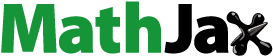
ABSTRACT
Light Steel Frame (LSF) system is mainly used for construction of short and intermediate-height buildings in developed countries whereas considerable heed is not given to it in developing countries. Unfamiliarity to LSF risks is one of the main reasons for this averseness so risk management can remedy this challenge and develop its application. Hence, this paper investigates the risk management of LSF considering design, construction and operation phase. Three steps entailing risk identification, assessment and responding using fuzzy Failure Mode and Effect Analysis (FMEA) technique are suggested for risk management implementation and validation of responses, a novel index with respect to weighted combination of project quality, time and cost is calculated. The methodology is demonstrated on a pilot study in a developing country. By using interview, 29 significant risks are extracted in design, construction and operation and then evaluated by proposed fuzzy method. Results showed that the share of the risks in these steps are 21%, 31% and 48% respectively. The results revealed that the risks in the construction and operation phases are higher than those in the design phase. The results also show that involving safety as a project object in the risk management process could eventuate acceptable results.
GRAPHICAL ABSTRACT
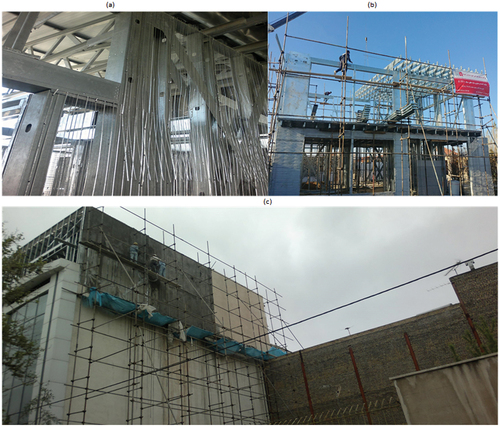
1. Introduction
The light steel frame (LSF) is a load-bearing wall system made of cold-formed steel sections (CFS) and has various applications in the construction industry, such as short, intermediate building and extra-floor residential houses or apartments. LSF components are made of CFS sheets with thicknesses varying from 0.45 to 2.45 mm and protected against rust and corrosion by using zinc alloys (Schafer Citation2011). The load-bearing elements consist of single or combined sections, mainly consisting of C or U shape or their combination. The walls are formed by the arrangement of the vertical components of the U shape sections (studs), which are restrained from above and below inside the horizontal U shape components (runner or track). The roof of the last floor is often sloped and made of CFS load-bearing members called “joists” and the track is typically referred to as the “rim joist”. Connections in LSF are usually cold and made with automatic screws, despite that other connections such as rivets and welding are used in special cases (Soares et al. Citation2017).
LSF system has multiple advantages including high-speed construction, low weight of building, resistance against earthquake and insect damage, almost 100% recyclability, economical usage of energy, the easiness of maintenance and repair, possibility of modular construction and pre-construction of panels, the comfortable construction of mechanical and electrical equipment, excellent thermal insulation, designability of various external views by request of employer and adapted with architectural concept, long lifespan, quick return of initial capital investment, adapted with environment, durability and stability of the structure and increase the net area (Soares et al. Citation2017). In contrast, LSF system has some disadvantages such as low resistance of wall insulation core against fire, complexity of thermal bridge modelling due to several types of materials, weakness against severe wind, the lack of expert and labour force, unknown structural behaviour of the system, higher prices than traditional materials in countries that have not yet developed this system and height limitation (Jatheeshan and Mahendran Citation2015; Soares et al. Citation2014).
Having been appeared in the early 20th century, most likely to mimic the dimensioned wood houses become the common construction method for shelter, LSF system grew rapidly in Europe. Also, destruction of buildings during the World War II caused shortage of homes in several countries such as Germany, Japan, France and hence LSF was one of the best alternatives to meet this demand (Yu Citation2016). Although the origin of LSF system was rooted in shortage of building materials, environmental concerns and introducing an alternative option for wood frame building, the mentioned advantages turned LSF constructions to a reliable option for construction industry in developed countries and one of the most popular system in dry (i.e. prefabricated) construction category (LSK Citation2007).
In contrast to developed countries, this approach was not clearly embraced in developing countries such as Iran, Malaysia, South Africa and China (Dosumu and Aigbavboa Citation2018; Mahdavinejad, Hajian, and Doroodgar Citation2012; Saikah et al. Citation2017; Shi and Yu Citation2009). Lack of information in practitioners, clients, engineers, project managers and other related experts about the LSF risks and proper strategies for dealing with them is one of the main reasons for failure to expand the LSF system in the construction industry (Luo et al. Citation2015). Similarly, the investigation in Australia and some cases in Italy and Mediterranean countries as a matter of successful examples also implies that the LSF system should be coordinated with consumers’ culture (Celik and Kamali Citation2018; Franklin, Heffernan, and McCarthy Citation2020). It was found that having more knowledge and experience about the LSF system dampens its current risks. Therefore, this paper aims to recognize the relevant risks in LSF structures and appropriate strategies to respond during design, construction and operation steps. To deal with this problem in a systematic approach, we intend to employ risk management procedure in LSF buildings in developing countries.
Accordingly, a comprehensive framework for investigation of LSF risks is provided here and this paper aims to increase the understanding and knowledge about the LSF system for engineers, managers, employers and other related people by applying the risk management to enhancing the chance of using this alternative building system. This framework entailing identification, assessment and responding to each risk event is defined in a way that it could be employed in similar problems and case studies.
This paper organises as follows: Firstly, a literature review of the works investigating the LSF risks and risk management in construction industry are given in the next section. The proposed framework steps are then introduced. The results and discussion are also represented in fourth section. Finally, the conclusions are drawn and some recommendations are made for future studies.
2. Literature review
2.1. LSF systems
Despite a plethora of research works conducted on structure of LSF system, there is not any specific research focused on the risk management in LSF system. Some sporadic researchers such as Shi and Yu (Citation2009), Barnard (Citation2011), Eren (Citation2013) and Saikah et al. (Citation2017) have focused on risk identification of LSF system but not as comprehensive as one could be considered them as risk management procedure. In a similar manner, Dosumu and Aigbavboa (Citation2018) investigated adopting LSF system in South Africa by considering risk identification and evaluation and reviewing challenges and solutions, but had not presented a specific framework for risk management. Franklin, Heffernan, and McCarthy (Citation2020) have also conducted an on-line survey for evaluation of structural resistance, construction time and cost and acoustics responses of LSF buildings and suggested some modifications on the LSF system.
Some researchers focused on a specific aspect of the system. For example, Veljkovic and Johansson (Citation2006) introduced the LSF systems as a dry construction system and studied the manufacturing industry in Sweden by examining ways to reduce production costs in a recession. Researchers also investigated the LSF technology for economic housing in developing countries such as Iran and China and concluded that LSF system can be economic for these countries (Li et al. Citation2014; Mahdavinejad, Hajian, and Doroodgar Citation2012; Noorzai and Golabchi Citation2020).
Some modifications in construction of the system were also provided by Darcy and Mahendran (Citation2008) and Schafer (Citation2011). Suggestions of these papers can be useful in energy efficient and affordable architectural concept of LSF system. A multidimensional comparison between reinforced concrete and LSF buildings was also made in Mediterranean countries and Iran (Celik and Kamali Citation2018; Zeynalian, Trigunarsyah, and Ronagh Citation2013). It has been proofed that using of LSF system allows a great improvement in cost, quality, time and earthquake-related risks.
Some LSF-related researchers analysed sustainable performance of LSF system with the main focus on environmental and energy saving aspects. Fallah (Citation2005) found steel and its derivatives are a very appropriate option with respect to sustainable development. For the energy saving subject see for example Soares et al. (Citation2014), Santos, Martins, and Silva (Citation2014), Soares et al. (Citation2017) and Steau and Mahendran (Citation2020). They suggested strategies such as changing the insulation core, use of modern construction methods that optimize heat exchange transference and double plasterboards for reducing thermal bridges and for improving the thermal resistance of LSF envelope elements. Similar to Trevathan and Pearse (Citation2008), Paul, Radavelli, and Da Silva (Citation2015) analysed the sound insulation coefficient in LSF walls. They tested materials such as cement and plaster boards, smart resin, PVC and polymer mortar covered XPS panels as a matter of insulation core. Besides, they examined the effect of using the sealing strip between panels and sub-runners.
Safety-related researches in this field were devoted to experimental and numerical studies for analysing the resistance of LSF system against fire and earthquake. For example, Jatheeshan and Mahendran (Citation2015) examined the fire resistance of LSF walls by finite element method and real experiments on constructed specimens. In their research, they have confirmed the high ability of the finite element method to model and demonstrate the performance of these structures subjected to fire. We can conclude from this research and other similar not mentioned papers that using of incombustible materials in insulation core of the walls significantly reduce the ignition risk.
Regarding seismic behaviour and structural analysis, it is shown that LSF system in combination with shear walls can be considered as an appropriate choice in areas with high seismic hazards or high important buildings like schools and hospitals based on experimental researches of Fiorino, Iuorio, and Landolfo (Citation2014), Iuorio, Fiorino, and Landolfo (Citation2014), Khalifa et al. (Citation2020) and Wang and Hutchinson (Citation2020).
2.2. Risk management in construction industry
Intending to apply risk management in LSF construction system, a brief literature review of the risk management in construction industry is provided here. Risk management in construction projects has been applied since 1990 to identify, analyse and respond to risk factors in a project and maximise the results of positive events and minimise the consequences of negative events effected project objectives (Renuka, Umarani, and Kamal Citation2014; Wang, Dulaimi, and Aguria Citation2004).
Construction projects are among the most important projects that are being implemented in any country. These projects are of great importance due to the consumption of many resources, the existence of different stakeholders and the impact on other sectors. One of the first applications of risk identification and classification can be seen in the research conducted by Mustafa and Al-Bahar (Citation1991). They categorised project risks based on project objectives into six groups of hazards including uncontrollable natural forces, physical, financial, political, design and job related ones. Zou, Zhang, and Wang (Citation2007) and Zou and Zhang (Citation2014) identified and classified risks of construction projects in China and Australia based on Project Life Cycle (PLC) defined through feasibility, design and construction phases. Other researchers also used PLC and project objectives for risk classification (Mehdizadeh et al. Citation2012; Zeynalian, Trigunarsyah, and Ronagh Citation2013). Also Goh, Abdul-Rahman, and Samad (Citation2013) used PLC to categorise university project’s risks in Malaysia, and calculated likelihood, impact and risk level for each risk. Oduyemi, Okoroh, and Fajana (Citation2016) suggested that by detection of risk factors in design stage, improvement in project goals was acquired and hence risk classification based in PLC can be useful.
Comparing risk importance in each class is one of the main objectives of risk classification. To compare risks in each class, statistical method is very common. For example, Wu et al. (Citation2019) classified risks in off-site constructions into four categories including general, design-related, construction-related, and people and organisation-related and compared expert’s opinion with statistical tests. Delphi technique, brainstorming, expert judgment and interview are the most common tools for risk identification and classification. Although risk identification and classification as a key part of risk management need no complex calculations, few researches have exclusively done this part of risk management and most of the papers report previous risks in their model (Renuka, Umarani, and Kamal Citation2014). Some researchers such as Dey (Citation2012) and Franklin, Heffernan, and McCarthy (Citation2020) simultaneously used literature review and risk identification techniques.
Several techniques such as probability-impact matrix, Monte Carlo simulations, likelihood occurrence of risk, Fuzzy Set Theory (FST) and FMEA could be used for risk assessment or evaluation (Renuka, Umarani, and Kamal Citation2014). The significance of the risks is usually determined based on probability of risk occurrence and the risks impact or degree of loss (Wu et al. Citation2019) or relation of some risk factors (Forcael et al. Citation2018; Wang et al. Citation2018).
In a general case, these methods require probability and effect of the risks in the project objective based on expert’s opinions. In projects, we deal with the risks associated with the project objectives. Therefore, a clear prerequisite for identifying project risks is a clear understanding of the project objectives (Liu, Zhao, and Yan Citation2016). For transferring expert judgment to numeric information for risk evaluation, we can use FST or fuzzy reasoning Membership Function (MF). Various publications since 1996 until now have shown the performance of this technique in risk assessment in construction projects (Chan, Chan, and Yeung Citation2009). Fuzzy rules have also been used in risk management in construction project (Asadi et al. Citation2018).
The next step in risk management is risk responding. Wu et al. (Citation2018) introduced five main categories for risk response methods including zone based, Work Breakdown Structure (WBS) based, trade-off based, optimisation based and other methods. The first three groups have fewer complex calculations and can be easily used in construction projects but it could not be known whether the risk response actions are the optimal solution in these methods. Seyedhoseini, Noori, and Hatefi (Citation2009) and Abdelgawad and Fayek (Citation2010) are the instances of the works in these groups respectively. On the other hand, the researchers in fourth and fifth groups such as Wu et al. (Citation2018) employed optimisation problems and heuristic or meta heuristic algorithm to reach an optimal answer among risk responses. These methods not only are defined by complex problem-solving algorithms but also need precise information about project objective such as cost, time and quality. It is a common way to firstly detect different risk response strategies that optimise the performance of construction projects and then relevant solutions to the best strategy are compared. Choudhry and Iqbal (Citation2013) proposed some response strategies entailing avoidance, transferring, reduction, sharing and retaining and ranked them based on experts’ judgment.
FMEA-based techniques investigate adverse effect of risks of the entire system during the failure of the system. These techniques have been applied to the US aerospace industry from the 1960s for safety and reliability analysis (Bowles and Peláez Citation1995). Then, they have been frequently used as a tool to evaluate the risk in various industries such as automotive health-related problems, marine fields, nuclear processes, electronic and asset management (Abrahamsen, et al., Citation2016; Baghery, Yousefi, and Rezaee Citation2018; Braaksma, Klingenberg, and Veldman Citation2013; Kang et al. Citation2017; Yeh and Chen Citation2014). In addition, it has widely been applied to construction management problems (Kim and Kim Citation2012). Abdelgawad and Fayek (Citation2010) applied FMEA for risk assessment of construction projects and used fuzzy logic to improve its capability with uncertain information of experts’ judgments similar to Cheng and Lu (Citation2015). Considering some modifications in the FMEA formulation, Seifi Azad Mard et al. (Citation2017) introduced a novel approach for risk evaluation of occupational outcomes. There are several other researches about implementation of FMEA in the construction industry that can be found in Chin et al. (Citation2009), Gargama and Chaturvedi (Citation2011), Liu et al. (Citation2019), Ma and Wu (Citation2019).
In contrast to focusing in a specific part of risk management, some researchers such as Wang, Dulaimi, and Aguria (Citation2004), Abdelgawad and Fayek (Citation2010), Dey (Citation2010), Dey (Citation2012) and Ahmadi et al. (Citation2017) proposed a framework for risk management in construction projects and consider the entire aspects of risk management in their methods. The risk responding procedure in these frameworks is related to risk assessment parameters and can be easily applied to all types of construction project. For example, Ahmadi et al. (Citation2017) proposed a framework for a roadway project consisting of risk evaluation and response based on FST and risks were evaluated based on probability, severity of consequence on project objectives and control ability of the project team.
In some researches, the risk management term is incompletely utilised for the above process in construction project (see e.g. Goh, Abdul-Rahman, and Samad (Citation2013)). Using risk management term, we should perform several accurate steps based on PLC from identifying to responding and controlling of project risks so it is better to say risk identification and analysing for above research. Ashley, Diekmann, and Molenaar (Citation2006) and Iqbal et al. (Citation2015) have completely defined risk management in construction projects and described the matters should be considered in applying risk management, but in more cases risk identification, evaluation and responding have been tangibly seized by researchers. Also, Choudhry and Iqbal (Citation2013) described risk management barriers in construction project and compared different tools in risk management based on expert judgment and showed that there was lack of systematic risk management in construction projects especially in developing countries.
As we want to apply risk management in special type of construction project (LSF system), some similar works in other types of projects have been hitherto done. Cause of delays in construction projects (Banobi and Jung Citation2019), investigation of risks magnitude in tunnel and railway projects by three simple risk indices including probability, severity and frequency (Forcael et al. Citation2018), risk analysing in modular construction (Li et al. Citation2013) and determination of risk importance in industrialized building system (IBS) projects (Bari et al. Citation2012) are some instances of risk identification and assessment in special type of projects.
2.3. The aims and innovations of the study
Previous researches on the risk management in the construction industry did not mainly focus on the implementation of the risk management in the LSF system and a wide knowledge gap was identified. This paper provides a comprehensive framework for risk management of the LSF system entailing risk identification, risk evaluation and proposing appropriate strategies to respond the risks during design, construction and operation steps. Moreover, due to the limited expansion of the LSF system in developing countries, this research would be of high importance for application of this system in these regions. The main contributions of the paper can be summarised as follows:
Identifying key risks of LSF structures;
Classifying the identified risks under PLC and other relevant subjects;
Evaluating the identified risks through a novel Fuzzy FMEA approach;
Proposing appropriate response strategies for the identified risks;
Demonstration of the proposed methodology in a real-world case study;
The FMEA method as a risk assessment technique can identify and evaluate potential risks and their causes and effects. Risk management of construction projects has many ambiguities and unknowns (Chin, Chan, and Yang Citation2008). These uncertainties sometimes result in either better or worse outcomes (Kumru and Kumru Citation2013). These uncertainties and associated risks can lead to some complexities between the project components and even unstable conditions that can change the project outcome due to some external reasons such as governmental laws (Chin, Chan, and Yang Citation2008). Fuzzy theory has shown to be a useful tool to deal with these types of uncertainties in the decision making.
Fuzzy theory is a computing method using “degrees of truth“ rather than the traditional ”true or false” (1 or 0) Boolean logic that underpins modern computers (Meng Tay and Peng Lim Citation2006). The concept of fuzzy sets was introduced by Zadeh in 1960s for the first time (Jong, Tay, and Lim Citation2013). In this approach, a fuzzy set described the concepts of a fuzzy number by using a degree of membership of its elements in a universe of discourse (Sang et al. Citation2018). Fuzzy numbers defined in the interval [0,1] provide semantics for terms in a linguistic term set, which are represented by MF that can be classified by types of functions. Fuzzy set theory is also used in a fuzzy inference system (FIS) to generate a model between inputs (features in the case of fuzzy classification) and targets (classes in the case of fuzzy classification). Due to the use of FIS, such transition may need a set of fuzzy rules in which gathering a complete one is difficult (Jee, Tay, and Lim Citation2015; Kerk et al. Citation2021). Previous researches have indicated all of the above concepts could adopt to the risk analysis due to the capability of fuzzy concept for modelling of uncertainty.
Combining the FMEA method with fuzzy theory provides a more efficient tool than the original FMEA method at the presence of vague concepts, insufficient information and uncertainty. Fuzzy logic could reduce the drawback in assessing and prioritizing failures of traditional FMEA (Chanamool and Naenna Citation2016). Hence, this paper provides a novel Fuzzy FMEA technique for risk assessment in construction projects.
3. Methodology
The proposed framework for dealing with risk management in the LSF system is illustrated in . The framework comprises three main phases including 1) risk identification and classification; 2) risk assessment; and 3) risk response. The first phase identifies the related hazards and potential risks to the LSF system through reviewing several LSF projects, relevant literature and interviewing relevant experts in these projects. The identified risks are also classified based on the life cycle, objectives and stakeholders of the projects.
The second phase entails quantifying the level of risk for each hazard identified in the LSF system by calculating risk parameters based on a Fuzzy FMEA approach. FMEA combines technology and experts’ experiences for identifying and planning for the removal of foreseeable failure modes of a product or process. It is used in various phases of the product life cycle in the manufacturing industries and is now becoming increasingly common in the service industry (Chin, Chan, and Yang Citation2008). In order to assess the risk level of a component or process, traditional FMEAs use the risk priority number (RPN). The RPN is determined by multiplying three factors: the probability/occurrence of failure, the seriousness of failure and the probability that a failure is not detected (Balaraju, Raj, and Murthy Citation2019; Chanamool and Naenna Citation2016; Kumru and Kumru Citation2013). Precision should not be imposed if the data is unreliable and scarce when conducting FMEA for safety assessment purposes. Hence, it would be unrealistic to ask an analyst or expert for scoring from 1 to 10 (as in the RPN method), for the various factors being examined. Although this simplifies the calculation, the probability is converted to another score system and the multiplication of factors is believed to cause problems. There are different relationships as either linear or nonlinear between probabilities and factors (Balaraju, Raj, and Murthy Citation2019).
A Fuzzy FMEA approach was utilized in this paper to overcome the weaknesses associated with the traditional RPN ranking system. As a proper guideline, the proposed method has been inspired by the in Balaraju, Raj, and Murthy (Citation2019). To this end, risks are then prioritised and ranked based on a multi-criteria decision analysis and fuzzy reasoning method. Appropriate strategies for risk response are then considered as solutions to mitigate the impact of each risk in phase three. A new objective-based index is defined based on the main criteria of the construction projects and used in this phase to prioritise the analysing strategies in this phase. The methodology is demonstrated through a real-world case study in Iran as a developing country. The steps taken at each phase are described below in further details.
3.1. Risk identification and classification
This step comprises two parts: (1) risk identification and (2) risk classification. Potential hazards and related risk events are first identified through various resources including interview with individuals involved in LSF construction projects and literature review of previous works (Luo et al. Citation2015). The interview is worthwhile because it can reveal new potential risks that have yet to be identified or analysed by researchers. The individuals participating in the interview could be from a wide range of expertise and different roles such as designers, workers, owners, engineers, residents and employers.
Risk classification is mainly used to compare the significance of the risk events in the classes sharing the same characteristics. Hence, identified risks in LSF system are classified here under three major categories with respect to: (1) PLC i.e. design, construction and operation; (2) main project objectives including cost, time, quality, safety and environmental sustainability; and (3) project stakeholders including clients, designers, contractors, government bodies and external issues (Zou and Zhang Citation2014; Zou, Zhang, and Wang Citation2007). Expert judgment is used here to identify the class of each identified risk through a questionnaire based on the greatest number of votes received for each class.
3.2. Risk assessment
This phase entails two main steps for risk analysis and prioritisation of the identified risks. The FMEA technique and the FST method are adopted here to analyse qualitative expert’s judgements and convert them to risk factors (Ahmadi et al. (Citation2017). This technique quantifies each risk with three main components including Control Number (CN) or the control ability of the project team to handling the risk, Probability of occurrence (P) and Consequence (C) of occurring corresponding hazard (risk magnitude) on the project criteria or objectives. In fact, the risk magnitude calculates severity of consequence for five project’s subcomponents entailing cost (Cc), quality (Cq), time (Ct), safety (Cs) and environment (Ce). Hence, Risk Criticality Number (RCN) in the FMEA method is defined as (hereafter fuzzy numbers are shown withand crisp (real) values are simple)
Thus, this model not only considers probability and impact of the risks but also involves the ability to control the risk and provides a comprehensive risk index for evaluation process. It should be noted that the CN index, indicating the ability of identifying or controlling the risk is performed in reverse; in other words, the higher risk control, the less severity it would have on the effect of risk and so smaller CN. Cost, quality and time are three common objectives in the construction industry but safety and environmental factors are added here due to their importance within the sustainability framework of development. Hence, the overall risk consequence () is calculated by using a weighted combination (i.e., related weights) of the above objectives:
To calculate the fuzzy number components of the with FST and FMEA methods, the following steps are needed which are described in more detail.
3.2.1. Step 1: Definition of linguistic terms
The same approach as Ahmadi et al. (Citation2017) is utilised for definition of qualitative factors or linguistic terms here. Linguistic terms for pairwise comparison of the criteria’s weight are strongly more, more, equal, less and strongly less with triangular MF and for and
are very low, low, equal, high and very high with trapezoidal MF.
3.2.2. Step 2: Determination of criteria’s relative weights
Risk consequence related to five criteria including cost, quality, time, safety and environmental issues and the relative weight of these criteria (Wc, Wq, Wt, Ws and We) are obtained with ten pairwise qualitative comparison of criteria relative preferences (i.e., cost vs time, cost vs quality, cost vs safety, cost vs environmental issues, time vs quality, time vs safety, time vs environmental issues, quality vs safety, quality vs environmental issues, safety vs environmental issues). The final criteria relative weights are obtained by implementation of fuzzy Analytic Hierarchy Process (AHP) technique which enables a pairwise comparison between these criteria by using
linguistic terms. Finally, relative weight of each criterion will be used to acquire a single severity of consequence for each risk event based on Equationequation (2)
(2)
(2) .
3.2.3. Step 3: Applying relative weight of experts
Determination of respondents’ score or weight usually is a part of risk analysis. To this end, respondents were ranked and weighted here based on their professional experience (from less than 5 years to over 30 years), job position (from simple worker to employer), and educational level (from elementary education to PhD). For each item, respondents could earn 1 to 5 score. So, for each person, final score ranges between 3 to 15. The relative weight of each expert is calculated by dividing the absolute weight of the expert by sum of absolute weights of all experts.
The expert chosen linguistic terms of and pairwise comparison of criteria (through questionnaire survey) are multiplied into the expert relative weight then by combining the expert judgments with
method into a single fuzzy number, the final MF of each component is obtained.
3.2.4. Step 4: Prioritising the risk events
To calculate a single fuzzy number for severity of consequence
is calculated from Equationequation 2
(2)
(2) using
method in the first step. Then, a single fuzzy number for probability of occurrence
and control ability
is also obtained by incorporating the fuzzy numbers of experts’ judgements. The fuzzy number of
is calculated by fuzzy multiplying of these three fuzzy numbers using
method through Equationequation (1)
(1)
(1) . The risks are ranked in accordance with their crisp values of
in doing so important risks have greater RCN.
3.3. Risk responding and validation of responses
Following consecutive steps lead to suitable responses for a risk event considering the risk response strategy. Also, a case-based validation scheme for evaluating the reliability and accuracy of the responses is suggested.
3.3.1. Step 1: Identifying possible risk solutions
Risk solutions, as open-ended form questionnaire the same as Dosumu and Aigbavboa (Citation2018), are questioned from experts for each risk. Hence a list of risk solutions is provided for each one.
3.3.2. Step 2: Calculating the risk response strategy
Abdelgawad and Fayek (Citation2010) proposed risk response strategy selection based on RCN value and Ahmadi et al. (Citation2017) modified this method considering RF and CN. The proposed method here as shown in considers crisp values of RCN, CN and RF in which the risk action is classified under four ranges: Range 1: risk acceptance; Range 2: risk transference; Range 3: risk mitigation/risk avoidance; Range 4: risk mitigation/risk avoidance/risk transference. The ranges are specified based on three crisp limits i.e., L1, L2 and L3 which are indicator of RF, CN and RCN decision limits. To this end, the fuzzy number is calculated with
method as:
By defuzzification of two states i.e. RCN-based state and CN-based state are generated in the response strategy chart shown in .
If the RF is lower than L1, the crisp value of RCN (without consideration of CN) determines the response strategy hence if RCN would be greater than L3, the risk assigns and the transference strategy is suggested else the risk can be accepted. In other words, risk transference is used for range 2 in our proposed method instead of acceptance in comparison to Ahmadi et al. (Citation2017) and it means that the risks with low control ability (high value of CN) should be transferred (Ashley, Diekmann, and Molenaar Citation2006). Note that the risks located in range 2 have small value of RF and high value of RCN due to high value of CN. For the risks that have RF value greater than L1 or located in CN-based state, the risks are assigned to each range based on CN and the RCN is not considered.
For justification about other ranges reader is referred to Abdelgawad and Fayek (Citation2010). We suggested 30% of maximum value of MF for then the average of CN for the risks with RF values greater than L1 is considered as L2. In other words, the predefined MF of P and C are within the range of 0 to 10 in this paper so the RF value would be within 0 and 100. With this aim, L1 is equivalent to 30 and the average of CNs for the risks that have RF value greater than 30 are considered as L2. The value of L3 is approximated based on risks located near the point (L1,L2) providing that the values of RCN are alleviated toward centre (for details see in Ahmadi et al. (Citation2017)).
3.3.3. Step 3: Validation of risk responses
To establish the reliability of the survey, Choudhry and Iqbal (Citation2013), Oduyemi, Okoroh, and Fajana (Citation2016) and Forcael et al. (Citation2018) used statistical methods such as correlation between results and hypothesis test and acceptable range for the results is mathematically calculated in this manner. In a different manner, Wang, Dulaimi, and Aguria (Citation2004) and Dey (Citation2012) validated their methods based on expert judgment about research findings. If we want to use statistical or mathematical methods in the results validation, the risk evaluation should be also done based on them. Because the risk evaluation is performed based on expert judgment, validation of results once again by experts would not be a scientific manner and will be intensively influenced by respondent responses. Hence, we avoid of using of these methods for validation of responses.
In this paper, the Scope Expected Deviation (SED) index proposed by Seyedhoseini, Noori, and Hatefi (Citation2009) as shown in Equationequation (4)(4)
(4) is used in order to validate the responses. The SED index will be used in the case that we have only one project and all the scopes are defined and information about time, quality and cost of the project are needed. For this purpose, the procedure should be performed in a pilot LSF project the same as Asadi et al. (Citation2018).
In the above formula, the zero index in quality, time and cost (Q0, T0, C0) means the aim of the project while the ultimate state of quality, time and cost are shown with Q, T and C respectively. Seyedhoseini, Noori, and Hatefi (Citation2009) suggested drawing of WBS, Quality Breakdown Structure (QBS) and Cost Breakdown Structure (CBS) and determination of final time, quality (equal to 1) and cost based on these charts. The final value of cost, time and quality (or specification of project outputs) have been broken down hierarchically to lower levels based on expert judgment or Delphi method. To calculate the project quality, reaching to project specifications is measured and the final quality is obtained by summation of independent items’ quality and production of dependent ones.
After calculation of the effect on each risk action on these three criteria, the SED index is obtained. If the suggested solutions generate negative value of RCN, the results will be validated and vice versa. For more description the reader is referred to Seyedhoseini, Noori, and Hatefi (Citation2009).
It should be noted that it is possible to include safety and environmental issues in the SED index but there is not a simple and practical manner for determination of these factors after and before the risk solutions. To this end, for each risk the SED is calculated for all suggestive solutions that coordinated to risk response strategy. If minimum value of SED among suggestive solutions is negative for all the risks, the validity of the results is confirmed. Should we offer a proper justification or recommendation for solutions with positive SED, the results can still be accepted otherwise some necessity actions should be suggested for them.
As shown in , the questionnaire is used for gathering the expert’s views. The structure of the questionnaires given to each respondent is shown in . Risk consequence is related in this research to five criteria including cost, quality, time, safety and environmental issues and the first questionnaire (first row of ) is related to preference of cost, quality, time, safety and environmental issues (Wc, Wq, Wt, Ws and We). So, this questionnaire consists of ten questions about pairwise comparison of criteria’s preferences and finally provides a relative weight for each criterion. The questionnaire is also comprised nine other sections (second to tenth row of ) to identify three main components such as and five subcomponents including
and
If N risks were identified through risk identification process, N + 1 sets of questionnaires are given to each respondent. The first one in this set has only Questionnaire No. 1 but other sets include the Questionnaires No. 2, 3, …, 9 and 10 for each risk. Additionally, risk class and risk solution, as open-ended form (ninth and tenth rows of ) the same as Dosumu and Aigbavboa (Citation2018), are questioned from experts.
Table 1. The structure of the questionnaire.
4. Results and discussion
LSF buildings in the design, construction and operation phase are applied as a case study in this research in two main cities of Iran, as a developing country i.e. Tehran and Mashhad. The data for identifying the key risks was collected through a 3-months face to face interview with engineers, designers, residents, employers and other related persons to designing, construction and operation phase of LSF system. Overall, through information collected from interviews and a comprehensive reviewing of literature, 29 risks (N=29) are extracted. In the next step, 30 questionnaires as defined in were sent by hand or through an email to ask people’s opinion and among them, 132 persons (representing about 60% of the sample frame) filled and returned the questionnaires. These 132 interviewees earned 58 % of total scores of respondents’ weight considering professional experience, job position and educational level.
4.1. Identified risks
lists the identified risks and other characteristics of each one (described in the following sections). Among them, DAC (Barnard Citation2011; Celik and Kamali Citation2018), RWF (Darcy and Mahendran Citation2008; Yu Citation2016), DEB (Khalifa et al. Citation2020) and IG (Zeynalian, Trigunarsyah, and Ronagh Citation2013) were extracted from literatures. Note that we merged similar risk suggested by interviewees as one risk, for example different problems related to façade of LSF buildings were stated by some people and we represented all these issues as DFI risk.
Table 2. Calculating the model’s components for identified risk in the ascending order of RCN.
4.2. Classification of identified risks
The second column of shows the classification of each risk based on PLC, project objective and stakeholders respectively. For better illustration, association of risks in PLC with stakeholders and objective shown in with two fishbone diagrams. It is concluded that the share of risks in design, construction and operation steps are 21%, 31% and 48%, respectively. This finding is partly in line with Zou, Zhang, and Wang (Citation2007), Bari et al. (Citation2012), Mehdizadeh et al. (Citation2012), Goh, Abdul-Rahman, and Samad (Citation2013) and Forcael et al. (Citation2018), which showed more risks are related to construction than designing in the construction industry. But technically, they did not consider operation because they thought operation risks have root in designing or construction. On the other hand, albeit Zou and Zhang (Citation2014) had considered operation phase in their model, they identified more risks in construction than other phases in high-rise building projects.
Figure 3. The fishbone diagram in accordance to stakeholder vs PLC (first panel) and objectives vs PLC (second panel).
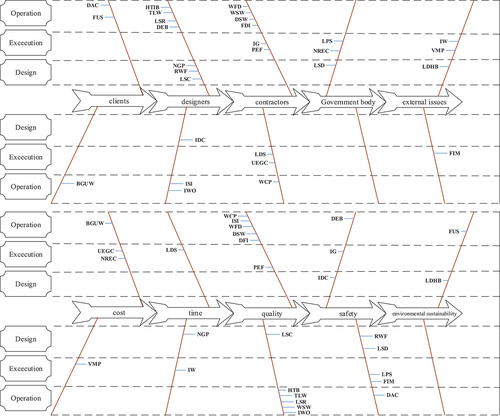
. The fishbone diagram in accordance to stakeholders vs PLC (first panel) and objectives vs PLC (second panel)
Although we only located each risk in one class based on experts’ opinion, the classification results were mostly the same with other research. For example, locating of LPS in safety category (Zou, Zhang, and Wang Citation2007), LSC in designing (Mehdizadeh et al. Citation2012), IW in construction or construction (Zou and Zhang Citation2014), VMP in external issues (Forcael et al. Citation2018), VMP and FIM in safety (Lu et al. Citation2018) are some instances. Some inconsistencies have also been shown in classification in contrast to other researches. Forcael et al. (Citation2018) assigned hazardous conditions to contractors but FIM is located in external issues class based on our experts. Several reasons such as not carrying out fire preventing actions, using of arsonist materials in building construction and lack of firefighting equipment in workshop during construction phase can be expected on the occurrence of ignition in construction projects. In contrast to these contractor-related factors, some external reasons like wind, thunderbolt and electrocution could be mentioned for firing’s cause and our experts classified FIM into second group.
The majority of contractor’s risks are related to construction (Zou and Zhang Citation2014) but our experts ascribed these to both construction and operation phases. It means that if the contractor incorrectly performs the construction process, this might cause defects in operation phase. For example, DSW or hearing some annoying noises from LSF walls could be caused due to wrong installation of studs in construction phase. But it will be usually discovered in thermal expansion and contraction conditions (i.e., studs’ length may be reduced or augmented because of expansion and contraction) after installing gypsum board and painting.
Liu, Zhao, and Yan (Citation2016) stated that lack of labour’s experience effects on project quality, but our finding showed that the UEGC risk impacts on the cost of project. This risk derived from labour’s mistake causes rework in project, so excess time and cost (time or cost overrun) are needed and the effect of cost is greater than time based on our experts.
4.3. Determination of the risk response strategy
The risk response range is illustrated in last column of based on the RCN, RF and CN described in the third, fourth and fifth columns of . To calculate the limits based on considered MF, L1 is equivalent to 30 and L2 is obtained equal to 3.59 (average of CNs for the risks that have RF value greater than 30). Based on the risks located near the point (30, 3.59) the value of L3 is approximated to 149. The risks in each range and their classification in terms of PLC (design with triangular, construction with circle and operation with square shape) are shown in .
Figure 4. Assignment of risk response range in term of design (triangular), construction (circle) and operation (square) phase.
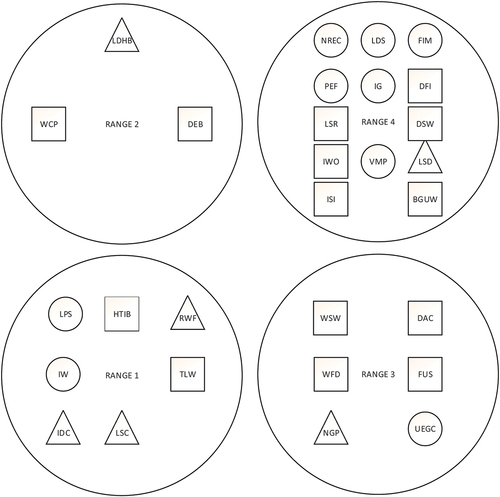
We can conclude that only 15% of the risks can be accepted based on the proposed method and the solutions for other risks should be defined considering suitable response strategy. Among non-accepted risks, only three risks including LDBH, WCP and DEB are located in range 2 in which there are low probability of occurrence, risk impact and control ability.
Dealing with these risks, we have not several solutions for controlling them. Our model suggested transferring strategy instead of acceptance for these risks. For instance, should we want to construct a high rise building with LSF system (LDBH), Ahmadi et al. (Citation2017)’s model accepts this risk and has any solution. Combination of shear wall with LSF system is a good and feasible choice and may be considered as a transfer strategy. Although Franklin, Heffernan, and McCarthy (Citation2020) stated that overdesigning could occur in combination of other structure systems with LSF, using of the LSF system can reduce danger of earthquake for some seismic regions. Construction of LSF combined with shear wall for a 7-story school is shown in left panel of . Yu (Citation2016) suggested panelization or assembling the components of the LSF in a controlled manufacturing environment, and this system as shown in right panel of fits very well in high-rise buildings.
Figure 5. Left panel: Combination of LSF system with shear wall in a 7-story school. Right panel: panelization in LSF buildings (Yu Citation2016).
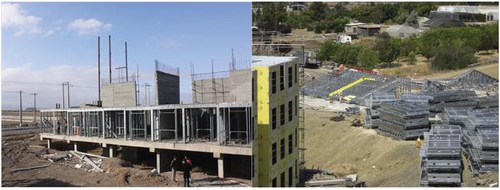
If high-rise buildings were executed with LSF system, the construction industry in developing countries would enable to reach a rapid expansion and as mentioned by Fallah (Citation2005) and Celik and Kamali (Citation2018) a recyclable construction system with a lot of positive environmental impacts regarding its sustainability, refurbishment, recyclables and reusability issues. For a specific detail, Celik and Kamali (Citation2018) mentioned minimum rework, waste and preparation work in running piping and electrical wiring in LSF system. Hence, this risk has a constructive impact on the environmental sustainability and this is the main reason of categorizing this risk and FUS in environmental sustainability group by our experts. In other words, if people’s perception and feeling of unreliability of LSF structure is reduced, an environmentally friendly system with many environmental benefits will expand.
4.4. Discussion on the risks’ magnitude
After applying the model on the data obtained from the interviews and questionnaires, computational indexes for each risk were calculated. Risks are sorted by RCN in third column of . The objectives’ weights were assigned equal to Wq = 0.14, Wc = 0.4, Wt = 0.22, Ws = 0.13 and We = 0.11 by experts’ judgment and fuzzy AHP technique from pairwise comparisons of the criteria (results are not shown) and these weights are the same for all the risks. As an example, the single fuzzy numbers related to the five criteria ( and
for consequence of the risk event of IWO is shown in respectively.
Having incorporated these fuzzy numbers ( and
the single fuzzy number
is then obtained for each risk event by Equationequation (2)
(2)
(2) . The combination of these five criteria using relative weights and the
method yields
shown in the first panel of for IWO. This fuzzy number
shown in the fourth panel of is resulted by the fuzzy multiplication of
,
and
based on Equationequation (1)
(1)
(1) . These fuzzy values (
and
) are also calculated by combining the experts’ judgment for this risk event shown in second and third panels of . Also, the fuzzy number of
based on Equationequation (3)
(3)
(3) is shown in the last panel of .
To check the reliability of risk’s ranking, a comparative analysis on the risk importance, risk response strategy and risk solutions is performed with other works discussed the same risk as us. Since the question on the solution to the risks was open-ended i.e. the respondents were required to mention and explain their opinions, for the sake of brevity all the solution’s results were not reported and principal items have been briefly discussed. It is worth mentioning that some unimportant risks in this research like LSC, DAC, RWF and NGP are region-sensitive and if a similar research is done in a different country, the risk’s rank may be changed. For example, the danger of corrosion (DAC) in most of the provinces in Iran is low or designer usually consider lowest possible wind speed in construction design in Iran. So, this could be the possible reason why the respondents did not consider DAC and RWF as highly ranked risks.
DFI: The DFI relates to dry façade in LSF buildings is shown in . Two unbearable problems relating to dry facades were extracted from expert’s suggestions. The first which has low importance is occurred in striking some heavy things like stone to dry facades (right panel in ) but the second risk that also has high probability in Zeynalian, Trigunarsyah, and Ronagh (Citation2013) means that the dry façade destructs during the time because of bad construction or insulation (left panel in ) because of penetration of rain water into the building facades.
Figure 8. Dry facades problems in LSF system: right panel: striking some heavy things to dry facades. Left panel: bad performance of construction or insulation.
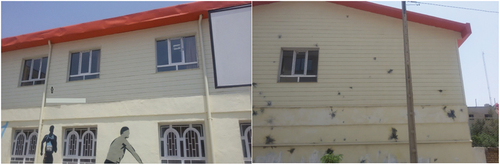
A solution for that is to use movable roof for the purpose of prevention against raining. Of course this is too expensive, and using of insulated material such as sarking materials (Barnard Citation2011) can be more feasible. Other remedies suggested by Soares et al. (Citation2017) are using two membrane layers and using external wind-tightness layer for avoiding moisture. Yu (Citation2016) opined that failure in workmanship of facades caused this risk. They proposed offsite construction and prefabrication as a transferring strategy. Also, a feasible and optimized solution called white cement facades, discussed in validation section, was suggested by one expert.
IWO: Among all risks related to LSF system identified in the current research, IWO is the one with higher priority. Most of individuals interviewed have declared that the most important problem of dry-wall systems is the impossibility of installing heavy objects on the walls. As shown in , there are many solutions to this issue including use ribbed plastic anchor, self-drilling anchor, toggle bolts, molly bolts and marking the place of studs on walls or finding the studs placement. In addition, walls with double boards have more capacity for installing heavier objects (Veljkovic and Johansson Citation2006). LSK (Citation2007) has also suggested some useful guidance about screw, pin, clinch and rivet in LSF walls.
Figure 9. (a): ribbed plastic anchor, (b): self-drilling anchor, (c): toggle bolts, (d): molly bolts and (e): marking the place of studs.
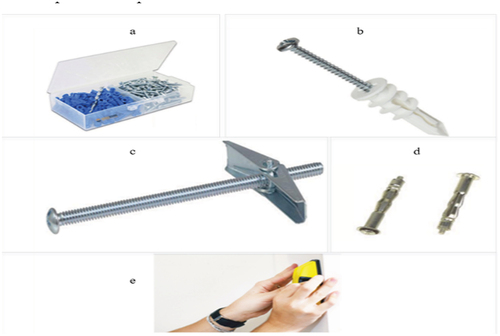
Despite these solutions, designers, engineers and clients have a negative attitude to dry-wall system among respondents specially because of comparing to masonry systems; in other words, there is a relationship between this risk and FUS; however, possibly people’s awareness to dry-wall system can be very effective in their belief.
4.5. Comparing average of RCN for each class
draws a comparison between the risks of the PLC, project objective, project stakeholders and risk response range classes based on average acquired RCN. Results show that construction, cost, contractor and range 4 have higher importance among other classes.
Figure 10. The average RCN of the risks in the PLC, project objective, project stakeholders and risk response range classes.
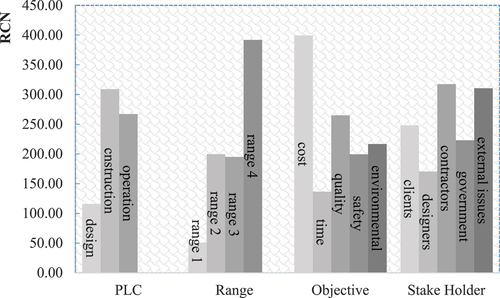
Based on the ranges of the PLC risks, those belonging the higher RCN i.e. range 4 seems rational. On the other hand, we can conclude from that the importance of construction and operation risks are higher than that in design risks. It should be noted that literature has different findings for the level of importance of the relevant risks that can be either in line or against the findings of this study. For example, Wang et al. (Citation2018) showed the most significant risks based on Pareto principle are those related to the operation phase while Mehdizadeh et al. (Citation2012) showed that risks associated with the construction phase are more important than the design risks in construction projects. Contradictory findings have also been reported for prefabricated buildings in which the design risks have the greatest impact on the final performance of the system (Yuan et al. Citation2020). These various results can be due to the several reasons such as construction methods, the risk analysis model entailing meta network analysis, grounded theory, Analytic Network Process (ANP), the linear weighted sum method and structured self-intersection matrix that might have been effective in these conclusions. Some other related justifications and discussions can be found in Xiahou et al. (Citation2018) and Lu et al. (Citation2018).
The cost and quality are the most important objectives in this model and time has the minimum average of RCN. But Zou and Zhang (Citation2014) consider cost and time as important risk’s group. Having higher speed of construction procedure in LSF buildings than conventional buildings can justify this contradiction so the time-related risks have lower important. Zeynalian, Trigunarsyah, and Ronagh (Citation2013) stated that fabrication and installation of LSF components in the factory could enhance the control ability of time and quality related risks.
4.6. Effect of CN in the risk evaluation
Only do conventional methods consider probability and impact of the risk in determination of risk magnitude while control ability is also considered in determination of risks’ rank here. Intending to discard CN in risk evaluation, we can evaluate risks based on RF. The risk rank based on RCN, RF and absolute difference between them are shown in .
We can conclude that the maximum difference in risks’ rank is appeared in risks with low or high value of CN. For example, the WFD and IWO risks are the highest important risks based on RF and RCN. The low value of RCN for WFD shows that this risk could be controlled easily in contrast to IWO. Considering CN in risk evaluation is more rational than discarding this index because clients deal with IWO risk more than WFD based on our observation and it is more suitable as a high ranked risk.
Another important finding from is related to FUS, having maximum value of difference. Although this risk has a higher importance without consideration of CN, the high ability of controlling this risk makes it as a 15th important risk. It could be concluded that by introducing LSF risks and managing them, the main objective of this study, we can reduce the impact of FUS especially in developing countries.
4.7. A pilot study for results’ Validation
The methodology was illustrated and validated through its application to the pilot case study once the Fuzzy FMEA model was developed for the identified risks entailing evaluation and responding procedure. The following approach with the aim of the SED criteria was applied to validate the solutions for each risk.
A pilot study comprising a two-story LSF residential building in Iran with 220 m2 built-up area in design phase with stick’s construction method (Yu Citation2016) was considered. The WBS, CBS and QBS of this building was designed by the project team brainstorming the construction process suggested by Barnard (Citation2011) and Eren (Citation2013) based on ten phases including Ph1: casting the concrete slab, Ph2: runner and stud’s erection, Ph3: screw fixing, Ph4: roof erection, Ph5: Insulation and weatherproofing, Ph6: plumbing and electrical services, Ph7: gypsum board attaching, Ph8: doors and windows installation, Ph9: façade’s installation and coating and Ph10: painting. The SED calculations in the above project phases are shown in the first row of . Note that the final quality (the value of quality in for SED computation) in the planning state was assumed equal to 1.
Table 3. SED calculations in terms of project’s phases for target state, UEMLMC and FRP response solutions.
The WBS chart of the project tasks is shown in . The implication of each risk solution(s) based on SED was calculated for each phase and the final values of cost, time and quality were determined. Note that the modified values of objective’s weights without consideration of safety and environmental issues based on pairwise fuzzy calculation are Wt = 0.24, Wc = 0.42 and Wq = 0.34. The results show that increasing in the SED was shown only in one risk. Only two important cases are reported here for brevity.
DFI obtained minimum value of SED among all the risks. After removing improper responses to risk strategies, the best solution for this risk (has minimum value of SED) is “using expanded metal lath with white mortar cement (UEMLMC)” instead of dry façade as shown in . To use this solution, the expanded metal lath should be screwed to studs and runners (panel (a) and (b) in ) and then white (or other colours) mortar cement covers all the metal lath and thus a flat white surface will be obtained (panel (c) in ).
Figure 13. Panel (a) and (b): Screwing expanded metal lath on studs and runners. Panel (c): Covering white mortar cement on the metal lath.
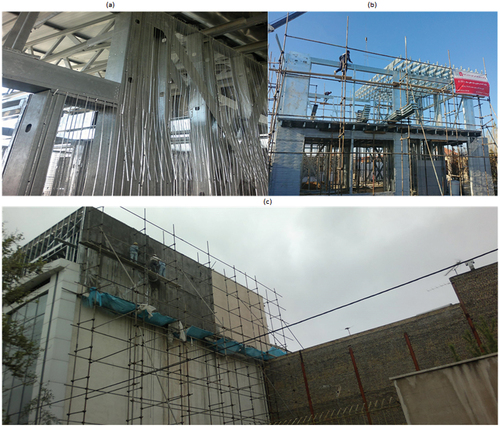
This solution reduces the façade’s cost from $3,000 to $1,900 and has no change in the project time because of being parallel with other tasks (based on , this task has 6 days buffer). A part of the project QBS is shown in and the quality of the project specification based on the project team opinion is specified without and with consideration of suggested solution (final quality for second condition is (0.4 + 0.05 + 0.2)0.4 = 0.26). The SED calculation for the project phases considering UEMLMC response solution for the risk event DFI isshown in the second row of .
Despite different solutions mentioned by several authors and our experts for FIM (Jatheeshan and Mahendran Citation2015; Veljkovic and Johansson Citation2006), the SED values for all these solutions are positive. Among the suggested solutions, Fire-Resistant Plasterboard (FRP) was chosen as the best one with minimum value of SED for this risk. Fire resistant gypsum boards are 60% more expensive than normal ones that have effects on project time and quality so the SED is nearly equal to 1%.
The high value of RCN for FIM implies that an applicable preparation should be considered for this risk, but SED has no recommendation for a proper solution. The main reason for this inconsistency can be referred to the exclusion of safety in SED calculation as a criterion. In other words, the main effect of FRP is on the project safety instead of quality, time and cost. Calculation of SED based on safety can be considered for future researches. The SED related calculations in terms of project’s phases considering FRP response solution for the risk event FIM is shown in the third row of .
Applying the proposed method on the above real case revealed that the selected solutions generally can obtain remarkable validations based on the expert judgments. However, the solutions may need some modifications and justifications in some complicated risks hence considering experts’ opinion is highly recommended after implementation of this method.
4.8. The key findings of the study
In this subsection, the findings of this paper are summarised as some practical guidelines. They might be useful in other related cases and problems to prevent any adverse outcomes of the risks. The following key findings can be noted from the application of the methodology in the paper
Based on the PLC classification, the risks in the operation phase are larger than those in the design and construction phases.
Only 15% of the identified risks in the construction projects could be accepted.
Due to being an environmentally friendly system, decreasing people’s unreliable feelings to the LSF system causes expansion of the system and then several environmental benefits.
Among non-accepted risks, three risks including cracks in the walls, limitation in designing of high-rise buildings and danger of explosion and blast have low probability of occurrence and risk impact, but control ability against them is very limited. Hence, transferring strategy is a reasonable choice for dealing with these risks.
Some of the identified risks relating to the average temperature, corrosion and wind force in this study are specific to the region and country of the case study and hence different results may be obtained in other countries.
Implementation of dry façade and dry walls may have major challenges and need principal considerations and modifications in the design phase.
Construction and operation risks have higher importance than design risks based on the average obtained RCN.
Investigation of the safety as a criterion in the risk management process can give more acceptable results.
5. Conclusions
This paper contributes to the body of knowledge of risk management implementation in the LSF systems by using the Fuzzy FMEA approach. Risk management main process entailing identification, evaluation and response was applied to the design, construction, and operation steps of the LSF system in a pilot study in Iran as a developing country. 29 important risks were extracted through interviewing with people related to the LSF system. The proposed Fuzzy FMEA model considered five criteria entailing cost, quality, time, safety and environmental issues and determined risk magnitude based on three components comprising the control ability of the project team to handling the risk, probability of occurrence and consequence on the project criteria.
Results revealed that the share of risks in design, construction and operation steps are 21%, 31% and 48% respectively and the construction and operation risks have higher importance than design risks. Also, the cost and quality are the most important criteria in this model according to average of risk magnitude. Using Multiple-Criteria Decision Making (MCDM) under Hesitant fuzzy sets is recommended for identification and risk analysis of sustainable building projects in future works.
Data Availability Statement
Some or all data including the questionnaire information, fuzzy computations, etc., that support the findings of this study are available from the corresponding author upon reasonable request.
Additional information
Notes on contributors
Ali Yeganeh
Ali Yeganeh is currently a PhD graduated at the Department of Industrial Engineering, Faculty of Engineering, Ferdowsi University of Mashhad in Mashhad, Iran. He has graduated from Ferdowsi University of Mashhad with a master's degree in Civil Engineering. His main research interests are statistical process control and profile monitoring.
Moein Younesi Heravi
Moein Younesi Heravi received the master's degree in civil engineering with a specialty in construction management and engineering from Ferdowsi University of Mashhad. His background includes working on construction safety, risk management and data analytics.
Kourosh Behzadian
Kourosh Behzadian is currently associate professor and program leader of civil engineering at the University of West London, UK. He is expert in water and environmental engineering including planning and management of water systems, urban water and wastewater systems, urban flood management and environmental protection and waste management.
Hashem Shariatmadar
Hashem Shariatmadar is a Professor at the Department of Civil Engineering, Faculty of Engineering, Ferdowsi University of Mashhad in Mashhad, Iran. His main research interests are earthquake and concrete.
References
- Abdelgawad, M., and A. R. Fayek. 2010. “Risk Management in the Construction Industry Using Combined Fuzzy FMEA and Fuzzy AHP.” Journal of Construction Engineering and Management 136 (9): 1028–1036. doi:10.1061/(ASCE)CO.1943-7862.0000210.
- Abrahamsen, H. B., E. B. Abrahamsen, and S. Høyland. 2016. “On the Need for Revising Healthcare Failure Mode and Effect Analysis for Assessing Potential for Patient Harm in Healthcare Processes.” Reliability Engineering & System Safety 155: 160–168. doi:10.1016/j.ress.2016.06.011.
- Ahmadi, M., K. Behzadian, A. Ardeshir, and Z. Kapelan. 2017. “Comprehensive Risk Management Using Fuzzy FMEA and MCDA Techniques in Highway Construction Projects.” Journal of Civil Engineering and Management 23 (2): 300–310. doi:10.3846/13923730.2015.1068847.
- Asadi, P., J. R. Zeidi, T. Mojibi, A. Yazdani-Chamzini, and J. Tamošaitienė. 2018. “Project Risk Evaluation by Using a New Fuzzy Model Based on Elena Guideline.” Journal of Civil Engineering and Management 24 (4): 284–300. doi:10.3846/jcem.2018.3070.
- Ashley, D. B., J. E. Diekmann, and K. R. Molenaar. 2006. Guide to Risk Assessment and Allocation for Highway Construction Management. US Department of Transportation, Federal Highway Administration.
- Baghery, M., S. Yousefi, and M. J. Rezaee. 2018. “Risk Measurement and Prioritization of Auto Parts Manufacturing Processes Based on Process Failure Analysis, Interval Data Envelopment Analysis and Grey Relational Analysis.” Journal of Intelligent Manufacturing 29 (8): 1803–1825. doi:10.1007/s10845-016-1214-1.
- Balaraju, J., M. G. Raj, and C. S. Murthy. 2019. “Fuzzy-FMEA Risk Evaluation Approach for LHD machine–A Case Study.” Journal of Sustainable Mining 18 (4): 257–268. doi:10.1016/j.jsm.2019.08.002.
- Banobi, T., and W. Jung. 2019. “Causes and Mitigation Strategies of Delay in Power Construction Projects: Gaps between Owners and Contractors in Successful and Unsuccessful Project.” Sustainability 11 (21): 5973. doi:10.3390/su11215973.
- Bari, N. A. A., R. Yusuff, N. Ismail, A. Jaapar, and R. Ahmad. 2012. “Factors Influencing the Construction Cost of Industrialised Building System (IBS) Projects.” Procedia - Social and Behavioral Sciences 35: 689–696. doi:10.1016/j.sbspro.2012.02.138.
- Barnard, D. 2011. Light Steel Construction and Modular Homes as Alternative Building Methods in South Africa. South Africa: University of Pretoria.
- Bowles, J. B., and C. E. Peláez. 1995. “Fuzzy Logic Prioritization of Failures in a System Failure Mode, Effects and Criticality Analysis.” Reliability Engineering & System Safety 50 (2): 203–213. doi:10.1016/0951-8320(95)00068-D.
- Braaksma, A. J. J., W. Klingenberg, and J. Veldman. 2013. “Failure Mode and Effect Analysis in Asset Maintenance: A Multiple Case Study in the Process Industry.” International Journal of Production Research 51 (4): 1055–1071. doi:10.1080/00207543.2012.674648.
- Celik, T., and S. Kamali. 2018. “Multidimensional Comparison of Lightweight Steel and Reinforced Concrete Structures: A Case Study.” Tehnicki Vjesnik 25 (4): 1234-1242.
- Chan, A. P. C., D. W. M. Chan, and J. F. Y. Yeung. 2009. “Overview of the Application of Fuzzy Techniques in Construction Management Research.” Journal of Construction Engineering and Management 135 (11): 1241–1252. doi:10.1061/(ASCE)CO.1943-7862.0000099.
- Chanamool, N., and T. Naenna. 2016. “Fuzzy FMEA Application to Improve Decision-making Process in an Emergency Department.” Applied Soft Computing 43: 441–453. doi:10.1016/j.asoc.2016.01.007.
- Cheng, M., and Y. Lu. 2015. “Developing a Risk Assessment Method for Complex Pipe Jacking Construction Projects.” Automation in Construction 58: 48–59. doi:10.1016/j.autcon.2015.07.011.
- Chin, K.-S., A. Chan, and J.-B. Yang. 2008. “Development of a Fuzzy FMEA Based Product Design System.” The International Journal of Advanced Manufacturing Technology 36 (7–8): 633–649. doi:10.1007/s00170-006-0898-3.
- Chin, K.-S., Y.-M. Wang, G. K. K. Poon, and J.-B. Yang. 2009. “Failure Mode and Effects Analysis Using a Group-based Evidential Reasoning Approach.” Computers & Operations Research 36 (6): 1768–1779. doi:10.1016/j.cor.2008.05.002.
- Choudhry, R. M., and K. Iqbal. 2013. “Identification of Risk Management System in Construction Industry in Pakistan.” Journal of Management in Engineering 29 (1): 42–49. doi:10.1061/(ASCE)ME.1943-5479.0000122.
- Darcy, G., and M. Mahendran. 2008. “Development of a New Cold-Formed Steel Building System.” Advances in Structural Engineering 11 (6): 661–677. doi:10.1260/136943308787543621.
- Dey, P. K. 2010. “Managing Project Risk Using Combined Analytic Hierarchy Process and Risk Map.” Applied Soft Computing 10 (4): 990–1000. doi:10.1016/j.asoc.2010.03.010.
- Dey, P. K. 2012. “Project Risk Management Using Multiple Criteria Decision-making Technique and Decision Tree Analysis: A Case Study of Indian Oil Refinery.” Production Planning & Control 23 (12): 903–921. doi:10.1080/09537287.2011.586379.
- Dosumu, O., and C. O. Aigbavboa. 2018. “Adoption of Light Steel (LS) for Building Projects in South Africa.” Journal of Engineering, Design and Technology 16 (5): 711–733. doi:10.1108/JEDT-04-2018-0075.
- Eren, Ö. 2013. “A Comparison with Light Steel Frame Constructional Building Systems for Housing.” World Applied Sciences Journal 25 (3): 354-368.
- Fallah, M. H. 2005. “Sustainable Building Construction“. World Sustainable Building Conference. https://www.irbnet.de/daten/iconda/CIB3980.pdf
- Fiorino, L., O. Iuorio, and R. Landolfo. 2014. “Designing CFS Structures: The New School Bfs in Naples.” Thin-Walled Structures 78: 37–47. doi:10.1016/j.tws.2013.12.008.
- Forcael, E., H. Morales, D. Agdas, C. Rodríguez, and C. León. 2018. “Risk Identification in the Chilean Tunneling Industry.” Engineering Management Journal 30 (3): 203–215. doi:10.1080/10429247.2018.1484266.
- Franklin, N., E. Heffernan, and T. McCarthy 2020. “The Case for Cold-Formed Steel Construction for the Mid-Rise Residential Sector in Australia: A Survey of International CFS Professionals“. Proc., ACMSM25, Springer Singapore, 841–851.
- Gargama, H., and S. K. Chaturvedi. 2011. “Criticality Assessment Models for Failure Mode Effects and Criticality Analysis Using Fuzzy Logic.” IEEE Transactions on Reliability 60 (1): 102–110. doi:10.1109/TR.2010.2103672.
- Goh, C. S., H. Abdul-Rahman, and Z. A. Samad. 2013. “Applying Risk Management Workshop for a Public Construction Project: Case Study.” Journal of Construction Engineering and Management 139 (5): 572–580. doi:10.1061/(ASCE)CO.1943-7862.0000599.
- Iqbal, S., R. M. Choudhry, K. Holschemacher, A. Ali, and J. Tamošaitienė. 2015. “Risk Management in Construction Projects.” Technological and Economic Development of Economy 21 (1): 65–78. doi:10.3846/20294913.2014.994582.
- Iuorio, O., L. Fiorino, and R. Landolfo. 2014. “Testing CFS Structures: The New School BFS in Naples.” Thin-Walled Structures 84: 275–288. doi:10.1016/j.tws.2014.06.006.
- Jatheeshan, V., and M. Mahendran. 2015. “Numerical Study of LSF Floors Made of Hollow Flange Channels in Fire.” Journal of Constructional Steel Research 115: 236–251. doi:10.1016/j.jcsr.2015.08.021.
- Jee, T. L., K. M. Tay, and C. P. Lim. 2015. “A New Two-Stage Fuzzy Inference System-Based Approach to Prioritize Failures in Failure Mode and Effect Analysis.” IEEE Transactions on Reliability 64 (3): 869–877. doi:10.1109/TR.2015.2420300.
- Jong, C. H., K. M. Tay, and C. P. Lim. 2013. “Application of the Fuzzy Failure Mode and Effect Analysis Methodology to Edible Bird Nest Processing.” Computers and Electronics in Agriculture 96: 90–108. doi:10.1016/j.compag.2013.04.015.
- Kang, J., L. Sun, H. Sun, and C. Wu. 2017. “Risk Assessment of Floating Offshore Wind Turbine Based on correlation-FMEA.” Ocean Engineering 129: 382–388. doi:10.1016/j.oceaneng.2016.11.048.
- Kerk, Y. W., C. Y. Teh, K. M. Tay, and C. P. Lim. 2021. “Parametric Conditions for a Monotone TSK Fuzzy Inference System to Be an n-Ary Aggregation Function.” IEEE Transactions on Fuzzy Systems 29 (7): 1864–1873.
- Khalifa, Y. A., M. Campidelli, M. J. Tait, and W. W. El-Dakhakhni. 2020. “Mitigating Risk of Confined Explosion via Lightweight Sacrificial Cladding.” Journal of Performance of Constructed Facilities 34 (1): 1. doi:10.1061/(ASCE)CF.1943-5509.0001331.
- Kim, B.-Y., and Y.-S. Kim. 2012. “The Analysis of the Major Cost-increasing Risk Factors from the Perspective of Construction Management-focusing on Pre-construction Phases.” Korean Journal of Construction Engineering and Management 13 (2): 147–155. doi:10.6106/KJCEM.2012.13.2.147.
- Kumru, M., and P. N. Y. L. Z. Kumru. 2013. “Fuzzy FMEA Application to Improve Purchasing Process in a Public Hospital.” Applied Soft Computing 13 (1): 721–733. doi:10.1016/j.asoc.2012.08.007.
- Li, H. X., M. Al-Hussein, Z. Lei, and Z. Ajweh. 2013. “Risk Identification and Assessment of Modular Construction Utilizing Fuzzy Analytic Hierarchy Process (AHP) and Simulation.” Canadian Journal of Civil Engineering 40 (12): 1184-1195.
- Li, X., J. Wang, X. Meng, and J. Wang. 2014. “Comparison and Analysis of Lightweight Steel Structure Residential Housing.„ In International Conference on Mechatronics Control and Electronic Engineering (MCE 2014), pp. 720-721. 2014.
- Liu, H.-C., X.-Q. Chen, C.-Y. Duan, and Y.-M. Wang. 2019. “Failure Mode and Effect Analysis Using Multi-criteria Decision Making Methods: A Systematic Literature Review.” Computers & Industrial Engineering 135: 881–897. doi:10.1016/j.cie.2019.06.055.
- Liu, J., X. Zhao, and P. Yan. 2016. “Risk Paths in International Construction Projects: Case Study from Chinese Contractors.” Journal of Construction Engineering and Management 142 (6): 05016002. doi:10.1061/(ASCE)CO.1943-7862.0001116.
- LSK. 2007. European Lightweight Steel-framed Construction. Sweden: European Light Steel Construction Association.
- Lu, W., K. Chen, F. Xue, and W. Pan. 2018. “Searching for an Optimal Level of Prefabrication in Construction: An Analytical Framework.” Journal of Cleaner Production 201: 236–245. doi:10.1016/j.jclepro.2018.07.319.
- Luo, L.-Z., C. Mao, L.-Y. Shen, and Z.-D. Li. 2015. “Risk Factors Affecting Practitioners’ Attitudes toward the Implementation of an Industrialized Building System: A Case Study from China.” Engineering, Construction and Architectural Management 22 (6): 622–643. doi:10.1108/ECAM-04-2014-0048.
- Ma, G., and M. Wu. 2019. “A Big Data and FMEA-based Construction Quality Risk Evaluation Model considering Project Schedule for Shanghai Apartment Projects.” International Journal of Quality & Reliability Management 37 (1): 18–33. doi:10.1108/IJQRM-11-2018-0318.
- Mahdavinejad, M. J., M. Hajian, and A. Doroodgar. 2012. “Modeling of LSF Technology in Building Design and Construction Case-Study: Parand Residential Complex, Iran.” Advanced Materials Research 341-342: 447–451. doi:10.4028/scientific.net/AMR.341-342.447.
- Mehdizadeh, R., T. Franck, B. Denys, and N. Halidou. 2012. “Methodology and Tools for Risk Evaluation in Construction Projects Using Risk Breakdown Structure.” European Journal of Environmental and Civil Engineering 16 (sup1): s78–s98. doi:10.1080/19648189.2012.681959.
- Meng Tay, K., and C. Peng Lim. 2006. “Fuzzy FMEA with a Guided Rules Reduction System for Prioritization of Failures.” International Journal of Quality & Reliability Management 23 (8): 1047–1066. doi:10.1108/02656710610688202.
- Mustafa, M. A., and J. F. Al-Bahar. 1991. “Project Risk Analytic Assessment Using the Hierarchy Process.” IEEE TRANSACTIONS ON ENGINEERING MANAGEMENT 38 (1): 46–52. doi:10.1109/17.65759.
- Noorzai, E., and M. Golabchi. 2020. “Selecting a Proper Construction System in Small and Medium Mass Housing Projects, considering Success Criteria and Construction Volume and Height.” Journal of Engineering, Design and Technology 18 (4): 883–903. doi:10.1108/JEDT-09-2019-0227.
- Oduyemi, O., M. Okoroh, and O. S. Fajana. 2016. “Risk Assessment Methods for Life Cycle Costing in Buildings.” Sustainable Buildings 1: 3. doi:10.1051/sbuild/2016005.
- Paul, S., G. F. Radavelli, and A. R. Da Silva. 2015. “Experimental Evaluation of Sound Insulation of Light Steel Frame Façades that Use Horizontal Inter-stud Stiffeners and Different Lining Materials.” Building and Environment 94: 829–839. doi:10.1016/j.buildenv.2015.08.010.
- Renuka, S. M., C. Umarani, and S. Kamal. 2014. “A Review on Critical Risk Factors in the Life Cycle of Construction Projects.” Journal of Civil Engineering Research 4 (2): 31-36.
- Saikah, M., N. Kasim, R. Zainal, N. Sarpin, and M. H. I. A. Rahim. 2017. “Potential Implementation of Light Steel Housing System for Affordable Housing Project in Malaysia.” IOP Conference Series: Materials Science and Engineering 271: 012106. doi:10.1088/1757-899X/271/1/012106.
- Sang, A. J., K. M. Tay, C. P. Lim, and S. Nahavandi. 2018. “Application of a Genetic-Fuzzy FMEA to Rainfed Lowland Rice Production in Sarawak: Environmental, Health, and Safety Perspectives.” IEEE Access 6: 74628–74647. doi:10.1109/ACCESS.2018.2883115.
- Santos, P., C. Martins, and L. S. D. Silva. 2014. “Thermal Performance of Lightweight Steel-framed Construction Systems.” Metall. Res. Technol 111(6): 329-338.
- Schafer, B. W. 2011. “Cold-formed Steel Structures around the World.” Steel Construction 4 (3): 141–149. doi:10.1002/stco.201110019.
- Seifi Azad Mard, H. R., A. Estiri, P. Hadadi, and M. Seifi Azad Mard. 2017. “Occupational Risk Assessment in the Construction Industry in Iran.” International Journal of Occupational Safety and Ergonomics 23 (4): 570–577. doi:10.1080/10803548.2016.1264715.
- Seyedhoseini, S. M., S. Noori, and M. A. Hatefi. 2009. “An Integrated Methodology for Assessment and Selection of the Project Risk Response Actions.” Risk Analysis : An Official Publication of the Society for Risk Analysis 29 (5): 752–763. doi:10.1111/j.1539-6924.2008.01187.x.
- Shi, S., and J. Yu. 2009. “Development of Chinese Light Steel Construction Residential Buildings.” Journal of Sustainable Development 2 (3). doi:10.5539/jsd.v2n3p134.
- Soares, N., A. R. Gaspar, P. Santos, and J. J. Costa. 2014. “Multi-dimensional Optimization of the Incorporation of PCM-drywalls in Lightweight Steel-framed Residential Buildings in Different Climates.” Energy and Buildings 70: 411–421. doi:10.1016/j.enbuild.2013.11.072.
- Soares, N., P. Santos, H. Gervásio, J. J. Costa, and L. Simões da Silva. 2017. “Energy Efficiency and Thermal Performance of Lightweight Steel-framed (LSF) Construction: A Review.” Renewable and Sustainable Energy Reviews 78: 194–209. doi:10.1016/j.rser.2017.04.066.
- Steau, E., and M. Mahendran. 2020. “Thermal Modelling of LSF Floor-ceiling Systems with Varying Configurations.” Fire Safety Journal 118: 103227. doi:10.1016/j.firesaf.2020.103227.
- Trevathan, J. W., and J. R. Pearse. 2008. “The Effect of Workmanship on the Transmission of Airborne Sound through Light Framed Walls.” Applied Acoustics 69 (2): 127–131. doi:10.1016/j.apacoust.2006.09.001.
- Veljkovic, M., and B. Johansson. 2006. “Light Steel Framing for Residential Buildings.” Thin-Walled Structures 44 (12): 1272–1279. doi:10.1016/j.tws.2007.01.006.
- Wang, S. Q., M. F. Dulaimi, and M. Y. Aguria. 2004. “Risk Management Framework for Construction Projects in Developing Countries.” Construction Management and Economics 22 (3): 237–252. doi:10.1080/0144619032000124689.
- Wang, T., S. Gao, X. Li, and X. Ning. 2018. “A Meta-network-based Risk Evaluation and Control Method for Industrialized Building Construction Projects.” Journal of Cleaner Production 205: 552–564. doi:10.1016/j.jclepro.2018.09.127.
- Wang, X., and T. C. Hutchinson. 2020. “Evolution of Modal Characteristics of a Mid-rise Cold-formed Steel Building during Construction and Earthquake Testing.” Earthquake Engineering & Structural Dynamics 49 (14): 1539–1558. doi:10.1002/eqe.3316.
- Wu, D., J. Li, T. Xia, C. Bao, Y. Zhao, and Q. Dai. 2018. “A Multiobjective Optimization Method considering Process Risk Correlation for Project Risk Response Planning.” Information Sciences 467: 282–295. doi:10.1016/j.ins.2018.07.013.
- Wu, P., Y. Xu, R. Jin, Q. Lu, D. Madgwick, and C. M. Hancock. 2019. “Perceptions Towards Risks Involved in Off-site Construction in the Integrated Design & Construction Project Delivery.” Journal of Cleaner Production 213: 899–914. doi:10.1016/j.jclepro.2018.12.226.
- Xiahou, X., J. Yuan, Y. Liu, Y. Tang, and Q. Li. 2018. “Exploring the Driving Factors of Construction Industrialization Development in China.” Int J Environ Res Public Health 15(3): 442.
- Yeh, T.-M., and L.-Y. Chen. 2014. “Fuzzy-based Risk Priority Number in FMEA for Semiconductor Wafer Processes.” International Journal of Production Research 52 (2): 539–549. doi:10.1080/00207543.2013.837984.
- Yu, C. 2016. Recent Trends in Cold-Formed Steel Construction. Elsevier Science.
- Yuan, Z., G. Ni, L. Wang, Y. Qiao, C. Sun, N. Xu, and W. Wang. 2020. “Research on the Barrier Analysis and Strength Measurement of a Prefabricated Building Design.” Sustainability 12 (7): 2994. doi:10.3390/su12072994.
- Zeynalian, M., B. Trigunarsyah, and H. R. Ronagh. 2013. “Modification of Advanced Programmatic Risk Analysis and Management Model for the Whole Project Life Cycle’s Risks.” Journal of Construction Engineering and Management 139 (1): 51–59. doi:10.1061/(ASCE)CO.1943-7862.0000571.
- Zou, P. X. W., and G. Zhang. 2014. “Managing Risks in Construction Projects: Life Cycle and Stakeholder Perspectives.” International Journal of Construction Management 9 (1): 61–77. doi:10.1080/15623599.2009.10773122.
- Zou, P. X. W., G. Zhang, and J. Wang. 2007. “Understanding the Key Risks in Construction Projects in China.” International Journal of Project Management 25 (6): 601–614. doi:10.1016/j.ijproman.2007.03.001.