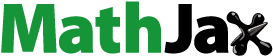
ABSTRACT
Systematic management of safety factors can improve project performance in terms of schedule and cost effectiveness as well as lead to a significant reduction of safety hazards. The purpose of this study is to determine critical safety factors focusing on the construction environments of a developing country, the Mongolia. Through extensive literature review, the authors selected prospective safety factors considering specific construction project environments of Mongolia. Rankings and significance of each safety factor are determined by the Relative Importance Index (RII) and t-test is applied to examine statistical differences among factor category groups. RII analysis indicates the failure of wearing personal protective equipment is the most critical factor among 58 factors. Meanwhile, adopting factor analysis methodology, 13 critical safety factor groups are identified and the most influential one is the regulatory group. In addition, comparative analysis between results of this study and those of previous studies is conducted. Findings of this study may contribute to the improvement of construction safety performance especially for those countries with similar construction environments as Mongolia. Analysis results and implications obtained suggest project managers or safety officials should devote more careful attention to critical safety factors and factor groups in safety planning and during project implementation stages.
1. Introduction
Contributions of the construction industry are significant in terms of level of economic output and employment in many countries, especially in developing countries. Accordingly, construction activity is one of the yardsticks measuring the level of economic development of a country (Al Haadir and Panuwatwanich Citation2011). However, construction sector is one of the most dangerous industries globally, in which fatalities and injuries bring significant losses to societies (Hamalainen, Takala, and Saarela Citation2007; Fang and Wu Citation2013; Fang, Wu, and Wu Citation2015). Sawacha, Naoum, and Fong (Citation1999) assert the risk of fatality in the construction industry is five times more likely than in the manufacturing industry, while the probability of a major injury is two and a half times higher. In similar vein, Jannadi and Bu-Khamsin (Citation2002) note the construction industry is one of the most hazardous industries compared to other industries. According to the Bureau of Labor Statistics (BLS) of U.S., the construction industry is a high-risk sector in terms of the high frequency of work-related fatalities and serious injuries. Fatality rates in the construction industry remain highest among all other industry sectors in most regions, including Australia, Europe, and North America (McCabe et al. Citation2017).
Accidents always entail cost and scheduling issues because work must be suspended for investigating accidents or due to penalties from government authorities. Work suspension causes interruption of work progress and scheduling compression requiring additional resources input. The above implies total construction cost is increased or contractor’s profit margins can be significantly reduced depending on the level of accidents. Hill (Citation2003) measures the impact of construction injuries on worker’s compensation, liability costs, businesses, society, and workers. According to Hill’s findings, worker’s compensation costs negatively impact on project profitability and the impact is significant. The National Safety Council (Liang Citation1997) of the U.S. concluded the cost of accidents is not visible, causing project budgets to increase. Estimated total cost of non-fatal injuries in the U.S. ranges from 131.2 USD to 145 USD billion per year (Hill Citation2003). Along with the cost issue, workers’ productivity is affected by accidents; frequently, crew teams must be reorganized to supplement worker(s).
Poor safety performance of the construction industry has raised diverse safety-related concerns at the international level (Haslam et al. Citation2005). Despite efforts to improve construction safety performance during past decades, accidents, fatalities and injuries are daily occurrences at construction sites (Hinze, Hallowell, and Baud Citation2013a; Guo, Yiu, and Gonzalez Citation2015). Safety-related practices must be measured during the construction phase to trigger positive responses before injuries and accidents (Hallowell et al. Citation2013). In addition, safety-related practices and safety programs should always be defined by considering every investigation of accident, health hazard identification, and exposure of site or off-site safety factors (Hill Citation2003).
Recently, in Mongolia, the investment level of infrastructure development has significantly increased. The construction industry has been expanding rapidly in terms of the portion of GDP (Gross Domestic Product) contributions and level of employment. Meanwhile, the safety performance of construction sector is at a poor level compared to advanced countries as shown in . Effective countermeasures have not been prepared to mitigate increasing construction accidents and injuries. In this study, the number of fatal construction accidents in Mongolia is first compared to another industry, such as, mining industry that has the largest investment level. Although the rate of fatal occupational accidents of construction industry is slightly decreased recently, it remains the highest. Rates of fatal occupational accidents in Mongolia must be calculated because values are not available from data source (Council of Labor Affairs, Taiwan Citation2017), and values are compared with other advanced countries such as, Singapore, Japan, the U.S., Canada, France, and the U.K. According to the Council of Labor Affairs, fatality occupational rates, expressed as the number of fatal work injuries per 1,000 workers, are calculated as follows:
Table 1. Rates of fatal occupational accidents (per 1,000 workers)
in which N is the number of worker fatalities, and W is the annual average number of employed workers.
Studies determining critical safety factors are insufficient in terms of sophisticated planning of construction projects (Odeh and Battaine Citation2002; Kim, Soibelman, and Groblera Citation2008). To provide safe construction environments, continuous monitoring of unsafe conditions and actions is imperative (Seo, Lee, and Kim Citation2015). Under safe working environments or jobsite conditions, potential hazards, injuries and accidents may be decreased remarkably.
This study describes and discusses findings obtained from analysis based on a structured questionnaire survey and interviews focused on critical safety factors. Objectives of this study are: (1) to identify critical safety factors affecting on the safety performance of construction projects; (2) to rank safety factors (3) to propose safety strategies for the preparation of remedial and preventive programs; and (4) to compare findings of this study and previous studies. This study is organized into three parts. The first part begins with a discussion on the concept of safety hazards and safety factors. The discussion section sheds light on the questions “What are the safety factors?” “What are they for?” and “How have they been studied?” The second part proposes an optimum methodology to determine critical safety factors. The third part presents detailed analysis results, findings, and implications.
2. Literature review (Theoretical framework)
A number of studies on safety have been conducted to identify causes of accidents, analyze rate of injuries, unwanted situations, fatalities, and delays caused by safety incidents. Although researchers and practitioners have exerted considerable efforts, guidelines for preparing effective safety planning or programming that may reduce accidents dramatically are limited. To prevent accidents, injuries, and fatalities, and to achieve superior safety performance, critical factors must be explored extensively. Studies that have examined or modeled safety performance include identifying critical safety factors or leading safety indicators, determining effective safety programs, and analyzing safety programs by adopting a variety of methods. For example, modeling and determining the framework of safety (Ng, Cheng, and Skitmore Citation2005; Teo, Ling., and Chong Citation2005; Choudhry, Fang, and Mohamed Citation2007; Awolusi and Marks Citation2017; Guo, Yiu, and Gonzalez Citation2015); analyzing safety practices and safety performance (Hinze, Thurman, and Wehle Citation2013b; Demirkesen and Arditi Citation2015; Choudhry and Zahoor Citation2016; McCabe et al. Citation2017); defining safety leading indicators (Guo and Yiu Citation2016); identifying critical safety factors for advantageous safety program (Aksorn and Hadikusumo Citation2008; Al Haadir and Panuwatwanich Citation2011; Bavafa, Mahdiya, and Marsono Citation2018); determining most influential safety factors for improvement of safety performance (Haslam et al. Citation2005; Shapira and Lyachin Citation2009; Shapira and Simcha Citation2009; Doloi et al. Citation2012; Ismail, Doostdar, and Harun Citation2012; Chen et al. Citation2013; Goh and Sa`Adon Citation2015); and identifying accident precursors or indicators (Pereira et al. Citation2018; Alruqi and Hallowell Citation2019).
The study of Sawacha, Naoum, and Fong (Citation1999) is the first literature that applied factor analysis methodology and proposed five key issues associated with the safety of construction sites: (1) management on talk; (2) provision of safety booklets; (3) provisions of safety equipment; (4) providing a safe working environment; and (5) appointing a trained safety representative on site. Teo, Ling., and Chong (Citation2005) suggest four different factors such as policy, process, personnel, and incentive (3P+I) adopting factor analysis methodology that may help in managing safety programs at construction sites. Based on questionnaire survey analysis, Aksorn and Hadikusumo (Citation2008) proposed the most influential safety factor; management support. However, those studies adopting factor analysis for identification of primary safety factors are not satisfactory (Fang et al. Citation2004; Teo, Ling., and Chong Citation2005; Aksorn and Hadikusumo Citation2008; Chen et al. Citation2013; Shen et al. Citation2015). The study of Fang et al. (Citation2004) focused on the site safety management, not considering overall safety program management perspectives. Teo, Ling., and Chong (Citation2005) also determined safety factors at construction site. Shen et al. (Citation2015) have focused on the limited number of safety factors. The study of Aksorn and Hadikusumo (Citation2008) used insufficient number of questionnaire surveys while factor analysis requires at least 150 samples (Hair et al. Citation2007). On the other hand, this study considered comprehensive safety factors and satisfy the requirements of factor analysis from a sample size perspective.
The authors of this study compare the results of this study with the findings of previous studies. Depending on the study, different safety classification schemes are applied. Numerous safety factors are classified into several groups according to the factor’s characteristics or features. Typical examples are summarized in .
Table 2. Classification schemes of safety factors
Most studies failed to consider diverse safety factors affecting safety performance during project planning and construction periods. Specifically, following limitations are identified: (1) survey responses may be biased because of respondents are not professional engineers, safety officials or project managers; (2) considered limited safety factors not capable of describing whole project safety performances or including inappropriate factors; and (3) lacked theoretical background in the selection of each safety factor.
3. Research methodology
3. 1. Research process
The first phase of the research process is extensive literature review. In the second phase, safety factors are screened and selected safety factors are used in multivariate analysis. A questionnaire survey form is designed for obtaining practitioners’ perception on the significance of safety factors and distributed to respondents. In the next phase, collected data are used for one sample t-test, RII, and factor analysis. From this implementation, factor rankings, population significance, and critical safety factors are obtained. In the final phase, analysis results are discussed along with a comparison between findings of this study and previous studies. illustrates the research flow according to the phase of study.
3. 2. Screening of safety factors and data collection
Built upon previous studies (Sawacha, Naoum, and Fong Citation1999; Abdelhamid and Everett Citation2000; Hill Citation2003; Fang et al. Citation2004; Tam, Zeng, and Deng Citation2004; Teo, Ling., and Chong Citation2005; Shapira and Lyachin Citation2009; Guo and Yiu Citation2016; Wang, Zou, and Li Citation2016; and others), this study screened numerous safety factors to select appropriate factors and to classify these factors into appropriate numbers of groups reflecting safety practices and project environments in the Mongolian construction industry. The authors consulted with the safety professionals of Mongolia to extract appropriate factors. Specifically, the professionals are asked to select suitable factors that best fits with the Mongolian construction environments.
Construction project environment of Mongolia can be defined as unsafe and it is attributable to the following three sources: (1) inclement weather; (2) perception on safety management; and (3) deficient regulatory systems. Firstly, Mongolian construction society has been exposed to inclement climatic weather condition. Pressure on the project schedule and cost is an everyday life under adversary weather condition. It implies that work should be executed under unfavorable weather condition and thus the likelihood of accidents can be dramatically increased. Frequently, it is reported that fatal accidents were caused by strong wind, slippery floor or other unfavorable weather conditions. Second, both workers and managers tend to perceive the importance of safety low. Low level of perceptions on the safety lead to poor safety management plan and fail to implement proper safety activities. On the other hand, safety authority does not strongly enforce safety regulations, codes, and laws. Construction firms also tend to violate safety norms due to tight schedule and cost overrun. Third, a sound regulatory system, in terms of code or law, is not established in the Mongolian construction society. The above sources are compounded and tend to increase the possibility of injuries and fatal accidents in the Mongolian construction society.
Candidate factors which are obtained from previous studies are provided to the professionals for the selection of critical safety factors. After the several rounds of communication and consulting, critical safety factors are determined. Eighteen factors are selected from Tam, Zeng, and Deng (Citation2004), 16 are from Hill (Citation2003), 9 are from Abdelhamid and Everett (Citation2000), 8 are from Sawacha, Naoum, and Fong (Citation1999), 2 are from Wang, Zou, and Li (Citation2016) and 1 is from Guo and Yiu (Citation2016), respectively. Accordingly, a total of 58 safety factors are selected for analysis (see ).
Table 4. Summary of mean and relative importance index ranking of safety factors
Questionnaire survey design is built upon numerous previous studies (e.g. Choudhry, Fang, and Mohamed Citation2007; Terwel and Jansen Citation2015; and others). The survey form is organized two parts; 1) demographic questions, 2) rating impact of safety factors on safety performance. Respondents are asked to assess the impact level of each safety factor on construction safety performance using a five-point Likert scale ranging from 1 (no impact) to 5 (high impact). The designed questionnaire survey form is then sent to construction companies and each company distributes survey forms to engineers, safety engineers, and project managers. Project managers have highest authority in a project and are, in general, responsible for organizing or implementing effective safety programs providing safe environments at sites. Meanwhile, engineers are responsible for inspecting hazards in buildings and machines, monitoring job site environments, and enforcing building codes and standards to prevent accidents at sites.
A total of 655 survey forms are distributed and the total number of collected responses is 219, and thus response rate is 33.4%. Of 219 responses, 111 (50.7%) are from engineers, 50 (22.8%) are from safety engineers, 39 (17.8%) are from project managers, and 19 (8.7%) are from professors. In terms of experience in the construction industry, 48.4% have less than or equal to 5 years of experience, 33.8% have 6 to 10 years, 6.4% have 11 to 15 years, 5.9% have 16 to 20 years, and 5.5% have equal or more than 20 years.
3. 3. Analysis methods
In this study, multivariate techniques, t-test, Relative Importance Index (RII), and factor analysis are used for data analysis. First, the t-test is conducted to check if population means are explained based on the sample’s ratings (positive t-value and p > 0.05) (Teo, Ling., and Chong Citation2005; Aksorn and Hadikusumo Citation2008; Lai, Liu, and Ling Citation2011; Martin and Lewis Citation2014). Subsequently, RII is used to highlight the relative significance of safety factors as perceived by respondents (Tam, Zeng, and Deng Citation2004; Ng, Cheng, and Skitmore Citation2005; Teo and Ling Citation2006; Doloi et al. Citation2012). Last, factor analysis is applied to extract relatively small numbers of critical factors that may be used to represent relationships among sets comprising many interrelated variables (Norusis Citation1993).
For proper analysis in this type of study, comprehensive and accurate data are indispensable. To conduct factor analysis, for example, the following guidelines are proposed to determine the quality of sample size: 50 is very poor, 100 is poor, 200 is fair, 300 is good and 1,000 is excellent (Tabachnick and Fidell Citation2007). The authors made best efforts to obtain reliable data by communicating with as many survey respondents as possible. To classify main safety category groups, an extraction of Unweighted Least Square (ULS) and rotation of Varimax with Kaiser Normalization are used. The ULS is the algorithm that minimizes residuals between input correlation matrix and reproduced correlation matrix. The ULS solutions are derived through an iterative principal axes computational method (Harman Citation1976; Cureton and D’Agostino Citation1983; Briggs and MacCallum Citation2003). Through an iterative process of adding “displacements” to each element of original factor loading matrix, a new factor matrix may be developed (Harman Citation1976). After different rotation techniques are conducted, the Varimax rotation is turned out to be the most suitable technique for the data of this study. There are two ways to rotate factors, oblique and orthogonal. Orthogonal rotation method (e.g. Varimax, Equamax, Quartimax, etc.) constrains factors independent of each other, while oblique rotation method (e.g. Promax, Oblimin, Quartimin, etc.) allows correlation among factors (Choi, Chan, and Chan Citation2011). Results of orthogonal rotation are more preferable and interpretable in this type of study.
The number of factors needed for retention may be decided based on Kaiser’s criterion. This criterion suggests retention of factors above eigenvalue of 1 (Kaiser Citation1960), and the Scree test may be used to determine the number of factors needed for retention. For testing appropriateness of data, two types of tests are conducted: the Kaiser-Meyer-Olkin (KMO) test determines sampling adequacy, and Bartlett’s sphericity test is used to test a hypothesis that correlation matrix is an identity matrix, indicating a relationship does not exist among variables. KMO values range from 0 to 1, and minimum value of 0.5 is specified as an acceptable threshold to proceed with factor analysis (Hair et al. Citation2007). Bartlett’s test should be significant (p <.05) to be appropriate (Tabachnick and Fidell Citation2007). To obtain reliable results, a large sample size is required in factor analysis, namely, minimum number of samples should be at least 100 (Rourke and Hatcher Citation2013). In this study, the sample size exceeds 200 and is therefore evaluated as fair according to the criterion proposed by Tabachnick and Fidell (Citation2007).
4. Analysis results and discussion
4. 1. Perception of safety factors
The questionnaire survey analysis results indicate perception estimates on the significance of safety factor groups and rankings are slightly different among respondent groups. Mean values of safety engineers and project managers are ranked first and followed by professors and engineers group. Significant group differences are observed between some groups as shown in . However, no significant differences are observed between professors and other groups.
Table 3. Test of group difference
To determine the relative significance of safety factors, scores are transformed into important indices based on the following formula (Tam et al. Citation2000; Doloi et al. Citation2012):
in which ω is weighting of each factor obtained from respondents, ranging from 1 to 5 and A is the highest weight (i.e. 5 is highest in this study) and A is the total number of samples. EquationEquation (2)(2)
(2) enables normalizing each factor’s significance ranging from 0 to 1 and it is the RII. The factor with the highest RII or rank 1 has a maximum impact on safety performance and vice versa.
RII of each safety factor and ranking is shown in . Failure of wearing personal protective equipment has the most critical impact on safety performance with an RII value of 0.89. This factor is a main concern in developing countries to prevent hazardous events and maintain safe construction sites. In Mongolia, personal protective equipment is insufficient. Usage of defective or improper ladders (0.88) and defective equipment and machinery (0.87) is evaluated as second and third critical factors, respectively. Ladders and machinery have been used repeatedly without proper maintenance. Such circumstances may result in large losses at construction sites if regulations and obligations regarding inspection are not strictly enforced. Last, warm-up physical exercise for workers prior to starting work, worker’s negative life events, and worker’s age are not critically significant.
4. 1. 1. Interpretation of perception estimate of category groups
This study classifies four-factor category groups considering the characteristics of each factor and examines statistical differences among category groups applying t-test. shows category group of regulatory has highest mean and higher impact on safety performance than other types of groups. The category group of regulatory consists of 11 sub-factors and has a mean value of 0.81 followed by category group of personal. The category group of personal has a significant influence on safety performance.
Table 5. Perception estimate according to factor groups
In addition, the significance of group t-test results and difference between category group of regulatory and environmental are significant at 0.005 level while those of regulatory and management group are significant at 0.05 level. Meanwhile, the difference between other groups shows no difference statistically. This finding suggests regulatory factors have a crucial impact on safety performance. Accordingly, sub-factors in regulatory category group should be considered carefully at the safety planning phase and implementation of safety programs at construction sites.
4. 1. 2. Comparison of the results of current study and those of previous studies
The authors examine the difference between findings of this study and previous studies. After extensive literature review, four literatures (Sawacha, Naoum, and Fong Citation1999; Fang et al. Citation2004; Aksorn and Hadikusumo Citation2008; Ismail, Doostdar, and Harun Citation2012) are chosen for comparison. Above four literatures are extensive in research scope perspective and systematic in terms of analysis methodology to determine critical safety factors compared to other literatures. In addition, factor classification of those studies is appropriate to conduct a comparative analysis with this study. First, Sawacha, Naoum, and Fong (Citation1999) found that a safety factor group named organizational policy and its sub-factors the most dominant group. Second, Fang et al. (Citation2004) examined a total of 11 factors, and concluded five factors are critically significant. Fang et al. validate that foreman related factor is significant, whose factor loadings on this factor are comparatively high among all factors. The foreman-related factor group includes management and inspection-related sub-factors revealing higher correlation with onsite safety management performance. Factors related to workers, crews, managers and safety training are critically significant according to factor analysis. Third, Ismail, Doostdar, and Harun (Citation2012) found that personal factor is the most influential on successful safety program performance among five factor groups. Ismail et al. reveal awareness about the proper use of equipment or tools can reduce risks substantially. Finally, Aksorn and Hadikusumo (Citation2008) grouped 16 success factors influencing on safety program performance into four category groups, and assert that the most influential factor group is worker involvement, representing personal attitude and motivation.
Results of four literatures reveal management and personal-related factors are most influential on safety performance (). Previous studies shown in analyzed different construction societies: 1) Sawacha, Naoum, and Fong (Citation1999) analyzed the U.K.; 2) Fang et al. (Citation2004) analyzed China; 3) Aksorn and Hadikusumo (Citation2008) analyzed Thailand, and 4) Ismail, Doostdar, and Harun (Citation2012) analyzed Malaysia. Sawacha, Naoum, and Fong (Citation1999) assert that organizational policy regarding safety performance in the U.K. is critically significant in reducing accidents. Sawacha, Naoum, and Fong (Citation1999) argue implementation of positive management actions could reduce or prevent construction accidents by 70%. Meanwhile, other previous studies in suggest personal-related factors are vital for the reduction of construction accidents, number of injuries and fatalities. Individual awareness on safety, communication, attitude, habits, and behavior may be the main reasons for construction accidents in those countries. In the past, technical supports, workforces, proper management actions, use of protective devices, economic issues, and safety programs are critical rather than safety-related regulations and enforcement.
Table 6. Comparison between current and previous studies
Findings of previous studies imply that fostering positive worker’s attitude and proper functional management is indispensable for effective safety planning as well as implementing safety actions at sites.
Most of the advanced countries such as Singapore, Japan, the U.S., and Canada have systematic safety regulations and implement safety regulation programs effectively. Accordingly, as shown in , these countries have been maintaining low rates of occupational accidents.
Meanwhile, in developing countries such as Mongolia, new construction regulations are replacing old regulations. Project managers and safety engineers have started to discuss safety matters and are struggling to prevent accidents and reduce injuries. Main reasons behind the high rate of injuries and fatalities in Mongolia are attributable to deficiency of proper regulations and implementation as well as specific construction project environments.
Conversely, as shown in and , there is some consistency between this study and previous studies because the personal-related factor group of this study is significant and ranked second.
In sum, depending on the social system and working environment, critical safety factors may vary across countries.
4. 1. 3. Significance of respondents’ experience
A t-test is implemented to assess respondents’ perception depending on experience level. All samples split into high experience (more than 10 years of experience) and low experience (below or equal to 10 years of experience) groups. As shown in , there is a significant difference between the two groups. Of the total 58 sub-factors, 36 sub-factors are significantly different in their perception. In detail, 26 sub-factors are significant at 0.05 level meanwhile 10 sub-factors are significant at 0.005 level. The numbers of sub-factors significant in the groups of management, personal, environmental and regulatory are 15, 10, 6 and 5, respectively.
Table 7. Perception difference according to experience level
4. 2. Factor analysis results
The SPSS 23.0 is used for factor analysis using 58 safety factors to determine critical factors. Initially, the appropriateness of the factor model is evaluated prior to implementing analysis. Various tests are required to examine the appropriateness of factor analysis for factor extraction and rotation. First, testing of sampling adequacy is assessed by the Kaiser-Meyer-Olkin (KMO) and is evaluated as 0.901 indicating a “good” degree of common variance and is well above the acceptable threshold of 0.50. Next, Bartlett’s test for sphericity is implemented to test null hypothesis that the original correlation matrix is an identity matrix ensuring no relationship among factors (Tabachnick and Fidell Citation2007). Test statistic of Bartlett’s sphericity is large (Chi-Square = 7548.15) and associated significance level is small (p-value = 0.000), implying the population correlation matrix is not an identity matrix and absence of correlations between factors.
The anti-image correlation matrix for individual factors is more than 0.81, except for two variables (worker’s age; worker’s working experience), according to the acceptable limit (i.e. 0.5) proposed by Field (Citation2009). If the above requirement is not met, distinct and reliable factors cannot be produced. To satisfy the multicollinearity matter, determinant score should be above the rule of thumb of 0.00001 indicating the absence of multicollinearity. Test results reveal the determinant score of 0.0002691, greater than the acceptable limit indicating multicollinearity is not an issue.
Unweighted Least Square (ULS) generated 13 factors with eigenvalues greater than 1.0 (Kaiser’s criteria), the generally accepted cut-off criterion for determination and extraction of critical factors (Hatcher Citation1994). The 13 factors account for 59.2% of total explained variance satisfying sample adequacy criterion of greater than 50% (Hair et al. Citation1995). Following Thurstone’s five criteria, the loading value of 0.4, sufficient to meet the requirement, is obtained. Most of the communalities of all variables are between 0.4 and 0.7. If communalities are 0.8 or greater, they are “high”, but it is rare in real-world data. Communalities are low to moderate if they are between.40 and.70 (Costello and Osborne Citation2005). Results of ULS provide variance of each factor, and the number of factors retained is computed by the Kaiser criterion (eigenvalue >1). Thirteen factors have eigenvalue greater than 1.0. Extracted factors with high loadings are shown in .
Table 8. Critical safety factors
Results shown in indicate the first 13 factors satisfy the Kaiser criterion (eigenvalue >1) with ULS extraction, and account for 59.2% of total variance. In addition, the result of scree test confirms that eigenvalue criterion is satisfied. Costello and Osborne (Citation2005) assert the scree test is reliable if the sample size is at least 200.
Table 9. Extraction sums of squared loadings
4. 2. 1. Reliability
A statistical reliability test is commonly conducted to assess stability and consistency in survey questions according to Cronbach’s alpha value. Cronbach’s alpha is a widely used criterion that assesses how well a set of items measures a unidimensional latent construct (Jugdev, Mathur, and Fung Citation2007). In this study, Cronbach’s alpha examines the correctness (accuracy) of extracted 13 factors. A reliability-coefficient value of 0.7 or higher is acceptable in social science studies (Hair et al. Citation2007; Jugdev, Mathur, and Fung Citation2007). Cronbach’s alpha values for 10 factors are greater than 0.70 and those for 3 factors (factors 9, 11, and 13) are slightly lower than 0.7.
4. 2. 2. Interpretation of safety factor groups
The ULS method is used to identify critical safety factor groups. ULS analysis with Varimax rotation is conducted for 58 individual factors and produced 13 factor groups (). Factor groups are appropriately labeled reflecting characteristics of sub-factors. Eigenvalues of factor groups after rotation are displayed in . Factor analysis results support findings regarding perception estimates shown in .
Factor 1 (regulatory) includes 10 sub-factors with regulatory characteristics (). This factor group reveals highest variance explanation (i.e. 30.4%) of total variance () indicating construction safety performance is significantly influenced by regulatory-related safety factors. Accordingly, strict enforcement of regulatory provisions may prevent or minimize such accidents as falling from higher locations and being struck by heavy equipment.
A total of eight sub-factors are included in the second factor group (improper operation) (). The second factor group accounts for 4.42% of total variance and is a bipolar factor ().
The third factor group (frequency of inspection) includes four sub-factors and accounts for 3.98% of total variance. Basically, inspections are indispensable for ensuring compliance with health and safety regulations. Inspection timing and frequency are critical and remedial actions must be taken promptly to prevent accidents. This factor group is useful in preventing falls, ensuring proper use of ladders and stepladders, avoiding obstructions, organizing site conditions, and operating plants, vehicles and equipment properly.
Construction managers and officials should pay close attention on those 13 factor groups if an effective safety planning is to be prepared. Those 13 factors explain 59.2% of total variance.
5. Conclusions
This study begins with a comprehensive literature review to identify and classify safety factors suitable for the Mongolian construction society. Collected data from the questionnaire survey and interviews are analyzed using factor analysis, t-test, and Relative Importance Index (RII). Exploratory factor analysis is conducted to extract latent variables (critical safety factors). This study determined the ranking of 58 factors affecting safety performance in Mongolia.
Among 58 safety factors, failure of wearing personal protective equipment is perceived as the most critical factor followed by usage of defective or improper ladders, defective equipment and machinery, and so forth.
Analysis results reveal the regulatory factor group is the most influential on safety performance. Meanwhile, organizational policy and personal-related factors have a significant impact on safety performance in previous studies. According to the analysis of respondents’ experience level, significant perception differences were observed between high and low experience groups.
Factors explored through factor analysis are classified into 13 primary groups: (1) regulatory; (2) improper operation; (3) frequency of inspection; (4) capital; (5) behavior; (6) hazard; (7) safety device; (8) environmental; (9) personal; (10) examination; (11) health; (12) pre-orientation; and (13) others. Among factor groups, the regulatory is the most significant (variance explanation of 30.4%) requiring immediate attention in developing training and safety programs. Extracted 13 safety factor groups are considered as critical because they may be the main reasons for accidents, injuries and fatalities. Considering RII analysis and factor analysis results, this study partially supports the results of previous studies. It is shown that the personal-related safety factor is critical regardless of advanced or developing countries. However, regulatory-related factors are more crucial for developing countries compared to advanced countries. Therefore, for effective safety management, different strategies or plans should be applied according to construction environments and levels of safety systems.
Through this study, the authors highlight the benefits of determining critical safety factors. This study identified the main factors affecting construction safety performance. The findings of current study can be utilized in preparing safety planning and management in Mongolian construction society. Safety planners, safety managers and project managers are prospective groups to utilize the results of current study. Also, the results of this study may benefit other countries with similar construction characteristics and social systems as Mongolia. From an academic perspective, the results of current study may contribute to the body of knowledge of safety management research field for under developing and developing countries.
Conversely, this study has limitations that may be considered in prospective studies. First, quantitative analysis using real project data has not been conducted. Second, the results of this study may not be generalized because only Mongolian construction society is considered.
Disclosure statement
No potential conflict of interest was reported by the authors.
Additional information
Funding
Notes on contributors
Rentsendorj Usukhbayar
Rentsendorj Usukhbayar obtained his MS degree from the Dongguk University, Seoul, South Korea. His research interests include project management, construction technologies, safety management, and productivity measurement. He is currently working for a Mongolian project management firm.
Jongsoo Choi
Jongsoo Choi obtained his Ph.D. degree in the University of Wisconsin-Madison, US. His research topics include project performance evaluation, strategy analysis of construction firms, project management, and project development.
References
- Abdelhamid, T. S., and J. G. Everett. 2000. “Identifying Root Causes of Construction Accidents.” Journal of Construction Engineering and Management 126 (1): 52–60. doi:10.1061/(ASCE)0733-9364(2000)126:1(52).
- Aksorn, T., and B. H. W. Hadikusumo. 2008. “Critical Success Factors Influencing Safety Program Performance in Thai Construction Projects.” Safety Science 46 (4): 709–727. doi:10.1016/j.ssci.2007.06.006.
- Al Haadir, S., and K. Panuwatwanich. 2011. “Critical Success Factors for Safety Program Implementation among Construction Companies in Saudi Arabia.” Procedia Engineering 14 (4): 148–155. doi:10.1016/j.proeng.2011.07.017.
- Alruqi, W. M., and M. R. Hallowell. 2019. “Critical Success Factors for Construction Safety: Review and Meta-analysis of Safety Leading Indicators.” Journal of Construction Engineering and Management 145 (3): 04019005, 1–11. doi:10.1061/(ASCE)CO.1943-7862.0001626.
- Awolusi, I. G., and E. D. Marks. 2017. “Safety Activity Analysis Framework to Evaluate Safety Performance in Construction.” Journal of Construction Engineering and Management 143 (3): 05016022, 1–12. doi:10.1061/(ASCE)CO.1943-7862.
- Bavafa, A., A. Mahdiya, and A. K. Marsono. 2018. “Identifying and Assessing the Critical Factors for Effective Implementation of Safety Programs in Construction Projects.” Safety Science 106: 47–56. doi:10.1016/j.ssci.2018.02.025.
- Briggs, N. E., and R. C. MacCallum. 2003. “Recovery of Weak Common Factors by Maximum Likelihood and Ordinary Least Squares Estimation.” Multivariate Behavioral Research 38 (1): 25–56. doi:10.1207/S15327906MBR3801_2.
- Chen, W. T., S. S. Lu, S. S. Liu, and M. S. Wang. 2013. “Measuring the Perception of Safety among Taiwan Construction Managers.” Journal of Civil Engineering and Management 19 (1): 37–48. doi:10.3846/13923730.2012.734852.
- Choi, T. N. Y., D. W. M. Chan, and A. P. C. Chan. 2011. “Perceived Benefits of Applying Pay for Safety Scheme (PFSS) in Construction – A Factor Analysis Approach.” Safety Science 49 (6): 813–823. doi:10.1016/j.ssci.2010.10.004.
- Choudhry, R. M., D. Fang, and S. Mohamed. 2007. “Developing a Model of Construction Safety Culture.” Journal of Management in Engineering 23 (4): 207–212. doi:10.1061/(ASCE)0742-597X(2007)23:4(207).
- Choudhry, R. M., and H. Zahoor. 2016. “Strengths and Weaknesses of Safety Practices to Improve Safety Performance in Construction Projects in Pakistan.” Journal of Professional Issues in Engineering Education and Practice 142 (4): 04016011, 1–10. doi:10.1061/(ASCE)EI.1943-5541.0000292.
- Costello, A. B., and J. W. Osborne. 2005. “Best Practices in Exploratory Factor Analysis: Four Recommendations for Getting the Most from Your Analysis.” Practical Assessment, Research and Evaluation 10 (7): 1–9.
- Council of Labor Affairs, Taiwan. 2017. “Ministry of Labor Republic of China, Taiwan.” Accessed 20 March 2019. https://english.mol.gov.tw/6387/
- Cureton, E. E., and R. B. D’Agostino. 1983. Factor Analysis: An Applied Approach. Hillsdale, NJ: Lawrence Erlbaum Associates.
- Demirkesen, S., and D. Arditi. 2015. “Construction Safety Personnel’s Perceptions of Safety Training Practices.” International Journal of Project Management 33 (5): 1160–1169. doi:10.1016/j.ijproman.2015.01.007.
- Doloi, H., A. Sawhney, K. C. Iyer, and S. Rentala. 2012. “Analysing Factors Affecting Delays in Indian Construction Projects.” International Journal of Project Management 30 (4): 479–489. doi:10.1016/j.ijproman.2011.10.004.
- Fang, D., C. Wu, and H. Wu. 2015. “Impact of the Supervisor on Worker Safety Behavior in Construction Projects.” Journal of Management in Engineering 31 (6): 04015001, 1–12. doi:10.1061/(ASCE)ME.1943-5479.0000355.
- Fang, D., and H. Wu. 2013. “Development of a Safety Culture Interaction (SCI) Model for Construction Projects.” Safety Science 57 (7): 138–149. doi:10.1016/j.ssci.2013.02.003.
- Fang, D. P., F. Xie, X. Y. Huang, and H. Li. 2004. “Factor Analysis-based Studies on Construction Workplace Safety Management in China.” International Journal of Project Management 22 (1): 43–49. doi:10.1016/S0263-7863(02)00115-1.
- Field, A. 2009. Discovering Statistics Using SPSS: Introducing Statistical Method (3rd ed.). Thousand Oaks, CA: Sage Publications.
- Goh, Y. M., and B. N. Sa`Adon. 2015. “Cognitive Factors Influencing Safety Behavior at Height: A Multi Method Exploratory Study.” Journal of Construction Engineering and Management 141 (6): 04015002, 1–8. doi:10.1061/(ASCE)CO.1943-7862.0000972.
- Guo, B. H. W., and G. T. W. Yiu. 2016. “Developing Leading Indicators to Monitor the Safety Conditions of Construction Projects.” Journal of Management in Engineering 32 (1): 04015016, 1–14. doi:10.1061/(ASCE)ME.1943-5479.0000376.
- Guo, B. H. W., G. T. W. Yiu, and V. A. Gonzalez. 2015. “Predicting Safety Behavior in the Construction Industry: Development and Test of an Integrative Model.” Safety Science 84 (4): 1–11. doi:10.1016/j.ssci.2015.11.020.
- Hair, J. F., B. Black., B. Babin., R. E. Anderson, and R. L. Tatham. 2007. Multivariate Data Analysis. 6th ed. New Delhi, India: Pearson.
- Hair, J. F., R. E. Anderson., R. E. Tatham, and W. C. Black. 1995. Multivariate Data Analysis. 4th ed. New Jersey: Prentice-Hall.
- Hallowell, M. R., J. W. Hinze., K. C. Baud, and A. Wehle. 2013. “Proactive Construction Safety Control: Measuring, Monitoring, and Responding to Safety Leading Indicators.” Journal of Construction Engineering and Management 139 (10): 04013010, 1–8. doi:10.1061/(ASCE)CO.1943-7862.0000730.
- Hamalainen, P. I., J. Takala, and K. L. Saarela. 2007. “Global Estimates of Occupational Accidents.” Safety Science 44 (16): 137–156. doi:10.1016/j.ssci.2005.08.017.
- Harman, H. H. 1976. Modern Factor Analysis. 3rd ed. Chicago: University of Chicago Press.
- Haslam, R. A., S. A. Hide., A. G. F. Gibb., D. E. Gyi., T. C. Pavitt., S. Atkinson, and A. R. Duff. 2005. “Contributing Factors in Construction Accidents.” Applied Ergonomics 36 (4): 401–415. doi:10.1016/j.apergo.2004.12.002.
- Hatcher, L. 1994. A Step-by-Step Approach to Using the SAS System for Factor Analysis and Structural Equation Modeling. Cary, NC: SAS Institute.
- Hill, D. C. 2003. Construction Safety Management and Engineering. Des Plaines, IL: American Society of Safety Engineers.
- Hinze, J., M. Hallowell, and K. Baud. 2013a. “Construction-safety Best Practices and Relationships to Safety Performance.” Journal of Construction Engineering and Management 139 (10): 04013006, 1–8. doi:10.1061/(ASCE)CO.1943-7862.
- Hinze, J., S. Thurman, and A. Wehle. 2013b. “Leading Indicators of Construction Safety Performance.” Safety Science 51 (1): 23–28. doi:10.1016/j.ssci.2012.05.016.
- Ismail, Z., S. Doostdar, and Z. Harun. 2012. “Factors Influencing the Implementation of a Safety Management System for Construction Sites.” Safety Science 50 (3): 418–423. doi:10.1016/j.ssci.2011.10.001.
- Jannadi, O. A., and M. S. Bu-Khamsin. 2002. “Safety Factors Considered by Industrial Contractors in Saudi Arabia.” Building and Environment 37 (5): 539–547. doi:10.1016/S0360-1323(01)00056-7.
- Jugdev, K., G. Mathur, and T. S. Fung. 2007. “Project Management Assets and Their Relationship with the Project Management Capability of the Firm.” International Journal of Project Management 25 (6): 560–568. doi:10.1016/j.ijproman.2007.01.009.
- Kaiser, H. F. 1960. “The Application of Electronic Computers to Factor Analysis.” Educational and Psychological Measurement 20 (1): 141–151. doi:10.1177/001316446002000116.
- Kim, H., L. Soibelman, and F. Groblera. 2008. “Factor Selection for Delay Analysis Using Knowledge Discovery in Databases.” Automation in Construction 17 (5): 550–560. doi:10.1016/j.autcon.2007.10.001.
- Lai, D. N. C., M. Liu, and F. Y. Y. Ling. 2011. “A Comparative Study on Adopting Human Resource Practices for Safety Management on Construction Projects in the United States and Singapore.” International Journal of Project Management 29 (8): 1018–1032. doi:10.1016/j.ijproman.2010.11.004.
- Liang, P. M. 1997. Supervisor`s Safety Manual. 9th ed. United States of America: National Safety Council.
- Martin, H., and T. M. Lewis. 2014. “Pinpointing Safety Leadership Factors for Safe Construction Sites in Trinidad and Tobago.” Journal of Construction Engineering and Management 140 (2): 04013046, 1–10. doi:10.1061/(ASCE)CO.1943-7862.
- McCabe, B. Y., E. Alderman., Y. Chen, and D. E. A. Hyatt. 2017. “Safety Performance in the Construction Industry: Quasi-longitudinal Study.” Journal of Construction Engineering and Management 143 (4): 04016113, 1–13. doi:10.1061/(ASCE)CO.1943-7862.
- Ng, S. T., K. P. Cheng, and M. Skitmore. 2005. “A Framework for Evaluating the Safety Performance of Construction.” Building and Environment 40 (10): 1347–1355. doi:10.1016/j.buildenv.2004.11.025.
- Norusis, M. J. 1993. SPSS for Windows. Profession Statistics, Release 5. Chicago: SPSS.
- Odeh, A. M., and H. T. Battaine. 2002. “Causes of Construction Delay: Traditional Contracts.” International Journal of Project Management 20 (1): 67–73. doi:10.1016/S0263-7863(00)00037-5.
- Pereira, E., S. Ahn, S. Han, and S. Abourizk. 2018. “Identification and Association of High-priority Safety Management System Factors and Accident Precursors for Proactive Safety Assessment and Control.” Journal of Management in Engineering 34 (1): 04017041, 1–12. doi:10.1061/(ASCE)ME.1943-5479.0000562.
- Rourke, N. O., and L. Hatcher. 2013. A Step-By-Step Approach to Using SAS for Factor Analysis and Structural Equation Modeling. 2nd ed. Cary, NC: SAS Press.
- Sawacha, E., S. Naoum, and D. Fong. 1999. “Factors Affecting Safety Performance on Construction Sites.” International Journal of Project Management 17 (5): 309–315. doi:10.1016/S0263-7863(98)00042-8.
- Seo, J., S. Lee, and H. Kim. 2015. “Computer Vision Techniques for Construction Safety and Health Monitoring.” Advanced Engineering Informatics 29 (2): 239–251. doi:10.1016/j.aei.2015.02.001.
- Shapira, A., and B. Lyachin. 2009. “Identification and Analysis of Factors Affecting Safety on Construction Sites with Tower Cranes.” Journal of Construction Engineering and Management 135 (1): 24–33. doi:10.1061/(ASCE)0733-9364(2009)135:1(24).
- Shapira, A., and M. Simcha. 2009. “AHP-Based Weighting of Factors Affecting Safety on Construction Sites with Tower Cranes.” Journal of Construction Engineering and Management 135 (4): 307–318. doi:10.1061/(ASCE)0733-9364(2009)135:4(307).
- Shen, Y., T. Y. Koh., S. Rowlinson, and A. J. Bridge. 2015. “Empirical Investigation of Factors Contributing to the Psychological Safety Climate on Construction Sites.” Journal of Construction Engineering and Management 141 (11): 04015038, 1–10. doi:10.1061/(ASCE)CO.1943-7862.
- Tabachnick, B. G., and L. S. Fidell. 2007. Using Multivariate Statistics. 5th ed. Boston, MA: Pearson.
- Tam, C. M., S. X. Zeng, and Z. M. Deng. 2004. “Identifying Elements of Poor Construction Safety Management in China.” Safety Science 42 (7): 569–586. doi:10.1016/j.ssci.2003.09.001.
- Tam, C. M., Z. M. Deng, S. X. Zeng, and C. S. Ho. 2000. “Quest for Continuous Quality Improvement for Public Housing Construction in Hong Kong.” Construction Management and Economics 18 (4): 437–446. doi:10.1080/01446190050024851.
- Teo, E. A. L., and F. Y. Y. Ling. 2006. “Developing a Model to Measure the Effectiveness of Safety Management Systems of Construction Sites.” Building and Environment 41 (11): 1584–1592. doi:10.1016/j.buildenv.2005.06.005.
- Teo, E. A. L., F. Y. Y. Ling., and A. F. W. Chong. 2005. “Framework for Project Managers to Manage Construction Safety.” International Journal of Project Management 23 (4): 329–341. doi:10.1016/j.ijproman.2004.09.001.
- Terwel, K. C., and S. J. T. Jansen. 2015. “Critical Factors for Structural Safety in the Design and Construction Phase.” Journal of Performance of Constructed Facilities 29 (3): 04014068, 1–12. doi:10.1061/(ASCE)CF.1943-5509.0000560.
- Wang, J., P. X. W. Zou, and P. P. Li. 2016. “Critical Factors and Paths Influencing Construction Workers’ Safety Risk Tolerances.” Accident Analysis and Prevention 93 (3): 267–279. doi:10.1016/j.aap.2015.11.027.