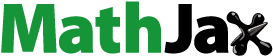
Abstract
This paper examines the impact of the technology frontier on the Total Factor Productivity (TFP) growth in African countries. The paper includes the human capital channel (i.e., low and high education) in the TFP growth function. The model also adds net foreign direct investment inflows and financial development as control variables. The long-run estimations indicate that the technology frontier hampers the TFP growth. The technology frontier adversely affects the TFP growth in a low education channel. Surprisingly, high education has a positive and insignificant impact on TFP growth. It is also found that financial development decreases the TFP growth while net foreign direct investment inflows have mixed effects. These findings suggest that educated labour embodied in rich technology frontier should also require the proper training for enhancing the TFP growth in African economies.
1. Introduction
Developing countries have faced the multifaceted challenges of rising poverty, income inequality and unemployment. Such challenges direct the government of developing countries to enhance economic growth. The process of economic growth can be increased if the factors’ productivity increases. Increasing factors’ productivity becomes possible for developing economies to catch up with advanced economies. Alternatively, how advanced economies tend to grow has to be imitated by the producers of developing economies. This is because producers in developed economies typically use augmented production functions where better technological usage and high-skilled labour forces are used. As a result, output in advanced economies increases, but this is not the case for developing countries due to the lack of better technological usage and the absence of skilled-labour forces (Gozgor & Can, Citation2017).
However, looking at the potential drivers of economic growth in developed countries, the developing economies should enhance the output level by using a better combination of higher technology and skilled-human capital in the production process. Once economic growth is enhanced, developing countries can invest in various infrastructures and welfare schemes. As a result, developing economies can retard poverty and reduce unemployment and income inequality. Similarly, consumers and producers play a pivotal role in contributing to higher economic growth through effective demand and supply generation. It implies that once market forces are influential, it will motivate producers to increase output through better technology (machine) and skilled labour forces (higher human capital).Footnote1 In this context, we are interested in empirically checking whether the technology frontier increases total factor productivity growth in developing economies in the presence of a human capital channel. Moreover, this study is interested in including low and high human capital to see their channel impacts on the technology frontier and, thereby, how the interaction between the technology frontier and the pattern of human capital contributes to the growth of total factor productivity in developing countries.
The above research questions motivate us to understand the growth theory before we proceed with the empirical investigation in developing countries. The endogenous growth theory claims the importance of human capital in stimulating economic growth (Acemoglu, Citation1996; Acemoglu et al., Citation2006; Romer, Citation1990). Motivated by the endogenous growth theory, numerous studies empirically understood the linkage between human capital and economic growth in developed and developing economies. For instance, Mankiw et al. (Citation1992) find evidence of a beneficial impact of human capital on economic growth, while Caselli et al. (Citation1996) observe a significant and negative impact of human capital, which is supported by the study of Islam (Citation1995) employing different estimators. In contrast, other studies like Knowles and Owen (Citation1995) and Pritchett (Citation2001) observe no linkage between human capital and economic growth. On the other hand, Griffith et al. (Citation2004), Kneller and Stevens (Citation2006), Madsen et al. (Citation2010) and also assert that a high level of education assists human capital in quickly adopting new and innovative technologies. Therefore, the nexus between human capital and economic growth is mixed.
Though literature affirmed the different kinds of the effect of human capital on fostering economic growth, they cannot accept the empirical puzzle given by Krueger and Lindahl (Citation2001). They claimed that human capital increases economic growth in the presence of low-level education. Regarding these issues, Vandenbussche et al. (Citation2006) attempted to resolve them by employing data from 19 OECD countries and state-level data for the United States from 1960 to 2000. They discovered that human capital does not impact imitation and innovation equally. They also added that skilled human capital could modify the technology with innovation.
In contrast, unskilled human capital encourages imitation or spreading of the remaining technology. Suppose the country is moving closer to the technology frontier. In that case, tertiary (high-level education) education is necessary for any economy because of the high growth rate. In contrast, primary (low-level education) education does not have prime importance on economic growth. The findings from the empirical analysis by Vandenbussche et al. (Citation2006) are supported by Aghion et al. (Citation2009). Though the study by Vandenbussche et al. (Citation2006) examines the role of human capital in innovation for OECD countries, they neglect the impact of the technology frontier on total factor productivity (TFP) growth through the channel of human capital in developing countries. The studies of Mendi (Citation2007) in OECD countries, Seo and Lee (Citation2006) for 38 countries and Heshmati and Kumbhakar (Citation2011) for China validate the concept of Vandenbussche et al. (Citation2006) by stating that technology enhances the TFP of the host countries.
Additionally, in terms of budgetary perspective, the returns from the different types of education are necessary. Suppose developing countries are mainly technology followers and absorb advanced technologies by adapting them to local conditions and applying them to alternative uses. In that case, the returns to lower-skilled workers exceed those of higher-skilled workers (Shahbaz et al., Citation2019b). Conversely, suppose human capital stimulates technological innovation in developed countries and raises incomes by making capital and labour more productive. In that case, the returns of high-skilled workers may be higher than those of low-skilled workers (Su et al., Citation2022c). This issue suggests that the way human capital composition affects growth may vary depending on the developmental stage of a country. Therefore, it highlights taking the developing countries as the sample area. In this context, Caselli and Coleman (Citation2006) state that skilled and unskilled workers are imperfect substitutes and that the skill differs substantially between developed and developing countries. If this is the case, the developing countries’ sample will provide important prime insights for the future.
To the best of our knowledge, few studies exist in understanding the determinants of TFP growth (Heshmati & Kumbhakar, Citation2011; Mendi, Citation2007; Seo & Lee, Citation2006; Vandenbussche et al., Citation2006). However, the impact of the technology frontier on TFP growth through the human capital channel was not considered for the African and other developing economies within a panel set-up. Therefore, our study makes an empirical understanding towards such a research gap for the African economies. However, we further believe this research gap that motivates us to investigate this issue empirically for the African economies as part of our sample analysis because of these stated reasons. First, African economies countries are chosen in our analysis because these economies are developing in nature with different problems of poverty, income inequality and unemployment.
Second, according to UNESCO Institute for Statistics (UIS), the African region has the highest rate of educational deprivation than any other region. Around one-third of adolescents between the ages of 12 and 14 are not enrolled in school, followed by roughly one-fifth of children between 6 and 11 who do not attend school. The UIS indicates that almost 60% of children between the ages of 15 and 17 aren’t even enrolled in school. Third, the economic outlook of African economies has become most severe in recent years due to the rising food and energy expenditures and more governmental debt. Besides providing a solution towards the region’s high inflation rate, fostering a higher economic growth rate is one of the most critical issues facing this region in the 21st century.Footnote2 This consequence makes this region the centre of attraction for policymakers and researchers working towards developing African economies.
In doing this analysis for the set of African economies within a panel framework, this paper contributes to some aspects of the growth and development literature. First, following the study of Vandenbussche et al. (Citation2006), we examine the role of the technology frontier in TFP growth for 27 African economies. Second, we also consider the role of low (primary) and high (tertiary) human capital as the suitable channel in TFP function through which we empirically understand the impact of the technology frontier on TFP growth. We are also adding high (tertiary) and low (primary) education with technological frontier to check the interactive impact of these variables on TFP growth. Third, in terms of econometric methodology, we introduced a new technique named the cross-sectional ARDL model proposed by Chudik and Pesaran (Citation2015) to estimate the relationship between technology frontier and economic growth in the presence of low and high levels of education in African countries. In light of our empirical analysis, our results could benefit African countries in enhancing their long-term TFP growth.
The following section 2 contains the empirical framework. Section 3 declares the data sources, models, and methodology. Section 4 represents the results and discussion. Section 5 highlights the important policy implications of the results. Section 6 concludes with critical findings. Section 7 discusses limitations and directions for future research.
2. Empirical framework
Literature evidenced that different educational levels of human capital affect TFP growth (Aghion et al., Citation2009; Vandenbussche et al., Citation2006). A high level of intellectual (skilled) human capital is required for innovation, and a low level of education (unskilled) is fitted for imitation. The impact of the technological frontier is negatively related to TFP growth. This theory follows the hypothesis of the advantages of backwardness proposed by Gerschenkron (Citation1962), which summarises that a country with less technology frontier enhances the TFP growth due to lower effective innovation cost, which allows a faster catch-up to the technology frontier. Therefore, the impact of human capital with higher education (skilled) and technological frontier enhances the TFP growth as innovation is a skill-intensive activity. On the other hand, unskilled or low-level education human capital decreases TFP growth with the technology frontier because imitation needs mostly physical and less educated human capital (Vandenbussche et al., Citation2006). The following empirical equation has been taken to test this hypothesis:
(1)
(1)
where
is the TFP growth,
is the relative TFP growth across the country i (1……27) and the United States (technology ladder), which is empirically calculated as a proxy of technological frontier; h is a vector which indicates different levels of education (primary and tertiary), X is a vector of the control variables (foreign direct investment and financial development),
captures the individual invariant time effects,
is the time-invariant country-specific fixed effects, and
is the stochastic error term.
The focus variables in the model proposed by Vandenbussche et al. (Citation2006) are human capital (levels of low and high education) and the interaction between human capital and the technological frontier. The model predicts that the signs of these variables’ coefficients depend on the education levels. Additionally, they argue that the interaction between human capital with tertiary (high) education and the technological frontier is positive. In contrast, human capital with primary (low) education and the technological frontier is negative. The low levels of education following the innovation in the frontier country is why the interaction effect adversely affects the TFP growth. Suppose the country is near the technological frontier. In that case, the imitation effect becomes more prominent as the effective imitation costs are less. They also added that the impact of primary and secondary education levels is insignificant. In contrast, tertiary education positively and significantly affected TFP growth because of the power of innovation.
3. Empirical models and data
3.1. Empirical models
The empirical estimation of this study is based on the cross-sectional autoregressive distributed lag (CS-ARDL) estimator proposed by Chudik and Pesaran (Citation2015) for a panel of 27 African countries from 1991 to 2019. A sample of 27 African countries is chosen based on the data availability. Following the existing literature, to make a comparison of the relative effects of technological frontier and human capital on TFP growth, we represent the models which describe TFP growth as a function of technological frontier, human capital (primary and tertiary education), foreign direct investment and financial development as follows:
(2)
(2)
(3)
(3)
Where i indicate the country from 1–27, and t denotes the time from 1991 to 2019. TFP growth (TFPG) is the annual TFP growth rate.Footnote3 TF is a proxy for the technological frontier (), which is the relative TFP gap between country i (i = 1….27) and the United States (technology leader). PR and TR are the proxies of human capital, which denotes primary and tertiary enrolment, respectively. FDI and FD are the two control variables included in the regression function of TFP, named foreign direct investment and financial development, respectively. The variables’ selection is based on Ang et al. (Citation2011), Gozgor (Citation2015), Liu and Li (Citation2022), Shahbaz et al. (Citation2019a), Tao et al. (Citation2022a), and Xiuwu et al. (Citation2022).Footnote4 The source-wise details of the variables collected are provided in . The summary statistics are also presented in . The mean and standard deviation values of PR, TR, FDI, and FD are higher than those of TFP growth and Technology frontier (TF).
Table 1. Variables details and sources.
depicts the correlation between TFP growth with technological frontier, human capital (primary and tertiary education), foreign direct investment, and financial development. Most variables are negatively correlated with TFP growth, i.e., technological frontier, tertiary education, foreign direct investment, and financial development, except primary education. The lower value of correlation between the independent variables is the primary concern. Still, it does not create any threat of multicollinearity issues for modelling the TFP growth function in the case of African countries. In this situation, the estimated coefficients are expected to be unbiased.
Table 2. Pairwise correlation matrix.
3.2. Econometric methods
Given the considerable linkage between TFP growth and the technological frontier among 27 African countries, we need to empirically estimate our variables’ cross-sectional dependence in the TFP growth function. In doing so, we use the cross-sectional dependence (CD) test proposed by Breusch and Pagan (Citation1980) and Pesaran (Citation2004, Citation2015). These tests follow the below statistical expression:
(4)
(4)
where i is the number of cross-sectional countries (1–27), t represents the time (1991–2019), and
is a (k × 1) matrix of the concerned regressors. While
is the intercept, and
is the slope coefficient, which varies across the panels. These methods are valid while N > T and T > N. Therefore, CDLM and bias-adjusted LM (LMadj) tests were found appropriate, and their test statistics can be calculated as follows:
(5)
(5)
(6)
(6)
where
and
represents variance, mean, and the sample estimate of the pairwise correlation of the residuals. In this context, the null hypothesis of cross-sectional independence (H0): COV (μit, μjt) = 0 for all t and i
j, which is tested against the alternative hypothesis (H1): COV (μit, μjt)
0 for at least one pair of i
j.
Because of the cross-sectional dependence established across panel units in this study, first-generation panel stationarity tests such as the IPS unit root test proposed by Im et al. (Citation2003) may fail to address the problem of cross-sectional dependence. We solve this issue, and the cross-sectionally augmented Im, Pesaran, and Shin (CIPS) suggested by Pesaran (Citation2007) has been employed on all the variables associated with this study. The method considers the cross-sectional dependencies in the data set and produces reliable results. EquationEquation (6)(6)
(6) represents the CIPS test estimation process:
(7)
(7)
Where notations is the operator for differences,
is the explanatory variable under investigation, T is the time,
is the intercept and
is the error term, respectively. However, in the presence of cross-sectional dependency, endogeneity and unit-root, we employ Cross-Sectional Autoregressive Distributed Lags (CS-ARDL) method proposed by Chudik and Pesaran (Citation2015) to estimate our empirical model. This method has many advantages over other panel estimation methods, such as providing robust results whether the series are co-integrated. The order of integration is I (0), I (1), or a combination of both (Chudik et al., Citation2017). As it is an ARDL version of the dynamic standard correlated estimator based on individual estimators with lagged dependent variables and cross-section averages, it considers the problems of cross-sectional dependence and endogeneity (Chudik & Pesaran, Citation2015). This issue allows the estimation of the group mean when the slope coefficients are heterogeneous. The group mean version of the CS-ARDL model is based on augmenting the ARDL estimate with the cross-sectional mean of each cross-section as a proxy for the unobserved common factors and their lags (Chudik et al., Citation2017). This method can work with the weak exogeneity problem when lagged dependent variables are added to the model. The authors claim that augmenting the model with lagged cross-section averaging avoids the endogeneity problem. The CS-ARDL estimate is based on the following regression.
(8)
(8)
where
refers to lagged cross-sectional averages [
]. The long-run coefficient of mean group estimates is:
(9)
(9)
where
represents the individual estimations of each cross-section. The error correction form of the CS-ARDL method is
(10)
(10)
where
represents error correction speed of adjustment.
(11)
(11)
where
is the dependent variable,
is the explanatory variables vectors, including FDI and FD,
denotes expected values of regressors for the long run,
expresses the long-run expected value of regressors,
is the short-run dependent variable,
is the short-run independent variable,
denotes the short-run expected value of explanatory variables,
is the error term. j is the cross-sectional units (1……. j), and t is the time index (1…… T). Unfortunately, this long-run method also does not free from limitations. This method does not exhibit homogeneity restrictions on parameters, which is a shortcoming of the CS-ARDL. Despite certain commonalities, African countries impose some degree of technological, political and economic reform differences that cannot be solved by this empirical method used in the analysis.
4. Results and discussion
In , we reported the results of the first-generation unit-root tests proposed by Im et al. (Citation2003) to examine the presence of the unit roots. The results show that all series have a mixed order of integration [i.e., I (0) and I (1)]. As we have employed the cross-sectional dependence test shown in , we find that the null hypothesis of cross-section independence is rejected, indicating the presence of cross-sectional dependence in the panel data set. It also implies the presence of serial correlation between cross-country error terms. According to Bhattacharya and Narayan (Citation2015), this is happening because of the heterogeneous effect across the countries.
Table 3. First-generation unit root test (IPS unit root test).
Table 4. Cross-sectional dependence test.
Moreover, one can argue that cross-sectional dependence between the countries is possible if any policy change in one country can affect another. In this context, the traditional unit root test is not ideal because it does not capture the cross-sectional dependence present in the data series. Therefore, to solve this issue, this study employs augmented cross-sectional IPS (CIPS) tests proposed by Pesaran (Citation2007). The CIPS unit root test results depict that most variables have unit roots mainly at intercept with the trend (see ). Thus, panel unit root results give a signal for applying panel cointegration.
Table 5. Second-generation unit root test (cross-sectional IPS unit root test).
After estimating the panel unit root tests, we employed the panel cointegration technique. As per Pedroni’s (Citation1999, Citation2004) cointegration test results, summarises that cointegration exists between the variables across all models. Therefore, we are getting the long-run association between the panel data series. Along with the result of the Pedroni cointegration test, the results of the residual cointegration of Westerlund’s (Citation2007) also support the validity of the cointegration across both models (see ). However, it is proven that TFP growth with other independent variables has significant evidence of the long-term relationship.
Table 6. Panel Pedroni cointegration test.
Table 7. Panel westerlund cointegration test.
4.1. Panel cross-sectional ARDL result analysis
We use the panel CS-ARDL model to study the short-run and long-run relationships between the variables for 27 African countries. The long-run results in Model1 of indicate that the technological frontier (TF) negatively affects TFP growth (TFPG). It suggests that the technological frontier is not utilised effectively by business firms in African economies. In this context, one can argue that factor productivity growth can be enhanced if the technological frontier is effectively introduced with the right labour forces (i.e., skilled human capital). At the same time, low-level education (primary) benefits TFP growth. This finding can be justified because people or low education workers get jobs in the employment market. But once they get the job, they would like to contribute to the production process effectively. This is because they know business firms can go through a loss-making situation if they don’t put in their best effort. At the same time, labour forces can find it very hard to survive in the business organisation.
Table 8. Panel cross-sectional-autoregressive-distributed lag (CS-ARDL) test results.
If both technological frontier and primary (low) education interact, they also negatively affect TFP growth. Because innovation is vital for skilled human capital, and low-level education is generally better suited for imitation. Therefore, low-skilled labour forces with low education could not contribute to the upward growth of business activity even if they are engaged in the production process. One can further argue that low-skilled labour forces do not know how to use better technology when it is available. As a result, TFP growth declines with the interaction between the technological frontier and low education. Finally, this finding supports the hypothesis of the advantage of backwardness suggested by Gerschenkron (Citation1962).
Surprisingly in Model 2 of , we find that tertiary (high) education has a significant and negative impact on TFP growth in African economies. It implies that although educated labour is embodied with rich technology frontier in TFP growth function, the labour forces are not provided with the proper training to use the technology when embodied in production. As a result, educated labour forces are employed in production but lack technical knowledge of correctly using the technology. This issue could be one of the reasons for the adverse impact of high education on TFP growth. We also find that the technological frontier through the higher education channel (interaction between the technological frontier and high education) increases TFP growth in the long run. Still, it is not practical because of its insignificant coefficient. In light of this finding, one can conclude that the higher education channel is not working when used in productivity functions. This is because labour forces with higher education also lack knowledge of technological usage.
Foreign direct investment increases TFP growth when higher education is included as an essential human capital channel in the TFP growth function. However, the reduced impact of foreign direct investment on TFP growth is insignificant in the presence of low-level education. It happens mainly because of the inability to use foreign assistance while enhancing TFP growth. Though our finding shows the mixed impact of foreign direct investment on TFP growth, the higher education channel (tertiary education) is compatible with foreign business because of the required knowledge capital. Finally, we find that financial development significantly reduces TFP growth. It may be true because business firms in African economies may get financial support from the banking sector but cannot utilise the financial resources effectively in production (Su et al., Citation2022b). This result could be one of the reasons for the adverse impact of financial development on TFP growth in African economies. This finding goes against the primary idea of Schumpeter (Citation1912), who noticed the enhancing impact of financial development on factor productivity growth.
This model observes the varying degree of short-run disequilibrium adjustments (error correction term, hereafter ECT) toward achieving the long-run TFP growth equilibrium and indicates panel CS-ARDL model accuracy. We conclude that the short-run results are comparatively matching with the long-run results. In comparison, the ECT is negative and significant across all the models.Footnote5 In terms of policy perspective, it suggests that policymakers of the African economies must scrutinise the adverse effects of tertiary education on TFP growth. In contrast, policymakers should consider issues like education inequality while formulating long-term educational policies.
5. Policy implications of the results
The long-run findings of this study, shown in , have some important policy implications for African countries. We find that the technology frontier significantly reduces TFP growth. It shows that technological progress adopted into the production function does not contribute to the growth of TFP. Somewhat it hampers the TFP growth. In light of this, one can argue that labour forces that handle the technology in production need to be trained enough to avoid declining TFP growth.
We also find that primary (tertiary) education positively (negatively) affects TFP growth. This evidence is surprising where low education benefits the TFP growth rather than high education. Suppose TFP growth is threatened by high education. In that case, growth sustainability in African economies cannot be ensured in the long run. Thus, it is a matter of concern for the policymakers and governments in African economies to understand the weakness of high education when it is included TFP growth function.
Furthermore, we find the mixed impact of technology frontier on TFP growth regarding human capital channels. It shows that TFP growth decreases with technology frontier when associated with low education. The positive impact of the technology frontier on TFP growth remains ineffective in the presence of high education. From the policy perspective, we suggest that these economies should enhance the technology-knowledge-related training opportunity for labour forces that are highly educated and newly employed. In doing this, it is not easy for the government of African countries because of their rising poverty, inequality and unemployment. Therefore, we suggest that the governments in these economies should utilise foreign assistance in developing the educational sector and invest more in making people well-trained. Once labour forces or people with higher education are well-trained to use the highest degree of technology, they can enhance TFP growth at a higher rate.
Suppose the African economies experience a higher rate of TFP growth. In that case, the governments in these countries can invest more in welfare-enhancing schemes like employment generation and poverty reduction. Perhaps, the governments in these economies can also import better frontier technology from other advanced countries that could be used extensively in production. Eventually, the production function should also include the right set of labour forces with higher human capital and better knowledge about technological usage. In light of this, we know that production functions can be augmented using a better combination of suitable technology and skilled human capital that could result in higher output in the long run. But the augmented production function can also be considered to replace a low-skilled labour force because of the focus more on skilled and educated people who are highly compatible with the technological frontier.
From a policy perspective, one can suggest that augmented production function in African economies should include both low and high-skilled labour forces besides using technology frontier to avoid rising unemployment in the labour market. The simple reason is that African economies are poor and developing and have poverty and unemployment problems. Suppose augmented production function can only use better technology frontier and skilled labour. In that case, it is a matter of concern for the low-skilled labours in African economies because of the fear that they might have an unemployment scenario in the long run due to the usage of low-skilled labour forces replacing technology. On a final note, we argue that increasing factor productivity is essential for the African economies, which can enable these economies to catch up with advanced economies if the governments invest more in training for both low and skilled labour forces and better education.
Surprisingly, we find the mixed effects of foreign direct investment on the technology frontier in African economies. The varying effects of foreign direct investment on TFP growth in African economies signal the policymakers and fiscal government to allow foreign investments that will contribute to the growth of TFP. This evidence is because TFP growth is the key to long-term sustainable development. If inefficient foreign investments are allowed, it not only hampers the growth of TFP but can also deny African economies to experience sustainable development goals (Azam et al., Citation2021).
We also find the adverse effect of financial development on TFP growth. It shows that financial development does not drive TFP growth. Instead, financial development hampers the growth of TFP in African economies. It shows that a developed financial system enables producers to take loans from the banking sector to invest in business activity. Even if producers use the money in business, financial investment inefficiency could result in declining TFP growth. The declining TFP growth rate can cause a loss of profit for the producers. So, producers cannot repay the principal amount and cost of borrowing to the banking sector. Such a situation can also multiply the occurrence of non-performing assets in the banking sector, which is a greater sign of a financial crisis. Because of this, we suggest that the monetary regulator should direct the banking sector to extend the loan to producers with creditworthiness and profit-making capacity. Then only financial crisis due to loan defaulters can be avoided in African economies.
6. Conclusions
This study has examined the impact of the technological frontier on TFP growth in 27 African economies from 1991 to 2019. This objective is also examined to see whether the human capital channel is helping the technological frontier to enhance TFP growth in African economies.
The result of the panel CS-ARDL method for long-run estimation observes that primary (tertiary) education positively (negatively) affects TFP growth. Hence, the technological frontier negatively affects TFP growth across both models. But interestingly, at the time of interaction between primary and tertiary education with the technological frontier, primary (tertiary) education negatively (positively) affects the TFP growth. This evidence implies that in the presence of professional education of human capital, the African economies can attain a higher rate of TFP growth as they can concentrate more on innovation rather than imitation by reducing the technological gaps. But this remains a distant dream for the African economies to attain a higher rate of TFP growth as the higher human capital channel becomes insignificant in the estimated model despite its positive impact. In light of this finding, one could view it as a concern for the African economies due to the ineffective positive influence of higher human channels on TFP growth.
We find that in the case of primary education, human capital cannot contribute to TFP growth as it is not driven through innovation and imitation, mainly by a highly educated person. Therefore, these findings prove the hypothesis of Vandenbussche et al. (Citation2006). Finally, financial development should be added to the TFP growth function as one of the crucial determinants because of its adverse impact on TFP growth in the long run. However, the varying effects of foreign direct investment indicate them as an essential determinant of TFP growth.
The findings cannot be generalised to another developing world because it is specific to the African economies. It is true because African countries’ socioeconomic and political characteristics differ from others in the developing world. However, this study is not free from some underlying limitations. First, this study has not analysed the African region’s low- and middle-income countries. Second, we have ignored the role of gender human capital as one of the potential channels in total factor productivity growth function while doing the empirical analysis for 27 African countries. Third, we have not included climate policy uncertainty, the Covid-19 outbreak, the global financial crisis, outward foreign direct investment and urbanisation as essential factors in the total productivity growth function.
Therefore, we believe that these determinants cited in the above limitations are crucial in productivity growth following the recent studies (Bajra et al., Citation2023; Pan & Yue, Citation2022; Ren et al., Citation2022; Shang et al., Citation2022; Song et al., Citation2021; Xiuwu et al., Citation2022). Hence, we suggest these gaps for future research, mainly in applied public and resources economics. In this context, one could do these suggested research gaps for the African countries or can think of doing a global analysis. Perhaps, findings based on the global data could be generalised across the developing world. Finally, we believe that exciting perspectives could arise when one engages in doing it within a panel framework with the help of advanced estimated techniques. Moreover, the TFP growth function can be augmented by adding climate policy uncertainty due to extreme natural events (i.e., tsunamis and floods) often-taking place and affecting all countries worldwide. Since all economies have been affected by the Covid-19 outbreak since 2020 (Atsalakis et al., Citation2021; Bajra et al., Citation2023; Pan & Yue, Citation2022; Qureshi, Citation2022; Su et al., Citation2022a; Tao et al., Citation2022b; Zhang et al., Citation2022), the future study needs to include it while understanding the TFP growth function for the developing economies.
Disclosure statement
No potential conflict of interest was reported by the authors.
Notes
1 A combination of better technological usage and high human capital refers to the technological frontier. However, the technology frontier is usually looked into the total factor productivity differences, which are measured by the ratio of factor productivity of a developing economy divided by the total factor productivity of the United States economy.
2 African economies are mostly facing reduced economic growth and development due to less trade openness and FDI flows (Buysse et al., Citation2018), higher level of poverty (Yameogo & Omojolaibi, Citation2021), and minimal opportunities for education and jobs (David & Grobler, Citation2020).
3 The TFP is estimated as a residual of the aggregate production function (Y/KαL1-α), where Y is real GDP per capita, K is physical capital stock, L is the labour force, and α is a portion of income which usually goes to capital stock. The capital stock is measured by employing the Solow model steady-state value of RIi0 / (dep + growth), where RIi0 is the real investment, dep is the rate of depreciation, and growthi is the real investment growth rate over the sample period. The value of the capital stock is generated by employing the perpetual inventory method by taking the current depreciation rate.
4 Liu and Li (Citation2022) pointed out the influential impact of the banking sector on total factor productivity for developing countries. Per their thought, the number of bank branches and expansion of the banking operation across the regions can improve the TFP. Xiuwu et al. (Citation2022) evidenced the importance of two-way foreign direct investment (FDI) on TFP. They stated that the enhancement of the overseas investment opportunity could produce more productivity growth in the domestic economy.
5 More extensive discussion of short-run results is not made to conserve the journal's space.
References
- Acemoglu, D. (1996). A microfoundation for social increasing returns in human capital accumulation. The Quarterly Journal of Economics, 111(3), 779–804. https://doi.org/10.2307/2946672
- Acemoglu, D., Aghion, P., & Zilibotti, F. (2006). Distance to frontier, selection, and economic growth. Journal of the European Economic Association, 4(1), 37–74. https://doi.org/10.1162/jeea.2006.4.1.37
- Aghion, P., Boustan, L., Hoxby, C., & Vandenbussche, J. (2009). The causal impact of education on economic growth: Evidence from US. Brookings Papers Economic Activity, 1(1), 1–73.
- Ang, J. B., Madsen, J. B., & Islam, M. R. (2011). The effects of human capital composition on technological convergence. Journal of Macroeconomics, 33(3), 465–476. https://doi.org/10.1016/j.jmacro.2011.03.001
- Atsalakis, G. S., Bouri, E., & Pasiouras, F. (2021). Natural disasters and economic growth: A quantile on quantile approach. Annals of Operations Research, 306(1–2), 83–109. https://doi.org/10.1007/s10479-020-03535-6
- Azam, M., Hunjra, A. I., Bouri, E., Tan, Y., & Al-Faryan, M. A. S. (2021). Impact of institutional quality on sustainable development: Evidence from developing countries. Journal of Environmental Management, 298, 113465.
- Bajra, U. Q., Aliu, F., Aver, B., & Čadež, S. (2023). COVID-19 pandemic–related policy stringency and economic decline: Was it really inevitable? Economic Research-Ekonomska Istraživanja, 36(1), 499–515. https://doi.org/10.1080/1331677X.2022.2077792
- Bhattacharya, M., & Narayan, P. (2015). Output and labor productivity in organised manufacturing: A panel cointegration analysis for India. International Journal of Production Economics, 170, 171–177. https://doi.org/10.1016/j.ijpe.2015.09.020
- Breusch, T. S., & Pagan, A. R. (1980). The Lagrange multiplier test and its applications to model specification in econometrics. The Review of Economic Studies, 47(1), 239–253. https://doi.org/10.2307/2297111
- Buysse, J., Can, M., & Gozgor, G. (2018). Globalisation outcomes and the real output in the SubSaharan Africa LICs: A cointegration analysis. Economic Research-Ekonomska Istraživanja, 31(1), 338–351. https://doi.org/10.1080/1331677X.2018.1426471
- Caselli, F., & Coleman, J. W. II. (2006). The world technology frontier. American Economic Review, 96(3), 499–522. https://doi.org/10.1257/aer.96.3.499
- Caselli, F., Esquivel, G., & Lefort, F. (1996). Reopening the convergence debate: A new look at cross-country growth empirics. Journal of Economic Growth, 1(3), 363–389. https://doi.org/10.1007/BF00141044
- Chudik, A., & Pesaran, M. H. (2015). Common correlated effects estimation of heterogeneous dynamic panel data models with weakly exogenous regressors. Journal of Econometrics, 188(2), 393–420. https://doi.org/10.1016/j.jeconom.2015.03.007
- Chudik, A., Mohaddes, K., Pesaran, M. H., & Raissi, M. (2017). Is there a debt-threshold effect on output growth? Review of Economics and Statistics, 99(1), 135–150. https://doi.org/10.1162/REST_a_00593
- David, O. O., & Grobler, W. (2020). Information and communication technology penetration level as an impetus for economic growth and development in Africa. Economic Research-Ekonomska Istraživanja, 33(1), 1394–1418. https://doi.org/10.1080/1331677X.2020.1745661
- Gerschenkron, A. (1962). Economic backwardness in historical perspective: A book of essays. Harvard University Press.
- Gozgor, G. (2015). Causal relation between economic growth and domestic credit in the economic globalization: Evidence from the Hatemi-J's test. The Journal of International Trade & Economic Development, 24(3), 395–408. https://doi.org/10.1080/09638199.2014.908325
- Gozgor, G., & Can, M. (2017). Causal linkages among the product diversification of exports, economic globalisation and economic growth. Review of Development Economics, 21(3), 888–908. https://doi.org/10.1111/rode.12301
- Griffith, R., Redding, S., & Reenen, J. V. (2004). Mapping the two faces of R&D: Productivity growth in a panel of OECD industries. Review of Economics and Statistics, 86(4), 883–895. https://doi.org/10.1162/0034653043125194
- Heshmati, A., & Kumbhakar, S. C. (2011). Technical change and total factor productivity growth: The case of Chinese provinces. Technological Forecasting and Social Change, 78(4), 575–590. https://doi.org/10.1016/j.techfore.2010.11.006
- Im, K. S., Pesaran, M. H., & Shin, Y. (2003). Testing for unit roots in heterogeneous panels. Journal of Econometrics, 115(1), 53–74. https://doi.org/10.1016/S0304-4076(03)00092-7
- Islam, N. (1995). Growth empirics: A panel data approach. The Quarterly Journal of Economics, 110(4), 1127–1170. https://doi.org/10.2307/2946651
- Kneller, R., & Stevens, P. A. (2006). Frontier Technology and absorptive capacity: Evidence from OECD manufacturing industries. Oxford Bulletin of Economics and Statistics, 68(1), 1–21. https://doi.org/10.1111/j.1468-0084.2006.00150.x
- Knowles, S., & Owen, P. D. (1995). Health capital and cross-country variation in income per capita in the Mankiw-Romer-Weil model. Economics Letters, 48(1), 99–106. https://doi.org/10.1016/0165-1765(94)00577-O
- Krueger, A. B., & Lindahl, M. (2001). Education for growth: Why and for whom? Journal of Economic Literature, 39(4), 1101–1136. https://doi.org/10.1257/jel.39.4.1101
- Liu, P., & Li, H. (2022). The impact of banking competition on firm total factor productivity. Economic Research-Ekonomska Istraživanja, 35(1), 4008–4028. https://doi.org/10.1080/1331677X.2021.2010109
- Madsen, J. B., Islam, M. R., & Ang, J. B. (2010). Catching up to the technology frontier: The dichotomy between innovation and imitation. Canadian Journal of Economics/Revue Canadienne D'économique, 43(4), 1389–1411. https://doi.org/10.1111/j.1540-5982.2010.01618.x
- Mankiw, N. G., Romer, D., & Weil, D. N. (1992). A contribution to the empirics of economic growth. The Quarterly Journal of Economics, 107(2), 407–437. https://doi.org/10.2307/2118477
- Mendi, P. (2007). Trade in disembodied technology and total factor productivity in OECD countries. Research Policy, 36(1), 121–133. https://doi.org/10.1016/j.respol.2006.09.028
- Pan, K., & Yue, X. G. (2022). Multidimensional effect of Covid-19 on the economy: Evidence from survey data. Economic Research-Ekonomska Istraživanja, 35(1), 1658–1685. https://doi.org/10.1080/1331677X.2021.1903333
- Pedroni, P. (1999). Critical values for cointegration tests in heterogeneous panels with multiple regressors. Oxford Bulletin of Economics and Statistics, 61(S1), 653–670. https://doi.org/10.1111/1468-0084.61.s1.14
- Pedroni, P. (2004). Panel cointegration: Asymptotic and finite sample properties of pooled time series tests with an application to the PPP hypothesis. Econometric Theory, 20(03), 597–625. https://doi.org/10.1017/S0266466604203073
- Pesaran, M. H. (2004). General diagnostic tests for cross section dependence in panels. IZA Discussion Paper, No. 1240. Institute for the Study of Labor (IZA).
- Pesaran, M. H. (2007). A simple panel unit root test in the presence of cross‐section dependence. Journal of Applied Econometrics, 22(2), 265–312. https://doi.org/10.1002/jae.951
- Pesaran, M. H. (2015). Testing weak cross-sectional dependence in large panels. Econometric Reviews, 34(6-10), 1089–1117. https://doi.org/10.1080/07474938.2014.956623
- Pritchett, L. (2001). Where has all the education gone? The World Bank Economic Review, 15(3), 367–391. https://doi.org/10.1093/wber/15.3.367
- Qureshi, F. (2022). COVID-19 pandemic, economic indicators and sectoral returns: Evidence from US and China. Economic Research-Ekonomska Istraživanja, 35(1), 2142–2172. https://doi.org/10.1080/1331677X.2021.1934508
- Ren, X., Zhang, X., Yan, C., & Gozgor, G. (2022). Climate policy uncertainty and firm-level total factor productivity: Evidence from China. Energy Economics, 113, 106209. https://doi.org/10.1016/j.eneco.2022.106209
- Romer, P. M. (1990). Endogenous technological change. Journal of Political Economy, 98(5, Part 2), S71–S102. https://doi.org/10.1086/261725
- Schumpeter, J. A. (1912). The theory of economic development: An inquiry into profits, capital, credit, interest and the business cycle. Harvard University Press.
- Seo, H. J., & Lee, Y. S. (2006). Contribution of information and communication technology to total factor productivity and externalities effects. Information Technology for Development, 12(2), 159–173. https://doi.org/10.1002/itdj.20021
- Shahbaz, M., Gozgor, G., Adom, P. K., & Hammoudeh, S. (2019a). The technical decomposition of carbon emissions and the concerns about FDI and trade openness effects in the United States. International Economics, 159, 56–73. https://doi.org/10.1016/j.inteco.2019.05.001
- Shahbaz, M., Gozgor, G., & Hammoudeh, S. (2019b). Human capital and export diversification as new determinants of energy demand in the United States. Energy Economics, 78, 335–349. https://doi.org/10.1016/j.eneco.2018.11.016
- Shang, Y., Han, D., Gozgor, G., Mahalik, M. K., & Sahoo, B. K. (2022). The impact of climate policy uncertainty on renewable and non-renewable energy demand in the United States. Renewable Energy. 197, 654–667. https://doi.org/10.1016/j.renene.2022.07.159
- Song, Y., Hao, F., Hao, X., & Gozgor, G. (2021). Economic policy uncertainty, outward foreign direct investments, and green total factor productivity: Evidence from firm-level data in China. Sustainability, 13(4), 2339. https://doi.org/10.3390/su13042339
- Su, C. W., Dai, K., Ullah, S., & Andlib, Z. (2022a). COVID-19 pandemic and unemployment dynamics in European economies. Economic Research-Ekonomska Istraživanja, 35(1), 1752–1764. https://doi.org/10.1080/1331677X.2021.1912627
- Su, C. W., Pang, L. D., Tao, R., Shao, X., & Umar, M. (2022b). Renewable energy and technological innovation: Which one is the winner in promoting net-zero emissions? Technological Forecasting and Social Change, 182, 121798. https://doi.org/10.1016/j.techfore.2022.121798
- Su, C. W., Yuan, X., Umar, M., & Lobonţ, O. R. (2022c). Does technological innovation bring destruction or creation to the labor market? Technology in Society, 68, 101905. https://doi.org/10.1016/j.techsoc.2022.101905
- Tao, R., Su, C. W., Naqvi, B., & Rizvi, S. K. A. (2022a). Can Fintech development pave the way for a transition towards low-carbon economy: A global perspective. Technological Forecasting and Social Change, 174, 121278. https://doi.org/10.1016/j.techfore.2021.121278
- Tao, R., Su, C. W., Yaqoob, T., & Hammal, M. (2022b). Do financial and non-financial stocks hedge against lockdown in Covid-19? An event study analysis. Economic Research-Ekonomska Istraživanja, 35(1), 2405–2426. https://doi.org/10.1080/1331677X.2021.1948881
- Vandenbussche, J., Aghion, P., & Meghir, C. (2006). Growth, distance to frontier and composition of human capital. Journal of Economic Growth, 11(2), 97–127. https://doi.org/10.1007/s10887-006-9002-y
- Westerlund, J. (2007). Testing for error correction in panel data. Oxford Bulletin of Economics and Statistics, 69(6), 709–748. https://doi.org/10.1111/j.1468-0084.2007.00477.x
- Xiuwu, Z., Zhangmin, H., & Sihan, J. (2022). The impact of two-way FDI on total factor productivity in China and countries of the belt and road initiative. Economic Research-Ekonomska Istraživanja, 35(1), 2868–2888. https://doi.org/10.1080/1331677X.2021.1984268
- Yameogo, C. E. W., & Omojolaibi, J. A. (2021). Trade liberalisation, economic growth and poverty level in sub-Saharan Africa (SSA). Economic Research-Ekonomska Istraživanja, 34(1), 754–774. https://doi.org/10.1080/1331677X.2020.1804428
- Zhang, W. W., Dawei, W., Majeed, M. T., & Sohail, S. (2022). COVID-19 and international trade: Insights and policy challenges in China and USA. Economic Research-Ekonomska Istraživanja, 35(1), 1991–2002. https://doi.org/10.1080/1331677X.2021.1930091