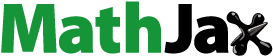
ABSTRACT
Green roofs are an important part of the ecological system in high-density cities and a crucial component of community public green spaces. Analyzing preferences of residents during spatial planning can help improve their satisfaction and efficiency in using community rooftop spaces. This study uses K-modes algorithm to perform cluster analysis on questionnaires from 699 residents, and summarizes user profiles with significant characteristics by combing the comprehensive ratings of residents regarding different roof space functions. The study shows that differences in preferences among people are not only related to demographic characteristics but also to their interests and environmental perceptions. For example, low-to-middle-income groups, being price-sensitive, tend to reduce energy consumption expenditure. The highly educated population, driven by social needs, shows a clear preference for activity spaces and sports facilities. The elderly population, emphasizing healthy eating and rural memories, gives higher ratings to agricultural spaces. Finally, this study explores the correlation between certain resident characteristics and their preferences for green roof space functions, and proposes a “1+X” spatial configuration strategy dominated by Landscape Leisure Space, with Ecological Low-carbon Space, Agricultural Production Space, and Activity Gathering Space as options, in order to optimize community green roof spaces guided by resident needs.
1. Introduction
High-density and high-intensity patterns of estate development have brought about manifold challenges confronting the escalating prominence of various environmental issues, along with rapid urbanization (Cho, Trivic, and Nasution Citation2017). Some compact metropolitan areas are experiencing severe issues such as the urban heat island effect, deteriorated air quality, stormwater runoff, lack of a natural habitat environment, etc (Zhang, Fukuda, and Liu Citation2019). Under the circumstances of intensified residential development characterized by high building loads and plot ratios, the intensification of urbanization has resulted in a dearth of per capita green space and a scarcity of outdoor recreational venues (Cho, Trivic, and Nasution Citation2015). In response, various agreements and treaties have been signed and formulated at the international level with the aim of enhancing the quality of life, promoting environmental stewardship, and ultimately realizing the concept of sustainable development (Debbarma and Choi Citation2022). Administrative departments around the world are dedicated to develop “greening quantity indicators” actively for the purpose of achieving sustainable development goals (Hernández-Moreno and Reyes-Paecke Citation2018). There are various operational approaches to promoting the coverage of vegetation by standardizing the greening of buildings. Considering the many constraints in implementing urban area greening, especially cost, operation and maintenance, and public participation and so on (Bustami et al. Citation2018), green roofs are a highly operational option. The research conducted by Teotónio et al. indicates that the benefits of green roofs increase over time and exceed their construction costs from a socio-environmental perspective (Teotónio, Silva, and Cruz Citation2018), while the study from Alexandri and Jones also points out that green roofs indeed have significant environmental and social benefits (Alexandri and Jones Citation2008). Researches also indicate that green roofs can restore urban green spaces (Rahman et al. Citation2015), alleviate urban heat island effect (Takebayashi and Moriyama Citation2007), increase biodiversity (J. W. Wang et al. Citation2017), and enhance stormwater management capacity (Qin Citation2012). Moreover, the appropriate use of roof space can bring corresponding social benefits and aesthetic values (Barreca Citation2016), effectively improve the living environment and enrich community leisure spaces, increase nature education spaces (Sherry Citation2022), and greatly reduce residents stress and negative emotions, thus contributing to their physical and mental well-being (Williams et al. Citation2019). Among the various types of green roofs, intensive green roofs, in particular, have become increasingly popular as a green space solution for urban issues and revitalizing cities (Kim et al. Citation2018).
Despite the extensive research into the construction costs, material selection, ecological benefits, and policy support for green roofs, investigations into public preferences and engagement with different green roof designs remain significantly underexplored (Jim and Ling Citation2022). In 2013, Jungels et al. conducted a survey on aesthetic preferences of users regarding publicly visible large green roofs, which revealed that different types of green roofs were perceived to have different aesthetic values, and that socio-demographic factors such as age, education level correlated with attitudes from individual, and preferences for green roofs (Jungels et al. Citation2013). People may have different views on green roofs due to their varying levels of their own awareness and experiences. Comprehending public awareness and preferences towards green roofs is crucial for enhancing public involvement and advancing the development of green roof initiatives. This approach not only prevents the misuse of precious urban spaces but also averts the creation of superfluous areas that fail to align with public expectations and social inclusivity. This study on green roofs is limited to residential communities of compact metropolitan areas, which include public spaces within the community such as building penthouses, podium penthouses, terrace levels, and large public terraces etc.
Communities, being the primary living spaces, significantly benefit from green roofs, which are crucial in enriching community gardens and public areas intended for various activities (Hou and Grohmann Citation2018). This study, through case studies and literature analysis, explores the diversity of green roof functions in communities and systematically categorizes the functions of green roofs into Landscape Leisure Space (LLS), Ecological Low-carbon Space (ELS), Agricultural Production Space (APS), and Activity Gathering Space (AGS). Moreover, the construction and maintenance of green roofs require cooperation and consultation among community residents and managers (He and Zhu Citation2018), with each community member being a participant and contributor in the operation of the space. An attractive green roof has an undeniable role to play in enhancing the well-being of community members. Consequently, understanding resident views on green roofs is crucial for designing plans based on how people actually use and experience green roof spaces. Sociology surveys are essential for gaining a better understanding of the spatial elements preferred by individuals. Traditional sociological survey methods can only derive general regularities based on typical phenomena through traditional methods such as sampling surveys and weighted classification, making it difficult to achieve comprehensive and effective data analysis (Bechhofer and Paterson Citation2012). This study collects preferences for green roof elements from different community populations through a questionnaire and comprehensively explored the impact of different community demographic characteristics on green roofs preferences. Using the K-modes clustering algorithm in machine learning to finely classify populations based on their average characteristics, this study explored green roof design forms that are more compatible and participatory with the target population, and can be implemented in high-rise residential communities, which provides designers with recommended objective and realistic functional configurations for green roof spaces, effectively promotes the precise design and refined operation and maintenance of green roofs in communities, enhancing public satisfaction and efficiency.
This study employs the K-modes algorithm to perform a cluster analysis on the questionnaires of 699 residents, evaluating distinct user profiles by sifting through residents’ comprehensive evaluations of different rooftop space functions. This study delves into the underlying motivations behind the formation of above user profiles, detailing that people preference variations are related not only to demographic characteristics but also to their preferences for green roof space functions. For instance, low-to-middle-income groups, being price-sensitive, tend to reduce energy consumption expenditure. The highly educated population, driven by social needs, shows a clear preference for activity spaces and sports facilities. The elderly population, emphasizing healthy eating and rural memories, gives higher ratings to agricultural spaces. The present study aims to evaluate the following problems: firstly, by employing the K-modes clustering algorithm as an alternative to traditional statistical methods in analyzing sociological questionnaires, it is possible to classify the social demographic characteristics of the surveyed population more objectively. This approach allows for the precise extraction of user profile characteristics across different groups, complementing the insights obtained through conventional methods. Secondly, this study effectively analyzes the correlation between the social demographic characteristics of the surveyed population and their preferences for various green roof space functions, thus forming a community green roof spatial configuration method guided by resident needs, aimed at facilitating the extensive adoption of green roofs across diverse communities. Thirdly, this study unveils that resident preferences for various functional spaces within rooftop greening are influenced by factors such as sensitivity to prices, degree of social demand, and attention to healthy diets, which further optimizes green roof design strategies for different types of communities. The research findings of this study can provide suggestions for optimizing the design of green roofs in first-tier and new-line cities along the southeastern coast of China.
2. Literature review
2.1. Conceptual category of green roofs in residential communities
Green roofs are widely recognized for adapting to numerous ecological functions and meeting the needs of diverse human activities. The International Green Roof Association (IGRA Citation2022) categorizes green roofs into extensive, intensive, and semi-intensive types based on their maintenance, soil depth, roof load capacity, water retention capacity, plant species, and human activity characteristics. Extensive green roofs are typically not open to the public, and typically have shallower substrate, lower maintenance costs, and a simpler plant selection compared to intensive green roofs or rooftop gardens (Getter and Rowe Citation2006). Intensive green roofs, also known as rooftop parks, are typically open to the public or specific user groups (such as building residents) depending on the discretion of owners (Bianchini and Hewage Citation2012). Intensive green roofs, characterized by extensive use and accessibility to the public, incur higher construction and maintenance costs due to the need for a diverse selection of plants and the incorporation of multifunctional spaces (Tam Citation2020). The construction of community roof gardens is a composite, multi-dimensional green infrastructure that combines open public activity spaces on the roofs of buildings in the community (H. Wang et al. Citation2022). Intensive green roofs have a similar appearance to traditional ground-level gardens and can increase the living and recreational space in densely populated urban areas (Oberndorfer et al. Citation2007). Semi-intensive green roofs, situated between extensive and intensive green roofs, possess characteristics that are a combination of both (Chow and Bakar Citation2019).
The construction of residential community green roofs is no longer simply a traditional pattern of planting and greening methods, but also involves multiple dimensions, such as ecosystem (Mishra and Sakharle Citation2022), people participation (Francis and Lorimer Citation2011), and operation and maintenance (Kosareo and Ries Citation2007). Due to the complex characteristics and diverse demands of people activities in residential community green roofs, the green roofs mentioned in this study belong to the category of intensive green roofs.
2.2. Classification of intensive green roof spatial functions in residential communities
The green roofs in residential communities are considered a crucial factor that influences the urban resident physical and mental health, as well as their quality of life (Sosnowska et al. Citation2022). By conducting a literature review and examining existing case studies, this study subdivides green roofs into four types of spaces: Landscape Leisure Space (LLS), Ecological Low-carbon Space (ELS), Agricultural Production Space (APS), and Activity Gathering Space (AGS). The functional modules of LLS include plant selection and planting, leisure and rest areas, as well as functional spaces such as landscape sculptures and installation art (Kural Citation2010; Taib et al. Citation2019). ELS functions include rainwater collection systems, organic waste reused systems, and solar photovoltaic systems (Sattler, Zluwa, and Österreicher Citation2020; Ureta et al. Citation2021). APS provides crop planting and harvesting experiences, neighborhood cold meal preparation and sales, fruit and vegetable markets, and functional spaces for child education on agriculture and nature (Appolloni et al. Citation2021; Nasr, Komisar, and de Zeeuw Citation2017). AGS comprises spaces for neighborhood chats, social gatherings, sports activities, and artistic and cultural events such as painting exhibitions and concerts (Kana Citation2012; Fr; Kwon, Lee, and Sohn Citation2020). Combing some successful green roof cases in recent years, presents a selection of representative examples integrated and summarized according to their spatial functions. Above categorization reflects the theoretical construct of the multifunctionality of green roof spaces and provides definitions of key variables for subsequent research.
2.3. K-modes clustering algorithm used in environmental spatial design
In previous research, sociological surveys have been indispensable methods utilized by numerous researchers to better understand resident preferences for the spatial elements of community green roofs. Machine learning has been developed rapidly in recent years and has led to an explosive growth in its application in urban landscape design (Stupariu et al. Citation2021). Its advantage lies in the analysis of existing data to discover patterns and uncover the underlying mechanisms, thereby enabling the inductive summarization of observed phenomena. Meanwhile, Cluster Analysis as a significant algorithm for processing and categorizing homogeneous data in machine learning, can be effectively applied in classifying user profiles based on quantitative data (Cufoglu, Lohi, and Everiss Citation2017), providing a valuable classification tool for sociological surveys. Clustering Analysis is an unsupervised machine learning method that explores correlations between clusters and evaluates the similarity of data within clusters. Clustering attempts to partition samples in a dataset into several non-overlapping subsets, each of which is typically referred to as a “cluster”. Through above partitioning, each cluster may correspond to certain underlying concepts or categories. The clustering process automatically forms the structure of clusters, while the semantic concepts corresponding to each cluster require interpretation and naming by the users (Hennig et al. Citation2015).
There are many methods for clustering analysis, and the currently common K-means clustering is a representative of local prototype objective function clustering method, which uses the Euclidean distance between data points and prototypes as the optimization objective function and is suitable for continuous numerical data (Feng et al. Citation2024; Jain Citation2010). However, the survey questions in the resident evaluation of open roof spaces usually involve selecting one or more options from a limited set of discrete options, such as information about gender, age, education level, etc. Categorical attribute data, which consist of discrete categories or labels rather than numerical values, present challenges for accurate analysis using the K-means clustering method. The K-modes clustering algorithm (Cao, Liang, and Bai Citation2009), an adaptation of the K-means algorithm, is specifically designed for clustering categorical data. It is recognized as a classic algorithm in this field due to its high time efficiency. The algorithm requires its user to pre-set the number of clusters and then continuously updates the cluster centers through multiple iterations, ultimately allowing the Hamming distance (i.e., information dissimilarity measure) between all data points and this corresponding cluster centers to become stable. Therefore, this study utilizes the K-modes clustering method to perform cluster analysis on the demographic characteristics involved in the survey questionnaire. After forming the clustering results, this study provides a descriptive user profile description for each cluster and evaluates real functional needs and participation levels about residential community green roofs from each feature group segmented by various population characteristics. Through a rational and effective analysis on correlation between the demographic characteristics of the survey participants and their preferences for residential community green roof spaces.
3. Methods
3.1. Study area
This study takes Hangzhou, Zhejiang Province, China, as an example, and the research results are applicable and referable within the limited scope of first-tier or new first-tier high-density cities along the southeast coast of China. Hangzhou is a rapidly developing coastal metropolis in China and is one of the fastest-growing cities in the country (Byrne, Lo, and Yang Citation2015). It is situated in a region with a typical subtropical monsoon climate and a varied terrain (Domrös and Peng Citation2012), whose climate is characterized by significant monsoons, distinct seasons, moderate annual temperatures, abundant sunshine, ample rainfall, humid air, synchronous changes between the rainy and hot seasons, diverse climate resources, and multiple meteorological disasters (Y. Wang et al. Citation2020). Meanwhile, Hangzhou is a new first-tier city with a deeply developed urbanization, high population density, and compact and intensive development patterns in most of its central communities. In 2019, the Development and Reform Commission of Zhejiang Province proposed relevant documents about construction of “The Future Community” (Development and Reform Commission of Zhejiang Province Citation2019). Among above documents, the “Pilot Work Plan for the Construction of The Future Community in Zhejiang Province” pointed out that the construction of the Future Community should focus on green and intensive land use, and innovative development with spatial concentration. It is necessary to scientifically and reasonably determine building volume ratio and other planning technical indicators. At the same time, the plan should allow the urban green space rate to encompass the public three-dimensional greening of involved pilot projects reasonably, and encourage and support the establishment of community agriculture or other types of comprehensive utilization mechanisms for three-dimensional greening.
3.2. Survey questionnaire and data collection
In order to systematically gather relevant information, this study designed a quantitative questionnaire aimed at collecting data on the social characteristics of the respondents, as well as their preferences towards various forms of green roofs. The survey questionnaire consists of 23 questions and 3 images (attached as Appendix A). The content is divided into three main parts: (1) The socio-demographic characteristics of the respondents: capturing basic information such as gender, age, and level of education. (2) Evaluating residents’ acceptance and participation for green roofs. This section refers to the rooftop greening classification by The International Green Roof Association (IGRA Citation2022) and assesses three types of roofs: Empty roof, Extensive green roof, and Intensive green roof, with images to aid respondents’ understanding. Responses are scored using a 5-point Likert scale (“1” - “very willing”, “5” - “very unwilling”). (3) Assessing residents’ attitudes towards 12 types of spaces within four categories of green roof spaces (LLS, ELS, APS, AGS). The questions in survey questionnaire are based on the research framework established from above literature review, aiming to delve into respondents’ preferences and evaluations of various green roof space functions. To capture the nuanced differences in respondents’ preferences and enhance the data analysis precision when applying the K-modes clustering algorithm, the study extends the Likert scale to a 10-point system (“1” - “very dislike”, “10” - “very like”) (Dawes Citation2008). By collecting data from above three sections, this study aims to analyze the correlation between respondents’ socio-demographic characteristics and their preferences for different green roof space functions.
The respondents of the questionnaire were Hangzhou residents over 14 years old. The sample size was calculated based on China Population Census data (Office of the Leading Group of the State Council for the Seventh National Population Census Citation2021), using random stratified sampling method (Parsons Citation2014). The sample size was sufficient to ensure a maximum margin of error of 5 % for a 95 % confidence interval on the population proportion. To capture the genuine perspectives and needs of community residents from different developmental stages towards green roofs, the survey conducted geographical stratification based on the construction era of communities. As shown in , six communities built in Hangzhou’s main urban area from 1990 to 2020 were selected for the survey. These communities were chosen considering multiple dimensions such as construction era and community type, including: communities built before 2000, primarily composed of multi-story buildings; residential communities built between 2000 and 2010, mainly consisting of high-rise commercial housing; and newer, more modern communities built after 2010 with superior living environments. Research assistants randomly and anonymously selected respondents within six communities and surrounding streets, aiming to cover a sample of respondents from different types of communities in Hangzhou’s main urban area with diverse socio-demographic characteristics. This study conducted a pilot test before the formal survey, which took place in late March, lasting for 20 days. This period typically marks the beginning of spring in the Hangzhou area, with gradually rising temperatures and a moist climate, ideal for planting on green roofs (Dunnett and Kingsbury Citation2008). Based on the pilot test results, the daily time slot from 12:00 to 22:00 was identified as the peak period for local residents’ social activities and foot traffic, hence the highest survey response rate. Therefore, the formal survey adopted an intermittent survey strategy during this time slot.
Ethical approval for the questionnaires was obtained in advance from the author’s affiliated university (Approval Document Number: DFL2023028001). Prior to filling the questionnaire, each respondent was informed of the research purpose. At the beginning of the survey questionnaire, each participant received written information about the purpose of the study and the ethical approval details. For participants aged 14 to 17, dual informed consent was obtained, with both the minors and their legal guardians providing approval. Throughout the survey process, research assistants interacted with 1000 pedestrians, securing 803 participants, resulting in an 80.3% response rate. Employing the questionnaire data validity techniques recommended by Saris & Gallhofer (Saris and Gallhofer Citation2014), 59 questionnaires were discarded due to incompleteness, 7 were invalidated by logical inconsistency, and 38 were excluded due to the presence of extreme values. Thus, 699 valid questionnaires were used for data analysis, marking an effective response rate of 87.0%.
The statistics of first section of questionnaire, presented in , collected demographic characteristics information among the 699 valid questionnaires. The proportion of females was slightly higher than that of males, at 53.1%. Regarding the age distribution, the respondents’ ages varied from 14 to 84 years. Individuals aged 25 to 40, formed the largest segment, making up 38.6% of the total. Subsequently, the age group of 41 to 55 years, comprised 28.2% of the respondents. In terms of education, 29.3% of respondents had a high school education or less, while 31.9% had a bachelor’s degree. For occupational statistics, the questions in the questionnaire are divided into 10 categories, of which company employees, students, and professionals and technical workers accounted for the highest proportion, accounting for 18.9%, 17.9%, and 17.3%, respectively. Regarding income levels, this study used the Hangzhou City National Bureau of Statistics’ first quarter 2023 average monthly (Statistics Citation2023) disposable income of 1120 USD as a basis to divide respondents into five income brackets. Individuals with a monthly income of less than 700 USD are categorized as low or no income, accounting for 25.3%; those earning 700–1400 USD monthly are considered middle-income, the largest group at 41.5%; individuals earning 1400–2800 USD are classified as upper-middle-income, representing 16.8%, while those earning over 2800 USD are high-income, accounting for 16.5%. Additionally, statistical data reveal that 58.5% of respondents live in multigenerational households, while 38.9% live alone or in non-family cohabitation.
Table 1. Distribution of respondent characteristics.
presents the second part of the questionnaire, which assesses respondent acceptance level of green roofs. Among the choices of green roof types, the majority of respondents (85.6%) selected Garden Roof, while the number choosing Empty Roof and Lawn Roof was relatively small, at 6.7% and 8.0%, respectively. In terms of activity frequency in the community, the highest proportion (28.1%) reported engaging in community activities 2–3 times per week, while the lowest proportion (13.5%) reported engaging in community activities once a week. Regarding the willingness to participate in community green roofs, a significant proportion, 77.7%, expressed a strong desire that were “very willing” or “willing” in participating. Conversely, a smaller proportion, 4.6%, indicated a lack of interest or strong disinterest, while 17.7% of the respondents remained neutral. Above findings suggest that participatory green roof designs are highly favored in the community, and that residents have a high level of acceptance towards them.
Table 2. Distribution of respondent acceptance level about green roofs.
The statistics of the third session of the questionnaire are shown in , the purpose of which is intend to explore the attitudes of various respondents towards different types of green roof spaces. This exploration serves as a foundation for subsequent research into the correlation between the social demographic characteristics of respondents and their preferences for various functions of green roof spaces.
Table 3. Respondent preference degree to roof functional forms.
3.3. Application of K-modes algorithm for data categorization
The K-modes clustering algorithm is a clustering algorithm designed for categorical attribute datasets. Its advantage is that it uses a simple 0–1 difference measure to solve the problem of dissimilarity measures between categorical attribute data. Let’s assume that is a set of
samples to be tested, while the samples
and
to be clustered are vectors consisting of
components categorical attributes, i.e.
,
.
In this study, the Hamming distance between two sets of vectors, , and
, are defined as the dissimilarity between two different samples, i.e.
Among the formula, . When the
-th attribute values of two samples are the same (
),
is set to 0. When the
-th attribute values of two samples are different (
),
is set to 1. The dissimilarity between samples
, and
is the sum of the differences between all
feature values between
, and
.
The initial number of clusters for the K-modes clustering algorithm can be specified according to the specific business needs. When there is no prior knowledge, a clustering criterion function that can judge the quality of the current clustering result needs to be considered. The better the clustering criterion function result, the higher the accuracy of the clustering result, and the clustering method can more effectively and accurately divide objects into subcategories. The clustering criterion function for the K-modes clustering algorithm usually uses Sum of Squared Error (SSE) (Taber Citation2009), whose specific function is as follows:
In this formula, is the membership matrix of set
;
represents the number of elements contained in the object set
, corresponding to the row vectors in the membership matrix;
represents the number of sub-classes in the partition, corresponding to the column vectors in the membership matrix.
is a non-empty finite set consisting of
objects;
represents the class center of the sub-class being partitioned.
represents the dissimilarity function between an object
and the class center
. As the cluster number
increases, the sample partition becomes finer, and the cohesion of each cluster gradually increases. Therefore, SSE naturally decreases. Moreover, when
is less than the true number of clusters, increasing
will significantly increase the cohesion of each cluster, leading to a large decrease in SSE. However, when k reaches the true number of clusters, the return on increasing k will quickly diminish, causing the decline in SSE to plummet sharply. Then, as the value of
continues to increase, the decline in SSE will gradually level off. In this study, the center points of the
clusters obtained by the K-modes clustering algorithm are denoted as
, and the clustering sets corresponding to each original point are denoted as
. For different values of k, different clustering metrics are obtained. By plotting
on the x-axis and SSE(
) on the y-axis, a graph with an elbow shape is obtained. The value of
corresponding to the elbow point is the recommended cluster number for the K-modes clustering algorithm.
The 699 valid survey questionnaires mentioned earlier included six demographic characteristics: gender, age, education level, occupation, income, and number of family members, among which occupation and income are correlated and not independent, with some redundancy, thus, need to be normalized dimensionality reduction. The frequency of community activities in the questionnaire can be calculated as a demographic characteristic. Therefore, this study used K-modes clustering to calculate six classification characteristics: gender, age, education level, occupation/income, number of family members, and frequency of community activities. This study calculated the SSE () values of K-modes corresponding to different cluster numbers k and obtained . According to the elbow method mentioned above, the inflection point
was considered an appropriate clustering number.
Based on the business requirements and the computational results of SSE, this study selects K = 16 as the cluster number for the K-modes clustering algorithm. The clustering calculation is performed on six discrete attributes of 699 samples, and the specific steps are as follows:
Randomly select 16 cluster centers
, where each cluster center
is a vector of length 6, i.e.
.
For each sample
, compare its Hamming distance with the other 16 clustering centers.
Assign each sample
, to the cluster with the minimum distance. After all samples have been assigned, re-select the cluster centers
Repeat steps (2) and (3) until the total Hamming distance no longer decreases, and then return the clustering results.
Table 4. Cluster centers of 16 clusters from K-modes clustering.
Finally, this study obtains 16 cluster centers through the K-modes clustering algorithm, as shown in . The maximum Hamming distance (MHD) represents the maximum number of dissimilar characteristic values between the data in each cluster and its corresponding cluster center. When new data needs to be grouped and classified, the Hamming distance between the data and each cluster center is calculated by summing up the number of different characteristic values between them. By comparing the Hamming distance between the data and each cluster center, the data should be classified into the cluster who has the smallest Hamming distance. This Hamming distance should be less than the MHD of the corresponding cluster shown in .
4. Results and discussion
4.1. Respondents reclassification based on clustering result
This study uses the K-modes clustering algorithm to divide 699 respondents into 16 clusters, with individuals within each cluster having similar or identical personal characteristics values. By combining the ratings of different survey questions within different spatial ranges, this study can summarize the preference levels of different clusters for each space, which is calculated through the following formula:
In the formula, (
) represents each cluster, with
respondents within each cluster.
represents different types of green roof spaces, corresponding to the four types of green roof spaces mentioned in and : Landscape Leisure Space (LLS), Ecological Low-carbon Space (ELS), Agricultural Production Space (APS), and Activity Gathering Space (AGS). Each space has
questions in the survey.
represents the score of each respondent in each question within each cluster.
represents the average score of the
-th (
) cluster for the green roof space
. By calculating average score from each respondent in each space within each cluster, this study obtains preference distribution of each cluster for the four types of green roof spaces. After aggregating the clusters that have the same preference distribution for spaces, as shown in , this study obtains six groups of people who have different focuses on each space elements.
From the spatial preference ranking in it is clear that the score for LLS is the highest among all six groups. This indicates that most respondents agree “rich plant configurations, open recreational space, and beautiful art installations” are the most important components of green roof design. Therefore, it can be seen that the elements related to viewing and recreation are the main functions of green roofs. However, for the ecological, production, and activity functions of green roofs, the preference deviations of different groups are more significant. Therefore, in the construction of green roofs, LLS is necessary as a design space, and the remaining three types of spaces should be ranked and reclassified according to user preferences.
reveals distinct spatial preference rankings among different clusters. Group 1 (Clusters 1, 4, 8, 16) prefers ELS, then APS, least AGS. Group 2 (Clusters 3, 11, 12, 14) likes ELS, then AGS, least APS. Group 3 (Clusters 2, 6, 7, 15) ranks AGS highest, ELS next, APS least. Group 4 (Cluster 9) favors AGS, then APS, least ELS. Group 5 (Clusters 5, 10) chooses APS, then AGS, least ELS. Group 6 (Cluster 13) prefers APS, then ELS, least AGS, albeit with weaker referentiality due to its small size. By integrating the scores of the six groups of respondent preferences for the four types of spaces, this study categorizes green roofs into Ecological Landscape Green Roofs, Agricultural Landscape Green Roofs, and Active Landscape Green Roofs. LLS scores highest across all categories, highlighting its core importance, while the ratios of the other three spaces can be optimized based on the target user group.
4.2. User profiles and preference analysis
Variations in social demographic factors such as age and education level lead to differences in knowledge and experience with green roofs, as well as differences in attitudes and preferences for different types of space functions. present the radar charts of the demographic characteristics for each group, highlighting the individual profiles. This study examines the correlation between the demographic characteristics of the respondents and their preferences for APS, ELS, and AGS in green roofs, based on the diverse rankings of scores for above three types of spaces.
As mentioned in Section 4.1, Group 1 and Group 2 prioritize ELS over APS and AGS in green roofs attributed to unequal income level and larger family size. As shown in , the monthly average income of Group 1 ranges from 700–1400 USD, while the income of Group 2 is concentrated below 700 USD. Both groups are middle-aged or young (25–55 years old) and have a relatively large number of family members (including dependent children with no income or low-income elderly), resulting in a significantly lower per capita disposable income compared to the average monthly per capita disposable income of 1120 USD in urban residents in Hangzhou during the first quarter of 2023, according to statistical reports (Statistics Citation2023). The research conducted by Wakefield and Inman has reported that this phenomenon is influenced by an income main effect, meaning that limited disposable income has a greater impact on the life quality of low-income families, which leads to a fact that low-income families exhibiting greater price sensitivity than high-income families (Wakefield and Inman Citation2003). Both groups are most concerned with the construction of ELS in community green roofs, as having a strong desire to reduce energy consumption such as water and electricity, and to improve energy-saving benefits, thus reducing energy spending, and cutting their cost of living. The reason for this is that energy expenditure accounts for a relatively high proportion of the total living expenses in low-income families, which places a heavy burden on them (Chen et al. Citation2021), and most low-income groups generally bear the burden of poor housing quality and low energy efficiency, which in turn leads to their high expectations for the construction and use of renewable energy represented by solar photovoltaic systems (Lee and McCuskey Shepley Citation2020). Most of the respondents in Group 1 are ordinary employees or blue-collar workers engaged in professional technical work. Such occupation generally involves long working hours, less leisure time, and a relatively low willingness to socialize with neighbors in the community, resulting in a weak demand for gathering spaces in the community green roofs. At the same time, the space available for leisure entertainment in the communities where low-income groups live is also significantly limited, which leads to a lower participation rate in leisure spaces (Dahmann et al. Citation2010). Compared with entertainment-oriented gathering spaces, productive agricultural spaces with tangible benefits are more attractive to low-income groups. In contrast to Group 1, Group 2 respondents have fewer family members living with them and generally have lower levels of education, with over half of the respondents having a high school education or below. Compared with people with higher education levels, people with lower education levels have relatively poor dietary habits (Axelsen et al. Citation2012). According to the survey information, there are many individual business owners, ordinary employees, and students without income in Group 2. Due to the lack of interest-related elements, their interest in rooftop agriculture and their willingness to experience rural life are relatively weak.
Group 3 and Group 4 both consider AGS to be more important than ELS and APS in green roofs. This result seems to be associated with individual social status, with higher-educated individuals showing a higher approval of entertainment spaces (Zanon et al. Citation2013). Both groups of respondents prefer community activity spaces with diverse choices and have a high social demand. This is because people with higher education levels value social activities in the community, and are more willing to participate in community activities and public welfare services (Jungels et al. Citation2013). In addition, some social factors (Pan et al. Citation2009), such as encouragement and suggestions from family and friends, positively influence the frequency of community residents participating in sports activities (McNeill, Kreuter, and Subramanian Citation2006). As shown in compared with Group 4, the respondents in Group 3 are generally young and well-educated. Combining employment status as indicated in the questionnaire, the respondents in Group 3 can be broadly divided into subgroups: low-income students pursuing higher education (master’s or doctoral degree), and high-income professionals with advanced degrees (mostly urban white-collar workers and corporate executives). Since having a relatively higher education level, Group 3 exhibits a deeper understanding of the low-carbon ecological concept and a higher acceptance of renewable energy, resulting in a comparatively higher evaluation of ELS (Sardianou and Genoudi Citation2013). However, since the relatively substantial income and the less sensitive to price, Group 3 demonstrates a commendable acceptance of ELS ranking second only to AGS. This group gives a relatively low rating to APS in community green roofs, primarily due to the skepticism towards the environmental and sanitary issues, fertilizer odors and summer mosquito problems, and other issues arising from APS (Hallett, Hoagland, and Toner Citation2016). Respondents in Group 4 are mostly middle-aged, high-income individuals with a bachelor’s degree or above. According to occupational options selected in the questionnaire, a significant number of respondents are executives or senior professionals, characterized by being the backbone of their families and often having three generations living together, including elderly individuals and children. Their focus on the functionality of green roof spaces primarily revolves around meeting the needs of the elderly and children, aiming to enhance the activity and entertainment areas for each family members. This particular group exhibits strong social attributes in terms of their entertainment and sports requirements, and the bonds of family and friendship play a vital role in sustaining these demands (Wienke and Jekauc Citation2016). Additionally, Group 4 has a high concern for dietary health and cares about community crop cultivation, pesticide-free fruit and vegetable procurement, and natural education experiences for children. Despite supporting the construction of ELS, Group 4 demonstrates a relatively lower of interest in ELS compared to AGS and APS.
Both Group 5 and Group 6 consider APS to be more important than ELS and AGS in green roofs, and this result seems to be related to individual age and social background. Research conducted by Fernandez-Canero et al. shows that respondent preferences are related to their childhood environment (Fernandez-Canero et al. Citation2013). From 1961 to 2020, the urbanization rate in Zhejiang Province has increased by 57.9% (Zhejiang Urban Development Research Centre Citation2021). Most of the respondents in above age group have experience participating in agricultural labor in rural areas and have deep memories of the countryside. They have a stronger sense of belonging and recognition of the rural culture of agricultural labor (Morse and Mudgett Citation2017), hence a higher interested in community APS participation. As shown in , the typical characteristics of Group 5 are middle-aged and elderly people with above-average income, relatively abundant spare time, high frequency of community activities. Among them are plenty of retired elderly, who are aging at home and being a consumer group with high interest in the health sector. The elderly make full use of their spare time and consider gardening and crop planting labor as a form of daily physical exercise, thereby fulfilling their daily entertainment and leisure needs (He and Zhu Citation2018). Meanwhile, Group 5 has a strong social willingness and acceptance of activity spaces in the community, focusing on the sense of participation and experience in community activities. Similar conclusions have been confirmed in previous study, where leisure activity spaces play an important role in improving the happiness of middle-aged and elderly people (Scott, Masser, and Pachana Citation2020). Participating in activity gathering spaces can help the elderly establish social relationships, satisfy their social needs, acquire new skills and knowledge and experience positive emotions, which contribute to enhancing their quality of life (Brajša-Žganec, Merkaš, and Šverko Citation2011). In , the most prominent characteristic of Group 6 is that the majority of its members are middle-aged individuals with low incomes, who have limited participation in community activities and often reside in three-generation households. Consistent with previous research results, respondents in the low-income group exhibit a higher price sensitivity and express greater concerns regarding agricultural yields from green roofs and energy-saving features of ELS, while their demand for sports and entertainment spaces in the community is relatively low. It should be noted that the number of respondents in Group 6 is relatively small, and the results should be interpreted with caution. The data from Group 6 alone may not possess significant reference value and should be considered in conjunction with other findings.
In summary, factors influencing community participation in green roof spaces can be categorized into individual factors, social factors, and environmental perception factors. Individual factors include age, income, occupation, and education level. Income directly affects respondent sensitivity to prices, which is highly correlated with the “willingness to construct ecological functional spaces for energy saving”. Correspondingly, gender differences among community members have no direct correlation with participation in green roofs, which is consistent with some previous research conclusions (Ho et al. Citation2017). Social factors mainly include family composition and the frequency of community activities. When individuals receive support and encouragement from family members, friends, and colleagues, their participation may become more active. The community’s social needs are closely linked to their willingness to gather and participate in the construction of activity spaces. Taking both individual and social factors into account, attention to healthy eating is highly correlated with the willingness to participate agricultural spaces for crop planting and green produce sharing. Environmental perception factors are intricately linked to the notion that community green roofs present valuable opportunities for children in high-density urban areas to play, entertain, and rest, meanwhile offering a diverse array of outdoor leisure experiences for the vibrant young and middle-aged population amidst the hustle and bustle of urban life. Additionally, green roofs can serve as a social platform for the middle-aged and elderly population, effectively alleviating their sense of loneliness and social isolation.
4.3. Green roofs “1+X” spaces constituted configuration
To establish a comprehensive spatial function configuration for community green roofs, this study integrates the scores of six groups of people for specific functions in the four categories of space, which provides an intuitive understanding of the attitude from each group towards specific functions in the four types of spaces. According to the specific ratings of each space shown in , this study proposes a “1+X” configuration space design and selection scheme for green roofs. “1” represents the primary function of green roofs, which is LLS (rated highest among all groups), while “X” represents the selection and matching of the remaining three types (ELS, AGS, APS) of spatial functions according to the characteristics and needs of target people. The specific selection and matching scheme are as follows: Group 1 and Group 2 prefer “Ecological Landscape Green Roofs”; Group 3 and Group 4 prefer “Activity Landscape Green Roofs”; Group 5 and Group 6 prefer “Agricultural Landscape Green Roofs”.
illustrates three types of Green Roofs based on spatial functions. The first type, Ecological Landscape Green Roofs, emphasizes low-carbon environmental protection and resource reuse. It integrates traditional green roof landscape features like planting, relaxation, and cultural sculptures with renewable energy systems such as photovoltaic power generation, rainwater collection, waste reuse, and fine waste classification. For Group 1, In addition to the spaces mentioned above, it is advisable to incorporate certain agricultural production spaces, such as areas for crop planting or natural education, etc. In the spatial configuration scheme of Group 2, people attach great importance on activity spaces in addition to landscape and ecological elements. On this basis, gathering-type activity spaces such as salon parties, sports and fitness, and festival activities can be appropriately added. It is worth noting that residents generally accept rainwater collection and photovoltaic power generation in ecological spaces, while their acceptance of fine waste classification spaces is lower due to concerns about odor issues during waste transportation, storage, and processing. The above two “LLS + ELS” spatial configuration schemes cater to energy-saving needs and preferences of price-sensitive users.
The second type, Activity Landscape Green Roofs, emphasizes entertainment, gathering, and sports activities. Its popularity lies in providing residents with space and facilities for gatherings, socializing, or sports. The activities it accommodates include, but are not limited to, festive exhibitions, salon parties, and sports and fitness, with a primary focus on community participation and a diverse range of activities. Spatial configuration scheme for Group 3 integrates landscape and activity spaces, while appropriately incorporating ecological elements, especially rainwater management space and photovoltaic power generation space. In the case of Group 4, agricultural space can be appropriately added alongside landscape space and activity space. However, in designing activity-oriented roof landscape spaces, noise issues should be taken into consideration, and gathering spaces should be situated on the top floors of public overhead layers or community podiums to minimize interference with residential areas. Above two “LLS + AGS” spatial configuration schemes can offer activity facilities and gathering places that meet the entertainment and leisure requirements of residents with activity and social needs.
The third type, Agricultural Landscape Green Roofs, emphasizes agricultural production, sharing, and education. It incorporates agricultural landscape design into traditional green roofs, enhancing the diversity of green spaces in the community and fostering closer relationships among neighbors. In the spatial configuration scheme of Group 5, gathering and activity spaces can also be appropriately added. In the case of Group 6, ecological low-carbon space can be appropriately added in addition to landscape space and agricultural space. It is worth mentioning that among the spaces for agricultural production, the rating for “crop market and sharing” are the lowest. Residents have a relatively low acceptance of commercial spaces for display and sales, but show a higher acceptance of crop cultivation and natural education spaces. On this basis, community residents hope for more convenient facilities such as barbecue pits, beverage sales, and snack areas. Above two types of “LLS + APS” space allocation schemes not only increase the community green space ratio and diversity but also meet the needs of residents who care about dietary health and love to participating in planting by providing opportunities for pastoral labor.
Based on the characteristics of each group, this study provides space ratio suggestions for designers in the early stages of the design process. Meanwhile, there exists a complementary relationship among the four types of functional spaces in green roofs. For example, the rainwater collection system and photovoltaic power generation system in ecological landscape space can provide irrigation and power support for agricultural production landscapes space, while composting of kitchen waste can serve as organic fertilizer for crop planting. Agricultural production landscapes space, who also belongs to the landscape green spaces in the community, expands the diversity and participation of landscape spaces. Crop production can offer more opportunities for diverse gathering activities, such as green agricultural product markets, cold buffet salons, and other theme activities. Therefore, the design of the scheme should consider demographic characteristics to meet the preferences of the majority while strategically integrating diverse space types to achieve optimization in terms of utilization efficiency and construction costs.
4.4. Community optimization strategies for increasing green roof applications
According to a report by the Zhejiang Development & Planning Institute, the communities in Hangzhou’s main urban area awaiting optimization are primarily categorized into three types: demolition and reconstruction, renovation and modification, and planned new construction (Zhejiang Development & Planning Institute Citation2021). This study integrates these three types of communities and their residents’ sociodemographic characteristics to conduct an analysis of green roof functional configuration strategies.
Communities slated for demolition and reconstruction were mostly established in the 1990s or earlier. These communities generally consist of multi-story buildings such as employees dormitories and relocation houses, predominantly inhabited by older local residents (Qian Citation2012). According to the clustering results of the K-modes algorithm, this demographic tends to exhibit higher price sensitivity, with a general preference for ecological landscapes that offer direct economic benefits. In such communities, green roof space configurations can emphasize ecological energy efficiency, integrating landscape construction with solar photovoltaics and rainwater harvesting systems to reduce residents’ energy consumption costs. For communities undergoing renovation and modification, these areas blend relatively well-conditioned mid-term buildings with older structures requiring improvement, commonly seen in high-rise commercial residences constructed around the year 2000. Given the generally high floor area ratio of high-rise residential buildings in urban centers, these communities have relatively limited per capita green space and activity areas (Tian, Jim, and Wang Citation2014). Residents show a high demand for community landscapes available for outdoor activities. Thus, green roof configurations that accommodate various functions of leisure and activity spaces should be prioritized in communities undergoing renovation and modification. Additionally, the newly planned residential communities in Hangzhou’s central area mainly cover modern residential areas built in the last decade. The higher property prices in these communities reflect the residents’ relatively superior economic conditions (Wen, Jin, and Zhang Citation2017). The sociodemographic characteristics of these residents indicate that the demand for green roofs in newly planned communities is not limited to leisure, entertainment, and natural education functions but also includes the integration of modern facilities such as intelligent ecosystems to enhance living quality (Xiao, Hui, and Wen Citation2019).
In the community optimization process, green roof functional configuration strategies are based on the results of an in-depth analysis of community types and resident characteristics. The aim is to enhance residents’ acceptance and participation, thereby promoting the widespread application of green roofs in various communities. Furthermore, the spatial configuration of green roofs should comprehensively consider key factors such as site conditions, spatial scale, and budget constraints to ensure the feasibility and maximization of project benefits.
5. Conclusion
Green Roofs have significant environmental and social benefits, however, research on public preferences and participation regarding different forms of green roofs remains limited. Understanding preferences is crucial for promoting public participation, avoiding wastage of urban space, and enhancing community well-being. This study explores the influence of resident sociodemographic characteristics on the preferences for functional elements of green roof spaces, centering on high-density urban communities along the southeastern coastal regions of China. For the first time, this study introduces the K-modes clustering algorithm from machine learning into clustering resident preferences for green roof spaces, identifying distinctive respondent categories objectively and precisely. By integrating the specific ratings of clusters resulting from K-modes clustering algorithm for four types of green roof spaces (i.e., Landscape Leisure Space (LLS), Ecological Low-carbon Space (ELS), Agricultural Production Space (APS), and Activity Gathering Space (AGS)), this study establishes user profiles and analyzes the correlation between user groups and space preferences. The key findings indicate that LLS is the most critical component in the configuration for community green roofs, favored by users from all groups. Meanwhile, low-to-medium income groups with larger family sizes demonstrate a stronger inclination towards ELS, aiming to reduce water and energy consumption, and enhance resource efficiency. Middle-aged individuals with higher educational levels exhibit a strong demand for social interaction and recreational activities, particularly favoring AGS such as community gathering places and sports facilities. Retirees and elderly individuals, grounded in health-conscious diets and nostalgic memories of rural life during their youth, highly appreciate APS.
Further analysis of the study reveals that factors influencing community green roof participation predominantly encompass individual, social, and environmental perception factors. Individual factors such as age, income, and education level exhibit significant effects on the public preferences for green roof space functions. Social factors mainly involve family composition, community activity frequency, and the support and encouragement received from family members, friends, and other companions, all of which play a positive role in individual participation. Environmental perception factors refer to the fact that personal perception and attitude towards the functions and benefits provided by green roofs vary accordingly, resulting from the diversity of individual and social factors among people. Furthermore, this study culminated in a set of green roofs spatial function configuration scheme, offering designers personalized spatial ratio recommendations during the preliminary planning stage of green roofs.
While this study aims to promote green roof applications through an in-depth analysis of community residents’ preferences, the process of community optimization actually involves many more factors than just the few resident preferences mentioned above. When developing and implementing community optimization strategies, various factors including site constraints, economic feasibility, policy support, and technological innovation must be considered. This study focuses on resident preferences for green roof functional spaces without delving into the specific implementation strategies of these spatial configurations in practice. Given these limitations, future research needs to explore more deeply how to enhance green roof applications through community optimization strategies, including the process of strategy formulation and balancing resident preferences with the needs for community sustainable development. Research should also focus on the long-term maintenance and management of green roofs, exploring ways to enhance economic and environmental benefits through policies and technological innovation, thereby promoting application on a broader scale.
CRediT authorship contribution statement
Yi-Tong Cui: Conceptualization, Methodology, Investigation, Formal analysis, Visualization, Funding acquisition, Roles/Writing – original draft, Writing-review & editing, Software, Investigation, Data curation.
Guang-Zhu Zhang: Conceptualization, Supervision, Validation, Funding acquisition, Writing-review.
Acknowledgements
The authors express their profound gratitude to Mr. Haidong Xi from Cisco Systems (China) Research and Development Co., Ltd., Hangzhou Branch, for his expert assistance with data processing, which has significantly enhanced the depth and rigor of our research. The authors also would like to extand their sincere thanks to Associate Professor Wenwen Shi, Associate Professor Ning Gao, Professor Xiaobo Tang, Dr. Liang Du, Dr. Hongjia Ge, and Dr. Weicong Li for their invaluable methodological guidance during the initial stages of this study.
Disclosure statement
No potential conflict of interest was reported by the author(s).
Additional information
Funding
Notes on contributors
Yi-Tong Cui
Yi-Tong Cui, a Lecturer at Zhejiang University of Finance & Economics Dongfang College, who specializes in landscape space creation and Behavior-Environment Relationship.
Guang-Zhu Zhang
Guang-Zhu Zhang, an Associate Professor at Northeast Forestry University and a Professor at The Catholic University of Korea, who specializes in green building materials.
References
- Alexandri, E., and P. Jones. 2008. “Temperature Decreases in an Urban Canyon Due to Green Walls and Green Roofs in Diverse Climates.” Building and Environment 43 (4): 480–493. https://doi.org/10.1016/j.buildenv.2006.10.055.
- Appolloni, E., F. Orsini, K. Specht, S. Thomaier, E. Sanyé-Mengual, G. Pennisi, and G. Gianquinto. 2021. “The Global Rise of Urban Rooftop Agriculture: A Review of Worldwide Cases.” Journal of Cleaner Production 296:126556. https://doi.org/10.1016/j.jclepro.2021.126556.
- Axelsen, M., M. Danielsson, M. Norberg, and A. Sjöberg. 2012. “Eating Habits and Physical Activity: Health in Sweden: The National Public Health Report 2012. Chapter 8.” Scandinavian Journal of Public Health 40 (9 Suppl): 164–175. https://doi.org/10.1177/1403494812459473.
- Barreca, F. 2016. “Rooftop Gardening: A Solution for Energy Saving and Landscape Enhancement in Mediterranean Urban Areas.” Procedia-Social and Behavioral Sciences 223:720–725. https://doi.org/10.1016/j.sbspro.2016.05.248.
- Bechhofer, F., and L. Paterson. 2012. Principles of Research Design in the Social Sciences. Routledge. Chapter 2. https://doi.org/10.4324/9780203136720.
- Bianchini, F., and K. Hewage. 2012. “Probabilistic Social Cost-Benefit Analysis for Green Roofs: A Lifecycle Approach.” Building and Environment 58:152–162. https://doi.org/10.1016/j.buildenv.2012.07.005.
- Brajša-Žganec, A., M. Merkaš, and I. Šverko. 2011. “Quality of Life and Leisure Activities: How Do Leisure Activities Contribute to Subjective Well-Being?” Social Indicators Research 102 (1): 81–91. https://doi.org/10.1007/s11205-010-9724-2.
- Bustami, R. A., M. Belusko, J. Ward, and S. Beecham. 2018. “Vertical Greenery Systems: A Systematic Review of Research Trends.” Building and Environment 146:226–237. https://doi.org/10.1016/j.buildenv.2018.09.045.
- Byrne, J. A., A. Y. Lo, and J. Yang. 2015. “Residents’ Understanding of the Role of Green Infrastructure for Climate Change Adaptation in Hangzhou, China.” Landscape and Urban Planning 138:132–143. https://doi.org/10.1016/j.landurbplan.2015.02.013.
- Cao, F., J. Liang, and L. Bai. 2009. “A New Initialization Method for Categorical Data Clustering.” Expert Systems with Applications 36 (7): 10223–10228. https://doi.org/10.1016/j.eswa.2009.01.060.
- Chen, C.-F., H. Nelson, X. Xu, G. Bonilla, and N. Jones. 2021. “Beyond Technology Adoption: Examining Home Energy Management Systems, Energy Burdens and Climate Change Perceptions During COVID-19 Pandemic.” Renewable and Sustainable Energy Reviews 145:145. https://doi.org/10.1016/j.rser.2021.111066.
- Cho, I. S., Z. Trivic, and I. Nasution. 2015. “Towards an Integrated Urban Space Framework for Emerging Urban Conditions in a High-Density Context.” Journal of Urban Design 20 (2): 147–168. https://doi.org/10.1080/13574809.2015.1009009.
- Cho, I. S., Z. Trivic, and I. Nasution. 2017. “New High-Density Intensified Housing Developments in Asia: Qualities, Potential and Challenges.” Journal of Urban Design 22 (5): 613–636. https://doi.org/10.1080/13574809.2017.1311770.
- Chow, M. F., and M. F. A. Bakar. 2019. “Environmental Benefits of Green Roof to the Sustainable Urban Development: A Review.” GCEC 2017: Proceedings of the 1st Global Civil Engineering Conference 1, 1525–1541. Springer Singapore. https://doi.org/10.1007/978-981-10-8016-6_110.
- Cufoglu, A., M. Lohi, and C. Everiss. 2017. “Feature Weighted Clustering for User Profiling.” International Journal of Modeling, Simulation, and Scientific Computing 8 (4): 1750056. https://doi.org/10.1142/S1793962317500568.
- Dahmann, N., J. Wolch, P. Joassart-Marcelli, K. Reynolds, and M. Jerrett. 2010. “The Active City? Disparities in Provision of Urban Public Recreation Resources.” Health & Place 16 (3): 431–445. https://doi.org/10.1016/j.healthplace.2009.11.005.
- Dawes, J. 2008. “Do Data Characteristics Change According to the Number of Scale Points Used? An Experiment Using 5-Point, 7-Point and 10-Point Scales.” International Journal of Market Research 50 (1): 61–104. https://doi.org/10.1177/147078530805000106.
- Debbarma, J., and Y. Choi. 2022. “A Taxonomy of Green Governance: A Qualitative and Quantitative Analysis Towards Sustainable Development.” Sustainable Cities and Society 79:79. https://doi.org/10.1016/j.scs.2022.103693.
- Development and Reform Commission of Zhejiang Province. 2019. Pilot Work Plan for the Construction of Future Communities in Zhejiang Province. https://fzggw.zj.gov.cn/art/2019/3/25/art_1599545_34126877.html.
- Domrös, M., and G. Peng. 2012. “The Climate of China.” Springer Science & Business Media. https://doi.org/10.1007/978-3-642-73333-8_1.
- Dunnett, N., and N. Kingsbury. 2008. Planting Green Roofs and Living Walls[M]. Portland, OR: Timber press.
- Feng, J., K. Zhang, Z. Xu, C. Du, X. Tang, and L. Zhang. 2024. “Quantitative Study on Color Characteristics of Urban Park Landscapes Based on K-Means Clustering and SD. Method.” Earth Science Informatics 17 (2): 999–1012. https://doi.org/10.1007/s12145-024-01235-4.
- Fernandez-Canero, R., T. Emilsson, C. Fernandez-Barba, and M. A. Herrera Machuca. 2013. “Green Roof Systems: A Study of Public Attitudes and Preferences in Southern Spain.” Journal of Environmental Management 128:106–115. https://doi.org/10.1016/j.jenvman.2013.04.052.
- Francis, R. A., and J. Lorimer. 2011. “Urban Reconciliation Ecology: The Potential of Living Roofs and Walls.” Journal of Environmental Management 92 (6): 1429–1437. https://doi.org/10.1016/j.jenvman.2011.01.012.
- Getter, K. L., and D. B. Rowe. 2006. “The Role of Extensive Green Roofs in Sustainable Development.” Hort Science 41 (5): 1276–1285. https://doi.org/10.21273/HORTSCI.41.5.1276.
- Hallett, S., L. Hoagland, and E. Toner. 2016. “Urban Agriculture: Environmental, Economic, and Social Perspectives.” Horticultural Reviews 44:65–120. https://doi.org/10.1002/9781119281269.ch2.
- Hennig, C., M. Meila, F. Murtagh, and R. Rocci. 2015. Handbook of Cluster Analysis. Boca Raton, FL: CRC Press.
- Hernández-Moreno, Á., and S. Reyes-Paecke. 2018. “The Effects of Urban Expansion on Green Infrastructure Along an Extended Latitudinal Gradient (23 S–45 S) in Chile Over the Last Thirty Years.” Land Use Policy 79:725–733. https://doi.org/10.1016/j.landusepol.2018.09.008.
- He, B., and J. Zhu. 2018. “Constructing Community Gardens? Residents’ Attitude and Behaviour Towards Edible Landscapes in Emerging Urban Communities of China.” Urban Forestry & Urban Greening 34:154–165. https://doi.org/10.1016/j.ufug.2018.06.015.
- Ho, C.-H., V. Sasidharan, W. Elmendorf, F. K. Willits, A. Graefe, and G. Godbey. 2017. “Gender and Ethnic Variations in Urban Park Preferences, Visitation, and Perceived Benefits.” Journal of Leisure Research 37 (3): 281–306. https://doi.org/10.1080/00222216.2005.11950054.
- Hou, J., and D. Grohmann. 2018. “Integrating Community Gardens into Urban Parks: Lessons in Planning, Design and Partnership from Seattle.” Urban Forestry & Urban Greening 33:46–55. https://doi.org/10.1016/j.ufug.2018.05.007.
- IGRA. 2022. “Types of Green Roofs.” https://www.igra-world.com/types_of_green_roofs/.
- Jain, A. K. 2010. “Data Clustering: 50 Years Beyond K-Means.” Pattern Recognition Letters 31 (8): 651–666. https://doi.org/10.1016/j.patrec.2009.09.011.
- Jim, C. Y., and C. H. Ling. 2022. “Offering Green Roofs in a Compact City: Benefits and Landscape Preferences of Socio-Demographic Cohorts.” Applied Geography 145:102733. https://doi.org/10.1016/j.apgeog.2022.102733.
- Jungels, J., D. A. Rakow, S. B. Allred, and S. M. Skelly. 2013. “Attitudes and Aesthetic Reactions Toward Green Roofs in the Northeastern United States.” Landscape and Urban Planning 117:13–21. https://doi.org/10.1016/j.landurbplan.2013.04.013.
- Kana, K. 2012. “An Experiment in Urban Regeneration Using Culture and Art in Senba, Osaka’s Historic Urban Center, with a Focus on the Regeneration of Urban Space.” City, Culture & Society 3 (2): 151–163. https://doi.org/10.1016/j.ccs.2012.06.006.
- Kim, E., J. Jung, G. Hapsari, S. Kang, K. Kim, S. Yoon, M. Lee, M. Han, Y. Choi, and J. K. Choe. 2018. “Economic and Environmental Sustainability and Public Perceptions of Rooftop Farm versus Extensive Garden.” Building and Environment 146:206–215. https://doi.org/10.1016/j.buildenv.2018.09.046.
- Kosareo, L., and R. Ries. 2007. “Comparative Environmental Life Cycle Assessment of Green Roofs.” Building and Environment 42 (7): 2606–2613. https://doi.org/10.1016/j.buildenv.2006.06.019.
- Kural, R. 2010. “Changing Spaces for Sports.” Sport in Society 13 (2): 300–313. https://doi.org/10.1080/17430430903523002.
- Kwon, Y. S., B. K. Lee, and S. Y. Sohn. 2020. “Optimal Location-Allocation Model for the Installation of Rooftop Sports Facilities in Metropolitan Areas.” European Sport Management Quarterly 20 (2): 189–204. https://doi.org/10.1080/16184742.2019.1598454.
- Lee, J., and M. McCuskey Shepley. 2020. “Benefits of Solar Photovoltaic Systems for Low-Income Families in Social Housing of Korea: Renewable Energy Applications as Solutions to Energy Poverty.” Journal of Building Engineering 28:28. https://doi.org/10.1016/j.jobe.2019.101016.
- McNeill, L. H., M. W. Kreuter, and S. V. Subramanian. 2006. “Social Environment and Physical Activity: A Review of Concepts and Evidence.” Social Science & Medicine 63 (4): 1011–1022. https://doi.org/10.1016/j.socscimed.2006.03.012.
- Mishra, S. S., and D. Sakharle. 2022. “Design of Green Roof for an Energy Efficient University Campus.” Materials Today: Proceedings 65:1546–1550. https://doi.org/10.1016/j.matpr.2022.04.505.
- Morse, C., and J. Mudgett. 2017. “Longing for Landscape: Homesickness and Place Attachment Among Rural Out-Migrants in the 19th and 21st Centuries.” Journal of Rural Studies 50:95–103. https://doi.org/10.1016/j.jrurstud.2017.01.002.
- Nasr, J., J. Komisar, and H. de Zeeuw. 2017. “A Panorama of Rooftop Agriculture Types.” Rooftop Urban Agriculture: 9–29. https://doi.org/10.1007/978-3-319-57720-3_21.
- Oberndorfer, E., J. Lundholm, B. Bass, R. R. Coffman, H. Doshi, N. Dunnett, S. Gaffin, M. Köhler, K. K. Y. Liu, and B. Rowe. 2007. “Green Roofs As Urban Ecosystems: Ecological Structures, Functions, and Services.” BioScience 57 (10): 823–833. https://doi.org/10.1641/B571005.
- Office of the Leading Group of the State Council for the Seventh National Population Census. 2021. China Population Census Yearbook - 2020. https://www.stats.gov.cn/sj/pcsj/rkpc/7rp/zk/indexch.htm.
- Pan, S. Y., C. Cameron, M. DesMeules, H. Morrison, C. L. Craig, and X. Jiang. 2009. “Individual, Social, Environmental, and Physical Environmental Correlates with Physical Activity Among Canadians: A Cross-Sectional Study.” BMC Public Health 9 (1): 1–12. https://doi.org/10.1186/1471-2458-9-21.
- Parsons, V. L. 2014. “Stratified Sampling.” Wiley StatsRef: Statistics Reference Online: 1–11.
- Qian, Z. 2012. “Post-Reform Urban Restructuring in China: The Case of Hangzhou 1990-2010.” The Town Planning Review 83:431–455. https://doi.org/10.2307/41509862.
- Qin, X. 2012. “A Green Roof Test Bed for Stormwater Management and Reduction of Urban Heat Island Effect in Singapore.” British Journal of Environment and Climate Change 2:410–420. https://doi.org/10.9734/BJECC/2012/2704.
- Rahman, S. R. A., H. Ahmad, S. Mohammad, and M. S. F. Rosley. 2015. “Perception of Green Roof As a Tool for Urban Regeneration in a Commercial Environment: The Secret Garden, Malaysia.” Procedia-Social and Behavioral Sciences 170:128–136. https://doi.org/10.1016/j.sbspro.2015.01.022.
- Sardianou, E., and P. Genoudi. 2013. “Which Factors Affect the Willingness of Consumers to Adopt Renewable Energies?” Renewable Energy 57:1–4. https://doi.org/10.1016/j.renene.2013.01.031.
- Saris, W. E., and I. N. Gallhofer. 2014. Design, Evaluation, and Analysis of Questionnaires for Survey Research. Hoboken, NJ: John Wiley & Sons.
- Sattler, S., I. Zluwa, and D. Österreicher. 2020. “The “PV Rooftop garden”: Providing Recreational Green Roofs and Renewable Energy As a Multifunctional System within One Surface Area.” Applied Sciences 10 (5): 1791. https://doi.org/10.3390/app10051791.
- Scott, T. L., B. M. Masser, and N. A. Pachana. 2020. “Positive Aging Benefits of Home and Community Gardening Activities: Older Adults Report Enhanced Self-Esteem, Productive Endeavors, Social Engagement and Exercise.” SAGE Open Medicine 8:205031212090173. https://doi.org/10.1177/2050312120901732.
- Sherry, C. 2022. “Learning from the Dirt: Initiating University Food Gardens As a Cross-Disciplinary Tertiary Teaching Tool.” Journal of Outdoor and Environmental Education 25 (2): 199–217. https://doi.org/10.1007/s42322-022-00100-6.
- Sosnowska, M., A. Kępkowicz, I. Woźniak-Kostecka, H. Lipińska, and L. Renaudie. 2022. “Exploring the Potential for Development of Urban Horticulture in the 1960s Housing Estates. A Case Study of Lublin, Poland.” Urban Forestry & Urban Greening 75. https://doi.org/10.1016/j.ufug.2022.127689.
- Statistics, H. 2023. Hangzhou Economic Operation in the First Quarter of 2023. http://www.hangzhou.gov.cn/art/2023/4/23/art_1229243368_59078732.html.
- Stupariu, M.-S., S. A. Cushman, A.-I. Pleşoianu, I. Pătru-Stupariu, and C. Fürst. 2021. “Machine Learning in Landscape Ecological Analysis: A Review of Recent Approaches.” Landscape Ecology 37 (5): 1227–1250. https://doi.org/10.1007/s10980-021-01366-9.
- Taber, R. 2009. “Clustering (Xu, R. and Wunsch II, DC; 2009)[Book review].” IEEE Computational Intelligence Magazine 4 (3): 92–95. https://doi.org/10.1109/MCI.2009.933101.
- Taib, N., Z. Ali, A. Abdullah, S. Y. Foong, and R. Prihatmanti. 2019. “The Performance of Different Ornamental Plant Species in Transitional Spaces in Urban High-Rise Settings.” Urban Forestry & Urban Greening 43:43. https://doi.org/10.1016/j.ufug.2019.126393.
- Takebayashi, H., and M. Moriyama. 2007. “Surface Heat Budget on Green Roof and High Reflection Roof for Mitigation of Urban Heat Island.” Building and Environment 42 (8): 2971–2979. https://doi.org/10.1016/j.buildenv.2006.06.017.
- Tam, W. C. V. 2020. “From Flat Roof to Roof Garden: Comparing Five Different Approaches on Rooftop Design.” Doctoral dissertation, Michigan State University. Chapter 2.
- Teotónio, I., C. M. Silva, and C. O. Cruz. 2018. “Eco-Solutions for Urban Environments Regeneration: The Economic Value of Green Roofs.” Journal of Cleaner Production 199:121–135. https://doi.org/10.1016/j.jclepro.2018.07.084.
- Tian, Y., C. Y. Jim, and H. Wang. 2014. “Assessing the Landscape and Ecological Quality of Urban Green Spaces in a Compact City.” Landscape and Urban Planning 121:97–108. https://doi.org/10.1016/j.landurbplan.2013.10.001.
- Ureta, J., M. Motallebi, A. E. Scaroni, S. Lovelace, and J. C. Ureta. 2021. “Understanding the public’s Behavior in Adopting Green Stormwater Infrastructure.” Sustainable Cities and Society 69:69. https://doi.org/10.1016/j.scs.2021.102815.
- Wakefield, K. L., and J. J. Inman. 2003. “Situational Price Sensitivity: The Role of Consumption Occasion, Social Context and Income.” Journal of Retailing 79 (4): 199–212. https://doi.org/10.1016/j.jretai.2003.09.004.
- Wang, J. W., C. H. Poh, C. Y. T. Tan, V. N. Lee, A. Jain, and E. L. Webb. 2017. “Building Biodiversity: Drivers of Bird and Butterfly Diversity on Tropical Urban Roof Gardens.” Ecosphere 8 (9): 8. https://doi.org/10.1002/ecs2.1905.
- Wang, H., R. Wang, L. Fu, Q. Miao, and N. Li. 2022. “Activities-Centered Participatory Community Design: Shoupa Community Service Station Rooftop Renovation Plan.” In International Conference on Human-Computer Interaction, 242–255. Springer.
- Wang, Y., Y. M. Zhu, M. J. Yu, and J. Xu. 2020. “Quantitative Evaluation and Spatial Differentiation of Ecoenvironmental Livability in Zhejiang Province, China.” Journal of Mountain Science 17 (6): 1491–1508. https://doi.org/10.1007/s11629-019-5477-9.
- Wen, H., Y. Jin, and L. Zhang. 2017. “Spatial Heterogeneity in Implicit Housing Prices: Evidence from Hangzhou, China.” International Journal of Strategic Property Management 21 (1): 15–28. https://doi.org/10.3846/1648715X.2016.1247021.
- Wienke, B., and D. Jekauc. 2016. “A Qualitative Analysis of Emotional Facilitators in Exercise.” Frontiers in Psychology 7:1296. https://doi.org/10.3389/fpsyg.2016.01296.
- Williams, K. J. H., K. E. Lee, L. Sargent, K. A. Johnson, J. Rayner, C. Farrell, R. E. Miller, and N. S. G. Williams. 2019. “Appraising the Psychological Benefits of Green Roofs for City Residents and Workers.” Urban Forestry & Urban Greening 44:126399. https://doi.org/10.1016/j.ufug.2019.126399.
- Xiao, Y., E. C. Hui, and H. Wen. 2019. “Effects of Floor Level and Landscape Proximity on Housing Price: A Hedonic Analysis in Hangzhou, China.” Habitat International 87:11–26. https://doi.org/10.1016/j.habitatint.2019.03.008.
- Zanon, D., C. Doucouliagos, J. Hall, and L. Lockstone-Binney. 2013. “Constraints to Park Visitation: A Meta-Analysis of North American Studies.” Leisure Sciences 35 (5): 475–493. https://doi.org/10.1080/01490400.2013.831294.
- Zhang, L., H. Fukuda, and Z. Liu. 2019. “Households’ Willingness to Pay for Green Roof for Mitigating Heat Island Effects in Beijing (China).” Building and Environment 150:13–20. https://doi.org/10.1016/j.buildenv.2018.12.048.
- Zhejiang Urban Development Research Centre. 2021. The 15th Anniversary of Urbanisation: An Overview of Zhejiang’s Urbanisation Development. https://jst.zj.gov.cn/art/2021/12/7/art_1229129262_58928384.html.