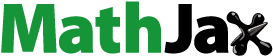
ABSTRACT
There have been repeated security incidents in megaproject tunnels, construction security issues are particularly serious, and the management of security risks is receiving increasing attention. This study was conducted to improve the efficiency of tunnel security risk management, the security risk management process was adopted as an entry point, based on the Observe-Orient-Decision-Action (OODA) model, the influencing factors index system for the efficiency of security risk management in megaproject tunnels from four dimensions: observe, orient, decision and action. The AHP-EWM method is applied to assign index weights to model the system dynamics of security risk management efficiency. The Vensim software was used to simulate and analyse the effect of different indices on the efficiency of security risk management under different conditions, which provides a reference for improving the efficiency of security risk management. The results of the study show that building a comprehensive and uninterrupted risk information awareness network, real-time adaptation of risk control strategies based on the complexity of risks, and shortening risk management delays can effectively increase the efficiency of dynamic security risk management. This provides a theoretical and practical reference for effectively improving the efficiency of risk management for tunnel security in megaproject construction.
1. Introduction
China has the largest number of tunnels, the fastest development rate, and the most complex geological and structural forms in the world (Lu and Cai Citation2019). While continuously accelerating the modernization of the transportation system, the development trend of large scale, complex geology and high difficulty of tunnel construction has led to an increasingly serious security situation (Jaafari Citation2003). The length, depth and cross-sectional size of tunnels are increasing, and more and more tunnels are built at high altitude, strong sand, high temperature and cold environment or high stress, strong karst area, resulting in complex and variable geological conditions, which are difficult to predict accurately (Xun, Zhang, and Yuan Citation2022). Adverse geology such as water and mud surges, large deformations in soft rock, rock bursts, gas and high ground temperatures lead to variable and uncertain security risks in tunnel construction. As a result, the environmental conditions faced by workers during tunnel construction are complex (Ma, Bin Luo, and Chen Citation2013). Given the complexity of tunnelling, there is a relatively high probability of security accidents during the construction phase of tunnelling, resulting in greater economic and human losses and a large negative impact on society.
A summary of the causes of tunnel security accidents shows that for tunnel construction security risks come from two main sources. Firstly, the complexity of the geological environment faced by the tunnel during the actual construction process (Chatzimichailidou and Dokas Citation2016; Reza et al. Citation2019). Secondly, human or subjective causes, mainly due to the increasing size of the tunnels and the management deficiencies of the construction units, which do not pay attention to security management in the actual construction management process (Liu et al. Citation2022). Although many scientific and reasonable security contingency plans have been proposed, they are mainly designed to deal with unexpected events in tunnel construction, and do not provide real-time monitoring and prevention of daily security risks, so the usual tunnel construction security risk management link is relatively weak (Liu et al. Citation2018; Sina et al. Citation2022). As a result, the increasingly challenging tunnel security situation and management weaknesses place greater demands on the efficiency of risk management.
However, tunnel construction is affected by multiple factors, both in the megaproject itself and in the external environment, and the efficiency of security risk management is fraught with uncertainties. A comprehensive, systematic and in-depth analysis and study of the efficiency of security risk management is an important practical guide to improve the level of security risk management in megaproject tunnels. Tunnel security risk management is a dynamic evolutionary process. System dynamics (SD), which starts from the microstructure of the system and simulates and analyses the dynamic behaviour of the system, provides methodological support for studying dynamic, complex multivariate nonlinear systems. Therefore, this study constructs an indexing system of influence factors for the efficiency of tunnel security risk management. Based on SD, an efficiency model for tunnel security risk management model is established, which provides a quantitative and scientific decision-making basis for studying how to improve the efficiency of tunnel security risk management for megaprojects.
The remainder of the paper is organized as follows. Section 2 reviews the relevant literature. Section 3 identifies the factors that affect the efficiency of security risk management. Section 4 constructs the SD model for the efficiency of tunnel security risk management. Section 5 performs empirical simulations and analysis. Section 6 provides a discussion based on the model results. Finally, the concluding remarks are provided in Section 7.
2. Literature review
2.1. Tunnel security risk identification
Security risk identification is a prerequisite for risk management (Cha and Shin Citation2012). On the one hand, risk identification can be judged by perception and historical experience. On the other hand, it can also be analysed, summarized and organized by various objective data and records of risk incidents, as well as necessary expert visits, in order to find out various obvious and potential risks and their loss patterns (Konstantinos and Konstantinos Citation2013). Nezarat, Sereshki, and Ataei (Citation2015) summarized eight categories of tunnel construction security risks through geological studies and interviews with experienced technicians. Zhou et al. (Citation2021) proposed a framework for automatic identification and classification of security risks in the metro design phase based on Building Information Modeling (BIM), which facilitates the digital transfer of security risk design information throughout the construction process. Lu et al. (Citation2020) developed a multi-agent based Security Computing Experimental System (SCES) to provide comprehensive risk factor identification for shield tunnel projects. Forcael et al. (Citation2018) identified the main risk factors that threaten the achievement of the tunnel construction project objectives and consider them to be inherent to the tunnel construction project. Chen et al. (Citation2020) extracted incident chains from tunnel security incidents and designed network-level performance metrics and node-level critical security risk identification metrics. Zhang et al. (Citation2016) integrated Building Information Modeling (BIM) and expert systems to propose a new tunnel security risk identification method to address the shortcomings of traditional identification methods. Ye et al. (Citation2022) propose a digital twin-based multi-information intelligent warning and security management platform where multi-source security risk information of tunnel construction can be automatically collected and transmitted. Dong et al. (Citation2018) developed a Knowledge-dynamics Integrated Map (KIM) that visualizes the identified tunnel construction security risks to form a knowledge flow for security risk management.
2.2. Tunnel security risk assessment
Security risk assessment is a quantitative measurement of the likely level of impact or loss from a risk event. Qiu et al. (Citation2020) proposed a comprehensive assessment method for gas tunnel construction security risk based on fuzzy Bayesian network, which determines the results of risk assessment in terms of both risk probability and risk hazard. Li and Qu (Citation2016) designed a two-segment search and constraint algorithm (BSSBA) to obtain the optimal solution for tunnel security facility selection. Han et al. (Citation2018) used Monte Carlo simulation techniques to quantitatively estimate the potential level of security risk for circumferential cracks in pre-existing shield tunnels. Ntzeremes, Kirytopoulos, and Filiou (Citation2020) proposed an evacuation simulation model to improve the efficiency of quantitative risk assessment, which can help security analysts to make more informed decisions about the security level of the tunnel. Hu et al. (Citation2021) developed a tunnel construction security risk assessment model for LSDDTPs based on the material element expansion method and validated it with the East Lake Deep Buried Tunnel project in Wuhan, China as a case study. Guo and Zhang (Citation2021) utilized D-S evidence theory to fuse information and determine tunnel construction security risk probabilities, and used evidence updating methods to capture the dynamic characteristics of risk states. Xun, Zhang, and Yuan (Citation2022) proposed a security risk assessment method based on Building Information Modeling (BIM), Intuitionistic Fuzzy Set (IFS) theory and Dempster-Shafer (D-S) evidence theory, which can perform dynamic security risk assessment for cross-harbour tunnel construction projects under uncertainty, ambiguity, and conflict environments.
2.3. Tunnel security risk decision-making
Security risk decision is the choice of more than two courses of action under the action of multiple uncertainties, and because of the existence of uncertainties, the result of the implementation of the course of action is not predetermined the value of profit and loss (Eirik, Willy, and Ruben Citation2012). When security risk incidents occur, they require rapid response and decision making by tunnel construction and management personnel (Kinateder et al. Citation2014; Reza, Sina, and Zoheir Citation2019). Uncertainty is one of the sources of security risk in tunnelling projects. Uncertainty information not only affects the accuracy of security risk evaluation results, but also affects the reliability of the decision-making scheme (Xia et al. Citation2018). In order to solve the problem of cognitive uncertainty caused by insufficient use of information in decision making, Guo et al. (Citation2022) proposed a continuous-time Bayesian network (CTBN) technology-based decision support method for subway tunnel construction security risks. Cai, Hu, and Ma (Citation2021) argued that the information fusion approach based on extension theory (ET) performs an effective tool for uncertain information reasoning to address the ambiguity and uncertainty of security risk decisions. Zhang and Chen (Citation2022) used TOPSIS to determine the ranking of various alternative tunnel security risk decision options to deal with multi-criteria decision problems under uncertainty.
2.4. Tunnel security risk control
Security risk control refers to the measures and methods adopted by risk managers to reduce the damage caused by security risk events (Konstantinos et al. Citation2010). Zhang et al. (Citation2013) extended the security control process to the entire lifecycle of the model application that is prone to security risk events, including continuous control during construction and post-incident control. Bjelland et al. (Citation2021) considered that the system theory approach is suitable to deal with the complexity of tunnel systems and facilitate the control of tunnel construction security risks. Li and Yuan (Citation2016) developed a security risk control framework for shield tunnel construction to address and manage security risks in a systematic way. Lei et al. (Citation2020) analysed the idea of security risk control for shield tunnel construction under existing railroads and formed a complete set of security risk control processes. Fang, Zhang, and Wong (Citation2011) proposed a risk management approach to achieve control of tunnel construction security risks through process control of ground settlement and nearby existing structures. Li et al. (Citation2017) established a sudden water surge mud risk evaluation of unsure measures model to effectively control the tunnel construction security risks caused by sudden water surge mud. Pan et al. (Citation2019) quantitatively analysed the coupling degree between the factors in the tunnel construction security risk system based on the coupling degree theory in physics, so as to achieve the control of the whole security risk system.
3. Influencing factors of efficiency of security risk management
The efficiency of tunnel security risk management for megaprojects is the extent to which construction and management personnel can achieve the desired goals of risk prevention and control by performing risk management tasks during the tunnel construction process (Liu et al. Citation2021). However, in order to enhance the efficiency of risk management, based on the basic structure of observation-orient-decision-action of the OODA cycle theory, as shown in , and combined with risk management-related approaches and relevant literature, an index system of influencing factors for the efficiency of security risk management in megaproject tunnels was constructed, as shown in .
Table 1. Influencing factors of efficiency of tunnel security risk management.
4. SD model of efficiency of tunnel security risk management
4.1. SD method and steps
The study of system dynamics focuses on the behavioural patterns and characteristics of the system. Based on the internal information feedback control of the system, computer techniques are used to seek the dynamic correlations between the system structure and behaviour patterns with the help of model simulation analysis. The system dynamics model consists mainly composed of system variables, including state variables, rate variables, auxiliary variables, and constants. The causal relations and feedback mechanisms between the variables are determined by constructing equations. The construction of the SD model is an important component to complete the analysis of the security risk management in the tunnel of the megaproject. The main specific steps are shown in , including (1) clarify the purpose of modeling. (2) define the system boundary. (3) determine the feedback relationship. (4) design the equations of variables. (5) run the model simulation.
4.2. Causal relationship of influencing factors
Tunneling is a dynamic and open system in the security risk management process with multiple input and output variables. There are dynamical features such as mutual transmission, derivation, and complexity among the variables. When the value of the security risk management variables is not sufficient to meet the base requirements, new risks may be created, which may be passed on to other risk factors or reduce the level of tunnel security risk management. For example, the geology of the tunnel was surveyed using advance geological predictions. The quality of the geological surveys varies considerably between different skill levels of staff. If their technical level is not rigorously managed, it can easily lead to inaccurate tunnel geology, making it difficult to reveal the unknown geological risk susceptibilities. As a result, the security level of tunnel construction will be reduced and security risk accidents will be induced.
According to the causal circuit and accident causation theory, the factors affecting the efficiency of security risk management in the megaproject tunnel are considered comprehensively, and a causal loop diagram of efficiency of security risk management is constructed based on the causal relationship between each factor, as shown in . The complexity of security risk is negative feedback, which has a negative impact on the efficiency metrics of security risk management. The higher the complexity of security risk, the worse the efficiency of security risk management. Moreover, all the other feedbacks in are positive feedbacks, which play a positive role in the efficiency of security risk management.
Figure 3. Causal loop diagram of efficiency of security risk management.
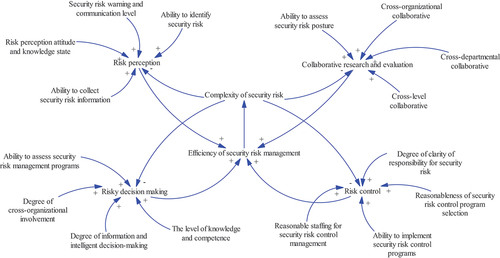
In the causal loop diagram of the efficiency of tunneling security risk management, the efficiency of security risk management is used as the horizontal variable. The efficiency of tunneling security risk management is generated by the joint action and interaction coupling of multiple factors. Therefore, a causal loop diagram of system dynamics is used to graphically describe the complex dynamical correlations of a dynamic security risk management system. With the help of Vensim software, the feedback loop for the efficiency of security risk management is analysed. ”+” indicates positive feedback and “-” indicates negative feedback. Based on the SD theory, the influencing factors in the OODA cycle, including risk perception, collaborative research and evaluation, risk decision making, and risk control, have a positive feedback loop that modulates the efficiency on efficiency of security risk management.
4.3. SD flow mapping
The tunnel security risk management system consists of a combination of four subsystems such as risk perception, collaborative research and evaluation, risk decision making, and risk control in the OODA loop. Based on the causal loop graph of efficiency of security risk management, the SD flow graph of efficiency of security risk management is plotted with the help of Vensim software, as shown in .
4.4. Construction of SD equation
Based on the influencing factors and system dynamics flow diagram of tunnel security risk management efficiency, the weights of the factors were selected as the variable coefficients to establish SD equations for the system dynamics model of security risk management efficiency, and the SD equations of the main variables are shown in . The weights of the four subsystems of risk perception, collaborative research and evaluation, risk decision making, and risk control are WA, WB, WC, and WD. The factor weights of the risk perception subsystem are WA1, WA2, WA3, and WA4. The factor weights for the collaborative research and evaluation subsystems are WB1, WB2, WB3, and WB4. The factor weights of the risk decision making subsystems are WC1, WC2, WC3, and WC4. The factor weights of the risk control subsystems are WD1, WD2, WD3, and WD4.
Table 2. SD equations of security risk management efficiency.
5. Empirical simulation and analysis
5.1. Analysis of indicator weights
The SD method is used to analyse the efficiency of tunnel security risk management, which requires the assignment of indicator weights. In order to reduce the limitations of the single assignment method, the hierarchical entropy weight method (hierarchical analysis method – entropy weight method) is invoked in the process of determining the weights. A combination of subjective and objective evaluations is used to modify the weights, which makes the weights of each indicator more reliable.
5.1.1. Analytic hierarchy process method
The AHP (Analytic hierarchy process) method, first proposed by American operations researcher Saaty in the 1970s, is a method of qualitative and quantitative analysis for decision making, which has the advantages of being simple, systematic and flexible. The AHP method is commonly used to solve complex multi-objective decision problems, and is also widely used to determine the weights of evaluation metrics. The specific calculational steps of the AHP method are as follows.
(1) Construct the judgment matrix. Through expert consultation and the application of 9 scaling method, a judgment matrix U is constructed based on a two-by-two comparison of the relative importance of indicators in the same tier relative to the indicators in the upper tier, where Uij indicates the comparison result of the ith indicator relative to the jth indicator.
(2) The weights of each indicator ωi were calculated. the weights of the indicators were determined by using the geometric mean method.
(3) Calculate the maximum characteristic root of matrix U.
(4) Calculate consistency metrics.
(5) Determine the consistency of the matrix.
The specific values of RI are shown in , and when CR < 0.1, the matrix consistency test is considered to be passed, while the opposite needs to be corrected.
Table 3. The RI reference value.
5.1.2. Entropy weight method
Entropy theory reflects the degree of information disorder, and the more information a certain indicator carries, the more influence it has on decision making. In general, the larger the dispersion of evaluation metrics, the larger the amount of information and weight. On the contrary, the smaller the dispersion of the evaluation index, the smaller the amount of information and weight. Due to the different magnitudes of each evaluation metric, the data needs to be standardized in order to achieve comparability between the data. The specific calculation procedure of the entropic weight method is as follows.
(1) Normalize the column vectors of the judgment matrix.
(2) Calculate the index entropy value.
(3) Calculate the information entropy redundancy.
(4) Calculate the entropy weight of each indicator.
(5) Calculate the modified indicator weights.
5.1.3. Indicator weights based on AHP-EWM
Based on the entropic hierarchical analysis method, the subjective index weights are determined by the hierarchical analysis method and then the objective index weights are modified by the entropic weight method.
In this paper, a number of experts, including senior engineers of megaprojects and professors of engineering security risk management, were invited to set up an expert scoring group. After consultation and discussion, the judgment matrix for the indicators in this paper was finally determined based on the scale of the explanation matrix, as shown in .
Table 4. Weighted decision matrix.
After the computation of the AHP steps, the weights of each indicator can be obtained.
= {0.063, 0.182, 0.058, 0.131, 0.051, 0.109, 0.071, 0.029, 0.025, 0.083, 0.051, 0.031, 0.019, 0.049, 0.030, 0.016}. CI = 0.142, CR = 0.088 < 0.1, therefore, the consistency test of the judgment matrix passes. The weights uj for each indicator can be obtained after computing the steps of the EWM. uj = {0.080, 0.052, 0.053, 0.072, 0.064, 0.070, 0.097, 0.054, 0.056, 0.075, 0.071, 0.006, 0.036, 0.069, 0.053, 0.038}.
Thus, the indicator weights qi based on AHP-EWM can be calculated. qi = {0.076, 0.144, 0.047, 0.143, 0.050, 0.116, 0.105, 0.024, 0.021, 0.095, 0.055, 0.029, 0.011, 0.050, 0.024, 0.010}. The corresponding weights of each factor for risk perception, collaborative research and evaluation, risk decision making, and risk control are (WA, WB, WC, WD) = (0.410, 0.295, 0.200, 0.095). The weight values of the secondary indicators for each subsystem are given in . Finally, the combined weight of security risk complexity and security risk management efficiency is 0.167 and 0.833 after expert analysis.
Table 5. Results of indicator combination weights.
The factor weights of risk perception subsystem are (WA1, WA2, WA3, WA4) = (0.186,0.351,0.114,0.349), which suggests that security risk warning and communication level, ability to collect security risk information are the main factors influencing risk perception. The factor weights of collaborative research and evaluation subsystem are (WB1, WB2, WB3, WB4) = (0.170, 0.393, 0.357, 0.080), which suggests that cross-organizational collaborative security risk research and evaluation ability, cross-departmental collaborative security risk research and evaluation ability are the main factors influencing collaborative research and evaluation. The factor weights of risk decision making subsystem are (WC1, WC2, WC3, WC4) = (0.107, 0.474, 0.276, 0.112), which suggests that degree of cross-organizational involvement in risk decision making, degree of information and intelligent decision-making for security risks are the main factors influencing risk decision making. The factor weights of risk control subsystem are (WD1, WD2, WD3, WD4) = (0.112, 0.533, 0.256, 0.099), which suggests that reasonableness of security risk control program selection, ability to implement security risk control programs are the main factors influencing risk control.
5.2. Simulation experimental design
For the purpose of exploring the impact of different metrics on the efficiency of security risk management in megaproject tunneling under different conditions. The experimental design of SD simulations for the efficiency of management of security risk in megaproject tunneling is shown in . Three simulation experiments are set up in this study. The main purpose of experiment a is to investigate the variation of different metrics and observe the overall variation in the complexity and efficiency of security risk management. The main purpose of experiment b is to observe the impact of risk perception on the efficiency of security risk management. The main purpose of experiment c is to analyse the impact of time delay on the efficiency of security risk management.
Table 6. Experimental design of SD simulation.
5.3. Model simulation experiments
Three representative model simulation experiments were conducted with simulation time T = 8 and simulation step size of 0.5 in hours. The simulations were performed by using the Vensim software. Vensim is a visual modeling tool that allows you to conceptualize, document, simulate, analyse, and optimize models of dynamic systems. Vensim provides a simple and flexible way to build simulation models from causal loops or stock and flow graphs.
5.3.1. Experiment a
The simulation of the Experiment a (1) model is shown in , where risk perception rises faster than collaborative research and evaluation, risk decision making, risk control. This indicates that risk perception has the greatest impact on the efficiency of security risk management, followed by collaborative research and evaluation and risk control with the least impact. The simulation of the Experiment a (2) model is shown in , complexity of security risk and efficiency of security risk management show a clear antagonistic trend. Security risk management activities have continued to advance over time. The efficiency of security risk management continues to grow, and the complexity of security risks is gradually being degraded and controlled.
5.3.2. Experiment b
The simulation of the model of Experiment b is shown in . As the initial value of risk perception increases, the upward trend of security risk management efficiency gradually increases, and correspondingly, the downward trend of security risk complexity significantly increases. Therefore, it can be seen that risk perception is a prerequisite for the implementation of risk management in the process of security risk management in megaproject tunnels. When the level of risk perception is high, the efficiency of security risk management increases rapidly as the level of risk management is improved.
5.3.3. Experiment c
The simulation of the model of Experiment c is shown in , where the simulation results with delays of 1, 2 and 3 h are compared and analysed. The results show that the larger the delay time, the slower and worse the rise in the efficiency of security risk management, and the faster and more complex the decrease in the complexity of security risk management. This indicates that when risk perception, collaborative research and evaluation, risk decision making, and risk control are inactive, security risk complexity is less affected by risk management behaviour and becomes progressively more complex.
6. Discussion
6.1. Strategies to improve the efficiency of security risk management
In the process of managing of security risks in megaproject tunnels, the complexity of security risks should be controlled in real time, and the perception, collaborative research, decision making and control of security risks should be comprehensively improved by construction managers and agencies. Real-time collection of construction site security risk information and enhanced communication and exchange between construction personnel and management regarding security risks. Form a real-time, comprehensive and uninterrupted security risk information perception network so that the acquisition, transmission, processing and sharing of security risk information form an efficient circuit. Based on the risk information, the participants and departments are organized to conduct collaborative research and assessment of security risks. Participants worked together to use information technology to make decisions about security risks and to select a reasonable security risk control program to implement risk control.
6.2. Risk perception is key influencing factor
Risk perception is a prerequisite and key element in the management of security risks, according to the weighting of the influencing factors in the management of security risks in megaproject tunnels. Risk perception, as a cognitive factor for tunneling construction and management agents, plays a leading role in the management behaviour of security risks. The core component of risk perception is the perception and awareness of external risks by individuals or groups, which emphasizes the experience gained by influencing the subjective judgment and feelings of individuals. Megaproject tunnel builders work with a large number of security risks, and the higher their level of risk perception, the more aware they will be of the possibility of being injured on the job. Therefore, the level of security risk warning and communication, and the ability to collect security risk information need to be focused on improving to adopt a higher level of security behaviour to meet security needs.
6.3. Reduce delays in management of security risks
Reducing delays in the managing of security risks is an effective measure to improve management efficiency. Participants can maintain close cooperation in security risk perception, research and evaluation, decision making and control. When security risks are found to be hidden, strategies are adopted to control them in a timely manner. The response of project construction and management personnel to security risks should be improved to reduce the delay in security risk management, which can improve the efficiency of security risk management. Moreover, the collaboration of multiple entities and sectors involved in megaproject tunneling can overcome the subjective and objective factors that cause time delays and effectively shorten the dynamic delay time of security risk.
The limitation of this study is that the research method is not applied to the actual project to be tested, but rather the law of influence of its indicator factors on the efficiency of tunnel security risk management is explored by means of simulation tests. In future research, the authors will focus on applying the research results to real megaprojects. This will enhance the objectivity of the indicator data, propose more detailed measures to improve the efficiency of tunnel security risk management, and increase the practicality of the model approach.
7. Conclusion
The analysis of the efficiency of security risk management in megaproject tunneling is an analysis of evolution of complex risk management processes. Based on the OODA model, this study analyzes that affect the efficiency of security risk management in megaproject tunnels in four aspects: observe, orient, decision and action. The SD method is used to construct a system dynamics model of the efficiency of security risk management in megaproject tunneling. Moreover, the influence factors on the efficiency of security risk management are assigned weights based on the AHP-EWM method, and the complex functional relationships of the system dynamics model are dynamically simulated. The conclusions are as follows.
The corresponding weights of each factor for risk perception, collaborative research and evaluation, risk decision making, and risk control are 0.410, 0.295, 0.200, 0.095. They are important influencing factors for the efficiency of managing security risks in megaproject tunnels. Risk perception is not only an important prerequisite for the implementation of dynamic security risk management activities, but also the most critical influencing factor.
Security risk warning and communication level (0.351), ability to collect security risk information (0.349) are the main factors influencing risk perception. Cross-organizational (0.393) and cross-departmental (0.357) collaborative security risk research and evaluation ability are the main factors influencing collaborative research and evaluation. Degree of cross-organizational (0.474) and degree of intelligent decision making (0.276) are the main factors influencing risk decision making. Reasonableness of security risk control program selection (0.533) and ability to implement security risk control programs (0.256) are the main factors influencing risk control.
Risk perception, collaborative research and evaluation, risk decision making, risk control can reduce the complexity of security incidents in megaproject tunnels. Real-time adjustment of risk control strategies based on the complexity of security risks can improve the efficiency of dynamic security risk management.
If managing security risks is delayed, it is difficult to degrade the complexity of the risks in a timely manner, which can reduce the efficiency of dynamic security risk management. Therefore, reducing the delay can effectively improve the efficiency of security risk management.
Disclosure statement
No potential conflict of interest was reported by the authors.
Additional information
Funding
Notes on contributors
Kai Liu
Kai Liu is a PhD student in the School of Economics and Management at Beijing Jiaotong University. He received his Master of Engineering degree from the School of Civil Engineering and Architecture of Shandong University of Science and Technology in Qingdao, Shandong Province, China. His research focuses on project governance and project management. He can be reached at [email protected].
Yuming Liu
Yuming Liu is a professor in the School of Economics and Management at Beijing Jiaotong University. He received his Ph.D. in Management from Beijing Jiaotong University and his M.S. in Engineering from Hunan University. Liu has published several articles in journals, including Energy Conversion and Management and Energy and Buildings. He is a reviewer for Energy and Buildings, Journal of Cleaner Production, Energy Efficiency, and Building and Environment. He can be reached at [email protected].
Yuanyuan Kou
Yuanyuan Kou is a Ph.D. scholar in the College of Economics and Management, Shandong University of Science and Technology. She completed her Master’s program of Management Science and Engineering from Shandong University of Science and Technology. Her areas of interest include project management, engineering technical management. She can be reached at [email protected].
Xiaoxu Yang
Xiaoxu Yang is a PhD student in the School of Economics and Management at Beijing Jiaotong University. He completed his master’s degree in civil engineering at the School of Civil Engineering and Construction of Beijing Jiaotong University. His research interests are project management and tunnel construction management. He can be reached at [email protected].
Guangzhong Hu
Guangzhong Hu is a master’s student at the School of Economics and Management, Beijing Jiaotong University. He completed his undergraduate program at Harbin Engineering University. His research interests are project management and project governance. He can be reached at [email protected].
References
- Bjelland, H., O. Nja, A. W. Heskestad, and G. S. Braut. 2021. “Emergency Preparedness for Tunnel Fires - a Systems-Oriented Approach.” Security Science 143:105408. https://doi.org/10.1016/j.ssci.2021.105408.
- Cai, Q. J., Q. J. Hu, and G. L. Ma. 2021. “Improved Hybrid Reasoning Approach to Security Risk Perception Under Uncertainty for Mountain Tunnel Construction.” Journal of Construction Engineering and Management 147 (9): 04021105. https://doi.org/10.1061/(ASCE)CO.1943-7862.0002128.
- Cha, H. S., and K. Y. Shin. 2012. “Predicting Project Cost Performance Level by Assessing Risk Factors of Building Construction in South Korea.” Journal of Asian Architecture and Building Engineering 10 (2): 437–444. https://doi.org/10.3130/jaabe.10.437.
- Chatzimichailidou, M. M., and I. M. Dokas. 2016. “RiskSoap: Introducing and Applying a Methodology of Risk Self-Awareness in Road Tunnel Security.” Accident Analysis and Prevention 90:118–127. https://doi.org/10.1016/j.aap.2016.02.005.
- Chen, F. Y., H. W. Wang, G. Y. Xu, H. C. Ji, S. L. Ding, and Y. C. Wei. 2020. “Data-driven security enhancing strategies for risk networks in construction engineering.” Reliability Engineering & System Security 197:106806. https://doi.org/10.1016/j.ress.2020.106806.
- Dong, C., F. Wang, H. Li, L. Y. Ding, and H. B. Luo. 2018. “Knowledge Dynamics-Integrated Map as a Blueprint for System Development: Applications to Security Risk Management in Wuhan Metro Project.” Automation in Construction 93:112–122. https://doi.org/10.1016/j.autcon.2018.05.014.
- Eirik, B. A., R. Willy, and J. Ruben. 2012. “A Practical Approach for the Evaluation of Acceptable Risk in Road Tunnels.” Journal of Risk Research 16 (5): 625–633. https://doi.org/10.1080/13669877.2012.761268.
- Fang, Q., D. L. Zhang, and L. N. Y. Wong. 2011. “Environmental Risk Management for a Cross Interchange Subway Station Construction in China.” Tunnelling and Underground Space Technology 26 (6): 750–763. https://doi.org/10.1016/j.tust.2011.05.003.
- Forcael, E., H. Morales, D. Agdas, C. Rodriguez, and C. Leon. 2018. “Risk Identification in the Chilean Tunneling Industry.” Engineering Management Journal 30 (3): 203–215. https://doi.org/10.1080/10429247.2018.1484266.
- Guo, K., and L. M. Zhang. 2021. “Multi-Source Information Fusion for Security Risk Assessment in Underground Tunnels.” Knowledge-Based Systems 227:227. https://doi.org/10.1016/j.knosys.2021.107210.
- Guo, Y. F., J. J. Zheng, R. J. Zhang, and Y. B. Yang. 2022. “An Evidence-Based Risk Decision Support Approach for Metro Tunnel Construction.” Journal of Civil Engineering and Management 28 (5): 377–396. https://doi.org/10.3846/jcem.2022.16807.
- Han, J. Y., W. Zhao, P. J. Jia, Y. P. Guan, Y. Chen, and B. F. Jiang. 2018. “Risk Analysis of the Opening of Shield-Tunnel Circumferential Joints Induced by Adjacent Deep Excavation.” Journal of Performance of Constructed Facilities 32 (1): 04017123. https://doi.org/10.1061/(ASCE)CF.1943-5509.0001122.
- Hu, K., J. W. Wang, H. Wu, and S. Yousefi. 2021. “Construction Security Risk Assessment of Large-Sized Deep Drainage Tunnel Projects.” Mathematical Problems in Engineering 2021:1–15. https://doi.org/10.1155/2021/7380555.
- Jaafari, A. 2003. “Project Management in the Age of Complexity and Change.” Project Management Journal 34 (4): 47–57. https://doi.org/10.1177/875697280303400407.
- Kinateder, M., M. Muller, P. Pauli, A. Mühlberger, and P. Pauli. 2014. “Social Influence in a Virtual Tunnel Fire – Influence of Conflicting Information on Evacuation Behavior.” Applied Ergonomics 45 (6): 1649–1659. https://doi.org/10.1016/j.apergo.2014.05.014.
- Konstantinos, A. K., A. R. Athanasios, P. T. Ilias, and P. George. 2010. “Quantitative Risk Analysis for Road Tunnels Complying with EU Regulations.” Journal of Risk Research 13 (8): 1027–1041. https://doi.org/10.1080/13669877.2010.494331.
- Konstantinos, K., and K. Konstantinos. 2013. “Challenges for Current Quantitative Risk Assessment (QRA) Models to Describe Explicitly the Road Tunnel Security Level.” Journal of Risk Research 17 (8): 953–968. https://doi.org/10.1080/13669877.2013.822916.
- Lei, M. F., D. Y. Lin, Q. Y. Huang, C. H. Shi, and L. C. Huang. 2020. “Research on the Construction Risk Control Technology of Shield Tunnel Underneath an Operational Railway in Sand Pebble Formation: A Case Study.” European Journal of Environmental and Civil Engineering 24 (10): 1–15. https://doi.org/10.1080/19648189.2018.1475305.
- Li, P., and X. B. Qu. 2016. “Optimal Allocation of Tunnel Security Provisions Based on a Quantitative Risk Assessment Model.” Scientific Programming 2016:1–5. https://doi.org/10.1155/2016/9628095.
- Liu, K., Y. M. Liu, X. Zhao, Y. Y. Kou, and F. M. Mahomed. 2022. “Study on Design Optimization in Major Railway Project with Fairness Preference Based on Stackelberg Game.” Mathematical Problems in Engineering 2022:1–10. https://doi.org/10.1155/2022/5336591.
- Liu, K., Y. F. Liu, H. M. Zhou, Y. Y. Kou, Q. Ji, and D. Li. 2021. “Evolutionary Game and Numerical Simulation of participants’ Collaborative Behavior in Integrated Project Delivery Project.” Alexandria Engineering Journal 60 (1): 373–385. https://doi.org/10.1016/j.aej.2020.08.038.
- Liu, W., T. S. Zhao, W. Zhou, and J. J. Tang. 2018. “Security Risk Factors of Metro Tunnel Construction in China: An Integrated Study with EFA and SEM.” Security Science 105:98–113. https://doi.org/10.1016/j.ssci.2018.01.009.
- Li, S. C., J. Wu, Z. H. Xu, and L. P. Li. 2017. “Unascertained Measure Model of Water and Mud Inrush Risk Evaluation in Karst Tunnels and Its Engineering Application.” KSCE Journal of Civil Engineering 21 (4): 1170–1182. https://doi.org/10.1007/s12205-016-1569-z.
- Li, X. G., and D. J. Yuan. 2016. “Development of the Security Control Framework for Shield Tunneling in Close Proximity to the Operational Subway Tunnels: Case Studies in Mainland China.” SpringerPlus 5 (1): 527. https://doi.org/10.1186/s40064-016-2168-7.
- Lu, C. F., and C. X. Cai. 2019. “Challenges and Countermeasures for Construction Security During the Sichuan–Tibet Railway Project.” Engineering 5 (5): 833–838. https://doi.org/10.1016/j.eng.2019.06.007.
- Lu, H., J. X. Qi, J. Li, Y. Xie, G. Y. Xu, and H. W. Wang. 2020. “Multi-Agent Based Security Computational Experiment System for Shield Tunneling Projects.” Engineering, Construction & Architectural Management 27 (8): 1963–1991. https://doi.org/10.1108/ECAM-12-2019-0726.
- Ma, L., H. Bin Luo, and H. R. Chen. 2013. “Security Risk Analysis Based on a Geotechnical Instrumentation Data Warehouse in Metro Tunnel Project.” Automation in Construction 34:75–81. https://doi.org/10.1016/j.autcon.2012.10.009.
- Nezarat, H., F. Sereshki, and M. Ataei. 2015. “Ranking of Geological Risks in Mechanized Tunneling by Using Fuzzy Analytical Hierarchy Process (FAHP).” Tunnelling and Underground Space Technology 50:358–364. https://doi.org/10.1016/j.tust.2015.07.019.
- Ntzeremes, P., K. Kirytopoulos, and G. Filiou. 2020. “Quantitative risk assessment of road tunnel fire security: Improved evacuation simulation model.” ASCE-ASME Journal of Risk and Uncertainty in Engineering Systems Part A-Civil Engineering 6 (1): 04019020. https://doi.org/10.1061/AJRUA6.0001029.
- Pan, H. Z., J. Gou, Z. H. Wan, C. X. Ren, M. J. Chen, T. Q. Gou, and Z. H. Luo. 2019. “Research on Coupling Degree Model of Security Risk System for Tunnel Construction in Subway Shield Zone.” Mathematical Problems in Engineering 2019:5783938. https://doi.org/10.1155/2019/5783938.
- Qiu, D. H., C. Qu, Y. G. Xue, B. H. Zhou, X. Li, X. M. Ma, and J. H. Cui. 2020. “A Comprehensive Assessment Method for Security Risk of Gas Tunnel Construction Based on Fuzzy Bayesian Network.” Polish Journal of Environmental Studies 29 (6): 4269–4289. https://doi.org/10.15244/pjoes/115979.
- Reza, M., B. Mojtaba, B. Ezzeddin, and S. H. Sina. 2019. “Assessment of Risks of Tunneling Project in Iran Using Artificial Bee Colony Algorithm.” SN Applied Sciences 1 (12): 1711. https://doi.org/10.1007/s42452-019-1749-9.
- Reza, M., S. H. Sina, and S. Zoheir. 2019. “Geotechnical Risk Evaluation of Tunneling Projects Using Optimization Techniques (Case Study: The Second Part of Emamzade Hashem Tunnel).” Natural Hazards 97 (3): 1099–1113. https://doi.org/10.1007/s11069-019-03688-z.
- Sina, S. H., S. H. Sami, A. A. Mohammed, Z. Shervin, and M. Reza. 2022. “Identifying and Ranking of Mechanized Tunneling Project’s Risks by Using a Fuzzy Multi-Criteria Decision Making Technique.” Journal of Soft Computing in Civil Engineering 6 (1): 29–45. https://doi.org/10.22115/scce.2022.305718.1366.
- Xia, Y. P., Z. M. Xiong, Z. Wen, H. Lu, and X. Dong. 2018. “Entropy-Based Risk Control of Geological Disasters in Mountain Tunnels Under Uncertain Environments.” Entropy 20 (7): 503. https://doi.org/10.3390/e20070503.
- Xun, X. L., J. Zhang, and Y. B. Yuan. 2022. “Multi-Information Fusion Based on BIM and Intuitionistic Fuzzy D-S Evidence Theory for Security Risk Assessment of Undersea Tunnel Construction Projects.” Buildings 12 (11): 1802. https://doi.org/10.3390/buildings12111802.
- Ye, Z. J., Y. Ye, C. P. Zhang, Z. M. Zhang, W. Li, X. J. Wang, L. Wang, and L. B. Wang. 2022. “A Digital Twin Approach for Tunnel Construction Security Early Warning and Management.” Computers in Industry 144:103783. https://doi.org/10.1016/j.compind.2022.103783.
- Zhang, L. M., and W. Y. Chen. 2022. “Multi-Criteria Group Decision-Making with Cloud Model and TOPSIS for Alternative Selection Under Uncertainty.” Soft Computing 26 (22): 12509–12529. https://doi.org/10.1007/s00500-022-07189-3.
- Zhang, L. M., X. G. Wu, L. Y. Ding, M. J. Skibniewski, and Y. J. Lu. 2016. “Bim-Based Risk Identification System in Tunnel Construction.” Journal of Civil Engineering and Management 22 (4): 529–539. https://doi.org/10.3846/13923730.2015.1023348.
- Zhang, L. M., X. G. Wu, L. Y. Ding, M. J. Skibniewski, and Y. Yan. 2013. “Decision Support Analysis for Security Control in Complex Project Environments Based on Bayesian Networks.” Expert Systems with Applications 40 (11): 4273–4282. https://doi.org/10.1016/j.eswa.2012.11.022.
- Zhou, M. K., Y. G. Tang, H. Jin, B. Zhang, and X. W. Sang. 2021. “A BIM-Based Identification and Classification Method of Environmental Risks in the Design of Beijing Subway.” Journal of Civil Engineering and Management 27 (7): 500–514. https://doi.org/10.3846/jcem.2021.15602.