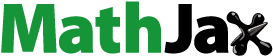
Abstract
The impact of human capital on the process of urbanization is very important. This research aims to examine the effect of heterogeneous human capital on the process of new urbanization and divides human capital into basic human capital, skill-based human capital, and knowledge-based human capital. The 2005–2019 provincial spatial panel data model analyzes the impact of heterogeneous human capital and spatial spillover effects on the process of new urbanization based on distinct spatial weight matrices. The results indicate that the three types of human capital have obvious spatial correlations with the evolution of new urbanization. Basic and skill-based human capital have a significant positive influence on boosting the process of new urbanization, while the influence of knowledge-based human capital is not obvious, and less effect on surrounding cities. In terms of regional heterogeneity, the promotion effect of human capital in the east is higher than that in the middle and west. According to the research, this paper suggests that the government should guarantee the social welfare of basic human capital and skilled human capital, because they are an important force in the construction of urbanization, and weaken the impact of academic qualifications on the introduction of urban talents.
JEL CODES:
1. Introduction
Since China’s reform and opening up, a large rural population has moved to cities, and China’s urbanization rate has risen rapidly, from 17.92% in 1978 to 60.6% in 2019 (Source: own research based on data from National Bureau of Statistics of China), with an average annual growth rate of 1.02 percentage points. Human capital is the core of urban development, and population migration and agglomeration can not only bring rapid urban development but also be an important source of modern economic growth (Glaeser et al., Citation2014). In the 1960s, Schultz (Citation1960) summarized the effect of education on human capital, which in turn impacted the economy. In the mid-1980s, neoclassical economists introduced human capital into the economic growth model and proposed "new growth theory". Human capital promotes urbanization by promoting the economic growth mode and industrial structure adjustment. The reform of the household registration system plays a positive role in the accumulation of human capital, narrowing the income gap and promoting the level of urbanization. The location policy of enhancing human capital accumulation is conducive to expanding the scale of cities, while the increase in the number of central cities is conducive to improving the quality of urbanization in China.
In 2014, the Central Committee of the Communist Party of China and the State Council of China jointly released the National Plan for New Urbanization (2014–2020), which provided the direction for China’s urban development. Different scholars have different ideas for the quality evaluation of new urbanization and have different emphases. In representative studies, most scholars select multiple measurable indicators based on six aspects: economic development quality, quality of life, social development quality, infrastructure quality, ecological environment quality, and overall urban–rural and regional coordinated development quality. They perform objective analysis to determine weights, comprehensively construct an urbanization index system, and form the ideological prototype of the urbanization evaluation method (Chen et al., Citation2018). China’s large floating population group mainly consists of migrant workers, and solving household registration for them and implementing the household registration reform system are the most important measures for promoting new urbanization (Kim, Citation2015).
China’s previous urbanization was government-led, which is a distinctive feature of traditional urbanization. New urbanization respects the laws of the market and adheres to demand orientation. This is the most significant difference between the two. We will distinguish between traditional urbanization and new urbanization in terms of development goals, development motivation and development methods. First of all, the goal of new urbanization is the all-round development of economy, society, culture and environment, while traditional urbanization only focuses on economic and social development, ignoring other factors. Secondly, new urbanization focuses on the coordinated development of urbanization, industrialization, and informatization, emphasizing the important role of innovation-driven development, and driving urbanization tends to be labor-intensive industries, lacking development momentum. Finally, new urbanization embodies a sustainable development model, while traditional urbanization places too much emphasis on the speed of development and ignores the negative impacts of rapid development, such as damage to the natural environment.
This paper analyzes the theoretical and empirical basis of the literature on the measurement of human capital in relation to urbanization and new urbanization. The marginal contribution of this research is as follows. First, most scholars use a single indicator to measure urbanization. Although this method is convenient for a simplified calculation, it cannot fully reflect the development quality of domestic cities. Therefore, this study constructs a new urbanization indicator system to widely investigate the development quality of urbanization in various regions. Second, various types of human capital are differentiated, and the mechanism of the impact of three kinds of human capital (basic human capital, skill-based human capital, and knowledge-based human capital) on new urbanization is explored. Third, this paper provides a new way of thinking for exploring the relationship between human capital and urbanization, because human capital has strong mobility, and the spatial econometric model can better analyze the spatial correlation between human capital and urbanization. Therefore, in this paper, based on a spatial econometric model, the empirical part of this paper uses two different spatial weight matrices (geographical distance and economic distance) to analyze the spatial transmission mechanism of heterogeneous human capital and to measure the spatial spillover effect. The rest of this paper is structured as follows: the second section conducts the literature review, the third section describes the research method and model setting, the fourth section presents the data, the fifth section performs the empirical analysis, and the sixth section draws the conclusion and policy recommendations.
2. Literature review
This review of the literature on human capital and urbanization mainly focuses on two aspects: studies on the causal relationship among human capital, urbanization, and economic growth and the studies on the influence of human capital and urbanization on the ecological environment.
Urbanization refers to the migration of the rural population to cities and the transformation of the industries engaged in from the primary industry to the secondary industry and the tertiary industry (Lucas, Citation2014). This means that population and capital gather in cities, which promotes the improvement in urban infrastructure and social public services (Zhou et al., Citation2015). Urbanization promotes economic growth through the accumulation of physical capital, human capital and intellectual capital, and economic growth and urbanization are mutually reinforcing (Liang & Yang, Citation2019). By analyzing low-income and middle-income areas in different countries, Flückiger and Ludwig (Citation2018) found that there is a close relationship between human capital and urbanization and that this relationship is an important factor in promoting regional economic development. As one of the most important assets of cities, human capital can actively promote economic development (Mingaleva et al., Citation2017). Hansen and Winther (Citation2014) pointed out that urbanization can promote human capital formation and employment growth, which in turn promotes economic development. Convenient infrastructure is very attractive to high-skilled human capital, and the innovative ability of high-skilled human capital plays an important role in urban development (Storper & Scott, Citation2009). Therefore, establishing a sound infrastructure and promoting sustainable urban development have become an important direction for China’s future urban development (Shen & Zhou, Citation2014).
However, the rapid development of urbanization also brings various problems, such as environmental degradation, energy depletion and food insecurity (Li et al.,Citation2017; Liu et al., Citation2017; Wille et al., Citation2017). The impact of human capital and urbanization on the ecological environment is a one-way causal relationship, and the causality between human capital, urbanization and economic growth is bidirectional (Ahmed et al., Citation2020). Nathaniel et al. (Citation2021) pointed out the environmental degradation caused by urbanization and economic growth in countries close to the Amazon because of their reliance on deforestation for agriculture and mining, which increases carbon dioxide emissions. In China, rapid economic growth has led to urbanization and population expansion, boosting household consumption growth, while the increases in urbanization, human capital and household consumption have all contribute to the increase in carbon emissions (Liu et al., Citation2011). Hossain (Citation2011) argued that newly industrialized countries emit more carbon dioxide as they urbanize, but this is normal in the long run. By analyzing panel data on 23 countries in Europe, Al-Mulali et al. (Citation2015) found that both urbanization and economic growth significantly contribute to carbon emissions.
In addition, scholars have conducted analyses from the perspective of the ecological environment and argued that improving China’s new urbanization process can reduce environmental pollution and facilitate energy efficiency, which has a clear positive role in improving the environment (Lin & Zhu, Citation2021; Yu, Citation2021). Wu et al. (Citation2019) argued that the relationship between urbanization and energy consumption is nonlinear and that China’s urbanization process is closely related to energy support. However, as the scale of energy consumption increases, this positive effect gradually weakens, and energy structure upgrading will significantly enhance the positive effect.
3. Methods and models
3.1. Spatial econometric model
This paper employs the Cobb–Douglas production function model as the basic framework to build the function model of new urbanization and human capital:
(1)
(1)
Taking the logarithm of both sides of EquationEquation (1)(1)
(1) simultaneously, we obtain the following:
(2)
(2)
where UR is the quality evaluation index of new urbanization.
is the human capital of province i in year t, including basic human capital, skill-based human capital and knowledge-based human capital.
is an error term; OPEN, EDU, MAR and RD are control variables.
A spatial autoregressive (SAR) model is a model in which the explained variables are set. It is appropriate for researching the mutual influence and spillover effects of economic behavior between neighboring regions. The formula is as follows:
(3)
(3)
When all the test results are significant, the spatial Durbin model (SDM) is a better choice; that is, it includes both the spatial lag term of the response variable and the spatial lag term of the explained variable. The SDM takes the following form:
(4)
(4)
where
is the dependent variable (endogenous variable),
is the independent variable (exogenous variable), and
is a random error term.
3.2. Spatial weight matrix
This paper adopts a geographical distance matrix and an economic distance matrix to objectively measure the impact of human capital on new urbanization, and it uses a geographical adjacency matrix to measure Moran’s I. In this paper, the linear Euclidean distance of provincial capitals is used to measure the geographical distance between province
and province
The equation is as follows:
(5)
(5)
Although the geographical distance between provinces can directly reflect the spatial relationship, it is insufficient to show the internal connections in their social and economic relations. Inevitably, deviations will occur when only a geographical distance matrix is adopted to measure the influence of provincial human capital on new urbanization. To pursue rigor and scientificity, this paper adopts per capita GDP as the matrix element to construct the economic distance matrix, as shown in EquationEquation (4)(4)
(4) :
(6)
(6)
where
(per capita GDP of province
) in period
and
3.3. Direct effect and spatial spillover effect
Based on the ordinary least squares (OLS) model, the SEM has three influences: endogenous, exogenous and error term influence. The direct effect refers to the effect of endogenous variables on the explained variable, and the spillover effect refers to the effect of exogenous variables on the explained variable (Elhorst, Citation2014). However, not all spatial regression models can measure the regional spillover effect. According to the definition of the spillover effect by Vega and Elhorst (Citation2015), only the SAR model and SDM can reflect the regional spillover effect. The simplification of the SDM model is shown below:
(7)
(7)
where
is the n-order identity matrix,
is the
-order matrix, and EquationEquation (7)
(9)
(9) can be obtained after transformation:
(8)
(8)
where
and
Convert to the matrix form:
(9)
(9)
where the mean value of the diagonal elements is the direct effect and the mean value of each nondiagonal element in each row or column is the spillover effect (indirect effect). There are two ways to calculate direct and spillover effects. The first method uses a partial derivative matrix to calculate the direct or spillover effect of each sampling process and then calculates the mean value extracted D times. Since the matrix operation is time consuming and the calculation process is complicated when N is large, the second method is generally used. The following decomposition formula is used:
(10)
(10)
By presetting the times of W and ρ on the right side of the equation, the tedious process of finding the inverse matrix for matrix can be simplified, and the direct effect and spillover effect of each sample can be better reflected. The direct effect, spillover effect and total effect are denoted as
and
respectively.
3.4. Research hypothesis
According to Tobler’s First Law of Geography, Everything is related to everything else, but near things are more related to each other. The energy efficiency is affected by the urbanization of other adjacent provinces in China, and there are positive indirect spillover effects (Li et al., Citation2018). By analyzing the spatial transmission mechanism of China’s urbanization and carbon emissions, Wang et al. (Citation2022) found that human capital and urbanization have spatial spillover effects on carbon emissions. The improvement of air quality in neighboring cities can increase the stock of local human capital, thereby promoting urban development, and there is an obvious spatial spillover effect (Le et al., Citation2022). According to these studies, The impact of intra-regional human capital on new urbanization should be affected by near regions, and the regional spatial spillover effect is an important manifestation of inter-regional effects. Therefore, I derive the following Hypothesis 1:
Hypothesis: The impact of heterogeneous human capital on new urbanization has spatial spillover effects.
Hypothesis. There is a positive relationship between heterogeneous human capital and China’s new urbanization.
4. Data
4.1. New urbanization index system
4.1.1. Index selection
Based on existing studies (Sun et al., Citation2017; Yu, Citation2021; Wei et al., Citation2015) and following the principles of scientificity, representativeness and comprehensiveness, this paper selects 25 specific measurement indicators across six categories, i.e. population urbanization, economic development and efficiency, the ecological environment, infrastructure, public services and urban–rural overall planning, to construct a quality evaluation system for new urbanization ().
Table 1. The index system for new urbanization.
4.1.2. Indicator interpretation
Population urbanization and new urbanization take human capital as the core and focus on high levels, high harmony, high quality and high inclusiveness. Different from traditional urbanization centered on land, in the process of promoting new urbanization, the migration of rural populations to cities has played an important role; thus, employment and quality of life must be fully guaranteed (Chen et al., 2021; Guan et al., Citation2018; Liu et al., Citation2017).
Since entering the new era, China has gradually realized the stage of economic development marked by the transition from high-speed to high-quality development. The economical and efficient recyclable development mode is suitable for the current economic development mode of Chinese cities. Electricity consumption per unit GDP and energy consumption per unit GDP reflect the efficiency of economic development. The indicators of the proportion of the added value of the tertiary industry, per capita GDP and the per capita disposable income of urban residents reflect the quality of the urban economic process.
The ecological environment, based on the concept of an ecological civilization for new urbanization development, is intended to meet the requirements of the new era (e.g. energy conservation, garbage classification, and strict control of carbon emissions) to create a sustainable ecological environment for residents to live in. The ecological environment will have an increasingly important role in new urbanization quality measures, embodying the carrying capacity of cities and reflecting the potential of urban sustainable development.
The equalization of infrastructure and urban development is inseparable from infrastructure construction, and urban infrastructure construction can provide strong support for population agglomeration and economic development and improve the quality of life of urban residents.
The most significant difference between new urbanization and traditional urbanization is whether the former can meet people’s material and spiritual needs. To meet these needs, one avenue is through the equalization of public services. Traditional urbanization is simply the migration of people from rural to urban areas, and the diverse needs of people are often not met. New urbanization is more important than making residents more “comfortable”; it works to improve their quality of life.
The coordinated development of urban and rural areas, at the same time as urban construction, must speed up the construction of a new era of a beautiful new socialist countryside hand in hand with the development of both urban and rural areas to narrow the gap in living standards.
4.1.3. Index weights
There are subjective and objective methods to determine the index weight. Subjective weighting methods are based on the subjective consciousness of the evaluator. Since the quality evaluation system for new urbanization has many indicators and is highly comprehensive, subjective weighting methods are not suitable. Principal component analysis and the entropy weight method are both objective analysis methods for determining index weight. However, in principal component analysis, it must be ensured that the first few principal components extracted reach a certain cumulative contribution rate. Moreover, the extracted principal components must be explained in line with the actual background and meaning; otherwise, there will be no actual meaning. Therefore, this paper chooses the entropy weight method to determine the index weights of new urbanization (Yu, Citation2021).
The entropy weight method judges the dispersion degree of an index. The greater the dispersion degree is, the greater the uncertainty and the less information is contained. Based on the entropy values of different indices, the weights can be determined, and the comprehensive score can be calculated. The specific steps are as follows:
Carry out a standardized processing of the indicators. Because the selected indicators are different in terms of their dimension, size, positivity and negativity, it is necessary to standardize the indicators:
(11)
(11)
(12)
(12)
Calculate the specific gravity of item in year
in region
as follows:
(13)
(13)
Calculate the entropy value:
(14)
(14)
where
Calculate the redundancy of each index:
(15)
(15)
Calculate the weight of each indicator:
(16)
(16)
Calculate the comprehensive score of each index:
(17)
(17)
The above calculation shows the average comprehensive score and ranking of the quality of new urbanization of 30 provinces, municipalities directly under the central government and autonomous regions in China from 2005 to 2019, as measured by the entropy weight method (Tibet is not included in this research because of missing data). The results are shown in .
Table 2. Average comprehensive score and ranking of the quality of new urbanization in China.
As shown in the table, the overall quality of new urbanization in China is not high, but the development potential is enormous. Only two megacities, Beijing and Shanghai, as political and economic centers, respectively, scored over 0.7 in the overall quality of new urbanization, which is consistent with the actual situation. The overall score of the quality of new urbanization in 19 provinces is less than 0.3, and most of these provinces are in the central and western regions. Overall, the situation of China’s new urbanization process is high in the east, low in the west, strong in the south and weak in the north.
4.2. Variable selection
In the selection of variables, the principles of scientificity, representativeness and operability are followed, and the explanatory variables and explained variables in this paper are selected based on the literature, as shown in . The explained variable is the general score of new urbanization, and the explained variable includes three types of human capital (basic human capital, knowledge-based human capital and skill-based human capital). Of course, the new city urbanization process is a complex dynamic process. To make a new type of city urbanization process to promote fairness and reasonableness, other factors must be considered to play a pivotal role in the new urbanization process. Thus, this paper selects the degree of openness to the outside world, the degree of marketization, R&D intensity and educational level as control variables. The degree of openness to the outside world and the degree of marketization represent urban economic development and system construction, respectively. R&D intensity reflects the innovation capacity of a city. Educational level reflects the comprehensive strength of a city.
Table 3. Description of the variables used in the model.
This paper refers to the method of measuring heterogeneous human capital in the literature (Huang et al., Citation2021). For human capital, the human capital level is measured by the number of years of education. The specific calculation process is as follows:
Basic human capital:
(18)
(18)
Skill-based human capital:
(19)
(19)
Knowledge-based human capital:
(20)
(20)
where
is basic human capital,
is skill-based human capital,
is knowledge-based human capital, and
represents the 7 educational level groups, i.e. illiterate, primary school, junior middle school, senior high school, junior college, undergraduate and postgraduate.
is the proportion of educated people in the total population, and
is the corresponding number of years of education.
This study constructs panel data on 30 Chinese provinces (municipalities and autonomous regions, excluding Tibet, Hong Kong, Macao and Taiwan) from 2005 to 2019. The data are mainly from the China Statistical Yearbook (2006–2020), China Labor Statistics Yearbook (2005–2020), and China Education Statistics Yearbook (2006–2020). In this paper, the total amount of the import and export trade is converted based on the average exchange rate of the year. The descriptive statistics of each variable are shown in .
Table 4. Summary statistics of the variables.
5. Empirical analysis
5.1. Spatial correlation
First, GeoDa software is used to test the spatial correlation between the three kinds of human capital and new urbanization. To facilitate calculation, the first-order Rook adjacency spatial weight matrix is adopted. shows the spatial Moran’s I of basic human capital, knowledge-based human capital and skill-based human capital and the urbanization level. As shown in the figure, the spatial correlation between the three kinds of human capital and the urbanization process is very significant, and all of them are significant at the 5% level. Basic human capital has a negative spatial autocorrelation with cities and towns; skill-based and knowledge-based human capital have a positive spatial autocorrelation with urbanization. Positive spatial autocorrelation means that the values of variables in adjacent areas are similar; negative spatial autocorrelation means that the values of variables in adjacent areas are significantly different. First, Moran’s I of basic human capital and the level of new urbanization has been rising in waves since 2005, from −0.1223 in 2005 to −0.2335 in 2019, because the level of new urbanization is highly correlated with economic development, infrastructure and public service improvement. Most basic human capital is in urban construction work, which also has an increasing degree of social openness, and the population flows more easily. The provincial spatial autocorrelation increases, while the negative spatial autocorrelation decreases because less fundamental human capital is necessary to achieve higher levels of education, certain skills and knowledge are lacking, the traditional ideology is serious, and workers want to work near home. Therefore, people will choose to work in a nearby province with a more developed economy. Second, Moran’s I of skill-based human capital and the urbanization level shows a trend of developing from stable to fluctuating. From 2005 to 2009, Moran’s I increased from 0.2456 to 0.3125 and decreased to 0.1852 by 2019. The correlation between the two is significant.
5.2. Estimation results
Referring to the spatial econometric test ideas adopted by most scholars, this paper adopts Moran’s I, the Hausman test, the Lagrange multiplier (LM) test, the log likelihood ratio (LR) test and the Wald test to select spatial models. The Moran’s I results are shown above, indicating the existence of spatial autocorrelation. Based on EquationEquations (3)(3)
(3) and Equation(4)
(4)
(4) , using Stata software to analyze the spatial model, the two kinds of spatial weight matrices (geographical distance and economic distance) under the LM test, LR test, and Wald test () strongly reject the null hypothesis at the 1% level of significance. These results show that the SDM is more suitable for the empirical analysis in this paper than the SEM and the spatial lag model. The Hausman test result is significant at the 1% level, rejecting the null hypothesis and indicating that the fixed effect model is more suitable for the analysis in this paper. Furthermore, for the two spatial weight matrices, the LR joint significance test results are significant at the 1% level. This result indicates that this paper should adopt a time and individual two-way fixed effect model for empirical analysis.
Table 5. LM test, LR test and Wald test for the two spatial weight matrices.
shows the regression results of the SDM based on geographical distance and economic distance. Model (1) is a random effect model, and Model (2) is a fixed effect model. All control variables are added to both models. The regression results show that basic human capital is not very significant in the random effect model, and its significance is weaker than that in the fixed effect model. There is no significant difference in the significance of skill-based human capital and knowledge-based human capital in the two models. And basic human capital and skilled-based human capital show a positive promotion effect, hypothesis 2 is verified.
Table 6. SDM results based on random effect and fixed effect models.
The estimation results of the SDM based on the geographical distance matrix and economic distance matrix are shown in . From the estimation results, Model (3) is a basic regression, while Model (4) controls for more relevant variables in the regression results. According to the analysis of the two models, basic human capital and skill-based human capital both play a significant positive role in the quality of new urbanization, and they are both significant at the 1% level. In addition, under different spatial matrices, the influence degree is different. According to the estimation results of the two models, the spatial regression coefficient of human capital under the geographical distance matrix is higher than that under the economic distance matrix, indicating that spatial distance has a greater influence on the quality of new urbanization. The fitting degree of Model (3) is significantly lower than that of Model (4). Therefore, this paper should control for variables that have a direct effect on new urbanization. However, in the two models, knowledge-based human capital is not significant, meaning that only basic human capital and skill-based human capital have a positive spillover effect on new urbanization.
Table 7. SDM regression results.
According to the Report on China’s Education and Human Resources issued by the Ministry of Education, the average educational level of those engaged in manufacturing and construction is junior high school; that is, most of those engaged in manufacturing and construction represent basic human capital. Thus, their influence on urban construction should be greater. The regression results also show that based on the two spatial weight matrices, the spatial regression coefficients of basic human capital (1.112, 0.725) are all due to skill-based human capital (0.518, 0.37), indicating that basic human capital has a greater impact on new urbanization than skill-based human capital. Basic human capital based on geographical distance (1.112) is also higher than basic human capital based on economic distance (0.725), indicating that those representing basic human capital prefer to work closer to home rather than farther away for higher returns. The spatial lag of basic human capital is also significant at the 5% level, showing a positive spatial spillover effect.
Moreover, skill-based human capital plays a positive role in promoting the level of new urbanization. From the regression results, the influence of geographical distance (0.518) is greater than the influence of economic distance (0.37) because the popularity of high school and college is increasing (as social progress continues). Thus, more people are now receiving a college education. At the same time, the demand for skilled talent in the workforce has gradually decreased; thus, most people will choose local employment. Therefore, geographical factors have a greater impact on such human capital. The spatial lag term has a positive effect but is not significant. Knowledge-based human capital based on the two spatial distance matrices is not effective, and the spatial lag term is not effective. In the process of promoting high-quality new urbanization, the joint effect of the three types of human capital is needed. Basic human capital is responsible for most urban infrastructure construction, and the improvement in infrastructure will attract skill-based human capital and knowledge-based human capital to flow to cities. Therefore, high-skilled human capital will drive urban economic development and accelerate the improvement in urban infrastructure. Among the other control variables, the degree of marketization (mar), R&D investment intensity (RD) and educational level (edu), based on the two weight matrices, are all significant at the 1% level, while the degree of openness (open) is not significant. Next, we will explore the mechanisms of the effect of heterogeneous human capital on new urbanization. After adding the control variables, the role of basic human capital and skilled human capital in promoting new urbanization is more obvious. The increase in local education level (edu) improves the level of human capital and enhances the impact of human capital on the construction of new urbanization. The increase in investment intensity (RD) has a positive impact on technological progress, improves production efficiency, and promotes the transformation of industrial structure, thereby affecting new urbanization. The improvement of the degree of marketization (mar) will optimize the allocation of factors, enable heterogeneous human capital to engage in work with a higher return on production, improve work efficiency, and promote the development of new urbanization.
5.3. Spatial spillover effect
The regression results of the SDM model cannot directly explain the impact of the independent variable on the dependent variable. Thus, it is necessary to introduce the total effect, direct effect and spatial spillover effect (indirect effect) to analyze the degree of influence of the independent variable on the dependent variable, as shown in . The results show that the direct effect and total effect of basic and skill-based human capital under the two weight matrices are very significant; however, knowledge-based human capital is still not significant. Specifically, first, the direct effect (1.104) and total effect (1.915) of basic human capital under geographical distance are both higher than those under economic distance (0.761, 1.706). These results indicate that the impact of such human capital on promoting local urbanization is higher than that on promoting surrounding areas and that the impact of geographical distance is higher than that of economic distance. However, the spillover effect of geographical distance is not significant, and the spillover effect based on economic distance (0.945) accounts for more than 50% of the total effect and has a positive driving effect. These results indicate that the spatial spillover effect caused by geographical distance has an important promoting effect on the development of new urbanization in surrounding areas with close economic ties. Second, skill-based human capital has a notable effect on the local urbanization process, but the spillover effect on neighboring provinces is not significant, and the spatial correlation of knowledge human capital is weak, which is consistent with the previous regression analysis. Third, the indirect and direct effects of the marketization level and R&D investment intensity are significant positive effects, and the spillover effect is higher than the direct effect. These results indicate that the marketization level and R&D improvement have an obvious driving effect on the urbanization process in neighboring provinces. The three effects of educational level based on geographical distance are all significantly negative, indicating that people with higher educational levels will choose to work in places with higher income returns, thus hindering the development of local cities to a certain extent and causing brain drain, which is basically consistent with reality. Overall, the spatial spillover effect of heterogeneous human capital on new urbanization is not significant based on geographical distance, and hypothesis 1 is not verified.
Table 8. Estimation results of the spillover effects.
5.4. Heterogeneity analysis
According to the regional classification standard of the National Bureau of Statistics of China, this paper divides 30 provinces into three regions: east, middle, and west, and performs regression based on the spatial Durbin model. From the regression results in , the spatial autocorrelation coefficients of basic human capital (0.279) and skilled-human capital (0.387) in the eastern region are significantly positive, indicating that there is a significant positive spatial effect on the construction of new urbanization. As for knowledge-based human capital (-0.045), there is a negative spatial effect, indicating that high-quality talents are more mobile and will choose regions with higher returns on production. While the spatial autoregressive coefficients of basic human capital (0.470) and skilled-human capital (0.119) in the western region are significantly positive, knowledge-based human capital is not significant, and the significance in the middle region is weak. Because the economic development of the middle and western regions behind that of the eastern regions, there is vicious competition among regions, and the efficiency of resource allocation is low, resulting in obvious regional differences. ()
Table 9. Heterogeneity test results.
Table 10. Robustness test results.
5.5. Robustness test
Considering the endogeneity of the core explanatory variables, this paper uses the instrumental variable method to deal with it and uses the first-order lag of the core explanatory variables as the instrumental variables for regression. Among them, lnhum1_lag(1), lnhum1_lag(2), and lnhum1_lag(3) are the first-order lag terms of lnhum1, lnhum2, and lnhum3, respectively. The results in the table below show that the coefficient sign of basic human capital has not changed; however, its significance has changed. The significance and coefficient sign of skill-based human capital have not changed much, and knowledge-based human capital is not significant, which is basically the same as the regression results above.
6. Discussion
In this study, we analyze the spatial impact of heterogeneous human capital on new urbanization. We found that basic and skilled human capital play a role in promoting the process of new urbanization. In addition, the spatial spillover effect of human capital on new-type urbanization based on economic distance is higher than that of geographical distance, indicating that people are more inclined to work in cities with better economic development. In further research, we have drawn many valuable conclusions from the perspective of regional heterogeneity, which are worthy of further exploration.
We divide China into three regions: East, Middle and West. On the whole, the heterogeneity of heterogeneous human capital in the eastern region has the most obvious spatial impact on new urbanization, while that in the central and western regions are weaker. This may be because the economic development of the eastern region is ahead of that of the central and western regions. The labor remuneration rate is higher, the infrastructure is well-developed, the transportation is convenient, and it is more attractive to talents. Therefore, it can quickly accumulate human capital and promote new urbanization. In contrast, the middle and western regions have a poor geographical location. Many cities lack pillar industries to promote economic development. The connections between cities are not strong. It is difficult to form urban agglomerations to drive regional development. There is a lack of effective market competition mechanism. Resource-based cities The proportion is relatively high, so the impact of human capital on the construction of new urbanization is relatively weak.
From the perspective of heterogeneous human capital, the impact of basic human capital on new urbanization in the eastern, central, and western regions is significantly positive, which is closely related to China’s vigorous promotion of infrastructure construction, which is related to construction projects. Workers are low-skilled human capital. However, skill-based human capital and knowledge-based human capital have a significant impact on urbanization in the eastern region, but not in the middle and western regions. These two types of human capital have a high level of skills and tend to work in the eastern region with higher wages. They also promote social, economic, and cultural development and are an important driving force for improving the quality of urban development.
Jiang et al. (Citation2022) found the similar results to this paper by using the spatial econometric model. The agglomeration of human capital is conducive to improving the city’s ability to resist crises and promote the development of urbanization, and the impact of human capital on urbanization has a positive spatial spillover effect. However, this paper argues that the spatial spillover effect of human capital on new urbanization is not obvious. Therefore, further research is needed in the future.
7. Conclusions
Through empirical testing, this research finds that, first, the eastern coastal region is the most developed regions in terms of China’s new urbanization, while the overall development of the central and western regions is poor. Second, heterogeneous human capital in China has an obvious spatial correlation with the process of new urbanization. Basic human capital shows negative spatial autocorrelation, the other two kinds of human capital show positive spatial autocorrelation, and the spatial autocorrelation of the three kinds of human capital generally shows a spiral rise. Third, basic human capital and skill-based human capital generally play a positive role in promoting the impact of new urbanization, while basic human capital has a greater effect. Based on the influence of the two weight matrices, the impact of the two kinds of human capital on new urbanization based on geographical distance is greater than that based on economic distance. Fourth, both the direct effect and the total effect of basic human capital and skill-based human capital are significantly positive, but most of the spatial spillover effect is not obvious, based on economic distance and geographical distance having little difference.
Human capital has played a significant role in the existing urbanization process in China. Under the background of slowing economic growth, China has proposed the development strategy of new urbanization. Various types of human capital will continue to provide labor and intellectual security for the path of urbanization. This paper makes the following suggestions: Firstly, the government should accelerate the promotion of the settlement guarantee of the permanent population among migrant workers; as new urbanization affects the largest human capital group, it should safeguard their rights. Secondly, the "talent attraction policy" issued by various places should no longer take people’s academic background as the sole rigid standard. People with professional skills have a positive and important impact on urban construction, and specific types of talent should be included in the scope of talent attraction. Finally, highly educated knowledge-based human capital is an important national source of innovation, but with higher education, those with human capital mostly choose jobs in large cities. The government should guide the migration of knowledge-based human capital to developing cities and provide more employment opportunities and preferential policies.
This paper still has certain limitations. There are insufficient analyses on the mechanism of the influence of heterogeneous human capital on new urbanization. Future research needs to explore the mechanism of action in depth, focusing on how heterogeneous human capital affects the construction of new urbanization.
The past experience of urban development in China shows that urbanization is the main driving force for economic development and poverty eradication in rural areas, and it is also an important way to realize the great rejuvenation of the Chinese nation (Guan et al., Citation2018). However, there are still deficiencies in the research on new urbanization. Urbanization is a cutting-edge interdisciplinary subject, and it is necessary to establish an urbanization science in Chinese universities (Chen, Citation2015; Solecki et al., Citation2013). Since this is a new policy proposed by the Chinese government, it is difficult to learn from the experience of urban development in developed countries abroad, which is also an important reason for limiting future research. Therefore, the development of China’s new urbanization still has a long way to go.
Disclosure statement
The authors declare no conflict of interest.
Additional information
Funding
References
- Ahmed, Z., Zafar, M. W., Ali, S., & Danish. (2020). Linking urbanization, human capital, and the ecological footprint in G7 countries: an empirical analysis. Sustainable Cities and Society, 55, 102064. https://doi.org/10.1016/j.scs.2020.102064
- Al-Mulali, U., Ozturk, I., & Lean, H. H. (2015). The influence of economic growth, urbanization, trade openness, financial development, and renewable energy on pollution in Europe. Natural Hazards, 79(1), 621–644. https://doi.org/10.1007/s11069-015-1865-9
- Bertinelli, L., & Black, D. (2004). Urbanization and growth. Journal of Urban Economics, 56(1), 80–96. https://doi.org/10.1016/j.jue.2004.03.003
- Black, D., & Henderson, V. (1999). A theory of urban growth. Journal of Political Economy, 107(2), 252–284. https://doi.org/10.1086/250060
- Chen, M. (2015). Research progress and scientific issues in the field of urbanization. Geographical Research, 34(4), 614–630. (in Chinese) https://doi.org/10.11821/dlyj201504002
- Chen, M., Liu, W., Lu, D., Chen, H., & Ye, C. (2018). Progress of China’s new-type urbanization construction since 2014: A preliminary assessment. Cities, 78(8), 180–193. https://doi.org/10.1016/j.cities.2018.02.012
- Elhorst, J. P. (2014). Spatial econometrics: from cross-sectional data to spatial panels. Springer. https://doi.org/10.1007/978-3-642-40340-8-1
- Flückiger, M., & Ludwig, M. (2018). Geography, human capital and urbanization: A regional analysis. Economics Letters, 168, 10–14. https://doi.org/10.1016/j.econlet.2018.03.027
- Glaeser, E. L., Ponzetto, G. A. M., & Tobio, K. (2014). Cities, skills and regional change. Regional Studies, 48(1), 7–43. https://doi.org/10.1080/00343404.2012.674637
- Guan, X., Wei, H., Lu, S., Dai, Q., & Su, H. (2018). Assessment on the urbanization strategy in China: Achievements, challenges and reflections. Habitat International, 71, 97–109. https://doi.org/10.1016/j.habitatint.2017.11.009
- Hansen, H. K., & Winther, L. (2014). Regional development and the impact of the public sector in Denmark: Employment growth and human capital. Geografisk Tidsskrift-Danish Journal of Geography, 114(2), 156–168. https://doi.org/10.1080/00167223.2014.952750
- Hossain, M. S. (2011). Panel estimation for CO2 emissions, energy consumption, economic growth, trade openness and urbanization of newly industrialized countries. Energy Policy, 39(11), 6991–6999. https://doi.org/10.1016/j.enpol.2011.07.042
- Huang, C., Zhang, X., & Liu, K. (2021). Effects of human capital structural evolution on carbon emissions intensity in China: A dual perspective of spatial heterogeneity and nonlinear linkages. Renewable and Sustainable Energy Reviews, 135, 110258. https://doi.org/10.1016/j.rser.2020.110258
- Jiang, J., Zhang, X., & Huang, C. (2022). Influence of population agglomeration on urban economic resilience in China. Sustainability, 14(16) 10407. https://doi.org/10.3390/su141610407
- Kim, J. U. (2015). A bumpy road to cities: Analysis of the obstacles and limits of China’s new urbanization. Pacific Focus, 30(3), 372–388. https://doi.org/10.1111/pafo.12057
- Le, D., Li, Y., & Ren, F. (2022). Does air quality improvement promote enterprise productivity increase? Based on the spatial spillover effect of 242 cities in China. Frontiers in Public Health, 10, 1050971. https://doi.org/10.3389/fpubh.2022.1050971
- Li, G., Lei, Y., Yao, H., Wu, S., & Ge, J. (2017). The influence of land urbanization on landslides: An empirical estimation based on Chinese provincial panel data. Science of the Total Environment, 595, 681–690. https://doi.org/10.1016/j.scitotenv.2017.03.258
- Li, K., Fang, L., & He, L. (2018). How urbanization affects China’s energy efficiency: A spatial econometric analysis. Journal of Cleaner Production, 200, 1130–1141. https://doi.org/10.1016/j.jclepro.2018.07.234
- Liang, W., & Yang, M. (2019). Urbanization, economic growth and environmental pollution: Evidence from China. Sustainable Computing: Informatics and Systems, 21, 1–9. https://doi.org/10.1016/j.suscom.2018.11.007
- Lin, B., & Zhu, J. (2021). Impact of China’s new-type urbanization on energy intensity: A city-level analysis. Energy Economics, 99, 105292. https://doi.org/10.1016/j.eneco.2021.105292
- Liu, L., Wu, G., Wang, J., & Wei, Y. (2011). China’s carbon emissions from urban and rural households during 1992–2007. Journal of Cleaner Production, 19(15), 1754–1762. https://doi.org/10.1016/j.jclepro.2011.06.011
- Liu, M., Liu, X., Huang, Y., Ma, Z., & Bi, J. (2017). Epidemic transition of environmental health risk during China’s urbanization. Science Bulletin, 62(2), 92–98. https://doi.org/10.1016/j.scib.2016.12.004
- Liu, Y., Xiao, H., Lv, Y., & Zhang, N. (2017). The effect of new-type urbanization on energy consumption in China: A spatial econometric analysis. Journal of Cleaner Production, 163, S299–S305. https://doi.org/10.1016/j.jclepro.2015.10.044
- Lucas, J. R. E. (2014). Life earnings and rural-urban migration. Journal of Political Economy, 112(1), 29–59. https://doi.org/10.1086/379942
- Mingaleva, Z., Karpovich, Y., & Kozlova, O. (2017). Features of human capital in urban territories. Journal of Fundamental and Applied Sciences, 9(2S), 1518–1531. https://doi.org/10.4314/jfas.v9i2s.859
- Nathaniel, S. P., Nwulu, N., & Bekun, F. (2021). Natural resource, globalization, urbanization, human capital, and environmental degradation in Latin American and Caribbean countries. Environmental Science and Pollution Research, 28(5), 6207–6221. https://doi.org/10.1007/s11356-020-10850-9
- Schultz, T. (1960). Capital formation by education. Journal of Political Economy, 69, 571–583. https://doi.org/10.1086/258393
- Shen, L., & Zhou, J. (2014). Examining the effectiveness of indicators for guiding sustainable urbanization in China. Habitat International, 44, 111–120. https://doi.org/10.1016/j.habit-atint.2014.05.009
- Solecki, W., Seto, K. C., & Marcotullio, P. J. (2013). It’s time for an urbanization science. Environment: Science and Policy for Sustainable Development, 55(1), 12–17. https://doi.org/10.1080/00139157.2013.748387
- Storper, M., & Scott, A. J. (2009). Rethinking human capital, creativity nd urban growth. Journal of Economic Geography, 9(2), 147–167. https://doi.org/10.1093/jeg/lbn052
- Sun, D., Zhou, L., Li, Y., Liu, H., Shen, X. Y., Wang, Z., & Wang, X. (2017). New-type urbanization in China: Predicted trends and investment demand for 2015–2030. Journal of Geographical Sciences, 27(8), 943–966. https://doi.org/10.1007/s11442-017-1414-4
- Vega, S. H., & Elhorst, J. P. (2015). The SLX model. Journal of Regional Science, 55(3), 339–363. https://doi.org/10.1111/jors.12188
- Wang, X., Zhou, D., & Telli, S. (2022). The impact of semi-urbanization on carbon emissions: A spatial econometric perspective. Environmental Science and Pollution Research, 29(36), 54718–54732. https://doi.org/10.1007/s11356-022-19709-7
- Wei, Y., Huang, C., Li, J., & Xie, L. (2015). An evaluation model for urban carrying capacity: A case study of China’s mega-cities. Habitat International, 53, 87–96. https://doi.org/10.1016/j.habitatint.2015.10.025
- Wille, M., Lindqvist, K., Muradrasoli, S., Olsen, B., & Jarhult, J. D. (2017). Urbanization and the dynamics of RNA viruses in Mallards (Anas platyrhynchos). Infection, Genetics and Evolution, 51, 89–97. https://doi.org/10.1016/j.meegid.2017.03.019
- Wu, H., Hao, Y., & Weng, J. H. (2019). How does energy consumption affect China’s urbanization? New evidence from dynamic threshold panel models. Energy Policy, 127, 24–38. https://doi.org/10.1016/j.enpol.2018.11.057
- Yu, B. (2021). Ecological effects of new-type urbanization in China. Renewable and Sustainable Energy Reviews, 135, 110239. https://doi.org/10.1016/j.rser.2020.110239
- Zhou, J., Zhang, X., & Shen, L. (2015). Urbanization bubble: Four quadrants measurement model. Cities, 46, 8–15. https://doi.org/10.1016/j.cities.2015.04.007
- Zhu, Y., Wang, W. W., & Lin, L. (2021). Return migration and in situ urbanization of migrant sending areas: Insights from a survey of seven provinces in China. Cities, 115, 103242. https://doi.org/10.1016/j.cities.2021.103242