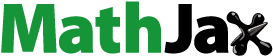
Abstract
A farmer field school programme aims to impart knowledge and promote new technology. The programme is expected to enhance farmers’ performances. However, the empirical evidence remains unclear. A few studies have examined the impact of this programme on technical efficiency in Indonesia. The current study specifically investigates the causal impact of the Farmer Field School of Integrated Crop Management (FFS-ICM) programme on technical efficiency of maize production in Indonesia. We used a stochastic production frontier model and propensity score matching (PSM) to examine the causal impact of FFS-ICM on the technical efficiency of maize production. The results show that the average farm technical efficiency is 68.3%, which suggests that the production can be further improved. The PSM estimation indicates that FFS-ICM increased the technical efficiency by 1.48%, demonstrating the importance of agricultural extension programmes in rural areas in Indonesia. Nevertheless, the relatively small estimated impact raises the issue of cost effectiveness of the programme.
1. Introduction
The agricultural sector plays an essential role in Indonesia’s economy. For example, it accounted for roughly 13.7% of the Gross Domestic Product (World Bank, Citation2021) and generated about 38 million employments in 2020 (Statistics Indonesia, Citation2021a). Agricultural products’ shares in non-oil commodities’ exports reached 2.66% in 2020, growing by almost 15% from the previous year (Statistics Indonesia, Citation2021b). This growth is a key instrument for alleviating poverty, which is mainly concentrated in rural areas (Purwono et al., Citation2021; Solihin et al., Citation2021; Suryahadi et al., Citation2009).
One strategic crop commodity in Indonesia is maize, whose production reached 22.59 million tons in 2019 (Ministry of Agriculture, Citation2020a)—one of the largest productions globally (US Department of Agriculture, Citation2021). Approximately 5.10 million households earn a living from the industry, equivalent to 20% of the total farmers in Indonesia (Ministry of Trade, Citation2017). Aside from being a staple food, maize is also the main material of the farm feed and food industry (Ministry of Agriculture, Citation2020a). Therefore, any disruptions to maize production will have a ripple effect on other foods’ prices, such as chicken eggs and meat (Freddy et al., Citation2018; Magfiroh et al., Citation2018; Olagunju et al., Citation2021).
The current state of maize production needs to be improved to meet domestic demand (Magfiroh et al., Citation2018). For instance, the average annual maize production was 9.04 million tons from 2009 to 2018, and the average domestic demand was 11.14 million tons yearly (Freddy et al., Citation2018). In terms of productivity, Indonesia is behind other maize-producing countries. It was 3.16 metric tons per hectare in 2020, lower than Thailand (3.70 metric tons per hectare), Vietnam (4.80 metric tons per hectare), and China (6.32 metric tons per hectare) (US Department of Agriculture, Citation2021). The low productivity is also compounded by other prevalent agricultural challenges. Rapid urbanisation in Indonesia, particularly in Java Island, often results in agricultural land conversion (Agus et al., Citation2019; Bren d‘Amour et al., Citation2017; Suwandari et al., Citation2020). Meanwhile, expanding cultivation to dryland areas is often constrained by irrigation issues (Wangiyana & Kusnarta, Citation2020). Also, production mainly occurs once a year, only during the dry season, because rice cultivation, a prioritised commodity in Indonesia, occurs in the rainy season. Many farmers (roughly 59%) are smallholder farmers with land ownership of less than 0.5 ha (Statistics Indonesia, Citation2018). They often have a low level of education (Mariyono et al., Citation2021); so, they do not have much capacity to adopt advanced agricultural technology (Mariyono et al., Citation2021; Paltasingh & Goyari, Citation2018).
The government of Indonesia launched a programme called Sekolah Lapang Pengelolaan Tanaman Terpadu (SL-PTT) or Farmer Field School of Integrated Crop Management (FFS-ICM) for maize farmers to improve their capacity. Farmers are trained to manage land, water, crops, plant diseases, and climate with a participatory, experimental, and reflective-learning approach. All activities are conducted in the farm fields. The programme also introduces agricultural technology based on the local needs, potentials, and challenges—an approach called participatory rural appraisal (Larsen & Lilleør, Citation2014; Ministry of Agriculture, Citation2008). The training is expected to impart science-based knowledge to farmers and equip them with practical skills (Mariyono et al., Citation2013), hence enhancing the human capital in the agricultural sector that will increase productivity, efficiency, and prosperity (Davis et al., Citation2012; Mariyono et al., Citation2021; Yamazaki & Resosudarmo, Citation2008).
Nevertheless, evidence shows mixed conclusions about the impact of the training on farmers’ performances (Larsen & Lilleør, Citation2014; Mariyono et al., Citation2021; Paltasingh & Goyari, Citation2018). Prior works by Abdulai and Huffman (Citation2014) in Africa and Luther et al. (Citation2018), Mariyono (Citation2019), and Mariyono et al. (Citation2022) in Indonesia confirmed the positive impact on yields and productivity, but a study by Feder et al. (Citation2004) in Indonesia and Guo et al. (Citation2015) in China found no evidence of the impact of field training on yields, pesticide use, and cultivation knowledge.
In Indonesia, previous works have investigated the impact of FFS-ICM on maize production. For example, Kariyasa (Citation2016) considered the role of infrastructure and governmental support in East Java and West Nusa Tenggara. By comparing the means’ differences, Kariyasa (Citation2016) found that FFS-ICM participants with better infrastructure and governmental support produced more maize by 9.37%. A subsequent study by Kariyasa (Citation2014) analysed the impact of FFS-ICM on productivity and maize farmers’ income, which showed that participants produced 27% more maize and earned around 34% higher income than non-participants. Nevertheless, these studies did not capture the systematic differences between participants and non-participants. These differences could arise from the non-random geographical placement of the programme and the voluntary nature of the programme participation (Godtland et al., Citation2004; Guo et al., Citation2015). For instance, FFS-ICM areas might be selected due to their relative advantages in land fertility or infrastructure. It is also possible that farmers who voluntarily participate in the programme are more educated than the non-participants, which means that they have a competitive advantage even without joining the programme.
Therefore, this recent study aims to examine the impact of FFS-ICM of maize on farm performance. This study has two main contributions to the literature. First, this research applies the propensity score matching (PSM) method to control potential systematic differences between participants and non-participants. The employment of PSM is expected to yield robust and reliable findings. Second, this study focuses on the impact of FFS-ICM on technical efficiency of maize production, a topic that is still limited due to most previous works focused on the impact of FFS-ICM on productivity, pesticide use, and farmers’ welfare.
The following section begins with a literature review of the concept of technical efficiency and the relationship between FFS and technical efficiency. The third section sets out data and methodology. The fourth section presents the result and discussion; the last section concludes the discussion.
2. Literature review and hypothesis development
2.1. Technical efficiency concept
Technical efficiency reflects ‘how well’ a farmer can combine different production inputs into a production process to produce maximum output. An increase in technical efficiency, or a reduction in technical inefficiency, raises productivity because the same set of resources produces more output (FAO, Citation2018). Technical efficiency should not be confused with productivity, which measures how much a given number of resources produces output. Technical efficiency is the gap between frontier technology and a farm’s actual productivity, and this gap can boost growth (Kim & Han, Citation2001). The technical efficiency concept can be illustrated in a diagram introduced by Farrell (Citation1957). shows a unit isoquant SS’, which depicts the various combinations of two inputs that a perfectly efficient farm can use to produce a unit of output. Point P represents the actual combination of inputs. A farm produces the same output as P using only a fraction OQ/OP as much as each input. The line segment OQ/OP indicates farm efficiency. To put it differently, a technically efficient farm produces OP/OQ times as much output from the same inputs. The difference between the inefficient production (observed) and the frontier production (estimated) is a measure of technical efficiency.
Figure 1. Technical efficiency in a two-input space.
Adopted from Farrell (Citation1957).
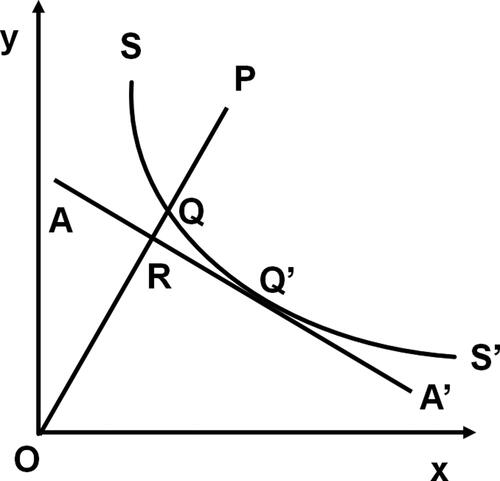
Technical efficiency is improved when existing inputs are combined or used more efficiently. The factors that influence technical inefficiency include socioeconomic profiles and farmers’ management skills, access to extension services, credit constraints, and poor infrastructures such as irrigation systems (Heriqbaldi et al., Citation2014; Huy, Citation2009; Komicha & Öhlmer, Citation2008; Wicaksono, Citation2014).
2.2. Farmer field school and technical efficiency
The main public channel to impart agricultural knowledge to farmers is usually through an extension service (Anderson & Feder, Citation2004; Feder & Slade, Citation1986). World Bank (Citation1994) defines agricultural extension as a programme assisting farmers to become aware of and adopt improved technology from various sources to enhance production efficiency, income, and welfare. In general, the aim of an extension is to transfer knowledge that empowers farmers to make more informed decisions by considering the goals and possibilities; for example, by choosing the right farming system (van den Ban, Citation1999). Evenson (Citation1968) believed that extension accelerates the adoption of new input and improves efficiency by combining the new input with the existing inputs in the production.
An extension comes in various forms depending on the purposes, with seminars being the most common. The programme is usually organised off-farm, such as in government offices and hotels. Recently, more programmes have been held on farms as they attempt to combine theoretical knowledge and technical skills, such as technology demonstrations, on-farm trials, and field trips. The World Bank introduced a visitation training system in the 1970s, which was later improved into a participatory approach referred to as farmer field school (FFS) by the United Nations Food and Agriculture Organization (FAO), aiming not only to train farmers to learn new skills and decision making but also how to communicate them to the wider community. Depending on the programme or module, the FFS lasts for several days, weeks, months, or the entire planting season.
FFS is an innovative, participatory, and interactive learning approach conducted in fields that emphasises problem-solving and discovery-based learning (FAO, Citation2016). FFS serves as a platform for technology transfer (National Research Council, Citation2002) and provides inputs to enhance human capital and welfare (Anderson & Feder, Citation2004). In developing countries, the adoption and commercialisation of emerging technologies have not been optimally achieved due to cultural constraints, ethical concerns, regulatory issues, and lack of understanding of the science and technology being used (Ugochukwu & Phillips, Citation2018). Investment in new technology is low because stakeholders could not see immediate returns or they do not know how to manage technology so that it helps generate high returns (Foster & Rosenzweig, Citation2010). FFS aims to address these constraints. Thus, the hypothesis derived from the theoretical framework above is:
Hypothesis: Farmer Field School (FFS)-ICM significantly increases technical efficiency of maize production.
3. Methodology
3.1. Data
The study employs the most recent dataset from the Indonesian Secondary Food Crops Cultivation Farm Holders Survey collected in 2014 by the Statistics Indonesia (Badan Pusat Statistik or BPS). The survey is a part of the 2013 Indonesia Agricultural Census—conducted every ten years—which presents national data of secondary crop commodities including maize, soybean, peanut, mung bean, cassava, and sweet potato. It provides the general information, cost structure, and socio-economic conditions of each commodity’s households (Statistics Indonesia, Citation2015). Specifically, the current study uses a dataset of 28,178 maize-producing households across ten major maize-producing regions in Indonesia: North Sumatera, South Sumatera, Lampung, West Java, Central Java, East Java, West Nusa Tenggara, North Sulawesi, South Sulawesi, and Gorontalo. illustrates the distribution of research areas.
Moreover, the rainfall data are obtained from Statistics Indonesia (Citation2017). In-depth interview with government officials was also conducted to assess the current situation of the implementation of the FFS-ICM programme as well as complement the apparently outdated survey data.
3.2. Estimation of technical efficiency
In the literature, there are two widely applied approaches to estimate technical efficiency: parametric approaches such as stochastic frontier analysis and non-parametric approaches such as data envelopment analysis (DEA) (Belek & Jean Marie, Citation2021; Ma et al., Citation2018; Martey et al., Citation2019). The stochastic frontier analysis employs econometric modelling to determine the frontier production function. Thus, it requires a prior functional relationship between inputs and output (Assaf & Josiassen, Citation2016). This approach assumes that any deviation from the production frontier could be attributed to inefficiency and random noise such as measurement errors, natural disasters, and agricultural diseases (Afrin et al., Citation2017; Laha, Citation2013; Mango et al., Citation2015). On the other hand, DEA estimates the frontier production function based on the piecewise function of the data. Therefore, it does not impose any functional form of the input-output relationship (Assaf & Josiassen, Citation2016). Nevertheless, the method assumes no stochastic errors, so it considers any output deviation from its production frontier as inefficiency. Therefore, the method is insensitive to the outlier, so it is unsuitable for agricultural research where unpredictable factors may affect production (Ma et al., 2018). Thus, this study uses stochastic frontier analysis to estimate the technical efficiency of maize production. In general, the stochastic frontier is specified as follows:
(1)
(1)
where represents the output variable,
denotes a vector of inputs,
indicates a vector of parameters to be estimated. Meanwhile,
is an error term consisting of two components:
is the random error and
denotes the inefficiency error term. The
is assumed to be independently and identically distributed as N(0,
). Meanwhile,
follows an asymmetric distribution, usually in the half-normal, truncated, exponential, or gamma distribution, as the efficiency score cannot have negative values (Assaf & Josiassen, Citation2016; Martey et al., Citation2019).
Furthermore, EquationEquation (1)(1)
(1) requires a specific functional form
that describes the relationship between inputs and output. Following the work of Anang et al. (Citation2017) and Ma et al. (Citation2018), the current study employed the Cobb-Douglass production function to link the relationship, described as follows:
(2)
(2)
where is maize yield (kg) of farmer ith and
denotes the production inputs. The inputs consist of fertiliser, seeds, labour, farm size, and pesticide use, and rainfall. This study includes four dummy variables representing geographical differences across regions, namely Sumatera for farmers in Sumatera Island, Java, West Nusa Tenggara, and Sulawesi. The baseline of the variable of the dummy region is the dummy Java. A dummy variable of the type of land is also introduced in the production function. Meanwhile,
and
are the parts of the composed error term. The quantity of output and inputs are converted into a natural logarithm, except the dummy variables. Additionally,
and
are unknown intercepts and parameters to be estimated, respectively using the maximum likelihood method. Further description of the inputs and output is shown in .
Table 1. Descriptive statistics.
After obtaining the production frontier, the technical efficiency of farmer i could be obtained by finding the ratio of the actual output and the estimated frontier output against the number of inputs. Specifically, the technical efficiency could be expressed by:
(3)
(3)
where
is the actual output and
is the predicted output obtained from the frontier estimation. A farm is classified as perfectly efficient if the actual output lies on the production frontier and inefficient if the actual output produced is below the production frontier.
3.3. Estimation of impact of FFS on technical efficiency
Analysing the causal impacts of FFS programme participation on technical efficiency could be convoluted by a potential endogeneity bias (Baiyegunhi et al., Citation2019; Wossen et al., Citation2017). Because participation is often voluntary and non-random, participating farmers may be systematically different from non-participating farmers. This may directly affect outcomes (Heckman & Robb, Citation1985). Simply calculating the means’ differences between the treatment group (participants) and the control group (non-participants) may result in biased estimates. Using a standard regression analysis—considering the outcomes as a dependent variable with participation/treatment (dummy) variable as the independent variable—will not solve the potential bias either (Martey et al., Citation2019). Estimating an accurate impact of a specific intervention or programme participation requires a comparison of the outcomes with the conditions where the programme is absent, which is called counterfactual (Khandker et al., Citation2010; Martey et al., Citation2019). However, a counterfactual could not be observed directly. Technically, following the framework of Imbens and Wooldridge (Citation2009), the causal impact of a particular programme participation is measured by the average treatment effect on the treated (ATT), which is defined as:
(4)
(4)
where Y(1) and Y(0) are outcome variables (technical efficiency) when farmers participate and do not participate, respectively. T denotes a programme participation indicator where T = 1 indicates participation and 0 otherwise. Based on EquationEquation (4)
(4)
(4) , E[Y(1)|T = 1] is observable, while E[Y(0)|T = 1] is the counterfactual (unobservable missing data). Simply put, it is not possible for a farmer to participate and not participate in the programme simultaneously (Suwandari et al., Citation2020).
Many approaches are used to mimic a counterfactual, including propensity score matching (PSM). The fundamental idea behind PSM is to match each treated participant with an identical untreated participant and then estimate the mean differences of the outcomes. The PSM approach’s matching process could reduce bias and result in more reliable estimates (Baiyegunhi et al., Citation2019). Specifically, PSM mimics the counterfactual by estimating the propensity score—the probability of participation—of the given pre-treatment variables. The propensity score is specified as follows:
(5)
(5)
where p(
) is a propensity score, Pr is the probability of FFS-ICM participation (T = 1 represents farmers joining the programme and T = 0 otherwise) given the
a vector of observed covariates. F{.} indicates the probability distribution either normal or logistic cumulative. This study employs the logistic cumulative distribution or Logit model to estimate the propensity score or predicted probability of participation.
After obtaining the propensity score of joining the FFS-ICM programme, we used matching algorithms to match each farmer who participated with a farmer who did not participate with similar propensity scores. The current study uses several matching algorithms, including Nearest Neighbours and Kernel-based matching, to assure robust estimates. The requirement of a reliable matching algorithm should not eliminate too many original observations from the final stages of analysis and provide balanced covariates means between the treated and untreated groups statistically (Baiyegunhi et al., Citation2019). To check the matching quality, we employed a statistical t-test to examine the covariates balance between the treatment and control groups are balanced.
Afterward, the causal impact of FFS participation on technical efficiency is estimated by the equation below:
(6)
(6)
where T shows participation status in the FFS-ICM programme by the farmer, and it takes two values: T = 1 if the farmer participates and T = 0 if the farmer does not participate. (1) indicates the outcome indicators of the participating farmers and (0) denotes the outcome indicators of the non-participating farmers. Meanwhile, () is the estimated propensity score used to match each observation.
4. Result and discussion
4.1. Descriptive statistics
The measurement and descriptive statistics of the farmers’ profiles, along with the input and output used in the technical efficiency analysis, are shown in . The data are presented by the FFS-ICM participation and pooled sample. In general, 1,625 farmers, or 5.76% of the total sample, participated in the FFS-ICM programme (treatment group). About 26,553 farmers, or 94.24% of the total sample, did not participate (control group). The treatment group produced more maize than the control group. Specifically, the average production of the treatment group was roughly 2,507 kg, and the control group was 2,291 kg. The treatment group also utilised slightly more inputs than the control group. For instance, the mean fertiliser used by the treatment group was around 255 kg, and the control group 248 kg; the seeds used by the treatment group was 8.92 kg, and the control group 8.89 kg; the labour used by the treatment group was 34.72, and the control group 33.80. The average farm size of the treatment group was 5,549.93 meters square, and the control group was 5,370.66 meters square. About 63% of the farmers in the treatment group used pesticides, while in the control group, 61%. Around 46% of the farmers in the treatment group used irrigated land, while in the control group, 33%.
Regarding socioeconomic variables, the participating farmers were older (average age 50.23 years old) than the non-participating farmers (average age 49.53 years old). Most of the farmers in both groups were male. For education, the participants’ average years of education are higher (6.29 years) than the non-participants (5.55 years). About 88% of the participants joined a farmers’ group, and only 49% of the non-participants joined a farmers’ group. Only 6% of the participants and 2% of the non-participants signed a contract with a third party such as a cooperative or private company. Most of the farmers are landowners. As for the geographic locations, 65 and 54% of the participants and the non-participants, respectively, lived in Java Island.
4.2. The estimation of technical efficiency
shows the maximum likelihood estimation of the Cobb-Douglas stochastic production function parameters. There are three models employed: model (1) used conventional input variables (fertiliser, seeds, labour, land, pesticide, type of land, and rainfall); model (2) employs the same variables as model (1) with additional variable of rainfall squared; and model (3) extends model (2) by including dummy regions.Footnote1
Table 2. Estimation of the parameters for stochastic production function.
All conventional inputs of maize production such as fertilisers, seeds, labours, and farmland show positive signs, in line with the a priori expectation. Moreover, all coefficients of the variables are statistically significant. The findings indicate that fertilisers, seeds, labours, and farmland determine the maize output, which corroborates the study by Mango et al. (Citation2015) in Zimbabwe. In addition, the coefficients of the conventional inputs in the production model could be interpreted as partial elasticity. For example, a 1% increase in fertiliser is associated with a 0.1122% increase in maize production, holding other inputs constant. Likewise, a 1% increase in seeds is associated with a 0.28% increase in maize production, assuming other inputs are fixed. The analogous interpretation could be applied to labour and farmland variables.
Meanwhile, the coefficient of the dummy variable of pesticides is 0.0415 and statistically significant, which means, on average, farmers who used pesticides produced 4.15% more maize than those who did not. Pesticides could control pests and diseases in maize and prevent production loss (Sun et al., Citation2020). The same is true for the land types (irrigation) dummy variable. On average, farmers who grew maize in irrigated land produced 7.10% more maize than those who grew maize in non-irrigated land. Vaidyanathan et al. (Citation1994) argue that crop productivity in irrigated land. The coefficient of rainfall is 0.0027, indicating that 1 mm rainfall is associated with 0.27% increase of maize production, holding other variables constant. Nevertheless, the variable of rainfall square is negative, implying that up to a certain point, high rainfall will reduce the maize production. Location dummy variables such as Sumatera, West Nusa Tenggara, and Sulawesi are statistically significant, indicating pronounced differences in maize output produced by farmers in Sumatera, West Nusa Tenggara, and Sulawesi relative to those in Java. For instance, the coefficient of dummy Sumatera is 0.0603, which means that, on average, farmers in Sumatera produced 6.03% more maize than farmers in Java. The analogous interpretation could be used to other dummies region.
The average technical efficiency of maize production in Indonesia is only 68.3%, meaning that farmers only produced 68.3% of the optimum potential. The use of the existing technology with better management could improve this production rate. This finding is similar to the previous studies by Sodiq and Haryanto (Citation2021) in Gorontalo, Indonesia; and Belete (2020) in Ethiopia. Specifically, Sodiq and Haryanto (Citation2021) estimated that the average technical efficiency of maize farming in the region was 57%, with only 5% of the total farmers operating with maximum technical efficiency. Meanwhile, research by Belete (2020) showed that the efficiency in Ethiopia was 69%. illustrates a comparison of the average technical efficiency by FFS-ICM participation, suggesting that FFS-ICM participants (70.5%) were higher than the non-participants (68.2%).
4.3. Determinants of FFS-ICM participation
The Logistic model of the determinants of FFS-ICM participation is shown in . The significant independent variables are gender, education, farmers’ group membership, contract farming partnership, farm size, and other-land ownership. Meanwhile, the insignificant independent variables are age and rented land. Furthermore, there are some variations of the statistical significance of dummies provinces.Footnote2
Table 3. Logistic regression of determinants of FFS-ICM participation.
The estimated coefficient of gender is positive, suggesting it is more probable for male farmers to join the FFS-ICM programme. This result is consistent with previous studies that claim a gender gap in agricultural extension access (Buehren et al., Citation2019; Jiggins et al.,Citation1997). The coefficient of education is positive, indicating that as the education level increases, the probability of participating in the FFS-ICM programme also increases. Baiyegunhi et al. (Citation2019) mentioned that educated farmers are more likely to access and interpret new information better than non-educated farmers. The coefficient of group membership is positive, which means that farmers who join a farmers’ group are more likely to participate in the FFS-ICM programme than the non-members. Vu et al. (Citation2020) argue that farmers association plays a vital role in enhancing farmers’ access to new information and technology. Similarly, a contract farming indicates a positive coefficient, meaning those with a farming contract are likely to join the FFS-ICM programme.
Dubbert et al. (Citation2023) argued that a farming contract modernises practices, including the access to agricultural extension. This finding aligns with a similar study by Kosim et al. (Citation2021). The coefficient of farm size is also positive, suggesting that farmers with large land areas had more probability of joining the programme. Meanwhile, the estimated coefficient of other-land ownership shows a negative sign, indicating that farmers with free-lease farms tend not to join the FFS-ICM programme compared to those who own the land. Additionally, the variation of statistical significance of dummies province suggests unequal probability of FFS-ICM participation across provinces.
4.4. The impact of FFS-ICM participation on technical efficiency
The Propensity Score Matching (PSM) is utilised to estimate the impact of FFS-ICM on the technical efficiency of maize production. The result of the impact evaluation of FFS-ICM participation on technical efficiency is shown in .
Table 4. Impact of FFS-ICM on technical efficiency.
The estimation shows that all matching algorithms yield similar results. It indicates that the participation of the FFS-ICM programme had a positive and statistically significant impact on technical efficiency. Specifically, the FFS-ICM participation raised the technical efficiency by about 1.48% or 1.9%. This finding is similar to previous work by Mariyono (Citation2008) and Waddington et al. (Citation2014), showing that FFS Integrated Pest Management (FFS-IPM) led to a significant reduction in the use of pesticides among rice and cotton farmers. FFS serves as a channel to influence intermediates outcomes such as knowledge and facilitate speed of adoption of new inputs and improved practices (Evenson, Citation1968). In this instance, farmers learn to lower their subjective economic thresholds, for example through delayed spraying such that pesticides are used more efficiently. Furthermore, the balancing test based on 4-Nearest Neighbour is employed to check the reliability of the matching. shows that the matching process has produced balanced observable characteristics between the treatment and the control groups.
Table 5. After matching quality test.
In general, PSM estimation results corroborate the previous studies documenting the positive impacts of FFS-ICM on farmers’ performance indicators. This result is also in line with a study by Zubair et al. (Citation2021) in Pakistan and Mariyono (Citation2019) in Indonesia. Recent evidence from the experimental method indicates that FFS reduces learning frictions and increases technology adoption in India (Emerick & Dar, Citation2021). Qualitative research also reveals that the participants of FFS-ICM felt that they gained substantial knowledge on more effective farming practices. The FFS-ICM programme changed the participants’ beliefs on certain farming practices that are not science-based (Luther et al., Citation2018). Concurrently, the current study’s finding opposes a study by Admassu (Citation2015), claiming that the FFS programme reduced the technical efficiency of maize production in Ethiopia; and that it might be caused by reduced family labour allocation.
It is worth noting that the estimated impact of the FFS-ICM programme on technical efficiency in this study is relatively small (only about 2%). According to Luther et al. (Citation2018), the small impact could be attributed to the low farmers’ willingness to implement the new farming practices obtained from the FFS programme. They claimed that there are four explanations behind the low willingness. First, the adoption is often hindered by the high cost of new farming practices that require new equipment. Second, the complexity of new farming techniques might discourage farmers. Third, new farming techniques may not be generic enough to implement in any crops. Lastly, new farming practices are an uncharted area for the farmers, so they are concerned about whether the implementation will result in positive outcomes. The in- depth interviews confirmed that the limited impact of FFS-ICM could be attributed to the difficulty of changing farmers’ behaviour or deep-rooted farming habits.
A corollary to the question of the marginal impact of the programme is the concern about its cost effectiveness. At present, the FFS-ICM for maize ceases to be a national priority programme. Its implementation, however, is devolved to local government (provincial or district level government) which is given the flexibility to decide whether to run the programme, subject to local agricultural condition and government targets in any particular year. For example, several villages in Central Sulawesi and West Sumatera still run FFS-ICM for maize in 2020 (READSI, Citation2020; Ministry of Agriculture, 2020b). Even though our finding suggests the impact of FFS on technical efficiency is small, local government may still implement the FFS. Nevertheless, there are some issues that should be addressed so that the FFS programme is still practical. The issue, for instance, is the expensive cost of implementation with limited participant outreach (Anderson & Feder, Citation2004; Dhamankar & Wongtschowski, Citation2014). Thus, local government may design FFS programme that encourages information sharing from FFS participants to non-participants or across social network. It is expected that the dissemination of information across farmers’ social network could speed up knowledge diffusion, and in turn, lowers the average cost of the FFS. Farmers were more likely to be most convinced with communicators who shared a group identity with them, or who face comparable agricultural conditions (BenYishay & Mobarak, Citation2019).
5. Conclusion
This research aims to determine the causal impact of FFS-ICM participation on technical efficiency. Contrary to the previous works that explored the impact of the FFS without controlling for the systematic differences between participants and non-participants, this study employed the PSM method that eliminates the issue of selection bias (the systematic differences) in FFS-ICM programme participation. In doing so, the study provides robust and reliable results. In other words, the current study enriches the body of knowledge on the impact evaluation of FFS by utilising a more suitable method that is distinct from what have been previously utilised in Indonesia.
Econometric results showed that the average technical efficiency of the total sample was mere 68.3%, suggesting the need for improvement. The estimation also revealed that the FFS-ICM participation increased technical efficiency by roughly 1.48 to 1.9%. The finding corroborates previous studies documenting the positive impact of FFS-ICM on-farm performance indicators.
The policy implication of this research is twofold. First, the relatively low rate of technical efficiency of maize production calls for the government and non-government organisations to continue improving farmers’ education, not only on raising production capacity but also on using the inputs efficiently. As seen in some studies, there exists a complementarity between FFS and efficiency where FFS can influence intermediates outcomes such as knowledge and facilitate speed of adoption of new inputs and improved practices. Second, the positive impact of FFS-ICM on technical efficiency implies that the government may continue to enhance farmers’ capacity through agricultural training, including FFS. At present, the running of FFS-ICM is devolved from the national to local government. However, the need to reconsider the FFS-ICM implementation from the cost-benefit standpoint remains imperative. As a response, the local government may design the programme that encourages sharing of information across social networks to speed up its diffusion in order to lower the average cost of the FFS. Lastly, this research had some limitations. First, the secondary dataset used was relatively outdated. Thus, future research may employ more recent dataset to produce precise findings. Second, the analysis focused on one specific commodity only (maize). Future research might explore other agricultural commodities.
Additional information
Funding
Notes
1 The rest of the analysis in the paper uses model (3) as the basis of discussion.
2 We included dummies province in the logistic regression with dummy East Java as the reference group. Provinces that had statistical significances: North Sumatera, South Sumatera, Lampung, Central Java, West Nusa Tenggara, South Sulawesi, and Gorontalo. In contrast, provinces that did not have statistical significance: West Java and North Sulawesi.
References
- Abdulai, A., & Huffman, W. (2014). The adoption and impact of soil and water conservation technology: An endogenous switching regression application. Land Economics, 90(1), 26–43. https://doi.org/10.3368/le.90.1.26
- Admassu, T. H. (2015). The Impact of Farmer Field School Training on Farmers’ Technical Efficiency: Evidence from Smallholder Maize Farmers in Oromia, Ethiopia. European Journal of Training and Development Studies, 2(3), 1–28.
- Afrin, S., Haider, M. Z., & Islam, M. S. (2017). Impact of financial inclusion on technical efficiency of paddy farmers in Bangladesh. Agricultural Finance Review, 77(4), 484–505. https://doi.org/10.1108/AFR-06-2016-0058
- Agus, F., Andrade, J. F., Rattalino Edreira, J. I., Deng, N., Purwantomo, D. K., Agustiani, N., Aristya, V. E., Batubara, S. F., Hosang, E. Y., Krisnadi, L. Y., Makka, A., Cenacchi, N., Wiebe, K., Grassini, P., Herniwati, & Samijan. (2019). Yield gaps in intensive rice-maize cropping sequences in the humid tropics of Indonesia. Field Crops Research, 237, 12–22., https://doi.org/10.1016/j.fcr.2019.04.006
- Anang, B. T., Bäckman, S., & Rezitis, A. (2017). Production technology and technical efficiency: Irrigated and rain-fed rice farms in northern Ghana. Eurasian Economic Review, 7(1), 95–113. https://doi.org/10.1007/s40822-016-0060-y
- Anderson, J. R., & Feder, G. (2004). Agricultural extension: Good intentions and hard realities. The World Bank Research Observer, 19(1), 41–60. https://doi.org/10.1093/wbro/lkh013
- Assaf, A. G., & Josiassen, A. (2016). Frontier analysis: A state-of-the-art review and meta-analysis. Journal of Travel Research, 55(5), 612–627. https://doi.org/10.1177/0047287515569776
- Baiyegunhi, L. J. S., Majokweni, Z. P., & Ferrer, S. R. D. (2019). Impact of outsourced agricultural extension program on smallholder farmers’ net farm income in Msinga, KwaZulu-Natal, South Africa. Technology in Society, 57, 1–7. https://doi.org/10.1016/j.techsoc.2018.11.003
- Belek, A., & Jean Marie, A. N. (2021). The impact of microfinance services on the efficiency of family farms in Sub-Saharan Africa: the case of Cameroon. Agricultural Finance Review, 81(1), 21–38. https://doi.org/10.1108/AFR-02-2019-0021
- BenYishay, A., & Mobarak, A. M. (2019). Social learning and incentives for experimentation and communication. The Review of Economic Studies, 86(3), 976–1009. https://doi.org/10.1093/restud/rdy039
- Bren d‘Amour, C., Reitsma, F., Baiocchi, G., Barthel, S., Güneralp, B., Erb, K.-H., Haberl, H., Creutzig, F., & Seto, K. C. (2017). Future urban land expansion and implications for global croplands. Proceedings of the National Academy of Sciences of the United States of America, 114(34), 8939–8944. https://doi.org/10.1073/pnas.1606036114
- Buehren, N., Goldstein, M., Molina, E., & Vaillant, J. (2019). The impact of strengthening agricultural extension services on women farmers: Evidence from Ethiopia. Agricultural Economics, 50(4), 407–419. https://doi.org/10.1111/agec.12499
- Davis, K., Nkonya, E., Kato, E., Mekonnen, D. A., Odendo, M., Miiro, R., & Nkuba, J. (2012). Impact of farmer field schools on agricultural productivity and poverty in East Africa. World Development, 40(2), 402–413. https://doi.org/10.1016/j.worlddev.2011.05.019
- Dhamankar, M., & Wongtschowski, M. (2014). Farmer field schools (FFS). Note 2. GFRAS Good Practice Notes for Extension and Advisory Services.
- Dubbert, C., Abdulai, A., & Mohammed, S. (2023). Contract farming and the adoption of sustainable farm practices: Empirical evidence from cashew farmers in Ghana. Applied Economic Perspectives and Policy, 45(1), 487–509. https://doi.org/10.1002/aepp.13212
- Emerick, K., & Dar, M. H. (2021). Farmer field days and demonstrator selection for increasing technology adoption. The Review of Economics and Statistics, 103(4), 680–693. https://doi.org/10.1162/rest_a_00917
- Evenson, R. E. (1968). The contribution of agricultural research and extension to agricultural production [Doctoral dissertation]. The University of Chicago.
- Farrell, M. J. (1957). The measurement of productive efficiency. Journal of the Royal Statistical Society: Series A (General), 120(3), 253–281. https://doi.org/10.2307/2343100
- Feder, G., Murgai, R., & Quizon, J. B. (2004). Sending farmers back to school: The impact of farmer field schools in Indonesia. Review of Agricultural Economics, 26(1), 45–62. https://doi.org/10.1111/j.1467-9353.2003.00161.x
- Feder, G., & Slade, R. (1986). The impact of agricultural extension: The training and visit system in India. The World Bank Research Observer, 1(2), 139–161. https://doi.org/10.1093/wbro/1.2.139
- FAO. (2016). Farmer Field School Guidance Document: Planning for quality programmes. Rome: Food and Agriculture Organization of the United Nations. https://www.fao.org/3/i5296e/i5296e.pdf
- Food and Agricultural Organization. (2018). Guidelines for the measurement of productivity and efficiency in agriculture.
- Foster, A. D., & Rosenzweig, M. R. (2010). Microeconomics of technology adoption. Growth Center Discussion Paper No. 984. Yale University Economic.
- Freddy, I. M., Respatiadi, H., & Gupta, G. E. K. (2018). Reforming trade policy to lower maize prices in Indonesia. Center for Indonesian Policy Studies, 1–36. https://doi.org/10.35497/270483
- Godtland, E. M., Sadoulet, E., Janvry, A. D., Murgai, R., & Ortiz, O. (2004). The impact of farmer field schools on knowledge and productivity: A study of potato farmers in the Peruvian Andes. Economic Development and Cultural Change, 53(1), 63–92. https://doi.org/10.1086/423253
- Guo, M., Jia, X., Huang, J., Kumar, K. B., & Burger, N. E. (2015). Farmer field school and farmer knowledge acquisition in rice production: Experimental evaluation in China. Agriculture, Ecosystems & Environment, 209, 100–107. https://doi.org/10.1016/j.agee.2015.02.011
- Heckman, J. J., & Robb, R. Jr, (1985). Alternative methods for evaluating the impact of interventions: An overview. Journal of Econometrics, 30(1-2), 239–267. https://doi.org/10.1016/0304-4076(85)90139-3
- Heriqbaldi, U., Purwono, R., Haryanto, T., & Primanthi, M. R. (2014). An analysis of technical efficiency of rice production in Indonesia. Asian Social Science, 11(3), 91. https://doi.org/10.5539/ass.v11n3p91
- Huy, H. T. (2009). Technical efficiency of rice-producing households in the Mekong Delta of Vietnam. Asian Journal of Agriculture and Development, 6(2), 35–50.https://ajad.searca.org/article?p=157
- Imbens, G. W., & Wooldridge, J. M. (2009). Recent developments in the econometrics of program evaluation. Journal of Economic Literature, 47(1), 5–86. https://doi.org/10.1257/jel.47.1.5
- Jiggins, J., Samanta, R. K., & Olawoye, J. E. (1997). Improving women farmers’ access to extension services. In: B. Swanson, R. P. Bentz, & A. J. Sofranko (Eds.), Improving agricultural extension: A reference manual (Chapter 9). Rome, Italy: Food and Agriculture Organization of the United Nations. https://www.fao.org/3/w5830e/w5830e00.htm#Contents
- Kariyasa, I. K. (2014). Economic impact assessment of integrated crop management farmer field school program on corn production in Indonesia. International Journal of Food and Agricultural Economics (IJFAEC), 2, 13–26.
- Kariyasa, I. K. (2016). Impact of infrastructure and government support on corn production in indonesia: A case on integrated crop management farmer field school. Jurnal Agro Ekonomi, 29(2), 147–168. https://doi.org/10.21082/jae.v29n2.2011.147-168
- Khandker, S. R., Koolwal, G. B., & Samad, H. A. (2010). Handbook on impact evaluation: Quantitative methods and practices. World Bank Publications. https://documents1.worldbank.org/curated/en/650951468335456749/pdf/520990PUB0EPI1101Official0Use0Only1.pdf
- Kim, S., & Han, G. (2001). A decomposition of total factor productivity growth in Korean manufacturing industries: A stochastic frontier approach. Journal of Productivity Analysis, 16(3), 269–281. https://doi.org/10.1023/A:1012566812232
- Komicha, H. H., & Öhlmer, B. (2008). Effect of credit constraint on production efficiency of farm households in southeastern Ethiopia. Ethiopian Journal of Economics, 15(1), 1–33. https://doi.org/10.4314/eje.v15i1.39816
- Kosim, M., Aji, J. M. M., & Hapsari, T. D. (2021). The impact of agricultural extension on productivity of smallholder sugarcane farmers in East Java. IOP Conference Series: Earth and Environmental Science, 892(1), 1–7. https://doi.org/10.1088/1755-1315/892/1/012009
- Laha, A. (2013). Technical efficiency in agricultural production and access to credit in West Bengal, India: A stochastic frontier approach. International Journal of Food and Agricultural Economics (IJFAEC), 1, 53–62.
- Larsen, A. F., & Lilleør, H. B. (2014). Beyond the field: The impact of farmer field schools on food security and poverty alleviation. World Development, 64, 843–859. https://doi.org/10.1016/j.worlddev.2014.07.003
- Luther, G. C., Mariyono, J., Purnagunawan, R. M., Satriatna, B., & Siyaranamual, M. (2018). Impacts of farmer field schools on productivity of vegetable farming in Indonesia. In: Natural resources forum (Vol. 42, No. 2, pp. 71–82). Blackwell. https://doi.org/10.1111/1477-8947.12144
- Ma, W., Renwick, A., Yuan, P., & Ratna, N. (2018). Agricultural cooperative membership and technical efficiency of apple farmers in China: An analysis accounting for selectivity bias. Food Policy, 81, 122–132. https://doi.org/10.1016/j.foodpol.2018.10.009
- Magfiroh, I. S., Zainuddin, A., & Setyawati, I. K. (2018). Maize supply response in Indonesia. Buletin Ilmiah Litbang Perdagangan, 12(1), 47–72. https://doi.org/10.30908/bilp.v12i1.309
- Mango, N., Makate, C., Hanyani-Mlambo, B., Siziba, S., & Lundy, M. (2015). A stochastic frontier analysis of technical efficiency in smallholder maize production in Zimbabwe: The post-fast-track land reform outlook. Cogent Economics & Finance, 3(1), 1117189. https://doi.org/10.1080/23322039.2015.1117189
- Mariyono, J. (2008). Direct and indirect impacts of integrated pest management on pesticide use: A case of rice agriculture in Java, Indonesia. Pest Management Science, 64(10), 1069–1073. https://doi.org/10.1002/ps.1602
- Mariyono, J. (2019). Farmer training to simultaneously increase productivity of soybean and rice in Indonesia. International Journal of Productivity and Performance Management, 68(6), 1120–1140. https://doi.org/10.1108/IJPPM-10-2018-0367
- Mariyono, J., Dewi, H. A., Daroini, P. B., Latifah, E., Hakim, A. L., & Luther, G. C. (2022). Farmer field schools for improving economic sustainability performance of Indonesian vegetable production. International Journal of Productivity and Performance Management, 71(4), 1188–1211. https://doi.org/10.1108/IJPPM-09-2019-0445
- Mariyono, J., Luther, G. C., Bhattarai, M., Ferizal, M., Jaya, R., & Fitriana, N. (2013). Farmer field schools on chili peppers in Aceh, Indonesia: Activities and impacts. Agroecology and Sustainable Food Systems, 37(9), 1063–1077. https://doi.org/10.1080/21683565.2013.819827
- Mariyono, J., Waskito, J., Kuntariningsih, A., Latifah, E., Suswati, E., & Suwandi, Tabrani. (2021). Farmer field school: Non-formal education to enhance livelihoods of Indonesian farmer communities. Community Development, 52(2), 153–168., https://doi.org/10.1080/15575330.2020.1852436
- Martey, E., Wiredu, A. N., Etwire, P. M., & Kuwornu, J. K. (2019). The impact of credit on the technical efficiency of maize-producing households in Northern Ghana. Agricultural Finance Review, 79(3), 304–322. https://doi.org/10.1108/AFR-05-2018-0041
- Ministry of Agriculture. (2008). Panduan Pelaksanaan Sekolah Lapang Pengelolaan Tanaman Terpadu (SLPTT) Jagung [Guidance on the implementation of farmer field school on integrated crop management of maize]. Kementerian Pertanian Republik Indonesia.
- Ministry of Agriculture. (2020a). Rencana Strategis Kementerian Pertanian 2020-2024 [Strategic planning of Ministry of Agriculture 2020-2024]. Kementerian Pertanian Republik Indonesia.
- Ministry of Agriculture. (2020b). Proyek Pengembangan dan Manajemen Irigasi Partisipatif Terpadu. [Integrated Participatory Irrigation Management and Development Project]. http://moaipdmip.org/
- Ministry of Trade. (2017). Potret Jagung Indonesia Menuju Swasembada Tahun 2017 [Snapshot on maize in Indonesia towards self-sufficiency target in 2017]. Kementerian Perdagangan Republik Indonesia.
- National Research Council. (2002). Publicly funded agricultural research and the changing structure of U.S. agriculture. The National Academies Press.
- Olagunju, K. O., Ogunniyi, A. I., Oyetunde-Usman, Z., Omotayo, A. O., & Awotide, B. A. (2021). Does agricultural cooperative membership impact technical efficiency of maize production in Nigeria: An analysis correcting for biases from observed and unobserved attributes. Plos One, 16(1), e0245426. https://doi.org/10.1371/journal.pone.0245426
- Paltasingh, K. R., & Goyari, P. (2018). Impact of farmer education on farm productivity under varying technologies: Case of paddy growers in India. Agricultural and Food Economics, 6(1), 1–19. https://doi.org/10.1186/s40100-018-0101-9
- Purwono, R., Wardana, W. W., Haryanto, T., & Mubin, M. K. (2021). Poverty dynamics in Indonesia: Empirical evidence from three main approaches. World Development Perspectives, 23, 100346. https://doi.org/10.1016/j.wdp.2021.100346
- READSI. (2020). Semangat Sekolah Lapang Kelompok Padi dan Jagung di Banggai Pasca Pembatasan Sosial [The spirit of the Farmer Field School Program of rice and maize in Banggai post social restrictions]. www.readsi.id
- Sodiq, S., & Tri, Haryanto. (2021). Analysis of technical efficiency of paddy and maize farming. Gorontalo Development Review, 4(1), 1–11. https://doi.org/10.32662/golder.v4i1
- Solihin, A., Wardana, W. W., Fiddin, E., & Sukartini, N. M. (2021). Do government policies drive economic growth convergence? Evidence from East Java, Indonesia. Cogent Economics & Finance, 9(1), 1992875. https://doi.org/10.1080/23322039.2021.1992875
- Statistics Indonesia. (2015). Sensus Pertanian 2013, Angka Nasional Hasil Survei Rumah Tangga Usaha Pertanian Palawija, 2014 [Agricultural Census 2013, National Figures of Indonesian Secondary Food Crop Cultivation Farm Holders Survey 2014]. https://www.bps.go.id/publication/2015/01/28/5b24b305ba6c0c38fa5206d7/sensus-pertanian-2013–angka-nasional-hasil-survei-rumah-tangga-usaha-tanaman-palawija–2014.html
- Statistics Indonesia. (2017). Jumlah Curah Hujan dan Jumlah Hari Hujan di Stasiun Pengamatan BMKG 2011-2015 [Total rainfall and number of rainy days at the BMKG Observation Station 2011-2015]. https://www.bps.go.id/statictable/2017/02/08/1959/jumlah-curah-hujan-dan-jumlah-hari-hujan-distasiun-pengamatan-bmkg-2011-2015.html
- Statistics Indonesia. (2018). Hasil Survei Pertanian Antar Sensus (SUTAS) 2018 [The result of inter-census agricultural survey]. Badan Pusat Statistik (BPS).
- Statistics Indonesia. (2021a). Penduduk 15 Tahun Ke Atas yang Bekerja menurut Lapangan Pekerjaan Utama 2011 – 2021 [Manpower by main occupation 2011-2021]. https://www.bps.go.id/statictable/2009/04/16/970/penduduk-15-tahun-ke-atas-yang-bekerja-menurut-lapangan-pekerjaan-utama-1986–-2021.html
- Statistics Indonesia. (2021b). Analisis Komoditas Ekspor 2013-2020 [Analysis of export commodity 2013-2020]. Badan Pusat Statistik.
- Sun, S., Zhang, C., & Hu, R. (2020). Determinants and overuse of pesticides in grain production: A comparison of rice, maize and wheat in China. China Agricultural Economic Review, 12(2), 367–379. https://doi.org/10.1108/CAER-07-2018-0152
- Suryahadi, A., Suryadarma, D., & Sumarto, S. (2009). The effects of location and sectoral components of economic growth on poverty: Evidence from Indonesia. Journal of Development Economics, 89(1), 109–117. https://doi.org/10.1016/j.jdeveco.2008.08.003
- Suwandari, A., Hariyati, Y., Agustina, T., Kusmiati, A., Hapsari, T. D., Khasan, A. F., & Rondhi, M. (2020). The impacts of certified seed plant adoption on the productivity and efficiency of smallholder sugarcane farmers in Indonesia. Sugar Tech, 22(4), 574–582. https://doi.org/10.1007/s12355-020-00821-2
- Ugochukwu, A., & Phillips, P. W. B. (2018). Technology adoption by agricultural producers: A review of the literature. In E. G. Carayannis (Ed.), From Agriscience to Agribusiness: Theories, policies and practices in technology transfer and commercialization (pp. 361–377). Springer International.
- US Department of Agriculture. (2021). World agricultural production. Circular Series WAP 9-21 September 2021.
- Vaidyanathan, A., Krishnakumar, A., Rajagopal, A., & Varatharajan, D. (1994). Impact of irrigation on productivity of land. Journal of Indian School of Political Economy, 6(4), 601–645.
- van den Ban, A. W. (1999). Agricultural development: Opportunities and threats for farmers and implications for extension organizations. The Journal of Agricultural Education and Extension, 6(3), 145–156. https://doi.org/10.1080/13892249985300291
- Vu, H. V., Ho, H., & Le, Q. H. (2020). Impact of farmers’ associations on household income: Evidence from tea farms in Vietnam. Economies, 8(4), 92. https://doi.org/10.3390/economies8040092
- Waddington, H., Snilstveit, B., Hombrados, J., Vojtkova, M., Phillips, D., Davies, P., & White, H. (2014). Farmer field schools for improving farming practices and farmer outcomes: A systematic review. Campbell Systematic Reviews, 10(1), i–335. https://doi.org/10.4073/CSR.2014.6
- Wangiyana, W., & Kusnarta, I. G. M. (2020). Effect of mini-sprinkler irrigation on yield of two hybrid maize varieties under two levels of fertilization on dryland of Nangakara area, Sumbawa, Indonesia. International Journal of Environment, Agriculture and Biotechnology, 5(3), 832–837. https://doi.org/10.22161/ijeab.53.35
- Wicaksono, E. (2014). The impact of agricultural credit on rice productivity. International Journal on Advanced Science, Engineering and Information Technology, 4(5), 322. https://doi.org/10.18517/ijaseit.4.5.427
- World Bank. (1994). Agricultural extension. IEG lessons and practices. No. 6. http://documents.worldbank.org/curated/en/956441468763806805/Agricultural-extension
- World Bank. (2021). Agriculture, forestry, and fishing, value added (% of GDP) – Indonesia. https://data.worldbank.org/indicator/NV.AGR.TOTL.ZS?locations=ID
- Wossen, T., Abdoulaye, T., Alene, A., Haile, M. G., Feleke, S., Olanrewaju, A., & Manyong, V. (2017). Impacts of extension access and cooperative membership on technology adoption and household welfare. Journal of Rural Studies, 54, 223–233. https://doi.org/10.1016/j.jrurstud.2017.06.022
- Yamazaki, S., & Resosudarmo, B. P. (2008). Does sending farmers back to school have an impact? Revisiting the issue. The Developing Economies, 46(2), 135–150. https://doi.org/10.1111/j.1746-1049.2008.00060.x
- Zubair, M., Karim, R., & Nasrullah (2021). Impact of farmer fields schools on technical efficiency of tobacco growers: A case study of district Swabi Pakistan. Business and Economic Journal, 12(1), 1–16.