minimum score of 10.29. Finally, the mean score for CO2 is found to be 13.98, with maximum and minimum scores of 14.05 and 13.88, respectively. The skewness values are positive for REC, EDU, and GDP and reported negative for the internet and CO2. The coefficients for the JB test are found to be statistically significant for all variables describing that the series is not normally distributed. These results indicate that the QARDL technique is good for this data set.
Table 1. Descriptive statistics.
Before processing the QARDL method, the study confirmed the order of data series integration properties using ADF and ZA methods. The results are illustrated in , which shows that internet and CO2 are I(0) stationary series while REC, EDU, and GDP are I(1) stationary series. Moreover, ZA detected structural breaks in the data series. It can be seen that 2019Q4 is the break period in the REC series, 1999Q1 in the EDU series, 1995Q4 in the internet series, 2010Q3 in the GDP series, and 2007Q1 in the CO2 series.
Table 2. Unit root results.
illustrates the findings of the QARDL model, where the coefficients of the constant term, speed of adjustment, and long-run and short-run coefficient estimates are displayed. The coefficient estimates of the constant term are reported to be significant and positive at all quantiles. The coefficient estimates for speed of adjustment are also significant at all quantiles. Moreover, the negative sign associated with the speed of adjustment parameters confirms the possibility of convergence toward long-term stabilization.
Table 3. Results of QARDL.
The findings of the QARDL model, in the long run, portray a significant role of education and digitalization in tempting renewable energy demand in Japan. It is reported that the coefficients for EDU are found to be significantly positive at all quantiles. It describes that the increase in secondary education level enhances REC at all intensities in Japan. The studies support our finding by (Z. Wang et al., Citation2022), who reported a similar positive nexus between education and renewable energy demand. Findings confirm that education can help increase the renewable energy demand. The result is not surprising at all because it is clearly mentioned in the available literature that education has an important role in improving environmental quality. Education, on one side, provides a strong base for the progress of human capital; on the other, it promotes awareness about the issues of energy supply and environmental degradation. In light of the available literature, we infer that people who are not highly educated may rely more on non-renewable energy sources because they are unaware of the adverse impact of burning fossil fuels (X. Li et al., Citation2022; Z. Wang et al., Citation2022). On the other side, people with higher education levels are much more aware of the issues related to environmental degradation and energy supply and, therefore, are inclined toward the demand for renewable energy, an environmentally friendly and sustainable energy source (Yang et al., Citation2017).
Moreover, the long-run coefficient estimates of the internet exhibit a positive association between digitalization and renewable energy demand, as confirmed by the significantly positive estimates of the internet at all quantiles. It reveals that an increase in internet results in increasing REC at all intensities. Indeed, the role of ICT in the green transformation of society cannot be ignored. With the increased popularity of ICT, the digitalization and dematerialization of the economy have also gathered pace (Usman et al., Citation2021). The digitalization of the economy has significantly improved energy efficiency in many sectors and thus decreased the reliance on primary energy resources (Lv et al., Citation2022). Moreover, ICT penetration can speed up the process of innovations in the energy sector due to the convenience provided by the ICT in storing, process, and diffusing data and scientific knowledge. Such activities may promote the progress of clean energy technologies through research and development activities, thus positively influencing the energy demand.
The coefficient of GDP is positively related to renewable energy consumption at all intensities except 0.05 and 0.10. The results show that GDP positively impacts REC (Zhao et al., Citation2020), providing a similar nexus between GDP and energy demand. The study justifies this relationship as the improvement in GDP per capita over time improves the lifestyle and people, and thus their renewable energy demand increases. The result also shows that the coefficient of CO2 has a significant positive effect on REC at all quantiles. It portrays that an upsurge in CO2 emissions plays a crucial role in enhancing renewable energy demand in Japan.
In the short-run, findings portray that EDU's impact on REC is significant and positive at all quantiles except 0.05. Internet variable reports significant and positive intensification in REC at all intensities except quantiles 0.05 and 0.10. The impact of GDP on REC is found to be significantly positive at all intensities. Likewise, the impact of CO2 on REC is observed significantly positive at all intensities. In the end, in order to confirm the nonlinearity of the concerned variables on REC in Japan, the Wald test is used. The outcomes of the Wald test are given in . The findings of the Wald test infer that the null hypothesis is negated for speed of adjustment and linearity parameter, so the asymmetries of coefficients are confirmed. The Wald test findings also confirm the long-run nonlinear association among EDU, Internet, GDP, CO2, and REC; conversely, the null hypothesis is accepted for all short-run parameters except EDU. Hence, reject the asymmetric association among variables in the short-run except EDU.
Table 4. Results of Wald test.
4. Conclusion and policy recommendation
Indeed, the issue of global warming has become the most serious challenge for developing and developed economies. According to environmentalists and empirics, the combustion of fossil fuels to support anthropogenic activities all around the globe is the major cause of CO2 emissions, triggering environmental degradation. Moreover, fossil fuels are depleting throughout the world, which may halt the growth process of many economies. Increasing renewable energy consumption is considered the most significant contributor to mitigating the effects of global warming. As a result, the empirics and policymakers have started to find the factors that can promote renewable energy consumption. Digitalization of society through increased reliance on ICT can drive the economy towards dematerialization that provides smart solutions for energy related-issues. Similarly, education can bring environmental awareness and encourage individuals and firms to rely more on renewable energy sources. We have tried to investigate the impact of education and digitalization on the renewable energy demand in Japan. The empirical strategy of the analysis is based on the QARDL model.
In the short and long run, the estimates attached to education are positive almost all quantiles, which confirms that the higher the level of education in Japan, the higher the renewable energy demand. Likewise, the estimated coefficients of ICT, in the short and long run, are positive and significant, confirming that increased digitalization help facilitate the renewable energy demand in Japan. The estimates of the control variables of GDP and CO2 emissions are also positively significant, signifying the beneficial role of these two in increasing renewable energy. Moreover, when we observe the Wald test, the asymmetric impact of education, digitalization, GDP, and CO2 emissions are confirmed on the renewable energy demand in the short and long run.
We have utilized the findings of the study to provide crucial guidelines for the stakeholders. Our analysis confirmed the positive impact of digitalization on increasing renewable energy demand. Therefore, policymakers must try to enhance the role of ICT in every sector, particularly the energy sector. ICT penetration in society can help the economy to shift from physical to information resources, which will speed up the process of storing, processing, and diffusing the data and help promote research and development activities, promoting renewable energy technologies. Moreover, ICT allows people and firms to control the renewable energy system and structure from a distant place through mobiles and laptops, increasing the renewable energy demand. ICT penetration conveniently transfers and propagates the data, making observing and managing power grids very easy, thereby promoting decentralization of energy generation by integrating clean energy projects. Further, the policymakers should pay attention to increasing formal education and encourage programs and workshops related to environmental awareness that can significantly increase the renewable energy demand by making people more conscious about the determinantal impact of the environment.
Though the findings of the study are crucial, yet lack in some areas. For instance, future studies must focus on other factors of renewable energy, such as technology shocks, trade openness, financial development, etc. Moreover, the factors of renewable energy consumption must be explored for other emerging and developed economies. The present study has explored the linear impact of digitalization and education on REC using the QARDL technique. It will be more beneficial if future studies explore these determinants’ asymmetric impact on Japan’s renewable energy demand. A similar objective can also be tested for other economies of the world.
Disclosure statement
No potential conflict of interest was reported by the authors.
References
- Akerlof, R. (2017). Value formation: The role of esteem. Games and Economic Behavior, 102, 1–19. https://doi.org/10.1016/j.geb.2016.10.011
- Akintande, O. J., Olubusoye, O. E., Adenikinju, A. F., & Olanrewaju, B. T. (2020). Modeling the determinants of renewable energy consumption: Evidence from the five most populous nations in Africa. Energy, 206, 117992. https://doi.org/10.1016/j.energy.2020.117992
- Bansal, M., & Kumar, V. (2021). Forcing responsibility? Examining earnings management induced by mandatory corporate social responsibility: Evidence from India. Review of Accounting and Finance, 20(2), 194–216. https://doi.org/10.1108/RAF-06-2020-0151
- Berger, D. (2022). Investor sentiment: A retail trader activity approach. Review of Accounting and Finance, 21(2), 61–82. https://doi.org/10.1108/RAF-06-2021-0152
- Brown, B. J., Hanson, M. E., Liverman, D. M., & Merideth, R. W. (1987). Global sustainability: Toward definition. Environmental Management, 11(6), 713–719. https://doi.org/10.1007/BF01867238
- Burke, M. J., & Stephens, J. C. (2018). Political power and renewable energy futures: A critical review. Energy Research & Social Science, 35, 78–93. https://doi.org/10.1016/j.erss.2017.10.018
- Cai, Y., & Menegaki, A. N. (2019). Convergence of clean energy consumption—Panel unit root test with sharp and smooth breaks. Environmental Science and Pollution Research International, 26(18), 18790–18803. https://doi.org/10.1007/s11356-019-04911-x
- Chao, T., Yunbao, X., Chengbo, D., Bo, L., & Ullah, S. (2022). Financial integration and renewable energy consumption in China: Do education and digital economy development matter? Environmental Science and Pollution Research, 1–9. https://doi.org/10.1007/s11356-022-22852-w
- Chen, J., Rojniruttikul, N., Kun, L. Y., & Ullah, S. (2022). Management of green economic infrastructure and environmental sustainability in one belt and road enitiative economies. Environmental Science and Pollution Research International, 29(24), 36326–36336. https://doi.org/10.1007/s11356-021-18054-5
- Cho, J. S., Kim, T., & Shin, Y. (2015). Quantile cointegration in the autoregressive distributed-lag modeling framework. Journal of Econometrics, 188(1), 281–300. https://doi.org/10.1016/j.jeconom.2015.05.003
- Churchill, S. A., Ivanovski, K., & Munyanyi, M. E. (2021). Income inequality and renewable energy consumption: Time-varying non-parametric evidence. Journal of Cleaner Production, 296, 126306. https://doi.org/10.1016/j.jclepro.2021.126306
- Dorfleitner, G., & Grebler, J. (2022). Corporate social responsibility and systematic risk: International evidence. The Journal of Risk Finance, 23(1), 85–120, Article 1. https://doi.org/10.1108/JRF-07-2020-0162
- Ferrat, Y., Daty, F., & Burlacu, R. (2021). Short- and long-term effects of responsible investment growth on equity returns. The Journal of Risk Finance, 23(1), Article 1. https://doi.org/10.1108/JRF-07-2021-0107
- Gao, J., O’Sullivan, N., & Sherman, M. (2021). Chinese securities investment funds: The role of luck in performance. Review of Accounting and Finance, 20(5), 271–297. https://doi.org/10.1108/RAF-07-2020-0182
- Guo, X., Liang, C., Umar, M., & Mirza, N. (2022). The impact of fossil fuel divestments and energy transitions on mutual funds performance. Technological Forecasting and Social Change, 176, Article 121429. https://doi.org/10.1016/j.techfore.2021.121429
- Guo, Y., Yu, C., Zhang, H., & Cheng, H. (2021). Asymmetric between oil prices and renewable energy consumption in the G7 countries. Energy, 226, Article 120319. https://doi.org/10.1016/j.energy.2021.120319
- Hasnaoui, J. A., Rizvi, S. K. A., Reddy, K., Mirza, N., & Naqvi, B. (2021). Human capital efficiency, performance, market, and volatility timing of Asian equity funds during COVID-19 outbreak. Journal of Asset Management, 22(5), 360–375. https://doi.org/10.1057/s41260-021-00228-y
- Hmaittane, A., Bouslah, K., & M’Zali, B. (2019). Does corporate social responsibility affect the cost of equity in controversial industry sectors? Review of Accounting and Finance, 18(4), 635–662. https://doi.org/10.1108/RAF-09-2018-0184
- Ielasi, F., Rossolini, M., & Limberti, S. (2018). Sustainability-themed mutual funds: An empirical examination of risk and performance. The Journal of Risk Finance, 19(3), 247–261. Article 3. https://doi.org/10.1108/JRF-12-2016-0159
- Ji, Q., & Zhang, D. (2019). How much does financial development contribute to renewable energy growth and upgrading of energy structure in China? Energy Policy, 128, 114–124. https://doi.org/10.1016/j.enpol.2018.12.047
- Ji, X., Zhang, Y., Mirza, N., Umar, M., & Rizvi, S. K. A. (2021). The impact of carbon neutrality on the investment performance: Evidence from the equity mutual funds in BRICS. Journal of Environmental Management, 297, Article 113228. https://doi.org/10.1016/j.jenvman.2021.113228
- Jian, L., Sohail, M. T., Ullah, S., & Majeed, M. T. (2021). Examining the role of non-economic factors in energy consumption and CO2 emissions in China: Policy options for the green economy. Environmental Science and Pollution Research International, 28(47), 67667–67676. https://doi.org/10.1007/s11356-021-15359-3
- Khan, Z., Malik, M. Y., Latif, K., & Jiao, Z. (2020). Heterogeneous effect of eco-innovation and human capital on renewable & non-renewable energy consumption: Disaggregate analysis for G-7 countries. Energy, 209, Article 118405. https://doi.org/10.1016/j.energy.2020.118405
- Khribich, A., Kacem, R. H., & Dakhlaoui, A. (2021). Causality nexus of renewable energy consumption and social development: Evidence from high-income countries. Renewable Energy. 169, 14–22. https://doi.org/10.1016/j.renene.2021.01.005
- Lei, W., Ozturk, I., Muhammad, H., & Ullah, S. (2021). On the asymmetric effects of financial deepening on renewable and non-renewable energy consumption: Insights from China. Economic Research-Ekonomska Istraživanja, 35(1), 1–18.
- Li, R., & Leung, G. C. (2021). The relationship between energy prices, economic growth and renewable energy consumption: Evidence from Europe. Energy Reports, 7, 1712–1719. https://doi.org/10.1016/j.egyr.2021.03.030
- Li, W., & Ullah, S. (2022). Research and development intensity and its influence on renewable energy consumption: Evidence from selected Asian economies. Environmental Science and Pollution Research, 29, 54448–54455. https://doi.org/10.1007/s11356-022-19650-9
- Li, X., Shaikh, P. A., & Ullah, S. (2022). Exploring the potential role of higher education and ICT in China on green growth. Environmental Science and Pollution Research, 1–8.
- Liang, C., Umar, M., Ma, F., & Huynh, T. L. (2022). Climate policy uncertainty and world renewable energy index volatility forecasting. Technological Forecasting and Social Change, 182, 121810. https://doi.org/10.1016/j.techfore.2022.121810
- Lobato, M., Rodríguez, J., & Romero, H. (2021). A volatility-match approach to measure performance: The case of socially responsible exchange traded funds (ETFs). The Journal of Risk Finance, 22(1), 34–43. Article 1. https://doi.org/10.1108/JRF-04-2020-0066
- Lv, Z., Liu, W., & Xu, T. (2022). Evaluating the impact of information and communication technology on renewable energy consumption: A spatial econometric approach. Renewable Energy. 189, 1–12. https://doi.org/10.1016/j.renene.2022.03.005
- Mirza, N., Reddy, K., Hasnaoui, A., & Yates, P. (2020). A comparative analysis of the hedging effectiveness of farmgate milk prices for New Zealand and United States dairy farmers. Journal of Quantitative Economics, 18(1), 129–142. https://doi.org/10.1007/s40953-019-00172-0
- Naqvi, B., Mirza, N., Rizvi, S. K. A., Porada-Rochoń, M., & Itani, R. (2021). Is there a green fund premium? Evidence from twenty seven emerging markets. Global Finance Journal, 50, Article 100656. https://doi.org/10.1016/j.gfj.2021.100656
- Nguyen, K. H., & Kakinaka, M. (2019). Renewable energy consumption, carbon emissions, and development stages: Some evidence from panel cointegration analysis. Renewable Energy. 132, 1049–1057. https://doi.org/10.1016/j.renene.2018.08.069
- Paris Agreement. (2015, December). Paris agreement. In Report of the Conference of the Parties to the United Nations Framework Convention on Climate Change, 21st Session, 2015 (Vol. 4, p. 2017). Paris: HeinOnline.
- Shahbaz, M., Ferrer, R., Shahzad, S. J. H., & Haouas, I. (2018). Is the tourism–economic growth nexus time-varying? Bootstrap rolling-window causality analysis for the top 10 tourist destinations. Applied Economics, 50(24), 2677–2697. https://doi.org/10.1080/00036846.2017.1406655
- Sohail, M. T., Xiuyuan, Y., Usman, A., Majeed, M. T., & Ullah, S. (2021). Renewable energy and non-renewable energy consumption: Assessing the asymmetric role of monetary policy uncertainty in energy consumption. Environmental Science and Pollution Research International, 28(24), 31575–31584. https://doi.org/10.1007/s11356-021-12867-0
- Su, C.-W., Mirza, N., Umar, M., Chang, T., & Albu, L. L. (2022). Resource extraction, greenhouse emissions, and banking performance. Resources Policy, 79, Article 103122. https://doi.org/10.1016/j.resourpol.2022.103122
- Su, C.-W., Pang, L.-D., Tao, R., Shao, X., & Umar, M. (2022). Renewable energy and technological innovation: Which one is the winner in promoting net-zero emissions? Technological Forecasting and Social Change, 182, Article 121798. https://doi.org/10.1016/j.techfore.2022.121798
- Umar, M., Farid, S., & Naeem, M. A. (2022). Time-frequency connectedness among clean-energy stocks and fossil fuel markets: Comparison between financial, oil and pandemic crisis. Energy, 240, Article 122702. https://doi.org/10.1016/j.energy.2021.122702
- Umar, M., Ji, X., Mirza, N., & Naqvi, B. (2021). Carbon neutrality, bank lending, and credit risk: Evidence from the Eurozone. Journal of Environmental Management, 296, Article 113156. https://doi.org/10.1016/j.jenvman.2021.113156
- Usman, A., Ozturk, I., Hassan, A., Zafar, S. M., & Ullah, S. (2021). The effect of ICT on energy consumption and economic growth in South Asian economies: An empirical analysis. Telematics and Informatics, 58, Article 101537. https://doi.org/10.1016/j.tele.2020.101537
- Usman, A., Ozturk, I., Naqvi, S. M. M. A., Ullah, S., & Javed, M. I. (2022). Revealing the nexus between nuclear energy and ecological footprint in STIRPAT model of advanced economies: Fresh evidence from novel CS-ARDL model. Progress in Nuclear Energy, 148, Article 104220. https://doi.org/10.1016/j.pnucene.2022.104220
- Uzar, U. (2020). Political economy of renewable energy: Does institutional quality make a difference in renewable energy consumption? Renewable Energy. 155, 591–603. https://doi.org/10.1016/j.renene.2020.03.172
- Wang, J., Zhang, S., & Zhang, Q. (2021). The relationship of renewable energy consumption to financial development and economic growth in China. Renewable Energy. 170, 897–904. https://doi.org/10.1016/j.renene.2021.02.038
- Wang, Z., Le Hoa Pham, T., Wang, B., Hashemizadeh, A., Bui, Q., & Nawarathna, C. L. K. (2022). The simultaneous impact of education and financial development on renewable energy consumption: An investigation of Next-11 countries. Environmental Science and Pollution Research, 29, 85492–85509.
- Wei, X., Ren, H., Ullah, S., & Bozkurt, C. (2022). Does environmental entrepreneurship play a role in sustainable green development? Evidence from emerging Asian economies. Economic Research-Ekonomska Istraživanja, 1–13. https://doi.org/10.1080/1331677X.2022.2067887
- Yan, L., Mirza, N., & Umar, M. (2022). The cryptocurrency uncertainties and investment transitions: Evidence from high and low carbon energy funds in China. Technological Forecasting and Social Change, 175, Article 121326. https://doi.org/10.1016/j.techfore.2021.121326
- Yang, B., Fu, X., Sidiropoulos, N. D., & Hong, M. (2017). Towards k-means-friendly spaces: Simultaneous deep learning and clustering. In International Conference on Machine Learning (pp. 3861–3870).
- Yao, Y., Ivanovski, K., Inekwe, J., & Smyth, R. (2019). Human capital and energy consumption: Evidence from OECD countries. Energy Economics, 84, Article 104534. https://doi.org/10.1016/j.eneco.2019.104534
- Yarovaya, L., Mirza, N., Abaidi, J., & Hasnaoui, A. (2021). Human capital efficiency and equity funds’ performance during the COVID-19 pandemic. International Review of Economics & Finance, 71, 584–591. https://doi.org/10.1016/j.iref.2020.09.017
- Yousaf, I., Nekhili, R., & Umar, M. (2022). Extreme connectedness between renewable energy tokens and fossil fuel markets. Energy Economics, 114, Article 106305. https://doi.org/10.1016/j.eneco.2022.106305
- Zhang, D., Mohsin, M., Rasheed, A. K., Chang, Y., & Taghizadeh-Hesary, F. (2021). Public spending and green economic growth in BRI region: mediating role of green finance. Energy Policy, 153, 112256.
- Zhao, P., Lu, Z., Fang, J., Paramati, S. R., & Jiang, K. (2020). Determinants of renewable and non-renewable energy demand in China. Structural Change and Economic Dynamics, 54, 202–209. https://doi.org/10.1016/j.strueco.2020.05.002