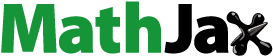
Abstract
In this paper we formulate and analyze a novel model on a retailer’s inventory and pricing decisions for fresh agricultural products with consumers’ forward consuming behavior under online channel. We consider a dynamic stochastic setting, where every period consists of two stages, discounting pricing stage and regular sale stage. At the beginning of the period, the retailer decides how much new fresh agricultural products to order and sets discount price for leftover inventories from the previous period which will be disposed otherwise, and determines regular price for fresh products on the second stage, respectively. Since forward purchase consumers may buy the products during discount pricing stage, which may cannibalize future sales at regular price, the retailer needs to make a trade-off decision between regular price and discounting price. We bring forward a dynamic optimization model and use nonlinear programming method of Karush Kuhn Tucker condition to obtain the optimal dynamic strategy, which is comparatively analyzed to dominate the related static strategy. We also show that consumers forward buying behavior will negatively influence the retailer’s profit. When the price is set too low in regular or discounting sales, the profit will show an up-down trend if the inventory exceeds a certain threshold. Meanwhile, when fresh goods returns are allowed and resold in the secondary stage, the retailer’s profit will increase. We finally conduct numerical studies to further characterize the optimal policy, and to evaluate the loss of efficiency under static policies when compared to the optimal dynamic policy.
JEL CODES:
1. Introduction
Since the development of E-commerce, more and more retailers supplement their brick-and mortar presence with an online store by providing cheaper and more efficient distribution channels for their products or services. For example, Amazon launched its business with an e-commerce-based model of online sales and product delivery. The efficiency of online channel also attracts a large number of fresh agricultural products sale businesses to enter this field. IResearch consulting company has found that the number of fresh agricultural products (FAPs) transactions in China’s e-commerce market exceeded 200 billion yuan in 2018. It is expected that the average annual growth of online retail fresh agricultural products transactions will remain at around 35% in the next three years. In the future, as the scale of mobile users and the penetration rate of mobile online shopping continues to increase, and the concept of online shopping becomes more and more popular, consumers’ demand for online fresh purchase will continue to expand.
However, as the perishability nature of fresh agricultural products that make the products deteriorating over time soon, those retailers are currently facing high operating costs and logistics costs even through online channel selling. Such as Stater Bros, a well-known grocery store in Southern California who focuses its business on fresh agricultural products selling, reported its higher commodity costs and competitive pressures in fiscal 2011Footnote1. Generally, they always set a discount price policy to sell non-fresh agricultural products a period of time for taking back some revenue. It is not a trivial problem for the seller to do that. However, with the development of information technology, some consumers may recognize the promotion sales and adopt strategic purchase policy, accordingly. As Angwin and Mattioli (Citation2012) and Shi (Citation2014) and other scholars mentioned, e-commerce greatly influences the transmission mode of price and changes the behavior pattern of consumers, such as the appearance of forward buying behavior. Some of the consumers who can afford the full price may be attracted by the price discount to buy non-fresh products, which has a negative impact on the revenue from selling at the full price. Meanwhile, pricing is also a double-edged sword in that pricing can not only affect the demand but also have great impact on the sellers’ profit, especially a poor pricing policy which can easily damage the seller’s benefit. For example, Tesco, the world’s third-biggest supermarket chain, failed to revive its sales performance despite spending £500 million on price cuts in EurozoneFootnote2. Although the discount selling on those units can generate some revenue for the otherwise disposed items, the new and old products will compete together among consumers who select their choice based on its utility from combination of quality and price. In addition, a report from Xinlang Finance on December 12, 2015, claims that about 20% of fresh products could be returned and exchanged within 48 hours without reasons. And the 4th China fresh e-commerce forum in 2017, officially releases the first Chinese community standards, ‘fresh e-commerce platform returning and exchange service requirements’, which provides consumers with rights to rescission. Since then, high rates of return and exchange of goods in online retailing appears, which increases channel inventory cost and incurs return cost, and then influences the retailer’s profit because all the returned products may be resell on a discount price or disposed directly. As Yao and Zhang (Citation2013), Altug and Aydinliyim (Citation2016), Fan and Wang (Citation2018) pointed out, an important difference between online and offline retail is that online retail has logistics costs because of a large number of returns. Therefore, some fundamental decisions are at the store manager’s discretion in such an environments, how much to order for the next time and what pricing policies to follow, the regular price and the discount price, respectively.
In this study, we formulate a novel model in which a retailer makes simultaneous decisions on regular and discount price as well as inventory control for fresh agricultural products under online retailing channel, in the presence of consumer’s forward buying behavior and demand uncertainty. We consider a dynamic stochastic setting over multiperiod planning horizon, in which each period consists of two parts, a discount pricing stage and a regular-sales stage. For the convenience of further study, we label the time periods such that every period starts with the discounting stage. Any unit from the previous period has to either sold or disposed at the end of the discounting stage, while leftover inventory from the regular-sales stage can be carried over to the discounting stage of the next period. In practice, for the sake of feasible retailing operation as the perishabiliy, the return and exchange policy applies only to the new fresh agricultural products except the old ones which still can be given satisfactory price discount compensation. Generally, new fresh products at the regular-sale stage could be returned and resold at the discount stage or disposed directly, while the old ones at the discounting stage are not allowed. And the return cost is assumed to be related to the sale quantity of fresh agricultural products based on previous studies. Demand in each period is random with some consumers forward buying behavior of preferring buy in the discounting stage.
Our model assumes a population of homogeneous (statistically identical and independent) customers making strategic choices from a set of different ages of FAPs. We set two factors to present the proportion of consumers who attempt to make forward purchases and the ratio of return and exchange, respectively. The retailer, through the integration of inventory control and dynamic pricing, influences consumers’ choices to his advantage to maximize profit. Obviously, the problem is extremely complicated even if the products are not perishable. Unlike standard inventory models of general products with same attributes, the products’ perishability, consumers forward buying portion and the ratio of return and exchange effect plays an important role in driving the dynamic pricing adjustments as it affects not only the overall probability of making a sale but also the sales probability of individual variables within the in-stock offerings/variables. As a result, the original formulation is not jointly concave in the decision variables and is therefore intractable. To deal with the complexity, we work with the portion parameters to transform the problem to be jointly concave and tractable. Therefore, we characterize the optimal policy and develop effective methods to solve it. At last, a numerical study is presented to illustrate the interplay of the price and inventory control of FAP on online channel. Our contribution to the literature as follows. Firstly, we formulate and analyze a novel model on a retailer’s dynamic inventory and pricing decisions for fresh agricultural products with consumers’ forward consuming behavior under online channel. Characterizing the structure of model, especially with conditions on some parameters, we present an optimal solution to the problem. Second, our numerical results provide insights on the behavior of the optimal dynamic prices, and highlight the complex interplay of inventory scarcity and consumers forward buying behavior. Thirdly, we generalize the regularity conditions of profit functions for lost-sales inventory-pricing models, using the concept of concavity.
The remainder of the paper is structured as follows. Section 2 reviews the literature. We introduce the model formulation and exploit the optimal solution, as well as some numerical experiments in section 3, Finally, section 4 provides concluding remarks.
2. Literature review
The development of online retailing put forward a profound impact on the traditional retail. Although, bringing shopping convenience to consumers, it also changes business model and cost structure of retailers (Chen et al., Citation2006) as well as consumers purchase behavior, such as forward purchase buying (Shi, Citation2014). Some studies (Altug & Aydinliyim, Citation2016; Fan & Wang, Citation2018; Yao & Zhang, Citation2013) have pointed out that there is an important difference between online and offline sales, which lies in the logistics cost and return or exchange. In decades of years, studies mainly focus on problems under different market environments, see listing the inventory control models of different market demand, which follows different stochastic distribution. With regard to fresh agricultural products retailing, most scholars generally add one or two more variables, such as freshness or time on the market demand function (Chen et al., Citation2014, Citation2018; San-José et al., Citation2015; Tripathi et al., Citation2015).
Table 1. Inventory control models based on different demand functions.
In line with offline sales, dynamic pricing strategy can facilitate the sales since the menu cost of online sales is almost zero, especially when demand is random and sensitive (Meng et al.,Citation2010). More scholars also point out that more efficiency and less decay can be achieved through dynamic pricing strategies (Adenso-Díaz et al., Citation2017; Akçay et al., Citation2010; Herbon et al., Citation2014; Lu et al., Citation2016).See , that many scholars explore the dynamic pricing of perishable products under offline business environment.
Table 2. Dynamic pricing model under offline setting.
Besides the above offline mode of operation, some studies involve dynamic pricing and inventory control on fresh agricultural products in online retail setting. Cheng (Citation2011) established a Berman recursive equation with dynamic pricing strategy under online sale setting based on the market demand state model, and finally used a Q learning algorithm to solve the dynamic optimization problem. Wang and Dan (Citation2014) assumed that the utility function of consumers’ random demand is related to freshness and price under multi-price discount, and constructed an economic order volume model of online sales of fresh products. Cohen et al. (Citation2017) proposed a linear integer programming approximation to solve the dynamic promotional pricing problem and showed that their approach could use sales data from online retailers to increase profits by 3%. Fisher et al. (Citation2018) proposed that online retailers could easily track price changes of competitors and studied competitive dynamic pricing strategies. In addition to the above studies, many scholars conduct consumers behavior into the online sales. For example, based on the newsboy model and considering the strategic behaviors of consumers, Tang et al., (Citation2018) discretized the value decline of fresh agricultural products and bring forward a single-stage and two-stage pricing inventory decision-making model. Li et al. (Citation2015) constructed an inventory system with stochastic perturbation into a continuous time stochastic differential equation model. Using dynamic pricing and production control, a stochastic dynamic programming problem is developed to optimize the total discount profit. Hu et al. (Citation2016) also considered a joint replenishment and discount stochastic dynamic inventory model when facing forward buying customers under the condition of demand uncertainty. Bi et al. (Citation2018) explored an online sales of two interchangeable limited stock perishable products under uncertain demand. Through the above survey of fresh agricultural products’ pricing, inventory control, and consumers’ purchase behavior under online setting, we can see that most studies concentrate on the offline operation mode with different factors, and constructed a variety of types of inventory control models under different external demand environments. And scholars have gradually integrated strategic consumers’ behavior into the model. Although it posed new challenges to the management decision-making, here are only a few studies involved in the strategic behavior of consumers and online retail setting at present. Our work is consistent with above papers of introducing purchase behavior factor to describe consumer ratio so as to optimize the joint pricing and inventory management under online sale setting.
3. Model formulation
Generally, the retailer orders new fresh agricultural products to sell at a determined regular price, and clear the inventory from the regular stage at a discount price due to the perishability, simultaneously deciding a new products order for continuously retailing, when facing consumers forward purchase behavior on online setting, where we call the former sale regular stage and the latter sale discount stage. For convenience of further study, we define a period of sale consisting of two sale stages, a discount stage followed by a regular sales stage, so that the sequence of retailer selling is a dynamic pricing and inventory control issue in T periods, as shown in by setting some variables or parameters in .
Table 3. Variables/parameters in the model.
In the first stage of period t, the retailer has some inventory on hand and decides the discount price to sell. The inventory, which comes from the regular stage of previous period and is non-fresh as the perishability, cannot be carried over to the second stage and have zero salvage value if unsold at last. In the meanwhile, the retailer also decides the order quantity of fresh products for the second stage. All unsold in the regular stage are carried to the next period, which incurs holding cost. The aggregated demand in period t, denoted by Dt, is random, stationary, bounded, and independent of each other. As shown in the , during the regular sales phase, we introduce to represent the portion of consumers who attempts to make purchases in the first stage. Therefore, the market demand would be
and
during regular and discounting stage, respectively. This study also adds strategic behaviors of consumers. Among them who try to purchase in the first stage, some can buy the regular price while are attracted by the discounting price in the second stage. In line with Hu et al. (Citation2016) and Shum et al. (Citation2017), let
represent the portion of such consumers. If
the two stages of demand are independent of each other. The retailer’s pricing and inventory decisions become straightforward. We focus on the case with
that is to say, the issue in the presence of strategic consumers. The inventory at period t only comes from the regular stage since the unsold products at the first stage are all disposed of, denoted as
and obviously,
Moreover, for convenience of further study, we assume that there is no dynamic demand substitution. That is to say, when the preferred fresh agricultural products are out of stock, customers will not buy the other type of agricultural products. Consumers will choose to buy in the first stage or the second stage, and each consumer will buy at most one kind of fresh agricultural product. We also assume that the loss of returned products is related to the sales quantity of fresh agricultural products when return policy is applied, denoted as where
is the ratio of returned products, which is a random variable between [0, 1], and
is the amount of returned products.
3.2. Dynamic model of offline retailing
In the offline setting, consumers pickup and buy products on site, so that there is generally no return loss for the retailer. The retailer’s goal is to maximize its profit by dynamically adjusting inventory and determining the price in the two sale stages. The profit in phase t is:
(1)
(1)
Obviously, all profit from the start to period T is a total of every period’s profit, which is We define a discount factor
and get the recursive function
(2)
(2)
where
is state variable and
is the decision variables, generally,
Denote function as
(3)
(3)
With the state transfer equation we can obtain the dynamic programming model as below.
As a result,
(4)
(4)
The first constraint equation is boundary condition, as we assume that all the unsold product at the last period will be disposed of with unit cost k. In other words, there is no second market for these inventory that can be treated with some salvage value.
Theorem 1
: With initial inventory at any period, the expected profit function,, is quadratic differentiable and jointly concave on
Proof:
At the last period, the optimal profit function is expressed as:
Obviously, the efficient is more than zero, which leads the function to be concave, ceteris paribus, and the optimal ordering quantity is
The Hessian matrix over
is as below.
When the matrix is negative semi-definite. In this case, the profit function is jointly concave on
That is to say, there could be an optimal solution that makes the retailer earn the highest profit. Furthermore, given pricing variables,
is proportional to
This suggests that the retailer could raise or cut down the regular price and ordering quantity simultaneously. Similarly, when
the expected profit function is also jointly concave on
Theorem 2:
With initial inventory at any period,
Proof:
We prove it by induction. First, we prove that is continuously quadratic differentiable. When t = T,
which is quadratic differentiable and convex on
Substituting the boundary condition,
into
we can get
According to theorem 1, we know is continuously quadratic differentiable and jointly concave on
Assume now that
is jointly concave for some t = 1,2,…,T-1 and that
is also concave and non-increasing. Then,
is jointly concave: joint concavity of the first term is verified according thereom1 as well as for the case t = T above.
According to the structural properties of the model, the following theorems can be obtained.
Theorem 3:
With initial inventory
Proof:
On the basis of the model analyzed above, we can construct an optimization problem of any period T with constraints, as shown below:
(5)
(5)
Through KKT conditions and the previous analysis, we can get the best pricing strategy and order quantity decision of multi-variables nonlinear dynamic programming.
(6)
(6)
Assuming that is the optimal strategy, KKT conditions can be constructed as follows:
The first equation represents the first order condition of the Lagrange function, and the last two equations represent the condition of complementary relaxation. It is worth noting that the optimal strategy of order quantity depends on parameters which means that enterprises need to consider these parameters to make the optimal strategy.
We conduct numerical analysis to illustrate the solution. Let T = 10,
γ = 0.9 and initial inventory be 0. By using the optimization toolbox of Matlab software, a series of order quantity and the optimal price can be obtained, as shown in
Table 4. Optimal solution of dynamic pricing and regular pricing in offline retail.
Table 5. Optimal solutions of dynamic pricing and single pricing in online retail.
According to the final profit margin of the two decision-making strategies, the two-stage dynamic pricing is obviously better than the ordinary single pricing. And the offline retailer’s profit can increase by 6.9%, which further proves the correctness of the above theoretical analysis.
3.3. Dynamic model of online retailing
Contrary to offline setting, consumers can not observe and feel the quality of products on site, which some orders would return at last. Therefore, the retailer’s profit should consider the logistics cost and return cost except for the shortage cost and holding cost, which will make the study more comprehensive. For convenience of study, the return cost is set down as being related to the sales quantity of fresh agricultural products based on the previous research. Meanwhile, in what follows, we consider the situation where the return of goods is allowed to be resold in discount stage.
As noted above, we formulate the retailer’s profit function as below.
(7)
(7)
Similar to previous section, we define as a function of the total expected profit with discount factor
from t to T, that is, when
the dynamic programming equation is expressed as:
(8)
(8)
Through the state transfer equation define
as
(9)
(9)
Then, when
(10)
(10)
where
We still put forward the above boundary condition that all inventory unsold at last will be disposed of. That is to say, there is no second market in which the inventory can be treated with salvage value. Instead, businesses pay unit cost
to clear inventory, even of unexpired inventory products.
What is different from offline retail is the change of profit function, we first analyze the property of the model.
Theorem 4:
With initial inventory at any period, the expected profit function is continuously quadratic differentiable and jointly concave on
Proof:
When the profit function at the last period is expressed as:
The Hessian matrix on is
The Hessian matrix is obviously negative semi-definite if In this case, the profit function is concave, that is, there is a maximum value to maximize the profits of fresh agricultural products retailers. Meanwhile, ceteris paribus, the optimal ordering quantity,
As shown in the solution, the regular negatively correlated with the order quantity. Similarly, when
the result holds by the profit equation and related Hessian matrix analysis.
Theorem 5:
With initial inventory at any period, the function
Proof:
At period T, then
And
is quadratic differentiable and concave on
With property of
according to theorem 1,
is continuously quadratic differentiable and jointly concave on
That is there is an optimal decision that maximizes the profit, and it is a decreasing function.
is also decreasing on
And then suppose that when
are concave and,
are decreasing on
When
which property also holds based on theorem 1.
Theorem 6
: With initial inventory at period t, there exists an optimal solution, i.e.
Proof:
On the basis of dynamic programming model, an optimization problem of arbitrary period t under constraint conditions is constructed, as shown below:
(11)
(11)
Through KKT conditions and the previous analysis, we can get the best pricing strategy and order quantity decision of multivariables nonlinear dynamic programming.
(12)
(12)
KKT conditions can be constructed as follows:
The first equation represents the first order condition of the Lagrange function, and the last two equations represent the condition of complementary relaxation. It is not hard to find the optimal solution, It is worth noting that the optimal strategy of online retail quantity much more complicated than offline retail since more parameters, such as
are considered. Similar to previous section, we conduct some numerical experiments analysis to illustrate the performance. Besides the parameters noted earlier, we add
With the help of the optimization toolbox using Matlab software, a series of order quantities and optimal prices can be obtained, as shown in .
Not surprisingly, the data shows that the profit under dynamic pricing and inventory control could increase by about 15.13%. This is because the discounted price in the two-stage pricing changes the demand realization, conforms to different consumers’ preferences on freshness and price, and promotes sales. This further unfolds that the retailer should adopt more dynamic strategies on online retail of fresh agricultural products when there are strategic consumers. From revenue management perspective, dynamic pricing and inventory control can enhance the competitiveness of fresh e-commerce.
4. Concluding remark
This paper investigates a problem of fresh agricultural products on online retail setting with strategic consumer behavior. We formulate a stochastic dynamic optimization model on a retailer’s inventory and pricing decisions by analyzing forward purchase behavior analysis and online setting. Then exploiting the characteristics of the model and using KKT condition of nonlinear programming method we obtain the dynamic optimal policy or solution, which is comparatively analyzed to dominate the related static strategy. Some numerical experiments are conducted to illustrate the performance on the solution and confirm that there exists effective pricing and inventory strategy to maximize the profits of retailers on online selling fresh agricultural products. The results show that the profit with dynamic strategy increases, to a certain extent, compared that with single pricing of static strategy. This is because that discount pricing attracts more strategic consumers to buy, especially the dynamic pricing policy can adjust the inventory level accordingly. Meanwhile, real-time adjustment of orders can also reduce holding costs, out-of-stock costs and other operating costs, so as to improve the profit. We also show that consumers forward buying behavior will negatively influence the retailer’s profit. When the price is set too low in regular or discounting sales, the profit will show an up-down trend if the inventory exceeds a certain threshold. Meanwhile, when fresh goods returns are allowed and resold in the secondary stage, the retailer’s profit will increase.
Furthermore, this paper is a preliminary attempt to study the dynamic pricing and inventory strategy of online retail of fresh agricultural products with forward purchase behavior. By now, online sale of fresh agricultural products is developing fast. The strategic behaviors of consumers are becoming more complicated and has much impact on the market demand. In this paper we only represent it with one parameter, the portion. Meanwhile, there is no specific analysis of the relationship between the degree of freshness loss and consumers’ choice, nor a more comprehensive analysis on market demand. In the future research, studies can investigate the problem by expanding the consumer behavior and/or the stochastic distribution of market demand, or some other situations, so as to provide decision-making reference for the e-commerce industry of fresh agricultural products.
Disclosure statement
No potential conflict of interest was reported by the authors.
Notes
References
- Adenso-Díaz, B., Lozano, S., & Palacio, A. (2017). Effects of dynamic pricing of perishable products on revenue and waste. Applied Mathematical Modelling, 45, 148–164. https://doi.org/10.1016/j.apm.2016.12.024
- Akçay, Y., Natarajan, H. P., & Xu, S. H. (2010). Joint dynamic pricing of multiple perishable products under consumer choice. Management Science, 56(8), 1345–1361. https://doi.org/10.1287/mnsc.1100.1178
- Altug, M. S., & Aydinliyim, T. (2016). Counteracting strategic purchase deferrals: The impact of online retailers’ return policy decisions. Manufacturing & Service Operations Management, 18(3), 376–392. https://doi.org/10.1287/msom.2015.0570
- Angwin, J., & Mattioli, D. (2012). Coming soon: Toilet paper priced like airline tickets. Dow Jones & Company Inc.
- Banerjee, P. K., & Turner, T. R. (2012). A flexible model for the pricing of perishable assets. Omega, 40(5), 533–540. https://doi.org/10.1016/j.omega.2011.10.001
- Bi, W., Liu, C., & Liu, H. (2018). Dynamic pricing strategies for perishable products considering demand substitution and social learning. Systems Engineering, 36(01), 53–62.
- Chen, J., Dong, M., Rong, Y., & Yang, L. (2018). Dynamic pricing for deteriorating products with menu cost. Omega, 75, 13–26. https://doi.org/10.1016/j.omega.2017.02.001
- Cheng, Y. (2011). Dynamic bundling strategy of perishable products for delayed purchase in e-commerce. Systems Engineering Theory and Practice, 31(10), 1892–1902. https://doi.org/10.12011/1000-6788(2011)10-1892-11
- Chen, X., Pang, Z., & Pan, L. (2014). Coordinating inventory control and pricing strategies for perishable products. Operations Research, 62(2), 284–300. https://doi.org/10.1287/opre.2014.1261
- Chen, Y., Wang, H., & Shen, H. (2006). Research on price competition between e-commerce retailers and traditional retailers. Systems Engineering Theory and Practice, 26(1), 35–41. https://doi.org/10.3321/j.issn:1000-6788.2006.01.005
- Cohen, M. C., Leung, N. Z., Panchamgam, K., Perakis, G., & Smith, A. (2017). The impact of linear optimization on promotion planning. Operations Research, 65(2), 446–468. https://doi.org/10.1287/opre.2016.1573
- Fan, S., & Wang, X. (2018). Joint decision of online retail pricing and ordering for resale of returned goods. Systems Engineering Theory and Practice, 38(1), 113–121. https://doi.org/10.12011/1000-6788(2018)01-0113-09
- Feng, Y., Wu, Q., & Yu, Y. (2014). Study on coordination mechanism of fresh agricultural products supply chain considering physical loss and value loss. Logistics Technology, 33(11), 282–286. https://doi.org/10.3969/j.issn.1005-152X.2014.06.089
- Fisher, M., Gallino, S., & Li, J. (2018). Competition-based dynamic pricing in online retailing: A methodology validated with field experiments. Management Science, 64(6), 2496–2514. https://doi.org/10.1287/mnsc.2017.2753
- Herbon, A., Levner, E., & Cheng, T. C. E. (2014). Perishable inventory management with dynamic pricing using time–temperature indicators linked to automatic detecting devices. International Journal of Production Economics, 147, 605–613. https://doi.org/10.1016/j.ijpe.2013.07.021
- Hu, P., Lu, Y., & Song, M. (2019). Joint pricing and inventory control with fixed and convex/concave variable production costs. Production and Operations Management, 28(4), 847–877. https://doi.org/10.1111/poms.12950
- Hu, P., Shum, S., & Yu, M. (2016). Joint inventory and markdown management for perishable goods with strategic consumer behavior. Operations Research, 64(1), 118–134. https://doi.org/10.1287/opre.2015.1439
- Liu, Y., Wang, L., Feng, L., & Shi, M. Y. (2015). New exploration of reducing agricultural prices–constructing e-commerce model of fresh agricultural products. Modern Management Science, 03, 112–114.
- Li, S., Zhang, J., & Tang, W. (2015). Joint dynamic pricing and inventory control policy for a stochastic inventory system with perishable products. International Journal of Production Research, 53(10), 2937–2950. https://doi.org/10.1080/00207543.2014.961206
- Lu, L., Zhang, J., & Tang, W. (2016). Optimal dynamic pricing and replenishment policy for perishable items with inventory-level-dependent demand. International Journal of Systems Science, 47(6), 1480–1494. https://doi.org/10.1080/00207721.2014.938784
- Meng, Q., Han, X., & Yu, D. (2010). An e-commerce dynamic pricing strategy for C2C and B2C modes [Paper presentation]. 2010 2nd IEEE International Conference on Information Management and Engineering, pp. 467–470. https://doi.org/10.1109/ICIME.2010.5477765
- San-José, L. A., Sicilia, J., & García-Laguna, J. (2015). Analysis of an EOQ inventory model with partial backordering and non-linear unit holding cost. Omega, 54, 147–157. https://doi.org/10.1016/j.omega.2015.01.007
- Shi, L. (2014). Research on customers’ shopping channel choice behavior in the omni-channel era. Contemporary Finance and Economics, 02, 69–78. https://doi.org/10.13676/j.cnki.cn36-1030/f.2014.02.008
- Shum, S., Shilu Tong, S., & Xiao, T. (2017). Uncertain cost reduction when selling to strategic customers. Management Science: Journal of the Institute of Management Sciences, 63(3), 843–860. https://doi.org/10.1287/mnsc.2015.2355
- Tang, Y., Fan, T., & Liu, S. (2018). Pricing and inventory decision of fresh agricultural product considering strategic consumers. China Management Science, 26(11), 105–113. https://doi.org/10.16381/j.cnki.issn1003-207x.2018.11.011
- Tripathi, R. P., & Tomar, S. S. (2015). Optimal order policy for deteriorating items with time-dependent demand in response to temporary price discount linked to order quantity. International Journal of Mathematical Analysis, 9, 1095–1109. https://doi.org/10.12988/ijma.2015.5235
- Wang, C. (2009). Dynamic pricing of seasonal products and integrated optimization of order volume based on the degree of decay. Journal of Operations Research, 13(04), 71–82. https://doi.org/10.15960/j.cnki.iss.1007-6093.2009.04.014
- Wang, L., & Dan, B. (2014). Research on fresh agricultural products preservation and pricing strategies based on consumer choice behavior. Journal of Management, 11(03), 449–454. https://doi.org/10.3969/j.issn.1672-884x.2014.03.020
- Yao, Z., & Zhang, L. (2013). Considering pricing and ordering strategies of online enterprises when customers return goods. Journal of Management Science, 16(6), 10–21. https://doi.org/10.3969/j.issn.1007-9807.2013.06.002
- Zhang, L., & Zhang, X. (2011). Study on the impact of external shocks on the price fluctuation of agricultural products in China: From the perspective of agricultural industrial chain. Management World, 01, 71–81. https://doi.org/10.19744/j.cnki.11-1235/f.2011.01.010