Abstract
The stabilities of seven agronomic traits were analyzed and the general stabilities of soybean [Glycine max (L.) Merr.] accessions were evaluated based on the additive main effects and multiplicative interactions (AMMI) model using the founder parent Tokachi nagaha and 137 of its derived cultivars as materials. The objective was to provide a theoretical basis for effectively using germplasm in soybean breeding and production. Analysis of variance (ANOVA) showed that genotype, environment, and genotype by environment interactions were significantly different for each trait. The first three interaction principal components axes (IPCA) were highly significant, accounting for 61.28–70.00% of the total variation. The stability differed for the different traits. 50 cultivars with high general stabilities were identified. The general stability of Tokachi nagaha was moderate, as the stability coefficients (Di) of its seven traits were relatively high; this must be considered by breeders using this cultivar as a breeding parent. There were significant positive correlations between the phenotypic values and their own Di values for number of branches per plant, number of pods per plant, number of seeds per plant, and seed weight per plant. This would lead to the expectation that the phenotypic stability would be lower when a cultivar had more branches, pods, seeds, and high per plant yields. Thus, it appears difficult to breed cultivars that simultaneously have high yields and high stability.
Keywords:
Classification:
Genetic stability under different environmental conditions is an important factor when evaluating the industrial value of plant cultivars (Wu, Citation2000). Because of genotype by environment interaction (G×E), the phenotypic values of a crop are different when grown under different environmental conditions. These differences can be significant. The stability and adaptability of a cultivar during growth is determined in part by the G×E interaction, and both stability and adaptability appear to decrease as the effects of these interactions become larger.
Currently, three methods, the regression approach (Eberhart & Russell, Citation1966; Perkins & Jinks, Citation1968; Tai, Citation1971; Yates & Cochran, Citation1938), the partitioning of genotype and environmental components of variability (Shukla, Citation1972), and the additive main effects and multiplicative interaction (AMMI) model (Mandel, Citation1971), are commonly used to analyze crop cultivar stability. Of these methods, the AMMI model is considered to be better at explaining the effects of G×E interactions; therefore, it has been widely used in the evaluation of the stability of yield-related traits (Adie et al., Citation2014; Kahram et al., Citation2013; Samonte et al., Citation2005; Sousa et al., Citation2015; Tang et al., Citation2013; Wang et al., Citation2016) and quality-related traits (Guo et al., Citation2004; Su et al., Citation2010).
In previous reports on soybean, Gurmu et al. (Citation2009) analyzed the stability of three traits, yield, protein content, and oil content, in 20 cultivars using three statistical methods, including the AMMI model. Asfaw et al. (Citation2009) used the AMMI model to screen soybean cultivars with high and stable yields. In China, most studies have focused on yield stability in regional testing (Chen et al., Citation2007; Liu et al., Citation2015; Zheng, Citation2005; Zheng et al., Citation2005). Liu et al. (Citation2011) conducted a stability analysis for four agronomic traits: plant height (PH), number of branches per plant (BN), 100-seed weight (SW), and yield. However, there have been no reports on the relationships among the stability coefficients of agronomic traits and the comprehensive evaluation of soybean cultivar stability.
Tokachi nagaha, introduced from Japan, is an important founder soybean parent in Northeast China. Its characteristics include short internodes, strong stems, numerous flowers and pods, and a strong combining ability; its utility to breeders has resulted in a large number of progeny cultivars with a large planting area (Guo et al., Citation2007). In this study, the stabilities of seven agronomic traits were compared and the general stability of Tokachi nagaha and 137 of its derived cultivars were evaluated using the AMMI model. The results will provide a theoretical basis for the enhancement and improvement of soybean cultivars.
Materials and methods
Materials
In this study, Tokachi nagaha and 137 derived cultivars, 54 from Heilongjiang province, 80 from Jilin province, 2 from Liaoning province, and 1 from Beijing, were used. Detailed information on the 138 accessions is shown in Table S1.
Phenotypic data collection
The trials were performed from 2011 to 2013 at three experimental stations (three locations × 3 years) in Fanjiatun (43°43′7″N, 125°5′21″E), Jinlin City (43°57′19″N, 126°27′49″E), and Tonghua (42°28′5″N, 125°59′44″E) in Jilin province. The field trials were conducted using a randomized blocks design with two replicates. Each genotype was grown in three rows, 3 m in length with .60 m spacing between rows, and the planting density was about 170,000 plants/hm2. The sowing dates at the Fanjiatun station were in 30 April, 2 May, and 7 May, in 2011, 2012, and 2013, respectively; the sowing date at the Jilin City station was 1 May in all three years; the sowing dates at the Tonghua station were 28 April, 1 May, and 5 May, respectively. At maturity, five uniform plants in the middle row of each plot were sampled to collect data on PH, BN, SW, number of nodes on main stem (NN), number of pods per plant (PN), number of seeds per plant (SN), and seed weight per plant (PSW). Those seven traits were measured following the method of Qiu et al. (Citation2006).
Stability analysis
Analysis of variance (ANOVA) was carried out to test the statistical significance of various sources of variation for each of the seven traits, and then the stability of each trait was analyzed based on the G×E interaction using the AMMI model. The AMMI model is as follows:(1)
where yijk is the observed value of accession i in block k of environment j; μ is the grand mean; αi is the deviation of accession i from the grand mean (main effect of genotype); βj is the deviation of environment j from the grand mean (main effect of environment); λr is the eigenvalue of the interaction principal component axis (IPCA) r, and is also the singular value for singular value decomposition (SVD) axis N; γir is the genotype eigenvector value of axis r; δjr is the environmental eigenvector value of axis r; N is the IPCAs numbers of the G×E interaction; ρij is the residual error after extracting N axes; and εijk is the experimental error.
In this study, the stability coefficient (Di) value, indicating the distance from a certain genotype to the base point in the first three IPCA spaces, was used to accurately judge the stability.(2)
where c is the number of significant IPCAs. Obviously, the lower the Di is, the greater the stability of an accession has.
A correlation analysis was conducted for the Di of each trait. The Di values of the traits that correlated significantly with PSW were used to calculate the general score (GS) according to a previously described method (Ren et al., Citation2012; Tang, Citation2006). Based on the GS, the accessions were divided into five groups as revealed in Table .
Table 1. General stability grades.
The statistical analysis was performed using the SAS 9.1 software (SAS Institute).
Results
Phenotypic variation analyses of seven traits
Large phenotypic variations were observed for all seven traits (Table ). The BN, which ranged from .3 to 3.4, with an average of 1.2, showed the highest coefficient of variation (CV) of 46.2%. The SW ranged from 7.4 to 25.4 g with an average of 19.6 g and had the lowest CV of 12.0%. The CVs of the other traits ranged from 12.2 to 20.6%.
Table 2. Descriptive statistics of the phenotypic values and agronomic characteristics of 138 accessions and Tokachi nagaha.
The BN of Tokachi nagaha was 3.4, which was larger than those of the other cultivars. The PN, SN, and PSW were 87.2, 155.2, and 28.2 g, respectively, which were greater than the mean value of all of the accessions. The SW of Tokachi nagaha was 18.2 g, which was rather low compared with the mean value of all of the accessions. The PH was moderate at 65.0 cm and the NN was comparatively low at 13.9. Thus, Tokachi nagaha had higher BN, PN, SN, and PSW values, but lower SW and PH values than the averages for all of the cultivars in this study.
AMMI model-based analysis
For each trait, ANOVA for the pooled data from nine environments (3 years, three locations) showed that there were significant differences in the genotype, environment, and G×E interactions (Table ), which revealed that there were remarkable differences among the various traits of the cultivars in these environments. These results also showed that it is necessary to evaluate the stability of each cultivar, as G×E interactions significantly affected traits.
Table 3. Analysis of variance for the AMMI model of seven traits.
Evaluation of trait stability
As noted, there were significant differences for seven traits using the AMMI model (Table ). The number of IPCAs with significance levels of .01 for PH, NN, BN, PN, SN, SW, and PSW were five, six, five, five, five, six, and five, respectively. The first three IPCAs in each trait could account for 61.28–70.00% of the effects of the G×E (Table , only lists the first three IPCAs). For example, the proportion of the effects for the first three IPCAs of PH are 34.61, 22.68, and 12.71%, respectively, with a cumulative effect rate that reached 70.0%. The Di value, the distance from a certain genotype to the base point in first three ICPA spaces, was used to evaluate the stability of the cultivars.
Table 4. Statistical parameters of the principal components of the seven traits.
Histograms of the Di values of the seven traits for the 138 cultivars are shown in Figure . PH showed the lowest CV of 37.68%, while BN had the highest CV of 65.27%. The Di values of the other traits ranged from 42.41 to 54.96% (Table ). In general, the stabilities of NN, BN, and SW were comparatively lower, with Di means of .63, .41, and .67, respectively, indicating that the influence of environment was small in these traits. However, PH, PN, SN, and PSW were sensitive to the influence of environment, having Di means of 1.82, 1.89, 2.93, and 1.30, respectively. The stability of Tokachi nagaha was rather low, as represented by the Di values of six of the seven traits (excluding SW) that were all higher than their respective mean values in the 138 accessions (Table ).
Figure 1. Histograms of the Di values of seven traits for the 138 cultivars.
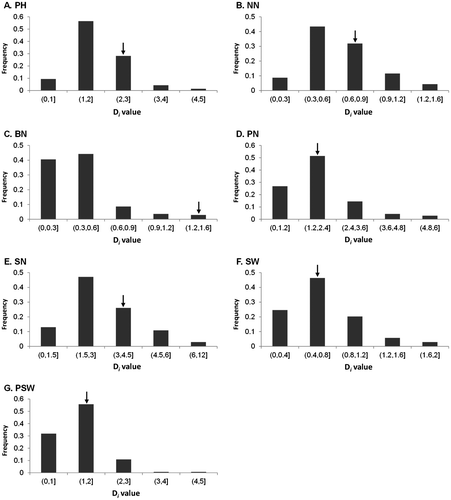
Table 5. Stability parameter Di of seven traits and Tokachi nagaha in the AMMI model.
Correlation analysis for genotypic value and Di value
A correlation analysis showed that the phenotypic mean values of BN, PN, SN, and PSW were extremely significantly positively correlated with their own Di values (Table ), indicating that the stabilities decreased with increases in BN, PN, SN, and PSW. In addition, the phenotypic value of PH was significantly positively correlated with Di value, which indicated that the PH became increasingly unstable as PH increased. Nevertheless, the correlation coefficients (r) between the phenotypic values of NN and SW with their own Di values were .0985 and −.0295, respectively; but were not significant, indicating that NN and SW appear to be fairly stable.
Table 6. Correlation analysis between phenotypic values and Di values.
The correlation coefficients among Di values of all of the seven traits were calculated (Table ). The Di of PSW was extremely significantly positively correlated with that of PN and SN, and the r between PSW and SW was −.0094, but not significant. In addition, significant positive correlations were observed between PN and SN, and PH and NN. The Di values of PN, SN, and PSW were all significantly positively correlated with the Di value of BN. The r values for the correlation between the Di values of PN and SW, and SN and SW were .0814 and .1161, respectively, but were not significant.
Comprehensive evaluation of the stability of yield-related traits
As shown above, the Di of PSW was significantly correlated with that of PN and SN; therefore, the stability of PSW appears to be greatly affected by the stabilities of PN and SN. The Di values of PSW, PN, and SN were selected to evaluate the general stability of the 138 accessions.
Principal component analysis (PCA) was performed for the Di values of PN, SN, and PSW. The number of principal components from this model was selected using the cumulative contributions of the principal components over 85% as the threshold. Two principal components, Z1 and Z2, were selected, and accounted for 79.48 and 13.87% of the variation (Table ).
Table 7. Eigenvalues of the correlation matrix of the principal component analysis.
The general stability scores across the 138 accessions showed ranges of −2.026–4.369; the stability grades, and the PSW are presented in Table . Two cultivars, Kenjiandou 1 (Heilongjiang province) and Jiyu 83 (Jinlin province) (Tables and ), were classed into Grade 1, with general stability scores of −2.026 and −1.887, respectively. In total, 48 cultivars, 19 of which came from Heilongjiang province, 28 from Jilin province, and 1 from Liaoning province, had Grade 2 stability levels. Jiunong 22 and Heihe 19, which have historically been very popular and widely grown in Northeast China, were included in Grade 2. There were 54 cultivars in Grade 3, including the founder parent Tokachi nagaha, and their general stability performances were moderate. Nine cultivars, including four from Jilin province, four from Heilongjiang province, and one from Beijing, had Grade 5 stability levels. These included Jinlinxiaoli 6 and Jinlinxiaoli 7, which are derived from wild soybean and are mainly used for sprout and natto production. There were some cultivars that had both relatively higher general stability levels and relatively higher PSWs, including Sinong 2, Jiyu 71, Fu 97-16, Jinong 15, Jikedou 1, Jiyu 201, Changnong 8, Jiunong 22, Tongnong 13, Heihe 19, and Suinong19, these cultivars also had high SW.
Table 8. The stability grades and the PSW for the 138 accessions.
Table 9. The number and origin of cultivars in different general stability grades.
Of the 138 cultivars, 104 cultivars (accounting for 75.36% of the total), including 63 from Jilin province (45.65%) and 39 (28.26%) from Heilongjiang province (Table ), showed Grades 1−3 stability levels, indicating, unsurprisingly, that the cultivars bred in Jilin province appear to be more suitable for growth there.
Discussion
The stabilities of crop cultivars under various environments have always been a concern to breeders. The AMMI model, in essence a combination of ANOVA and PCA, not only explains the characteristics and significance of the G×E interactions, but also evaluates the stability of cultivars using trait parameters. Thus, it has been considered an attractive method for the evaluation of cultivar stability (Yang et al., Citation2006). Soybean, a short-day crop, is sensitive to photoperiod and temperature, and a cultivar is usually adapted to grow in a narrow ecological (and latitude) environment. In this study, phenotypic measurements were performed on plants grown in Jilin province. Among the 104 cultivars (showing Grades 1−3 stability levels), 63 (accounting for 60.58%) were collected from Jilin province, indicating that most of the cultivars with higher stability were from Jilin province. This result confirm the suitability of using the AMMI model to analyze stability.
The AMMI model is often used to evaluate the stability of a single trait; however, the stability of each trait is known to vary differentially among cultivars (Guo et al., Citation2004; Liu et al., Citation2011, 2013; Zhang et al., Citation2004, 2014). Therefore, it is necessary to evaluate stability comprehensively. Stable yield is an essential characteristic, second only to high yield, for an elite cultivar. Soybean yield is determined by the number of plants per unit area, SN and SW. In this study, the number of plants per unit area was constant; therefore, the soybean yield was dependent only on the PSW. As shown in the correlation analysis, the stability of PSW was mainly affected by the stabilities of PN and SN, rather than the stability of SW. Therefore, it is reasonable to evaluate the general stability of a given cultivar using the Di values of the PSW, PN, and SN.
In the correlation analysis between the phenotypic values and their own Di values (Table ), extremely significant positive correlations were observed for the BN, PN, SN, and PSW. Thus, the stabilities of yield-related traits will decrease as the phenotypic values of those traits increase, meaning that cultivars with higher yields usually have poorer stabilities. It is relatively easy to obtain cultivars with high yields, but it is difficult to breed cultivars with high and stable yields. In this study, we identified not only cultivars from Jilin province (e.g. Jiyu 71 and Jiunong 22), but also cultivars from outside Jilin province (e.g. Sinong 2 and Heihe 19), that have relatively higher general stability levels and relatively high PSWs. These cultivars could be used either as parents in breeding efforts or as good production cultivars.
Liu et al. (Citation2011) reported only on SW; its Di was significantly positively correlated when correlation analyses were performed between the phenotypic values of PH, BN, SW, and yield with their own Di values using 60 landraces form the soybean mini core collection of the Huang-Huai-Hai region. In this study, our results were different from those of Liu et al. (Citation2011) when using 138 cultivars mainly from Northeast China as materials. This may be because of the different types of germplasms have different stability models. In the present study, SW was more stable than PN and SN. This may because the broad-sense heritability of SW (47.66%) was higher than that of PN (19.00%) and SN (17.04%); these findings are similar to those of Hao et al. (Citation2012).
In general, soybean cultivars with long-growth periods are expected to have relatively higher yields than short-growth cultivars (Chen et al., Citation2004). Our results in the present study were consistent with these expectations. The growth period of the 138 cultivars ranged from 97.6 d to 135.1 d, and averaged 119.9 d. The PSWs of the 138 cultivars were significantly positively correlated with the duration of their growth periods (r = .48, p < .01). The correlation coefficient (r) between the growth period and Di values of PSW was .10 (not significant). This indicated that the growth periods of cultivars did not appear to affect the stability of PSW. Thus, the growth period need not be taken into consideration by breeders seeking to generate soybean cultivars with stable yields.
Germplasm introduced from outside China has played important roles in the improvement of soybean cultivars within China. Tokachi nagaha soybean is an ancestral soybean parent used in soybean breeding in Northeastern China (Guo et al., Citation2007). By 2005, about 1300 cultivars had been developed in China, and 287 of them had Tokachi nagaha in their pedigrees. Qin et al. (Citation2008) reported that the genetic diversities of some elite cultivars, including Suinong 14 and Hefeng 25, were remarkably increased likely because they included Tokachi nagaha and Amsoy (from the United States) as parents. Guo et al. (Citation2007) suggested that Tokachi nagaha could be used with multiple crossing techniques to pyramid favorite traits among different parents. Although Tokachi nagaha had higher BN, PN, SN, and PSW values, its general stability was relatively low because of the relatively high Di values; this should be able to be overcome by breeders via hybridization with local cultivars having higher stability levels. The successful utilization of Tokachi nagaha in soybean breeding in China illustrates an effective approach for creating elite intermediate materials by crossing introduced germplasm with local elite cultivars.
Disclosure statement
No potential conflict of interest was reported by the authors.
Funding
This research was supported by the Development of Novel Elite Soybean Cultivars and Lines with High Oil Content [Z161100000916005-06]; the Crop Germplasm Resources Protection [2014NWB030, 2015NWB030-05]; the Platform of National Crop Germplasm Resources of China [2014-004], [2015-004]; the National Key Technology R&D Program [2011BAD35B06-2-9]; the Agricultural Science and Technology Innovation Program of CAAS.
Supplemental data
Supplemental data for this article can be accessed at https://doi.org/10.1080/1343943X.2017.1358095
TPPS_1358095_Supplementary_material.docx
Download MS Word (31.9 KB)Acknowledgments
We thank YH Xiao, PhD, from Rice Research Institute, Hunan Agricultural University, for help in AMMI model analysis.
References
- Adie, M. M., Krisnawati, A., & Gatut-Wahyu, A. S. (2014). Assessment of genotype × environment interactions for black soybean yield using Ammi and GGE biplot. International Journal of Agriculture Innovations and Research, 2, 673–678.
- Asfaw, A., Alemayehu, F., Gurum, F., & Atnal, M. (2009). AMMI and SREG GGE biplot analysis for matching varieties onto soybean production environments in Ethiopia. Scientific Research Essay, 4, 1322–1330.
- Chen, X. Z., Xie, H., & Li, X. (2004). Studies on correlation ship of development stages and agronomic traits of summer sowing soybean. Molecular Plant Breeding, 2, 247–252.
- Chen, Z., Hu, R., & Lin, G. (2007). AMMI model analysis on regional test for new vegetable soybean varieties. Soybean Bulletin, 1, 32–33.
- Eberhart, S. A., & Russell, W. A. (1966). Stability parameters for comparing varieties. Crop Science, 6, 36–40.10.2135/cropsci1966.0011183X000600010011x
- Guo, J. J., Chang, R. Z., Zhang, J. X., Zhang, J. S., Guan, R. X., & Qiu, L. J. (2007). Contribution of Japanese soybean germplasm Tokachi nagaha to Chinese soybean cultivars. Soybean Science, 6, 807–812, 819.
- Guo, T. C., Ma, D. Y., Zhu, Y. J., Wang, C. Y., Xia, G. J., & Luo, Y. (2004). Genotype, environment and their interactive effects on main quality traits of winter-sown wheat variety. Scientia Agricultura Sinica, 37, 948–953.
- Gurmu, F., Mohammed, H., & Alemaw, G. (2009). Genotype × environment interactions and stability of soybean for grain yield and nutrition quality. African Crop Science Journal, 17, 87–99.
- Hao, D. R., Cheng, H., Yin, Z. T., Cui, S. Y., Zhang, D., Wang, H., & Yu, D. Y. (2012). Identification of single nucleotide polymorphisms and haplotypes associated with yield and yield components in soybean (Glycine max) landraces across multiple environments. Theoretical and Applied Genetics, 124, 447–458.10.1007/s00122-011-1719-0
- Kahram, A., Khodarahmi, M., Mohammadi, A. R., Bihamta, M. R., Ahmadi, G. H., Ghandi, A., … Abdi, H. (2013). Genotype environment interaction analysis for grain yield of durum wheat new genotypes in the moderate region of Iran using AMMI model. World Journal of Agricutural Sciences, 9, 298–304.
- Liu, B., Wei, L., Fan, Y., Yang, H., Duan, X., Xiao, J., … Xiao, L. (2015). Yield analysis of national regional trial of soybean in Huang-Huai-Hai area based on AMMI model. Chinese Agricultural Science Bulletin, 31, 69–74.
- Liu, L. H., Hu, Y. F., Chen, Q., Li, H. Y., Qian, Y. D., Lv, Y. D., … Zuo, Y. H. (2013). Interaction of genotypes with environments for three quality traits of rice in cold region by AMMI model. Acta Agronomica Sinica, 39, 1849–1855.10.3724/SP.J.1006.2013.01849
- Liu, Z. X., Yang, C. Y., Xu, R., Lu, W. G., Qiao, Y., Zhang, L. F., … Qiu, L. J. (2011). Analysis of adaptability of soybean mini core collections in Huang-Huai region. Acta Agronomica Sinica, 37, 443–451.
- Mandel, J. (1971). A new analysis of variance model for non-additive data. Technometrics, 13, 1–18.10.1080/00401706.1971.10488751
- Perkins, J. M., & Jinks, J. L. (1968). Environmental and genotype-environmental components of variability. III. Multiple lines and crosses. Heredity, 23, 339–356.
- Qin, J., Li, Y. H., Liu, Z. X., Guan, R. X., Zhang, M. C., Chang, R. Z., … Qiu, L. J. (2008). Genetic relationship among parents of elite soybean (Glycine max) cultivars Suinong 14 pedigree revealed by SSR markers. Scientia Agricultura Sinica, 41, 3999–4007.
- Qiu, L. J., Chang, R. Z., Liu, Z. X., Guan, R. X., & Li, Y. H. (2006). Descriptors and data standard for soybean (Glycine spp.). Beijing: China Agriculture Press.
- Ren, X. B., Gao, J. Y., Dai, X. Y., Yao, T. M., Liu, C., & Zhou, W. J. (2012). Comprehensive evaluation of agronomic characters in early maturity cabbage. Northern Horticulture, 17, 44–46.
- Samonte, S. O. P. B., Wilson, L. T., McClung, A. M., & Medley, J. C. (2005). Targeting cultivars onto rice growing environments using AMMI and SREG GGE biplot analysis. Crop Science, 45, 2414–2424.10.2135/cropsci2004.0627
- Shukla, G. K. (1972). Some statistical aspects of partitioning genotype-environmental components of variability. Heredity, 29, 237–245.10.1038/hdy.1972.87
- Sousa, L. B., Hamawaki, O. T., Nogueira, A. P. O., Batista, R. O., Oliveira, V. M., & Hamawaki, R. L. (2015). Evaluation of soybean lines and environmental stratification using the AMMI, GGE biplot, and factor analysis methods. Genetics and Molecular Research, 14, 12660–12674.10.4238/2015.October.19.10
- Su, Z. X., Zhao, G. Z., Liao, X. H., Shi, R., Jiang, C., Zhu, Z. H., & Zou, Q. (2010). Stability of eating quality traits of Japonica soft rice from different locations at different altitudes in Yunnan province, China. Chinese Journal of Rice Science, 24, 320–324.
- Tai, G. C. C. (1971). Genotypic stability analysis and its application to potato regional trials. Crop Science, 11, 184–190.10.2135/cropsci1971.0011183X001100020006x
- Tang, H., Liu, H., Yu, H. Y., Zhang, Z. F., Xiao, Y. H., Yang, Y. S., & Chen, L. Y. (2013). Stability and importance of morphological characteristics in example varieties based on DUS test. Acta Agronomica Sinica, 39, 632–641.10.3724/SP.J.1006.2013.00632
- Tang, Y. Q. (2006). SAS statistical analysis tutorial (pp. 256–260). Beijing: China Agriculture Press.
- Wang, X., Liu, Z., Yang, C., Xu, R., Lu, W., Zhang, L., … Qiu, L. (2016). Stability of growth periods traits for soybean cultivars across multiple locations. Journal of Integrative Agriculture, 15, 963–972.10.1016/S2095-3119(15)61152-2
- Wu, W. R. (2000). An improvement on the method of variety stability analysis based on the AMMI model. Hereditas, 22, 31–32.
- Yang, T., Li, J. N., Tang, Z. L., & Shen, L. (2006). Comparison of three evaluation methods for variety stability. Guizhou Agricultural Sciences, 34, 28–31.
- Yates, F., & Cochran, W. G. (1938). The analysis of groups of experiments. The Journal of Agricultural Science, 28, 556–580.10.1017/S0021859600050978
- Zhang, F. Y., Li, H., Wang, C. R., Zhou, D. G., Zhou, X. Y., Situ, Z. M., … Zhou, S. C. (2014). Stability analysis of rice yield related traits of varieties derived from Huanghuazhan and its parents. Chinese Journal of Rice Science, 28, 487–495.
- Zhang, J. Y., Xiao, Y. H., Wan, X. Y., Liu, S. J., Wang, C. M., Chen, L. M., … Wan, J. M. (2004). Stability analysis for appearance qualities of rice cultivar. Acta Agronomica Sinica, 30, 548–554.
- Zheng, W. (2005). Analysis on regional test of soybean variety by AMMI model. Heilongjiang Agricultural Sciences, 2, 15–17.
- Zheng, W., Liu, Y. H., Wang, Y. X., Yu, Y., Wang, S. B., & Liu, G. M. (2005). The application of biplot in yield analysis of soybean regional test. Heilongjiang Agricultural Sciences, 6, 5–7.