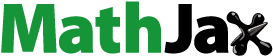
Abstract
Sustainable entrepreneurship is essential for solving the issue of economic and social stability. This paper is about the determinants of entrepreneurship among green innovation and strategic innovation. The research studies the communication and R&D variables that impact entrepreneurship development. The literature shows that green innovation is vital in developing strategic orientation. Entrepreneurship must work on R&D to gain economic and social stability. Entrepreneurship development is a dependent variable, and communication and R&D are independent variables. This paper evaluates the performance of Multiple Linear Regression (MLR) and the Artificial Neural Network Regression (ANN) approach for estimating entrepreneurship development (ED) in China from 2000 to 2021. For this work, nine independent variables related to entrepreneurial development were selected, which include CO2 emissions (CO2), GDP growth (GDP), Total greenhouse gas emissions (GHG), Computer, communications, and other services (CCS), Nitrous oxide emissions (NO2), Personal remittances, received (PRR), Research and development expenditure (R&D), New businesses registered (NBR), and patents in environment-related technologies (PERT). Data were gathered from the World Bank (WB) official data bank portal. The highest correlation (−0.85 and −0.71) with ED was observed with GHG, followed by a strong correlation with R & D. The MLR model generated 0.78 R2 (error = 0.26) whereas the ANN model produces 0.83 R2 (error = 0.01). The results of the ANN showed that the association among parameters is strong. Ten input variables and 15 hidden neurons were used to estimate the dependent variable. The process took 87 steps, and the optimum result had a Sum of Square Error of 0.001.The top 5 essential variables are NO, NBR, GHG, R&D, and CO2. The least essential variables are PPR and CCS, respectively, with the lowest variable importance (VI) score. This work is unique in the sense that it covers the research gap with new advanced techniques (ANN) applied to time series data. We believe the idea that we developed is a novel approach for social scientists, entrepreneurial development, social stability, and a sustainable environment. The findings of this research can benefit entrepreneurs in many different ways. By understanding the effects of social environmental stability on their success, entrepreneurs can better tailor their strategies to ensure they get the best possible results.
1. Introduction
The importance of sustainable entrepreneurs in solving today’s social and environmental issues is growing. These socially and environmentally conscientious people play a crucial role in society because they provide solutions to complicated societal problems that governments, established corporations, or civil society organizations either neglect, fail to address, or do not even exist. However, there is a lack of knowledge regarding sustainable entrepreneurs. For instance, there is still much to learn about how sustainable entrepreneurs launch their enterprises and deal with challenges at the beginning. Unlike typical business owners, sustainable entrepreneurs encounter unique difficulties when starting their enterprises. The difference between personal and societal value production may be the root of these difficulties. Companies are increasingly dealing with natural resource restrictions and rising expenses due to environmental deterioration as a result of responding to globalization and the problems of climate change. Businesses must address these ecological challenges to grow internationally and move toward environmental sustainability based on sustainable entrepreneurship (Islas-Moreno et al., Citation2021).
To achieve sustainable green development in emerging economies, we seek to understand the role of environmental entrepreneurship. Quite a broad concept, sustainable development encompasses not only long-term economic growth but also environmental preservation for present and future generations, as well as changing society’s social and economic orientation by promoting gender and economic equality (Graff Zivin & Neidell, Citation2013). As a result, environmental and empirical researchers are looking for variables that can aid in achieving sustainable development goals. Recent years have seen increased environmental entrepreneurship as a significant aspect of pursuing sustainable development goals. Entrepreneurs’ perspectives have evolved, especially in industrialized nations, and they are now considering the effects on the environment (Lenihan et al., Citation2019).
Sustainable entrepreneurship practice with an emphasis on ethics, responsibility, and society. Sustainable business practices strike a balance between resource conservation and profit production. It is crucial to have a tremendous environmental impact by combining social and environmental benefits from implementing resource efficiency programs (Rus-Casas et al., Citation2020). Additionally, it must accomplish with an ethical mindset that prioritizes social advantages; in other words, by luring in human potential, cooperating as a team, putting the relevant human rights laws into effect, and supporting inclusionary policies. The aim is to achieve a favorable equilibrium that maintains the market’s stability (Chege & Wang, Citation2020).
The rise of entrepreneurs over the past ten years has substantially affected the global economy. They contributed by funding R&D and using it to produce goods and services that the society needed, as well as by, among other things, resolving environmental conflicts and creating job possibilities. Both economic progress and quality of life depend on research and development (R&D) of creative activities to generate new goods and services or enhance existing ones. It leads us to conclude that while knowledge in and of it is vital for the execution of an idea’s development, there are instances when it is insufficient due to the usage of resources and technology. Inventors must consider how intellectual property laws protect their unique goods or services. It could aid them in converting their creativity into competitiveness, particularly in technology businesses where significant financial investments are required and discoveries are created daily (Islas-Moreno et al., Citation2021).
Entrepreneurship must be on green or ecological values to achieve environmental sustainability. The relationship between environmental values and sustainability entrepreneurship has been the subject of some theoretical studies. Entrepreneurs have launched businesses that include sustainable development in their plans as new environmental opportunities have surfaced due to escalating ecological concerns. The phrase ‘sustainable entrepreneurship’ is similar to green entrepreneurship or strategic orientation because it is sometimes mistaken for social and general entrepreneurship. Sustainable entrepreneurship is the process by which an entrepreneur achieves their goal of developing a business while considering the enterprise’s sustainability by structuring this process so that many economic, social, and ecological angles discuss (Ilonen, Citation2021).
However, the sustainable entrepreneurial intention is the cornerstone for transforming incentives into actions that could foretell sustainable entrepreneurial behavior. In this light, it is crucial to investigate the degree to which environmental values and sustainable entrepreneurial intent are related. According to the firm’s natural resource-based approach, companies should actively work to enhance and harmonize their interactions with the surrounding environment. The three different yet connected environmental methods of pollution reduction, product stewardship, and sustainable development can help them achieve this. Green innovation aims to make existing goods and procedures more eco-friendly. Green innovation can take many forms, including using environmentally friendly raw materials and avoiding waste. According to eco-design principles, the goods lower carbon emissions and footprints and consume less water, electricity, and other natural materials. Organizations may also pursue exploration-based green innovation, which focuses on creating fundamentally new methods and tools that let businesses have a beneficial impact on the environment and engage in sustainable practices, in addition to exploitation-based green innovation (Santosaa et al., Citation2020).
Sustainable business owners seek to balance their economic, environmental, and social objectives. The payment includes economic and non-economic improvements to individuals, the economy, and society (Prendes-Espinosa et al., Citation2021).
Sustainable entrepreneurship is being identified as the link between business and sustainable development to achieve the UN's sustainable development goals. In that sense, sustainable entrepreneurs are also significant actors and innovation drivers in shifting to a more circular economy through partnerships with bigger businesses, incorporating outside dynamic skills, or green technologies. In modern local and global economies, entrepreneurs play a significant role in development and progress. To enhance living standards and maintain product innovation, which promotes economic growth globally, entrepreneurial activities are required. Without efficient learning and business support capabilities, entrepreneurship will not grow sustainably in today’s society’s complex and demanding environment (Ferreira & Teixeira, Citation2019).
Economic issues neglect social prerequisites, and the monetary emergency progressively affects human culture and the typical environment. Thus, there is a contention between financial development and environmental protection, which inconveniently affects feasible events. These worries, credited to the local business area and foundation as entire organizations, rouse the inclination to make new action plans and social advancement (Zvavahera et al., Citation2018). Both new firms gave regular and biological assets and laid out organizations that embraced natural administration approaches or clean assembling strategies that could arrange as green business venture substances. Both require experts with dark green information. Both arising and created nations are similarly impacted by the absence of profoundly talented and experienced specialists, a boundary to the green economy, and the circumstance has not been significantly better. For rising countries, an absence of specialists sand an overall lack of college graduates, especially those with preparation in science, innovation, design, and math (STEM), have been issues (Mardani Shahrbabak & Solati, Citation2020).
Economic development is a reality regarding progressing financial and specialized improvement because of expanded economic development, concentrating on the social, political, and financial information spaces (Moridsadat & Moamelvand, Citation2020). Numerous areas have embraced research regularly upheld by colleges to help advance and address these issues. New reasonable materials, eco-accommodating conveyance organizations, roundabout monetary models, low-discharge item fabricating processes, enhancements for overseeing strong waste, and the charge of light-obligation and traveler vehicles are only a few of these accomplishments. It is critical to consider how they could impact a business visionary’s craving to change to a green business considering these mechanical improvements at the company and current levels in a robust climate and the open market doors (MOs) achieved by natural worries (Rosa et al., Citation2021).
On the one hand, a globally connected and fiercely competitive market necessitates a more strategic stance, forcing businesses to develop clear strategies to preserve their competitive advantage. On the other hand, customers demand unique new items, quality, and safety. Companies are beginning to focus on sustainability as a growth strategy in response to pressure from customers and the business world. A social system as a whole move higher in development thanks to organizations. It is characterized by induced and implemented exogenous aspects, such as governmental policies and laws, and endogenous economic and non-economic forces. The concept of development is multifaceted and includes factors related to the economy, environment, culture, society, and politics (Kanani & Gelard, Citation2019).
Environmental elements include entrepreneurship funding, public policy, entrepreneurship education, R&D transfers, commercial and legal infrastructure, internal market dynamics, entrance regulation, physical infrastructure, and cultural and social norms. The many components that make up each environmental condition and their varying values across different nations are the primary factors in the emergence and success of new firms. The effects of these variables on the entrepreneurial intention function will be the focus of our main research interest, which will cover in more detail below. Inventors must consider how intellectual property laws safeguard their unique products or services. It could help them become more competitive, especially in the technology sector, where significant financial investments are necessary, and discoveries are made daily (Liu, Citation2021).
Additionally, for the Entrepreneur, three various types of intellectual property, including patents, trademarks, and copyrights, said that ‘intellectual property is an asset for its owner and has a financial value attached to it’. Because it is an asset that can be bought and sold, it is a fact that this sort of protection is quite expensive and may take a long time due to all the paperwork. Entrepreneurs should perform a cost-benefit analysis, wrote an article for the Entrepreneur, since ‘for the value acquired, for example, launching a firm swiftly, the financial and other costs of doing it may transcend the benefit’. It leads us to conclude that entrepreneurship will be encouraged more by a cheap and speedy transfer of R&D than by an expensive and prolonged (Grant, Citation2018).
Entrepreneurs continuously strive to enhance their communication skills since doing so will help them share their ideas, convey them clearly, and work more effectively with their team, clients, and colleagues. They know the importance of communication in business and give themselves the most excellent advice to make their everyday encounters productive. An entrepreneur will benefit from practical communication skills while explaining projects, giving elevator pitches, giving presentations, providing training, and in various other situations. A person who can communicate well can quickly advance in their work. Every business must possess effective communication as a prerequisite to experiencing success quickly. Businesses and entrepreneurs frequently have trouble realizing their full potential when poor communication skills exist. Most individuals downplay the value of learning technological skills, which could hurt a company’s capacity to make money. On the other hand, good communication benefits a company greatly, impacting internal and external relationships (Al-Kumaim et al., Citation2020).
By introducing fresh concepts and experiences, effective communication fosters innovation and creativity within the company. Healthy dialogue and exchange of ideas enhance cognitive abilities by revealing the full range of the invention. Employee productivity soars as a result of creativity, which also motivates them to handle their work more effectively. Regardless matter the size of the organization, efficient communication has endless benefits. To improve the viability and future of their companies, entrepreneurs should identify the areas where communication training is needed and work on them immediately (Khotimah & Ruyani, Citation2021).
Sustainability entrepreneurs widely acknowledge as moving forward in the face of growing worries about global warming, climate change, and its severe environmental repercussions. In today’s debates, sustainable businesses prioritize addressing fundamental requirements while minimizing human ecological footprints on product markets (Kong et al., Citation2020). Therefore, for aspiring entrepreneurs to raise productivity, quality, and market value and fulfill the hopes and objectives of the rural community, it is essential to acknowledge sustainable firms’ creative practices in exchanging professional information.The core ideas of sustainable entrepreneurship and innovation ethics act as cornerstones for developing business solutions to the most persistent social and environmental problems facing the top sectors in the future (Sargani et al., Citation2021).
Contrarily, sustainable businesses enable others to explore their ideas and solutions by giving them access to inputs and knowledge about environmentally friendly resource management techniques, such as increasing soil fertility, conserving water, and using integrated pest management techniques. However, proponents of entrepreneurship claim that it may aid in the fight against climate change, lessen erosion and environmental scarcity, boost freshwater supply and agricultural output, protect biodiversity, and protect habitats. These initiatives will significantly impact both established and developing nations, increasing their socioeconomic stability, sustainable economies, chances for entrepreneurship, and self-reliance in the long run.
1.1. Research question
RQ1: How does environmental stability impact on entrepreneur development of a documentary?
RQ2: What determinants of entrepreneurial development strengthen the relationship between green innovation and strategic orientation?
RQ3: What is the role of research and development and communication in the entrepreneur development of a documentary?
2. Literature review
The French etymology of the word ‘entrepreneur’ might do translated as ‘taking the initiative to bridge’. Entrepreneurship is when businesspeople recognize and take advantage of new products, methods, or markets to turn creative ideas and resources into lucrative opportunities. Moreover, the business venture can bring about various outcomes in different arrangements, so cultivating economic development, growing work potential open doors, and resolving ecological issues. The green business venture is one of the many sorts that coordinate natural, social, and financial objectives. It satisfies the need for the economic turn of events (Parker, Citation2018).
Furthermore, a developing number of individuals presently acknowledge green utilization, giving entrepreneurs more freedom to lay out green undertakings. Be that as it may, green businesses should handle conventional monetary issues while focusing on social obligations and natural worries to find success. Therefore, green business is open to entrepreneurs with genuine concerns, social commitments, and abilities (Frederick et al., Citation2018).
General society is turning out to be more tolerant of green utilization. In this manner, numerous Western legislatures have given green business ventures a high need in their monetary strategy plan. Business visionaries are encouraged to seek green undertakings that balance ecological, social, and economic objectives. Moreover, the ‘green business-person’ envelops social obligations, environmental worries, and green abilities rather than the ‘customary business- person’. Business-people in the green business should battle with unfortunate adaptability and extensive venture recompense periods, which deter loan specialists from offering to fund—extra costs to a green business visionary’s cutthroat detriment. The green business venture is by an absence of exceptionally qualified and experienced individuals.
Notwithstanding how green business people should adjust monetary exercises, social and environmental elements, and honest convictions, the number of green organizations has consistently extended universally. It is critical to investigate the variables that rouse business people to switch entirely to green strategic approaches (Igielski, Citation2021).
The business venture is a convoluted human capacity due to conditions. The green business is a significant, complex idea that involves many issues, like financial, social, and ecological objectives. Thus, the ongoing review recognizes the push, pull, and mooring components that impact business and people’s exchanging conduct toward the green business venture. Thus, the ongoing study identifies the inspiration, pull, and securing factors of administrations or associations to meet the natural market and open doors as the elements that propel business visionaries to change to a green business venture. It proposes that organizations gain the upper hand through proactive ecological positions (Galindo-Martín et al., Citation2020). Green business venture is the most common way of recognizing, evaluating, and having business prospects established on maintainable, naturally cordial, and green standards, as per.
Moreover, green business venture alludes to the cycle by which business-people turn thoughts for items, innovation, and administrations into reality while accentuating natural protection and cultural government assistance. It consolidates good turn of events, which should consider the ‘triple primary concern’ of the climate, society, and financial matters. Green business should be visible as a social action that tries to save the indigenous habitat rather than forming a direct interaction.
Furthermore, a developing number of individuals presently acknowledge green utilization, giving entrepreneurs more freedom to lay out green undertakings. The green business should handle conventional financial issues while focusing on social obligations and natural worries. Subsequently, the green business venture is open to entrepreneurs with genuine concerns, social commitments, and green abilities (Tambovceva et al., Citation2019).
A person, in general, is turning out to be more tolerant of green utilization. In this manner, numerous Western states have given the green business a high need in their strategic financial plan. Business visionaries are encouraged to seek green undertakings that balance ecological, social, and monetary objectives. Likewise, the expression ‘green business-person’ envelops social obligations, natural worries, and green abilities rather than the expression ‘ordinary business visionary’. People in the green business should fight with unfortunate adaptability and extended speculation restitution periods, which deter banks from offering support (Demirel & Danisman, Citation2019). An extra cost to a green business-person’s cutthroat detriment, green business is an absence of profoundly qualified and experienced individuals. Regardless of how green business-people should adjust financial exercises, social and environmental factors, and biological convictions, the number of green organizations has consistently extended worldwide. Investigating the variables that inspire business-people to change over completely to green strategic approaches is essential. The business venture is a muddled human capacity inferable from an intersection of conditions (Ionescu et al., Citation2020).
The green business venture is a significant, multi-layered idea involving many financial, social, and ecological issues. Business-people support green organization projects that balance ecological, social, and monetary points. Moreover, rather than ‘customary business visionary’, the expression ‘green business visionary’ joins social obligations, natural worries, and green gifts. Green business-people have low adaptability and extended speculation restitution times, discouraging banks from giving capital. Extra expenses have a severe hindrance to green business visionaries. A deficiency of profoundly qualified and experienced representatives often hinders green business (Mathushan & Pushpanathan, Citation2020). Through excellent communication, entrepreneurs develop their businesses to the necessary levels of perfection. Entrepreneurs should be charismatic team organizers who can express their ideas effectively. A charismatic leader will benefit both the organization and the results of their followers. There is enough research to show that charismatic or transformative leadership directly affects followers’ attitudes, behaviors, and performance. Charismatic leadership’s capacity to effectively convey the vision is one of the essential characteristics. To qualified individuals, bring together into an effective team or teams for diverse reasons, the idea must be communicated verbally or in writing. These groups could include VPs or investors, employees or followers, and other stakeholders (Triantafillidou & Tsiaras, Citation2018).
Entrepreneurial thinkers give the transmission of the Entrepreneur’s vision to new venture teams a top priority. The most crucial task for transformative leaders is effectively conveying their vision to their followers. If effectively said, compelling images give employees a feeling of direction and boost commitment. Communication is essential when looking for company ventures and entrepreneurship. It must also play a critical role in imagining venture ideas, and persuading stakeholders of a venture’s potential must also be acknowledged. It is crucial to remember that language is in spoken and nonverbal communication (Muchunku, Citation2020). It should go without saying that the term ‘language’ refers to both verbal and non-verbal forms of communication. The ability to effectively use verbal and nonverbal clues in written and oral expressions in this context is ‘communication’. Due to this, businesspeople will need to master effective communication techniques and general communication skills. With the help of partners and other stakeholders, an entrepreneur would go through several rounds of discussion and negotiation to address any conceptual issues with their goal. Entrepreneurs and stakeholders can comprehend the advantages and disadvantages of the endeavor through communication. A complete understanding of the upcoming project is crucial for the stakeholders. It gives a business owner a chance to hear from a stakeholder’s feedback and decide whether or not they accept potential (Tohidyan Far & Rezaei-Moghaddam, Citation2019).
From an entrepreneurial perspective, communication should serve as an interaction that requires cultivating and using communicative skills, which can be innate and learned. Its lack or faulty presence accounts for certain teachers’ incapacity or challenges. Some of them possess highly technical information but also the capacity to put it into practice and translate it didactically. That is the ability to understand what, how, when, in what way, conditions, and to whom they can transfer knowledge. Communication is a continuous automated circuit rather than information primarily concerned with set norms. In contrast to intelligence subordinated to the necessary teaching capacity, intelligence used in communication is highly strategic (Gontareva et al., Citation2018).
Economic, entrepreneurial, and strategy research traditions developed over many decades are the foundation of strategic entrepreneurship. Strategic entrepreneurship is the first concept to explicitly describe the fusion of the knowledge and issues of both fields, creating a new area that crosses them. The study is on the relationship between entrepreneurship and strategic management. Processes of global economic development have altered the sports industry and turned teams into profitable businesses that now play a significant role in the active leisure sector. Companies that have undergone a commercial legal metamorphosis compete on many levels in the sporting and business spheres in this category of sports firms in Europe. This group of companies was given the moniker ‘sports sector’ and generated and consumed activity worldwide. A sports enterprise is a for-profit, service-based business that has received legal permission to conduct business in the sports industry and promote physical culture (Rosário et al., 2021).
For many years, academic research has been on entrepreneurial activity and orientation in residence halls. A company’s entrepreneurial approach pursues development or value creation through new businesses inside the organization’s framework resulting in green and corporate entrepreneurship. The management idea of entrepreneurship emphasizes the importance of identifying the factors generating commercial activity as a sort of driving force of this activity. The essence of entrepreneurship is the creation and expansion of a business venture. In contrast, strategic management is the search for a competitive edge and maintenance of this firm endeavor in a challenging environment (Kim-Soon et al., Citation2018).
Competitive advantage is the main requirement for success in this respect since strategic management aims to grow the wealth of the business and its owners. It prevents competitors from finding and appropriating their unique resources; companies must first position themselves in the market (Kalisz et al., Citation2021).
The emergence of a knowledge-based society has pushed for change in how economies operate, and innovation has emerged as the key to a new era of global competition. The creation of a national plan and innovation are related. Deepening the reform of entrepreneurship and innovation education in universities and nurturing creative and entrepreneurial talent are the driving forces behind increasing the nation’s capacity for innovation (Berman et al., Citation2022).
The education developed in these areas encourages innovative business owners and fosters innovation in the nation’s economy, employment, and other social issues. For one to succeed in the global competitiveness of the knowledge society, innovation and entrepreneurship are necessary. In addition to being a global trend in higher education, developing innovation and entrepreneurship education and cultivating innovative enterprise talent are internal driving forces behind China’s development of an entrepreneurial economy, an innovation-driven national strategy, and an innovative nation. The entrepreneurial economy serves as the primary engine for economic growth and is not simply a necessary internal component of industrial structure optimization and adjustment (Bradley et al., Citation2021).
Innovation is the norm, and entrepreneurship and entrepreneurial activities are its primary production elements. Small and medium-sized businesses, mainly acting as the source of power, are crucial to the entrepreneurial economy. SMEs are ‘machines for creating jobs’ and contribute significantly to the economic growth of all nations today. Young entrepreneurs, who receive systematic entrepreneurship education in higher education to support startups, are the new source of power for SME entrepreneurs(Islam & Abd Wahab, Citation2021). Based on the literature and the aim of this paper the conceptual framework is presented in .
3. Material and methods
3.1. Data collection
The World Bank collects and publishes data for research and academic writing. The World Bank collects, analyzes, and disseminates data related to economic development and poverty. Their data can help students and researchers do their research and academic writing. The World Bank enriches the data on its website daily (Zhongming et al., Citation2020). By using the data, researchers and students can make better-informed decisions. The World Bank collects and publishes data for research and academic writing (Business, Citation2020; Diaz, Citation1998; Zhongming et al., Citation2020). The World Bank collects, analyzes, and disseminates data related to economic development and poverty. Their data can help students and researchers do their research and academic writing. By using the data, researchers and students can make better-informed decisions (Business, Citation2020). The World Bank data can be used for academic research. presents a summary of data acquisition collected for ED estimation.
Table 1. Summary of data collected for ED analysis.
3.2. Methodology
3.2.1. Summary statistic
Summary statistics summarize some of the information given about the information. Describe the data in some useful way. A common use is central tendency statistics, which are the values that are the best middle numbers in a database like mean, median, mode, and range (Amrhein et al., Citation2019). Variability measures, or the spread of the data, can be compared within a group, and across groups. A good statistical summary is essential for data analysis and gives the user insight more easily, quickly, and effectively extracting data trends and patterns. The two types of summary statistics are descriptive (i.e., Characteristic), and inferential (i.e., Relation), and they’re intended for different purposes. Descriptive statistics are vital for describing your data, such as average, median, mode, quartile, and percentiles. They can be measured against other quantities within and between data sets (Mishra et al., Citation2019).
3.2.2. Correlation analysis
Correlation Analysis is a statistical approach that allows you to measure the strength and direction of the relationship between two or more variables. The purpose of this technique is to identify which factors are most likely to influence the occurrence of a particular event. There are many different applications for correlation analysis, but it is most commonly used in business and marketing contexts (Sheng et al., Citation2019). For example, a company might use this approach to determine which factors are most likely to influence sales or profitability. By understanding the relationship between different factors, businesses can make more informed decisions about how to allocate their resources. There are many different applications for correlation analysis, but it is most commonly used in business and marketing contexts (Gauthier et al., Citation2020). For example, a company might use this approach to determine which factors are most likely to influence sales or profitability. By understanding the relationship between different factors, businesses can make more informed decisions about how to allocate their resources. It is used to determine whether two or more numeric aspects of a dataset are related in some way. In R programming, the ‘Base’ package library was used to make correlation analysesbetween dependent and independent variables.
3.2.3. Multiple linear regression (MLR)
Multiple linear regression is a statistical technique that uses several explanatory variables to predict the outcome of a response variable. The goal of multiple linear regression is to model the relationship between the explanatory variables and the response variable. The coefficients of a multiple linear regression model can be interpreted as the estimated effect of each explanatory variable on the response variable (Wang et al., Citation2018; Zhu et al., Citation2019). The intercept term represents the estimated mean value of the response variable when all explanatory variables are set to zero. The significance of each coefficient can be tested using a t-test. The null hypothesis for each coefficient is that the true coefficient is zero. If the null hypothesis is rejected, then there is evidence that the corresponding explanatory variable has a significant effect on the response variable (Rasp et al., Citation2020; Rustam et al., Citation2020; Wang et al., Citation2018; Zhu et al., Citation2019). The overall fit of a multiple linear regression model can be assessed using various measures, such as R-squared and adjusted R-squared. R-squared measures the percentage of variability in the response variable that is explained by the model. Adjusted R-squared adjusts R-squared for the number of explanatory variables in the model. Multiple linear regression is a powerful tool for studying relationships between multiple explanatory variables and a response variable. It can help us understand how different factors influence the outcome of interest and can be used to make predictions about future outcomes.Multiple regressions involve an array of independent variables and a single dependent one (Dimitriadou & Nikolakopoulos, Citation2022; Wong et al., Citation2020). It can be expressed mathematically as:
(1)
(1)
In the preceding equation, y represents the mean response, and I is a variable that causes y to fluctuate. To be consistent with the same standard deviation, we suppose that the observed values of y deviate from their means y by the same amount (Balarabe & Bery, Citation2021; Dimitriadou & Nikolakopoulos, Citation2022; Scala et al., Citation2022).
3.2.4. Artificial neural network analysis (ANN)
An artificial neural network (ANN) is a computational model that is inspired by the way biological neural networks work. ANNs are used to estimate or approximate functions that can depend on a large number of inputs and are generally used to solve problems in computer vision and machine learning (Ding et al., Citation2022; Olden et al., Citation2004). ANNs are composed of a large number of interconnected processing nodes, or neurons, that each performs a simple mathematical function. These nodes are interconnected in a way that allows them to pass information between each other. The output of each node is determined by a set of weights that determine the strength of the connection between the nodes.The overall performance of an ANN depends on how well the weights are tuned to the training data (Das, Citation2018). The process of tuning the weights is known as training the network. Once the network has been trained, it can be used to make predictions on new data.ANNs are powerful tools for making predictions but they are not perfect. One of the limitations of ANNs is that they can be difficult to interpret. This is because the weight values learned by the network can be difficult to understand.Despite their limitations, ANNs are very successful at a variety of tasks including image recognition, speech recognition, and predicting financial markets (Araghi et al., Citation2019; Goh, Citation1995; Radojević et al., Citation2019).We applied Artificial Neural Networks (ANNs) method to analyze the dependent variable due to multiple advantages. The use of ANNs in data analysis has become increasingly popular in recent years due to the many advantages they offer. ANNs are computer algorithms that are inspired by the biological neural networks of the human brain and allow machines to learn from past experiences and make predictions (Sengupta et al., Citation2020; Zhang et al., Citation2019). They are used to find patterns and relationships within data sets, making them an invaluable tool for data analysis.One major benefit of ANNs is their ability to recognize patterns in data sets. ANNs can identify intricate correlations and interactions between features. This allows them to uncover relationships that might otherwise go unnoticed by traditional data analysis methods. Moreover, ANNs can capture non-linear relationships between features, meaning that complex phenomena can be modeled with high accuracy (Abduljabbar et al., Citation2019; Abiodun et al., Citation2018). Another great advantage of using an ANN for data analysis is its ability to identify non-obvious trends or anomalies in a dataset. ANNs can detect minute changes or irregularities in data that may not be obvious to the human eye. This makes them particularly useful in situations where a quick response is needed.Furthermore, ANNs can quickly process large datasets without needing a lot of computational resources. This makes them an attractive choice for businesses dealing with huge amounts of data regularly.Finally, ANNs are highly customizable and versatile. They can be tailored to suit the specific needs of different applications and industries, making them a great choice for businesses looking for a comprehensive data analysis solution (Abduljabbar et al., Citation2019; Abiodun et al., Citation2018; Sengupta et al., Citation2020; Zhang et al., Citation2019).
3.2.5. Variable importance analysis (VIA)
In artificial neural networks (ANNs) regression, one way to measure the importance of a particular node or connection weight is through the use of a technique called variable importance analysis (VIA). This technique can be used to determine which inputs are most important for the model to function properly (Ishak, Citation2016). There are a few different ways to calculate VIA, but one common method is to randomly perturb the values of the inputs and then measure the change in output. The more a particular input value changes the output, the more important that input is considered to be. another popular technique for calculating VIA is known as leave-one-out cross-validation (LOOCV). This approach iteratively removes one input from the model and then measures the change in performance (Ishak, Citation2016; Ruß & Brenning, Citation2010). The input that results in the largest decrease in performance is considered to be the most important. Both of these methods have their pros and cons, but they can be useful for understanding which inputs are most important to an ANN. Additionally, VIA can be used as a way to select which inputs to include in a model when there are a large number of potential inputs (Chehreh Chelgani et al., Citation2018; Hadavandi & Chelgani, Citation2019).
3.2.6. Model accuracy assessment
The three most commonly used measures for assessing the performance of a regression model are R squared (R2), the Root Mean Square Error (RMSE), and the R-value. R2 can be interpreted as the percentage of the variance in the dependent variable that is explained by the model. A value of 0% indicates that the model explains none of the variances, while a value of 100% indicates that the model explains all of the variances. In general, a higher R2 value is better (Nguyen et al., Citation2020). The RMSE is a measure of how close the predicted values are to the actual values. A low RMSE value indicates that the model is performing well. The R-value is a measure of how well the model fits the data. A high R-value indicates that the model fits the data well. When interpreting these measures, it’s important to keep in mind that they can be affected by factors such as overfitting, underfitting, and outliers. Therefore, it’s important to look at all three measures together to get a complete picture of how well the model is performing (Nguyen et al., Citation2020).
4. Results
4.1. Entrepreneurship development (ED)
China has experienced rapid development in entrepreneurship since 2000. This is mainly due to the government’s initiatives to encourage entrepreneurship and support the development of small and medium-sized businesses. The number of newly registered businesses has been growing rapidly, from 1.14 million in 2000 to 17.24 million in 2016. The total number of registered businesses reached 42.94 million by the end of 2016, up from 6.72 million in 2000 (Li et al., Citation2019; Lin et al., Citation2021; J. Liu et al., Citation2019; Wang et al., Citation2020). Over the past 17 years, entrepreneurship has developed into an important engine driving China’s economic growth. demonstrates ED (employment %) progress journey during the last 22 years. It is worth noting that the percentage of total employment has decreased since 2000.
4.2. Statistical summary
In data analysis, a statistical summary is a concise description of a set of data. It includes important measures of central tendency and variability, as well as the shape and distribution of the data. A good statistical summary allows you to quickly get a sense of the characteristics of a data set so that you can focus on the interesting parts.The basic statistics were calculated for CO2, GDP, GHG, CCS, NO, PRR, R & D, NBR, PERT, and ED. The statistical summary shows that the ED has a minimum % of 44.66, a maximum of 61.91, and an average of 51.83. The 25% data, the first quartile, has 47.08%, whereas the 75th percent of the data has a % of 56.70. The detailed summary statistics of all variables are listed in .
Table 2. Summary statistics of variables.
4.3. Correlation analysis
Correlation Analysis provides the estimate of a linear relationship between two variables. It represents the strength and direction of the relationship between a dependent and independent variable.In order to understand and interpret this conclusion, we would carry out a regression analysis by summarizing and interpreting the underlying concepts and assumptions that were made in the example above.To ensure that your understanding and interpretation were accurate, you would have confirmed that the model assumptions were correctly made before the analysis. presents the correlation matrix of all selected variables, which includes CO2, GDP, GHG, CCS, NO, PRR, R & D, NBR, PERT, and ED. The highest correlation (-0.85 and −0.71) with ED was observed with GHG, followed by a strong correlation with R & D. As for independent variables, the highest positive and negative correlations were observed among various variables. For example, 0.69 between CO2 and NO, 0.68 between CO2 and R & D. A low correlation (-0.01) between PERT and PRR was also observed.
Table 3. Correlation matrix of variables.
4.4. Multiple linear regression (MLR)
In statistics, linear regression is used to model the relationship between a set of independent variables and a dependent variable. Linear regression can be used to predict future values of the dependent variable, based on the values of the independent variables. Multiple linear regression is an extension of linear regression, used when there are multiple independent variables. In multiple linear regression, the goal is to find the best predictor variables (the independent variables that are most associated with the dependent variable) and to use these predictor variables to create a model that can be used to predict future values of the dependent variable. We calculated MLR to ass the influence of contributing parameters on ED. Results of the MLR model are presented in , in which estimated coefficients, Std. Error, t-value, and p-value are given. The Residual standard error for this model is 0.26 on 9 degrees of freedom along with R2 of 0.78, F-statistic 1145.50, and p-value of 0.000. The R2 of 0.78 states that the contributing parameters explain 78% variation in this model.
Table 4. MLR model statistics.
4.5. ANN analysis
As mentioned in the methodology section, an artificial neural network (ANN) is a computational model that is inspired by the way biological nervous systems, such as the brain, process information. The key element of an ANN is its connectivity, which reflects the degree of interconnection between the artificial neurons, or nodes. An artificial neural network can be either fully connected, meaning that each node is connected to every other node in the network, or sparsely connected, meaning that only some nodes are connected (Ameer et al., Citation2019; Russo et al., Citation2013). The nodes are interconnected in a pattern that resembles either a feedforward network, in which information flows in one direction from input nodes to output nodes, or a feedback network, in which information can flow in both directions. listed ANN model selection statistics in which details of hidden neurons, the threshold value of 0.01, repetitions, steps, and errors are provided. The best model based on minimum error (0.001) is bold and italic in the table.
Table 5. ANN Model selection statistics.
ANN Regression Model illustration with inputs, hidden layers, and output is shown in , with ED as the dependent variable. Each input vector has multiple parameters, and each dependent variable uses different neurons in the input layers based on the input. In addition, the output layer contains only one neuron, representing the estimated ED condition. The datasets for training the ANN were collected between 2000 and 2021 and analyzed for the dependent variable. The optimal combination of the transfer function for hidden and output layers was obtained by varying the neurons in hidden layers. Among these possibilities, the highest correlation coefficient and lowest RMSE were determined (Ahmad et al., Citation2019). Ten input variables and 15 hidden neurons were used to estimate the dependent variable. The process took 87 steps, and the optimum result had a Sum of Square Error of 0.001.
Figure 3. ANN model. I is input, B is the bias term in the model, H is hidden neurons and O is the output of the model. Red lines are positive coefficients and black lines are negative coefficients.
Note: CO (CO2 emissions (metric tons per capita)); GDP (GDP growth (annual %)); GHG (Total greenhouse gas emissions (kt of CO2 equivalent)); CCS (Computer, communications and other services (% of commercial service imports)); NO (Nitrous oxide emissions (thousand metric tons of CO2 equivalent)); PRR (Personal remittances, received (% of GDP)); R & D (Research and development expenditure (% of GDP)); NBR (New businesses registered (number)); PERT (Patents in environment-related technologies); ED (Entrepreneurship development)
Source: Authors Construction.
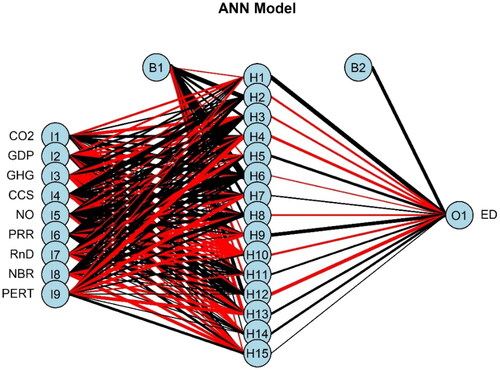
In recent years, Artificial Neural Networks’ (ANNs) ability to analyze complex datasets and generate meaningful insights, ANNs have been embraced by many businesses, governments, and other organizations for a variety of applications. One of the most prominent examples is from Microsoft, which recently announced its Project Brainwave platform which uses ANNs to speed up artificial intelligence operations. Project Brainwave enables the computer hardware to handle deep learning models in real-time, allowing organizations to process massive amounts of data faster and more efficiently than ever before (Abiodun et al., Citation2018; Antonopoulos et al., Citation2020). The technology has already been implemented in the company’s cloud computing products such as Azure and Office 365. The US military has also taken advantage of the capabilities offered by ANNs for data analysis. In 2019, the US Army announced that it would be using AI-based systems to process and analyze data from drones and other sources to improve battlefield decision-making. This system, dubbed Project Maven, is expected to help military personnel make informed decisions based on the insights generated from vast amounts of information. Another example of an organization utilizing ANNs for data analysis is Amazon (Khraisat et al., Citation2019; Tamiminia et al., Citation2020). The company’s Alexa AI assistant has become increasingly advanced over the years, leveraging the power of ANNs to provide users with an intuitive interface and a comprehensive understanding of their natural language requests. As a result, Alexa has become an indispensable tool for customers, giving them instant access to information and making their lives easier. Finally, ANNs are also being used for healthcare applications. For instance, a team of researchers at the University of Cambridge developed an AI system that can identify patterns in health records to predict a patient’s risk of developing cardiovascular disease. By leveraging the power of ANNs, this system can accurately assess risk levels and provide actionable insights to clinicians and patients alike (Abduljabbar et al., Citation2019; Antonopoulos et al., Citation2020; Huang et al., Citation2020).
4.6. Variable importance in ANN model (VIP)
VI is calculated using the Garson method by detecting all weighted connections between the nodes of interest. On the other hand, Olden’s approach takes the total of the raw connection weights between each input and output neuron and multiplies it by the number of hidden neurons (Garson, Citation1991). In numerous simulations, this has been demonstrated to outperform the Garson technique. An illustration of variable importance in the ANN model is presented in . It is worth noting that the top 5 essential variables are NO, NBR, GHG, R&D, and CO2. The least essential variables are PPR and CCS, respectively, with the lowest VI score.
Figure 4. Variable importance in ANN model.
Note: CO (CO2 emissions (metric tons per capita)); GDP (GDP growth (annual %)); GHG (Total greenhouse gas emissions (kt of CO2 equivalent)); CCS (Computer, communications and other services (% of commercial service imports)); NO (Nitrous oxide emissions (thousand metric tons of CO2 equivalent)); PRR (Personal remittances, received (% of GDP)); R & D (Research and development expenditure (% of GDP)); NBR (New businesses registered (number)); PERT (Patents in environment-related technologies)
Source: Authors Construction.
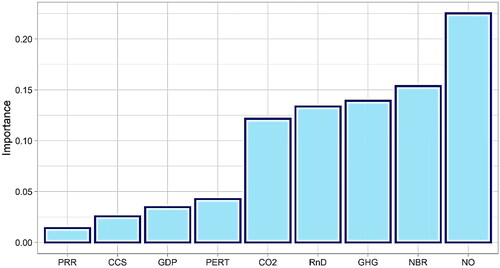
4.7. ANN model assessment
ANN model assessment results are presented in . This model performs well because of the lowest variance in annual data variation, the highest value of R2 and the lowest values of RMSE can be observed in the model. Observed and Predicted EDis presented in . In , ED has an R2 = 0.83 on the training set, followed by R2 = 0.79 for validation data. The correlation coefficient with the training set is R = 0.86. Similarly, the RMSE with the training set is 0.42. Hence, the ED achieved an accuracy of 91.70% on the training set, followed by the 88.87% on the training data
Figure 5. ANN model estimations of training and validation data for ED.
Source: Authors Construction.
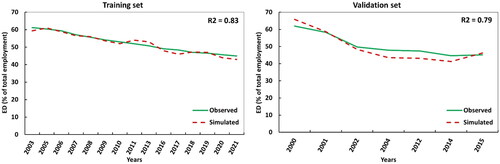
Table 6. ANN model estimations for the dependent variable (ED).
5. Discussion
The use of Artificial Neural Networks (ANNs) in data analysis has become increasingly popular in recent years due to the many advantages they offer. ANNs are computer algorithms that are inspired by the biological neural networks of the human brain and allow machines to learn from past experiences and make predictions (Khraisat et al., Citation2019; Sengupta et al., Citation2020). They are used to find patterns and relationships within data sets, making them an invaluable tool for data analysis. In this work, we used ANNs consideredto be a better option along with MLR for data analysis to assess its importance in today’s ever-growing digital world. The use of Artificial Neural Networks (ANNs) has become increasingly popular in the world of entrepreneurship, especially in the field of data analysis (Antonopoulos et al., Citation2020; Huang et al., Citation2020; Zhang et al., Citation2019). ANNs are highly accurate when it comes to predicting outcomes and making decisions. By using an ANN, entrepreneurs can make sound decisions quickly, often with a higher accuracy rate than traditional methods. An ANN can quickly analyze data and identify patterns that humans may not be able to see. It is also capable of making predictions based on those patterns and past experiences. This helps entrepreneurs make better decisions faster by providing them with more information and context (Huang et al., Citation2020; Khraisat et al., Citation2019; Tamiminia et al., Citation2020).
In addition, ANNs have proven to be extremely helpful in the process of detecting fraud and financial risk management. By analyzing data from customer profiles and other sources, an ANN can easily detect anomalies and alert the business of potential fraudulent activity. This helps businesses reduce their risks and protect themselves from potential losses (Antonopoulos et al., Citation2020; Tamiminia et al., Citation2020; Zhang et al., Citation2019). ANNs also have a wide range of applications in marketing, product development, and forecasting. They can provide marketers with detailed insights into customer behaviors and preferences. They can also be used to develop new products or services, as well as forecast future trends to make informed decisions about the company’s investments (Antonopoulos et al., Citation2020; Huang et al., Citation2020; Khraisat et al., Citation2019; Sengupta et al., Citation2020).
Entrepreneurship is the very core of China’s economic development and social change. Entrepreneurship brought about China’s economic leap and transformation in the past 15 years more than any other factor. These factors include industrialization, urbanization, technological innovation, finance development, or building capacity for innovation (J. Liu et al., Citation2020; Shahbaz et al., Citation2020). China’s economy grows mainly by supporting and encouraging entrepreneurship and the scale of economic transformation brought about by its innovators and entrepreneurs. More than two-thirds of China’s economically significant technological inventions are in domestic enterprises, of which individual inventors made more than half (Dalmarco et al., Citation2018; J. Liu et al., Citation2020). Over the past fifteen years, China has experienced extraordinary growth and development in the non-state sector. Whether we speak of established small and medium-sized businesses, growing startups, or rural entrepreneurship, entrepreneurship has rapidly become the engine of China’s economic growth.In the past 17 years, with the massive help of the government’s policies, entrepreneurship in China developed into an essential ingredient of sustainable economic growth (Dalmarco et al., Citation2018; Rosca et al., Citation2020; Secundo et al., Citation2020). The Chinese government launched a series of active measures to develop China’s entrepreneurship. Over the next two years, Geely will become the first Chinese OEM licensee of a technology company, BYD ('Build Your Dreams’). It is an important move achieved with the support of authoritarian network governments, including the Ministry of Industry and Information Technology of the State Council and the Ministry of Finance of the Government of China. In partnership with Geely, BYD will strengthen itself as ‘China’s first Chinese OEM' by bringing innovative professional R&D, product development, and marketing and sales excellence to the Chinese market.In March 2001, the 13th Five-year Plan of Make Poverty History became a milestone during China’s economic globalization. And it further strengthened Beijing’s approval of entrepreneurship and encouraged enterprises, particularly small and medium-sized enterprises to establish themselves to solve China’s problem of unemployment (Dalmarco et al., Citation2018; J. Liu et al., Citation2020; J. Liu et al., Citation2020; Rosca et al., Citation2020; Secundo et al., Citation2020). The China Venture Capital Development Center was founded as the first government venture-capital guidance center on September 23, 2003. In Yangzhou, the Jiangsu Province, entrepreneurs could provide better support to grow their businesses and thus stimulate the development of entrepreneurship.During the 14th Five-year Plan, the concept of ‘Creating a new China to make progress in entrepreneurship’ was first introduced. The government launched many policy initiatives to implement entrepreneurship in the country since 2000, one example is that it has set up various R&D centers to strengthen the entrepreneurial ecosystem and organizations to promote startups in every aspect of the industry (Bansal et al., Citation2019; Li et al., Citation2019; Wang et al., Citation2020). 3.1 million entrepreneurs registered to start their business projects 2016 last year, compared to the one million entrepreneurs of 2012, the increase is around 117.7%. Furthermore, there are large numbers of entrepreneurial incubators, startup funds, entrepreneurial training institutes, and other special zones in urban and rural areasparticularly because of the more relaxed laws on foreign enterprises, the more enterprises choose it as a result.20% of their employees were foreign workers before and now that figure had increased to 44%. There’s strength in numbers, just have a team of people from different cities, various backgrounds, and dialects (Lin et al., Citation2021; J. Liu et al., Citation2019; Wang et al., Citation2020). The first thing an entrepreneur needs to do right away is to get a business plan written and be prepared to negotiate for it with potential investors or banks. When applying for a loan, the bank wants to know they will be able to pay back a reasonable amount of money when it comes to loans. Thus, a business plan not only helps you pay by relaying a definite return value or invoicing system but also helps them know how they can expect to be repaid should they decide to own at a later date. Also, a good collection of professional advice from someone you trust can be instrumental, as it can put your business, at least into a positive perspective, and run all operations smoothly with a pseudo-all-knowing power. Create a set of rules on how to do all of your daily business tasks correctly, together with a set of deadlines that you can accomplish in a given time frame. Whether it’s planning purposes or orders, it’s best to have times allotted for it, and a weekly or monthly schedule, rather than jumping onto it all at once. Entrepreneurship development has a long history in China (Li et al., Citation2019; J. Liu et al., Citation2020).
The Chinese government has been promoting entrepreneurship development since the early 2000s. The main goals of this policy are to promote economic development and improve employment opportunities. The government has been successful in achieving these goals. The early years of entrepreneurship development were marked by trial and error (Li et al., Citation2019; Shahbaz et al., Citation2020). Many new businesses failed in the first few years. However, the Chinese government did not give up on entrepreneurship development. It continued to promote it and provide support to new businesses. As a result of the government’s efforts, the number of successful businesses has increased over the past few years. The Chinese economy has become more diversified, and there are more jobs available (Lin et al., Citation2021; J. Liu et al., Citation2019; Rosca et al., Citation2020).
The effects of social environmental stability on entrepreneurship are often underestimated. However, a stable social environment is essential for entrepreneurs to thrive. A stable social environment provides the necessary infrastructure and resources that entrepreneurs need to start and grow their businesses. It also offers a supportive network of people who can provide advice and assistance when needed (Bansal et al., Citation2019; J. Liu et al., Citation2020; Secundo et al., Citation2020). Additionally, a stable social environment helps to create an atmosphere of trust and cooperation, which is essential for business success. While a stable social environment is necessary for entrepreneurship, it is not sufficient on its own. Entrepreneurs also need access to green innovation and strategic orientation to be successful. Green innovation refers to the development of new products or services that are environmentally friendly (Lin et al., Citation2021; Wang et al., Citation2020). This type of innovation is important because it allows entrepreneurs to create businesses that are sustainable and have a positive impact on the environment. Strategic orientation focuses on creating a long-term plan for business growth. This type of planning is essential for ensuring that businesses can adapt and change as market conditions fluctuate. Entrepreneurship, green innovation, and strategic orientation are all important factors in achieving business success. However, they cannot operate in isolation from each other. The relationship between these three factors must be carefully managed to create a successful business venture (Lin et al., Citation2021; Rosca et al., Citation2020; Shahbaz et al., Citation2020).
6. Theoretical and practical implications
The relationship between entrepreneurship, green innovation, and strategic orientation has become increasingly important to consider when examining the success of an entrepreneur. There is much evidence that suggests that the environment an entrepreneur operates in can significantly influence their success. This includes social environmental stability, which can have both positive and negative effects on entrepreneurs.
Theoretically, it is suggested that social environmental stability can affect entrepreneurial success by providing or limiting access to resources, thus enabling, or inhibiting the process of starting and managing a business. For example, if an entrepreneur operates in a stable social environment, they are likely to have greater access to resources such as capital and networks. This can give them an edge in terms of obtaining financial resources, understanding the market, and making informed decisions. Additionally, having access to more networks can help entrepreneurs build relationships with potential partners and customers, thus increasing the likelihood of success.
On the other hand, if an entrepreneur operates in an unstable social environment, they may not have access to the same resources. This can make it more difficult to obtain financial support and identify potential opportunities in the market. Additionally, an unstable social environment could reduce an entrepreneur’s ability to build relationships with potential customers and partners, thereby hindering the success of their venture.
To assess how social environmental stability affects entrepreneurial success, researchers have developed documentary methods. These methods involve conducting interviews with entrepreneurs and analyzing data from existing studies to determine how social environmental stability influences entrepreneurial behavior and performance. Through these methods, researchers can gain a better understanding of how social environmental stability affects the success of an entrepreneur and how entrepreneurs should operate in different contexts.
By considering social and environmental stability, researchers can develop more effective strategies for promoting entrepreneurship, green innovation, and strategic orientation. Understanding how social environmental stability impacts entrepreneurial success can help guide policymakers in creating strategies that can support and encourage successful entrepreneurs. Additionally, understanding this relationship can help entrepreneurs adapt to their environment and make informed decisions about their businesses.
Sustainable business-people can act as the motors for passing on the ongoing economy by beginning to consolidate all parts of manageability instead of only one. Organizations and legislative bodies frequently miss giving essential social and ecological labor and products; a manageable economy covers such holes. The triple primary concern can likewise be a helpful device for organizations, noble causes, and legislative establishments to be involved in assessing their supportability regarding natural quality, civil rights, and financial thriving. According to this study, adopting sustainable practices in SMEs may be influenced by sociocultural factors, institutional realities, and factors other than an entrepreneurial will, which broadens understanding of sustainable entrepreneurship.
Because ethical and socially conscious behavior is not only socially desirable but also a common and accepted aspect of business life, it is advised that a company’s scope goes beyond only financial performance. The results add to the literature on entrepreneurial economics by offering an empirical method that supports the supposition that entrepreneurship affects sustainable development. By promoting certain forms of entrepreneurial activity, this strategy not only adds to the body of existing research but also provides policy and managerial consequences for implementing sustainable development goals. However, since our study only examined direct relationships between the categories mentioned earlier and the sample of nations, its limitations serve as a starting point for further research.
Future research about sustainable development goals may analyze many economic, social, and environmental indicators. Additionally, we only looked at how entrepreneurship directly affected the foundational elements of sustainable development. The path to sustainable entrepreneurship is convoluted and may involve several steps. As some other researchers have suggested, this is why entrepreneurship cannot simultaneously fulfill sustainability goals without putting in place suitable ecosystem conditions. Future studies might therefore build on this work by using mediating or moderating models to investigate the circumstances under which entrepreneurship could accomplish these goals.
The cost of transitioning from conventional to environmental entrepreneurship could be a problem because it might require additional spending. Green products are not well-known to customers or business owners. It can be challenging for business owners to transition from traditional to sustainable entrepreneurship, particularly for new business owners who periodically require training. It may be necessary to have green human resources for sustainable enterprises to succeed. Businesses in less developed countries may find developing green goods and services difficult. Hence some regions require support programs to encourage green entrepreneurship. The many highly educated, creative, and innovative entrepreneurs in less developed countries will be able to successfully run sustainable business models with a cash infusion and the dissemination of best practices.
6.1. Policy implications
This study presents recommendations for institutions, organizations, and politicians on how to adopt rules that will promote both countries’ entrepreneurial cultures. Based on the study’s findings, the authors propose that new legislation be enacted to assist students in developing sustainable business practices. Universities’ educational initiatives should foster less risk-taking, more effective implementation of sustainable entrepreneurial concepts, and the identification of opportunities. To foster a long-lasting entrepreneurial culture, government and institutional decision-makers should implement plans and regulations to protect the interests of aspiring entrepreneurs. To increase the amount of strategic innovation in nations, aspiring entrepreneurs must be rewarded in ways other than monetary benefits.
By increasing the chances of sustainable start-ups, sustainable possibilities will promote sustainability in both economies. The study supports the idea that work values should be given more weight to accelerate individual attitudes toward a sustainable environment. As a result, it has implications for the promotion of sustainability-driven work values. One possible strategy to promote sustainable as a career path while simultaneously advancing sustainable development is to use the methods of industry role models and interact with them to paint a positive picture of sustainable businesses.
7. Conclusion
Based on the literature review, this paper offers insight into the connections between entrepreneurial activity and sustainable development. As per our examination, different innovative undertakings seem to affect the economic turn of events differently. The discoveries show that enterprising movement driven by open doors and advancement impacts economic improvement while creative action propelled by need has an adverse consequence. Accordingly, our findings offer to persuade proof for the case that business ventures and practical improvement are connected. Various researchers agree that organization proprietors have made significant commitments to society, the economy, and humankind through making occupations, utilizing business valuable open doors, and item advancement.
Entrepreneurs committed to sustainability should have a distinct vision for their businesses’ routes. It is because most coordinated supportable tasks have a drawn-out degree, and profit from ventures might take some time; economic business is a gamble-taking endeavor. The inward culture and schedules of the SME ought to energize manageable business visionaries’ solid pioneering direction at the authoritative degree of investigation. Feasible business requests total and equivalent commitments to the drawn-out well-being of the economy, society, and climate.
8. Future direction
The current research can be improved by including more dimensions in the analysis, such as environmental and ecosystem indicators and elements like national culture, company culture, and family customs. The research can increase the time range because the scarcity of recent data limits our analysis. On the other hand, sustainable innovation is a collection of initiatives and a system of community-wide negotiation that pushes and pulls overlapping forces to address the acute problem of food and fiber preservation. Encouraging non-business-people to pursue entrepreneurship may also significantly impact the degree of long-term entrepreneurial goals and eventual implementation. To promote such entrepreneurs across various nations to meet society’s food and fiber needs without jeopardizing them. For future generations’ ability to survive using its resources, educational institutions should create opportunities for capacity development and foster competitive venturing themes within established businesses.
A typical mentality and intelligence would ultimately need to help our society use inventive commercial approaches to solve the most challenging issues. By the target date, sustainable, equitable, and healthy economies must actively involve youth to avoid the worst risks and challenges associated with sustainable development, such as the effects of climate change, gender disparities, war, displacement, probability, unemployment, and the alleviation of poverty.
9. Limitations
There are still a few issues with the study, one offering new prospects for future research. Before, this study’s hypothesized connections between sustainable value creation and sustainable entrepreneurship were context-specific and did not account for the distinction between actual acts and purpose, a study that establishes a solid framework for the examination would boost support for the proposed model and provide a comparison of various social strata, appropriately establishing any connections that might be homogenous economies. Third, gender was not considered in this study, even though doing so would have improved the current model, especially in developed and rising economies. A more extensive analysis needs to generalize the results.
Despite its shortcomings, this study offers academics, decision-makers, and political organizations helpful guidance that will aid them in determining how to enhance and expand sustainability in both economies. Future generations should consider these links by exploring various entrepreneurial possibilities that reflect different degrees and types of businesses.
Disclosure statement
No potential conflict of interest was reported by the authors.
Additional information
Funding
References
- Abduljabbar, R., Dia, H., Liyanage, S., & Bagloee, S. A. (2019). Applications of artificial intelligence in transport: An overview. Sustainability, 11(1), 189. https://doi.org/10.3390/su11010189
- Abiodun, O. I., Jantan, A., Omolara, A. E., Dada, K. V., Mohamed, N. A., & Arshad, H. (2018). State-of-the-art in artificial neural network applications: A survey. Heliyon, 4(11), e00938. https://doi.org/10.1016/j.heliyon.2018.e00938
- Ahmad, M., Alam, K., Tariq, S., Anwar, S., Nasir, J., & Mansha, M. (2019). Estimating fine particulate concentration using a combined approach of linear regression and artificial neural network. Atmospheric Environment, 219, 117050. https://doi.org/10.1016/j.atmosenv.2019.117050
- Al-Kumaim, H. S., Malaysia, O., Raja, P., & Malaysia, P. R. (2020). Impact of television documentary on student communication skills.
- Ameer, S., Shah, M. A., Khan, A., Song, H., Maple, C., Islam, S. U., & Asghar, M. N. (2019). Comparative analysis of machine learning techniques for predicting air quality in smart cities. IEEE Access, 7, 128325–128338. https://doi.org/10.1109/ACCESS.2019.2925082
- Amrhein, V., Trafimow, D., & Greenland, S. (2019). Inferential statistics as descriptive statistics: There is no replication crisis if we don’t expect replication. The American Statistician, 73(sup1), 262–270. https://doi.org/10.1080/00031305.2018.1543137
- Antonopoulos, I., Robu, V., Couraud, B., Kirli, D., Norbu, S., Kiprakis, A., Flynn, D., Elizondo-Gonzalez, S., & Wattam, S. (2020). Artificial intelligence and machine learning approaches to energy demand-side response: A systematic review. Renewable and Sustainable Energy Reviews, 130, 109899. https://doi.org/10.1016/j.rser.2020.109899
- Araghi, A., Mousavi-Baygi, M., Adamowski, J., & Martinez, C. J. (2019). Analyzing trends of days with low atmospheric visibility in Iran during 1968–2013. Environmental Monitoring and Assessment, 191(4), 249. https://doi.org/10.1007/s10661-019-7381-8
- Bansal, S., Garg, I., & Sharma, G. D. (2019). Social entrepreneurship as a path for social change and driver of sustainable development: A systematic review and research agenda. Sustainability, 11(4), 1091. https://doi.org/10.3390/su11041091
- Berman, A., Cano-Kollmann, M., & Mudambi, R. (2022). Innovation and entrepreneurial ecosystems: Fintech in the financial services industry. Review of Managerial Science, 16(1), 45–64. https://doi.org/10.1007/s11846-020-00435-8
- Balarabe, B., & Bery, A. A. (2021). Modeling of soil shear strength using multiple linear regression (MLR) at Penang, Malaysia. Journal of Engineering Research (Kuwait), 9(3), 40–51. https://doi.org/10.36909/jer.v9i3A.7675
- Bradley, S. W., Kim, P. H., Klein, P. G., McMullen, J. S., & Wennberg, K. (2021). Policy for innovative entrepreneurship: Institutions, interventions, and societal challenges. Strategic Entrepreneurship Journal, 15(2), 167–184. https://doi.org/10.1002/sej.1395
- Business, D. (2020). World Bank Group. Електронний Ресурс:–http://www.Doingbusiness.Org/ExploreTopics/PayingTaxes/CompareAll.Aspx.
- Chege, S. M., & Wang, D. (2020). The impact of entrepreneurs’ environmental analysis strategy on organizational performance. Journal of Rural Studies, 77, 113–125. https://doi.org/10.1016/j.jrurstud.2020.04.008
- Chehreh Chelgani, S., Shahbazi, B., & Hadavandi, E. (2018). Support vector regression modeling of coal flotation based on variable importance measurements by mutual information method. Measurement, 114, 102–108. https://doi.org/10.1016/j.measurement.2017.09.025
- Dalmarco, G., Hulsink, W., & Blois, G. V. (2018). Creating entrepreneurial universities in an emerging economy: Evidence from Brazil. Technological Forecasting and Social Change, 135, 99–111. https://doi.org/10.1016/j.techfore.2018.04.015
- Das, B. (2018). Evaluation of multiple linear, neural network and penalised regression models for prediction of rice yield based on weather parameters for west coast of India.
- Demirel, P., & Danisman, G. O. (2019). Eco innovation and firm growth in the circular economy: Evidence from European small and medium sized enterprises. Business Strategy and the Environment, 28(8), 1608–1618. https://doi.org/10.1002/bse.2336
- Diaz, Z. (1998). World Bank group. Journal of Business & Finance Librarianship, 3(4), 61–68. https://doi.org/10.1300/J109v03n04_05
- Dimitriadou, S., & Nikolakopoulos, K. G. (2022). Multiple linear regression models with limited data for the prediction of reference evapotranspiration of the Peloponnese, Greece. Hydrology, 9(7), 124. https://doi.org/10.3390/hydrology9070124
- Ding, J., Zhang, G., Wang, S., Xue, B., Yang, J., Gao, J., Wang, K., Jiang, R., & Zhu, X. (2022). Forecast of hourly airport visibility based on artificial intelligence methods. Atmosphere, 13(1), 75. https://doi.org/10.3390/atmos13010075
- Ferreira, J. J., & Teixeira, A. A. C. (2019). Open innovation and knowledge for fostering business ecosystems. Journal of Innovation & Knowledge, 4(4), 253–255. https://doi.org/10.1016/j.jik.2018.10.002
- Frederick, H., O’Connor, A., & Kuratko, D. F. (2018). Entrepreneurship. Cengage AU.
- Galindo-Martín, M.-A., Castaño-Martínez, M.-S., & Méndez-Picazo, M.-T. (2020). The relationship between green innovation, social entrepreneurship, and sustainable development. Sustainability, 12(11), 4467. https://doi.org/10.3390/su12114467
- Garson, D. G. (1991). Interpreting neural network connection weights. Artificial Intelligence Expert, 6(4), 47–51.
- Gauthier, J., Wu, Q. V., & Gooley, T. A. (2020). Cubic splines to model relationships between continuous variables and outcomes: A guide for clinicians. Bone Marrow Transplantation, 55(4), 675–680. (Nature Publishing Group. https://doi.org/10.1038/s41409-019-0679-x
- Goh, A. T. C. (1995). Back-propagation neural networks for modeling complex systems. Artificial Intelligence in Engineering, 9(3), 143–151. https://doi.org/10.1016/0954-1810(94)00011-S
- Gontareva, I., Chorna, M., Pawliszczy, D., Barna, M., Dorokhov, O., & Osinska, O. (2018). Features of the entrepreneurship development in digital economy. TEM Journal, 7(4), 813–822. https://doi.org/10.18421/TEM74-19
- Graff Zivin, J., & Neidell, M. (2013). Environment, health, and human capital. Journal of Economic Literature, 51(3), 689–730. https://doi.org/10.1257/jel.51.3.689
- Grant, C. (2018). Tactics for connecting entrepreneurship and technical communication through community engagement [Paper presentation]. SIGDOC 2018 - 36th ACM International Conference on the Design of Communication, 1–6. https://doi.org/10.1145/3233756.3233956
- Hadavandi, E., & Chelgani, S. C. (2019). Estimation of coking indexes based on parental coal properties by variable importance measurement and boosted-support vector regression method. Measurement, 135, 306–311. https://doi.org/10.1016/j.measurement.2018.11.068
- Huang, S., Yang, J., Fong, S., & Zhao, Q. (2020). Artificial intelligence in cancer diagnosis and prognosis: Opportunities and challenges. Cancer Letters, 471, 61–71.
- Igielski, M. (2021). Determinants of entrepreneurship development in Poland over the last 5 years. Entrepreneurship and Sustainability Issues, 9(1), 330–347. https://doi.org/10.9770/jesi.2021.9.1(20)
- Ilonen, S. (2021). Creating an entrepreneurial learning environment for entrepreneurship education in HE: The educator’s perspective. Industry and Higher Education, 35(4), 518–530. https://doi.org/10.1177/09504222211020637
- Ionescu, G. H., Firoiu, D., Pîrvu, R., Enescu, M., Rădoi, M. I., & Cojocaru, T. M. (2020). The potential for innovation and entrepreneurship in eu countries in the context of sustainable development. Sustainability (Switzerland), 12(18), 7218–7250. https://doi.org/10.3390/su12187250
- Ishak, A. B. (2016). Variable selection using support vector regression and random forests: A comparative study. Intelligent Data Analysis, 20(1), 83–104. https://doi.org/10.3233/IDA-150795
- Islam, A., & Wahab, S. A. (2021). The intervention of strategic innovation practices in between regulations and sustainable business growth: A holistic perspective for Malaysian SMEs. World Journal of Entrepreneurship, Management and Sustainable Development, ahead-of-print(ahead-of-print), 396–421. https://doi.org/10.1108/WJEMSD-04-2020-0035
- Islas-Moreno, A., Muñoz-Rodríguez, M., & Morris, W. (2021). Understanding the rural entrepreneurship process: A systematic review of literature. World Review of Entrepreneurship, Management and Sustainable Development, 17(4), 453–470. https://doi.org/10.1504/WREMSD.2021.116651
- Kalisz, D., Schiavone, F., Rivieccio, G., Viala, C., & Chen, J. (2021). Analyzing the macro-level determinants of user entrepreneurship. The moderating role of the national culture. Entrepreneurship & Regional Development, 33(3-4), 185–207. https://doi.org/10.1080/08985626.2021.1872934
- Kanani, K. A., & Gelard, P. (2019). The effect of charismatic leadership on strategic entrepreneurship with emphasis on network communication approach.
- Khotimah, I., & Ruyani, N. A. (2021). Influence of entrepreneurial skills training and work motivation toward entrepreneurship intention. Indonesian Journal of Office Administration, 3(2), 66–78.
- Khraisat, A., Gondal, I., Vamplew, P., & Kamruzzaman, J. (2019). Survey of intrusion detection systems: Techniques, datasets and challenges. Cybersecurity, 2(1), 1–22. https://doi.org/10.1186/s42400-019-0038-7
- Kim-Soon, N., Ahmad, A. R., & Ibrahim, N. N. (2018). Understanding the motivation that shapes entrepreneurship career intention. Entrepreneurship - Development Tendencies and Empirical Approach, 291. https://doi.org/10.5772/intechopen.70786
- Kong, F., Zhao, L., & Tsai, C.-H. (2020). The relationship between entrepreneurial intention and action: The effects of fear of failure and role model. Frontiers in Psychology, 11, 229. https://doi.org/10.3389/fpsyg.2020.00229
- Lenihan, H., McGuirk, H., & Murphy, K. R. (2019). Driving innovation: Public policy and human capital. Research Policy, 48(9), 103791. https://doi.org/10.1016/j.respol.2019.04.015
- Li, Y., Fan, P., & Liu, Y. (2019). What makes better village development in traditional agricultural areas of China? Evidence from long-term observation of typical villages. Habitat International, 83, 111–124. https://doi.org/10.1016/j.habitatint.2018.11.006
- Lin, S., Winkler, C., Wang, S., & Chen, H. (2021). Regional determinants of poverty alleviation through entrepreneurship in China. In Business, entrepreneurship and innovation toward poverty reduction (pp. 41–62). Routledge.
- Liu, J., Chang, H., Forrest, J. Y.-L., & Yang, B. (2020). Influence of artificial intelligence on technological innovation: Evidence from the panel data of china’s manufacturing sectors. Technological Forecasting and Social Change, 158, 120142. https://doi.org/10.1016/j.techfore.2020.120142
- Liu, J., Li, X., & Wang, S. (2020). What have we learnt from 10 years of fintech research? A scientometric analysis. Technological Forecasting and Social Change, 155, 120022. https://doi.org/10.1016/j.techfore.2020.120022
- Liu, J., Zhu, Y., Serapio, M. G., & Cavusgil, S. T. (2019). The new generation of millennial entrepreneurs: A review and call for research. International Business Review, 28(5), 101581. https://doi.org/10.1016/j.ibusrev.2019.05.001
- Liu, Y. (2021). Empathy narration and symbol communication of the documentary new domestic products in China. Tobacco Regulatory Science, 7(5), 3643–3651. https://doi.org/10.18001/TRS.7.5.1.141
- Mardani Shahrbabak, M., & Solati, S. (2020). Development of a model for strategic entrepreneurship by focusing on key competencies. Quarterly Journal of Industrial Technology Development, 18(41), 3–12.
- Mathushan, P., & Pushpanathan, A. (2020). Does green innovative practices matter? The effect of green innovation on green entrepreneurship sustainability. Journal of Business Studies, 7(1), 127. https://doi.org/10.4038/jbs.v7i1.56
- Mclean Cabaneros, S., Calautit, J. K., Hughes, B. R., & Author, C. (n.d). A review of artificial neural network models for ambient air pollution prediction author names and affiliations. 1–50.
- Mishra, P., Pandey, C. M., Singh, U., Gupta, A., Sahu, C., & Keshri, A. (2019). Descriptive statistics and normality tests for statistical data. Annals of Cardiac Anaesthesia, 22(1), 67–72. https://doi.org/10.4103/aca.ACA_157_18
- Moridsadat, p., & Moamelvand, s (2020). The factors affecting sustainable entrepreneurship development in rural tourism (case study: Goleyjan rural district, Tonekabon county). Researches in Earth Sciences, 10(4), 91–107. https://doi.org/10.52547/esrj.10.4.91
- Muchunku, P. B. K. (2020). Effective strategies for youth entrepreneurship development in the agribusiness sector. United States International University-Africa.
- Nguyen, T. N. T., Luu, V. H., Pham, V. H., Bui, Q. H., & Nguyen, T. K. O. (2020). Particulate matter concentration mapping from satellite imagery. TORUS 3–toward an Open Resource Using Services: Cloud Computing for Environmental Data, 103–130. https://doi.org/10.1002/9781119720522.ch5
- Olden, J. D., Joy, M. K., & Death, R. G. (2004). An accurate comparison of methods for quantifying variable importance in artificial neural networks using simulated data. Ecological Modelling, 178(3–4), 389–397. https://doi.org/10.1016/j.ecolmodel.2004.03.013
- Parker, S. C. (2018). The economics of entrepreneurship. Cambridge University Press.
- Prendes-Espinosa, P., Solano-Fernández, I. M., & García-Tudela, P. A. (2021). Emdigital to promote digital entrepreneurship: The relation with open innovation. Journal of Open Innovation: Technology, Market, and Complexity, 7(1), 63–14. https://doi.org/10.3390/joitmc7010063
- Radojević, D., Antanasijević, D., Perić-Grujić, A., Ristić, M., & Pocajt, V. (2019). The significance of periodic parameters for ANN modeling of daily SO 2 and NOx concentrations: A case study of Belgrade, Serbia. Atmospheric Pollution Research, 10(2), 621–628. https://doi.org/10.1016/j.apr.2018.11.004
- Rasp, S., Dueben, P. D., Scher, S., Weyn, J. A., Mouatadid, S., & Thuerey, N. (2020). WeatherBench: A benchmark data set for data‐driven weather forecasting. Journal of Advances in Modeling Earth Systems, 12(11), e2020MS002203. https://doi.org/10.1029/2020MS002203
- Rosa, A. C. M., Rosa, A. M., das Neves, J. M. S., Souza, C. P., & Dos Santos, B. R. O. B. (2021). Innovative entrepreneur and creativity: A bibliometric analysis. Global Scientific Journals, 9(3). https://www.researchgate.net/profile/Ramon-Santos-6/publication/350074692_Innovative_Entrepreneur_and_Creativity_A_Bibliometric_Analysis/links/604f9f3a458515e8344a4d85/Innovative-Entrepreneur-and-Creativity-A-Bibliometric-Analysis.pdf
- Rosário, A. T., Fernandes, F., Raimundo, R. G., & Cruz, R. N. (2020). Determinants of nascent entrepreneurship development. Handbook of Research on Nascent Entrepreneurship and Creating New Ventures, 172–193. https://doi.org/10.4018/978-1-7998-4826-4.ch008
- Rosca, E., Agarwal, N., & Brem, A. (2020). Women entrepreneurs as agents of change: A comparative analysis of social entrepreneurship processes in emerging markets. Technological Forecasting and Social Change, 157, 120067. https://doi.org/10.1016/j.techfore.2020.120067
- Rus-Casas, C., Eliche-Quesada, D., Aguilar-Peña, J. D., Jiménez-Castillo, G., & La Rubia, M. D. (2020). The impact of the entrepreneurship promotion programs and the social networks on the sustainability entrepreneurial motivation of engineering students. Sustainability, 12(12), 4935. https://doi.org/10.3390/su12124935
- Ruß, G., & Brenning, A. (2010). Spatial variable importance assessment for yield prediction in precision agriculture. Lecture Notes in Computer Science (Including Subseries Lecture Notes in Artificial Intelligence and Lecture Notes in Bioinformatics), 6065 LNCS, 184–195. https://doi.org/10.1007/978-3-642-13062-5_18/COVER
- Russo, A., Raischel, F., & Lind, P. G. (2013). Air quality prediction using optimal neural networks with stochastic variables. Atmospheric Environment, 79, 822–830. https://doi.org/10.1016/j.atmosenv.2013.07.072
- Rustam, F., Reshi, A. A., Mehmood, A., Ullah, S., On, B.-W., Aslam, W., & Choi, G. S. (2020). COVID-19 future forecasting using supervised machine learning models. IEEE Access. 8, 101489–101499. https://doi.org/10.1109/ACCESS.2020.2997311
- Santosaa, H., Basukib, A., & Salahudin, A. (2020). Building entrepreneurship readiness of vocational students through values-based education in Indonesia. International Journal of Innovation, Creativity and Change, Www. Ijicc, Net, 371–383.
- Sargani, G. R., Jiang, Y., Zhou, D., Chandio, A. A., Hussain, M., & Khan, N. (2021). Endorsing sustainable enterprises among promising entrepreneurs: A comparative study of factor-driven economy and efficiency-driven economy. Frontiers in Psychology, 12, 735127. https://doi.org/10.3389/fpsyg.2021.735127
- Scala, A., Trunfio, T. A., De Coppi, L., Rossi, G., Borrelli, A., Triassi, M., & Improta, G. (2022). Regression models to study the total LOS related to valvuloplasty. International Journal of Environmental Research and Public Health, 19(5), 3117. https://doi.org/10.3390/ijerph19053117
- Secundo, G., Rippa, P., & Cerchione, R. (2020). Digital academic entrepreneurship: A structured literature review and avenue for a research agenda. Technological Forecasting and Social Change, 157, 120118. https://doi.org/10.1016/j.techfore.2020.120118
- Sengupta, S., Basak, S., Saikia, P., Paul, S., Tsalavoutis, V., Atiah, F., Ravi, V., & Peters, A. (2020). A review of deep learning with special emphasis on architectures, applications and recent trends. Knowledge-Based Systems, 194, 105596. https://doi.org/10.1016/j.knosys.2020.105596
- Shahbaz, M., Nasir, M. A., Hille, E., & Mahalik, M. K. (2020). UK’s net-zero carbon emissions target: Investigating the potential role of economic growth, financial development, and R&D expenditures based on historical data (1870–2017). Technological Forecasting and Social Change, 161, 120255. https://doi.org/10.1016/j.techfore.2020.120255
- Sheng, Y., Ding, J., & Huang, J. (2019). The Relationship between farm size and productivity in agriculture: Evidence from maize production in Northern China. American Journal of Agricultural Economics, 101(3), 790–806. https://doi.org/10.1093/ajae/aay104
- Tambovceva, T., Tereshina, M., & Samarina, V. (2019). Green innovations in regional economy. Engineering for Rural Development, 18(24.05), 1832–1839. https://doi.org/10.22616/ERDev2019.18.N357
- Tamiminia, H., Salehi, B., Mahdianpari, M., Quackenbush, L., Adeli, S., & Brisco, B. (2020). Google Earth Engine for geo-big data applications: A meta-analysis and systematic review. ISPRS Journal of Photogrammetry and Remote Sensing, 164, 152–170. https://doi.org/10.1016/j.isprsjprs.2020.04.001
- Tohidyan Far, S., & Rezaei-Moghaddam, K. (2019). Multifunctional agriculture: An approach for entrepreneurship development of agricultural sector. Journal of Global Entrepreneurship Research, 9(1), 1–23. https://doi.org/10.1186/s40497-019-0148-4
- Triantafillidou, E., & Tsiaras, S. (2018). Exploring entrepreneurship, innovation and tourism development from a sustainable perspective: Evidence from Greece. Journal for International Business and Entrepreneurship Development, 11(1), 53–64. https://doi.org/10.1504/JIBED.2018.090020
- Wang, Z., He, Q., Xia, S., Sarpong, D., Xiong, A., & Maas, G. (2020). Capacities of business incubator and regional innovation performance. Technological Forecasting and Social Change, 158, 120125. https://doi.org/10.1016/j.techfore.2020.120125
- Wang, Z., Wang, Y., Zeng, R., Srinivasan, R. S., & Ahrentzen, S. (2018). Random Forest based hourly building energy prediction. Energy and Buildings, 171, 11–25. https://doi.org/10.1016/j.enbuild.2018.04.008
- Wong, Y. J., Arumugasamy, S. K., Chung, C. H., Selvarajoo, A., & Sethu, V. (2020). Comparative study of artificial neural network (ANN), adaptive neuro-fuzzy inference system (ANFIS) and multiple linear regression (MLR) for modeling of Cu (II) adsorption from aqueous solution using biochar derived from rambutan (Nephelium lappaceum) pee. Environmental Monitoring and Assessment, 192(7), 1–20. https://doi.org/10.1007/s10661-020-08268-4
- Zhang, W., Yang, D., & Wang, H. (2019). Data-driven methods for predictive maintenance of industrial equipment: A survey. IEEE Systems Journal, 13(3), 2213–2227. https://doi.org/10.1109/JSYST.2019.2905565
- Zhongming, Z., Linong, L., Xiaona, Y., Wangqiang, Z., & Wei, L. (2020). Data, data everywhere: New world bank water data portal.
- Zhu, C., Idemudia, C. U., & Feng, W. (2019). Improved logistic regression model for diabetes prediction by integrating PCA and K-means techniques. Informatics in Medicine Unlocked, 17, 100179. https://doi.org/10.1016/j.imu.2019.100179
- Zvavahera, P., Chigora, F., & Tandi, R. (2018). Entrepreneurship: An engine for economic growth. International Journal of Academic Research in Business and Social Sciences, 8(11). https://doi.org/10.6007/ijarbss/v8-i11/4884