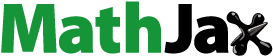
ABSTRACT
This study uses Shanghai to explore the effects of the COVID-19 pandemic on the spatial distribution patterns and driving factors of the urban catering industry. Through quantitative analysis of restaurant point-of-interest data and influencing factors in Shanghai’s main urban area from 2016 to 2022 using geographic information technology, machine learning, and spatial econometric models, this study predicts spatial changes in the catering industry. The number of restaurants in Shanghai’s main urban area continuously decreased from 2016 to 2022, with a significant pre-pandemic decline. The catering industry in this area has a significant spatial correlation in the spatial distribution, showing a pattern of “one core dominance with multiple cores coexisting,” with Jing’an and Huangpu Districts having the highest densities, which is expected to continue. Target markets remain a decisive factor in restaurant location selection post-COVID. Restaurant aggregation is most favorable when the population density is between 0 –50,000 people/km2, GDP is between 0–20 billion yuan/km2, housing prices are between 20,000 –130,000 yuan/m2, school density is between 0–20/km2, hospital density is between 0–10/km2, and attraction density is between 0–40/km2. Transportation conditions have no apparent threshold; the more developed the transportation, the more favorable the conditions for the catering industry. Post-COVID-19, areas with high housing prices and around tourist attractions are less likely to attract many restaurants. Areas around hospitals maintain restaurant aggregation, although this will decrease. Areas around schools will become popular for restaurants in the future.
1. Introduction
1.1. Research background
The catering industry is crucial to people’s livelihoods nationwide and occupies a significant position in the national economy as a vital sector that drives economic growth (Y. Li, Chiu, et al. Citation2022). According to previous surveys, in 2019 China’s catering industry reached 482.5 billion yuan in revenue, with a year-on-year growth rate of 9.1%, surpassing the overall consumer market growth rate by 1.1 percentage points. However, the COVID-19 outbreak at the end of 2019 had a profound impact on the consumption economy, as represented by the catering industry. In response to the call for COVID-19 prevention and control, most consumer entertainment venues and catering businesses in Shanghai ceased operations. Additionally, measures such as restricting population movement, closing communities, and suspending public activities inflicted comprehensive damage on Shanghai’s catering industry (Shen Citation2020). As an integral component of China’s tertiary industry, the catering sector is increasingly becoming a primary focus of urban planning and management against the backdrop of rapid urban development. Unlike other service sectors, the catering industry relies heavily on population mobility and aggregation. Therefore, studying the spatiotemporal pattern changes in this industry can reflect how population mobility resulting from a public health crisis affects urban spatiotemporal patterns. Thus, this research contributes to optimizing urban emergency management and policies, guiding industry upgrades, and enhancing resilience to risks.
Furthermore, restaurant openings and closures directly reflect the spatiotemporal transformation of urban functions, such as consumption, employment, and transportation. Investigating restaurant redistribution can provide insight into how cities undergo functional restructuring when affected by a public health crisis, thereby helping to expand urban spatiotemporal theories.
Therefore, this study focuses on the main urban area of Shanghai and utilizes web scraping techniques to collect restaurant point-of-interest (POI) data from the Amap website for 2016, 2018, 2020, and 2022. The evolving characteristics of the spatial patterns of the catering industry before and after COVID-19 are analyzed by integrating geographic information technology, machine learning, and spatial econometric models. Through an in-depth investigation of the changing patterns of factors influencing the spatial distribution of the catering industry, this study aims to provide a better understanding of the impact of COVID-19 on urban catering and the evolving mechanisms of the influence of public health crises on commercial spatial patterns in megacities. This research enriches the disciplines of urban and industrial geography by providing policy references for site selection, planning, and layout adjustments of restaurants in the post-COVID era.
1.2. Literature review
1.2.1. Public health crisis and urban functionality
Public health crises, such as outbreaks of infectious diseases or pandemics, profoundly affect urban functionality. Many studies have explored the effects of these events on urban economies, social life, and spatial arrangements. Sadique et al. (Citation2007) found that a major influenza pandemic could lead to an approximately 10% loss in the GDP of the United Kingdom. Siu and Wong (Citation2004) estimated that the economic losses caused by the 2003 SARS outbreak in Hong Kong amounted to as much as 6.7 billion USD. Restricted mobility, business closures, and decreased consumer demand are among the primary causes of economic losses Boissay and Rungcharoenkitkul (Citation2020). Consequently, studies are increasingly proposing strategies for strengthening public health considerations in urban planning. Acuto et al. (Citation2020) advocated integrating the concept of “healthy cities” into future urban planning, prioritizing public health needs in areas such as housing, environment, and transportation. Pak et al. (Citation2020) suggested using digital twin technology to simulate urban systems, providing decision support for evaluating and improving existing infrastructure and planning. Public health crises have also resulted in changes to urban spatial arrangements. Matsenko et al. (Citation2021) posited that COVID-19 would accelerate the shift in the restaurant industry towards suburban areas. The 2003 SARS outbreak led to a certain degree of commercial activity relocation in Hong Kong, with some remote areas (e.g., Tuen Mun and Sha Tin) experiencing thriving business scenes Carpio et al. (Citation2021). During the MERS outbreak, Seoul, South Korea, strengthened the planning and layout of medical facilities, increased the number of hospitals, and established emergency medical centers in various regions to promptly address public health emergencies. Additionally, the government provided dedicated transportation services to ensure the rapid dispatch and transportation of medical supplies and personnel (Moon and Han Citation2016).
1.2.2. COVID-19 and the catering industry
The COVID-19 pandemic has significantly affected the global restaurant industry, with existing research focusing on analyzing the actual effects and underlying causes of these changes. First, several studies have reported how the pandemic severely damaged the restaurant industry. Qiu et al. (Citation2020) used big data analysis to discover a sharp decline in revenue and widespread closure of restaurants in major Chinese cities during the COVID-19 pandemic. Baek et al. (Citation2021) reported a 27% decrease in restaurant revenue in the United States in 2020 compared with pre-COVID-19 levels. Field surveys by (Rizou et al. Citation2020) showed that 83% of restaurants in Greece experienced significant losses owing to the COVID-19 pandemic. Second, some studies explored the root causes of the effects of the pandemic on the restaurant industry. The industry relies heavily on personnel mobility and aggregation, whereas containment measures such as gathering bans and mobility restrictions contradict its operations (Brett-Ribeiro, 2020). Restaurant production and supply chain characteristics make the industry more vulnerable to the effects of the COVID-19 pandemic (Hu et al., 2021). Decreased consumer willingness and confidence to dine out during the pandemic are also significant contributing factors He and Harris (Citation2020). Third, various countries worldwide implemented corresponding measures in response to the challenges the restaurant industry faced during the pandemic. For instance, cities such as New York and San Francisco in the United States have allowed restaurants to set up tables on the streets to reduce the risk of indoor dining Mueller et al. (Citation2021). Melbourne, Australia, introduced the “City Recovery” plan, providing financial support and street renovations for the restaurant industry (Stobart and Duckett Citation2022). Rome, Italy, restricted vehicle access in the city center to provide more space for walking and cycling while supporting the development of the restaurant industry (Remuzzi and Remuzzi Citation2020). Toronto, Canada, launched the “Support Local” initiative, encouraging collaboration between restaurants and local suppliers to promote the development of local supply chains (Vaz Citation2021). The Singaporean government introduced “Safe Management Measures” to regulate the restaurant industry, including maintaining social distancing and enhancing hygiene measures Wong et al. (Citation2024). Furthermore, previous studies on coping strategies during the COVID-19 pandemic have primarily discussed three aspects: temporary emergency policies, such as personnel isolation and mobility restrictions (L. Wang, Lin, and Wong Citation2020); medium to long-term policies, such as establishing a more flexible and effective public health monitoring system (Hoernke et al. Citation2021); and strengthening public space management from the perspective of urban design (Carmona Citation2021). Song, Yeon, and Lee (Citation2021) proposed suggestions for transformation, upgrading, and reshaping layouts. Brizek et al. (Citation2021) advocated for restaurants to accelerate digital transformation and develop new models such as takeout and online ordering. Ardar (2022) Jeong et al. (Citation2022) proposed optimizing restaurant layouts and reducing personnel contact through automation and robotics technology.
1.2.3. Geospatial big data and urban structure
Spatial pattern analysis is of paramount importance in various fields, such as geography, urban planning, and environmental science. The stand-outs among the commonly used methods are spatial econometrics, geographic information systems (GIS), and machine learning.
First, spatial econometrics has significant advantages for spatial pattern analysis. It helps examine the spatial relationships between data points, including spatial autocorrelation, heterogeneity, and spatial lag effects. Spatial econometric models reveal interactions among spatial units to identify clustering patterns and dispersion phenomena, thereby enhancing our understanding of the mechanisms underlying spatial pattern formation and evolution. For example, a spatial autocorrelation analysis can help researchers uncover spatial clustering phenomena in urban development and provide scientific insights for urban planning and management. Neise (Neise, Verfürth, and Franz Citation2021) estimates the linear function of firm factors using a binary logistic regression model to determine whether firms have crisis resilience. M. Wu et al. (Citation2021) studied the influence of regional factors on restaurant distribution, birth, and demise by applying four technical regression models based on 2015 and 2017 POI data from Beijing. Tian et al. (Citation2021) analyzed the characteristics of urban differences and influencing factors of seafood restaurants using big data from 332 cities in China, employing variation coefficients, geographical concentration indices, bivariate Moran’s I, field strength models, and spatial econometric models.
Second, machine learning has become an increasingly prominent field for identifying factors that influence urban morphology and spatial heterogeneity. This approach has been applied in various directions as follows:
Utilizing machine algorithms, combined with diverse data sources such as census, mobile signaling, and social media data, to predict urban population distribution patterns (Gómez et al. Citation2020, Bao et al. Citation2022, Malki et al. Citation2020).
Employing classification algorithms such as support vector machines and random forest models to analyze remote sensing image and GIS data and identify urban land use types and land change patterns (Jamali Citation2021, Ahmed, N, and N Citation2023).
Using machine learning algorithms such as time series analysis, regression models, and neural networks to predict traffic flow in different periods (K. Wu et al. Citation2023, Yuan, Wang, and Yang Citation2021).
Employing techniques such as clustering analysis, association rule mining, and predictive modeling to analyze urban residents’ consumption behaviors, social networks, and economic activity characteristics (Furuhashi and Nakaya Citation2023, Taherdoost Citation2023).
Utilizing methods such as image classification, object detection, and feature extraction to identify the features and types of urban landscapes, thereby contributing to the preservation of cultural heritage and historic buildings (T. Huang et al. Citation2023).
Finally, GIS technology offers unique advantages for spatial pattern analysis by efficiently collecting, storing, managing, and analyzing geographic information data to provide powerful tools and support for spatial analysis. For example, creating spatial distribution maps, heatmaps, and interpolation maps intuitively displays the distribution characteristics of spatial patterns. In addition, spatial overlay operations and spatial buffer analysis can further explore the correlations between points of spatial data (Muller and Inman Citation1994; Prayag, Landré, and Ryan Citation2012). Walter (Citation2022) (Walter, Müller, and Långvall Citation2022) conducted a spatial analysis of the locations of gourmet restaurants in Sweden between 2005 and 2016 using GIS. Ponce-Lopez (Carpio, Ponce-Lopez, and Lozano-García Citation2021) utilized traffic and POI data from Google Place to identify and characterize popular nonwork destinations through grid cell clustering, logarithmic natural number testing, and a DBSCAN algorithm to analyze the geometric space and variable density of POIs.
However, using only individual research methods to study urban issues has limitations. Spatial econometrics often faces challenges such as spatial heterogeneity and autocorrelation in model parameter estimation and diagnostic processes, requiring corresponding adjustments and corrections. GIS technology encounters issues related to data quality and processing, such as data source uncertainty and spatial data matching, which may affect the accuracy of analysis results. Machine learning models require a large amount of high-quality data for training and validation; however, urban data is incomplete, missing, or inaccurate, which may affect model performance and results. Furthermore, while machine learning models have strong predictive capabilities, they cannot explain the reasons and mechanisms behind the model’s predicted results. Therefore, this study integrates the above methods by utilizing machine learning algorithms to identify the characteristics and patterns of the restaurant industry in the main urban area of Shanghai before and after the COVID-19 pandemic. Geographic information technology is used for spatial data visualization and analysis, while spatial econometric models are applied to explain the spatial dependence between urban data. This comprehensive approach aims to aid in forecasting and planning for future development in the restaurant industry during the post-COVID era in anticipation of potential future public health crises.
2. Methods and data sources
2.1. Study area and research design
Shanghai, one of China’s four municipalities directly under the central government, stands as a crucial component of the Yangtze River Delta urban economic cluster. Boasting a robust economic foundation, expansive market scale, and well-rounded industrial structure, Shanghai sees a relatively high proportion of tertiary industries. As a nationwide hub for commerce and population, the central urban area of Shanghai encountered significant impacts and changes during the COVID-19 pandemic. Therefore, examining the spatial distribution changes in the catering industry within this region can provide a better understanding of the influence of public health crises on urban commercial spaces. The main urban area of Shanghai comprises Huangpu District, Xuhui District, Jing’an District, Changning District, Yangpu District, Hongkou District, and Putuo District, covering a total area of approximately 289.44 square kilometers. As Zhabei District was merged into Jing’an District by the end of 2015, it is not separately listed in this study and is amalgamated into Jing’an District .
This study’s main objective is to analyze the evolving characteristics of the spatial distribution patterns of restaurants in the central urban area of Shanghai before and after the COVID-19 pandemic, as well as the changes in the driving factors. Therefore, the following hypotheses () are proposed:
H1:
The number of restaurants increased significantly before the COVID-19 pandemic, owing to the booming development of the food delivery market but decreased substantially after the of COVID-19 outbreak.
H2:
In the post-COVID era, the catering industry in the main urban area of Shanghai will continue to maintain a layout characterized by a core in the “Bund and surrounding commercial districts” and outward radial distribution.
H3:
In the post-COVID era, GDP production value will become the most important factor in determining the location of restaurants. The higher the GDP production value, the more restaurants there will be. In addition, lower housing prices will lead to a higher number of restaurants.
H4:
In the post-COVID era, subway stations will become the most influential transportation factor, affecting the spatial distribution of restaurants.
H5:
In the post-COVID era, areas surrounding hospitals will attract more restaurants, whereas areas near tourist attractions will become less popular choices for restaurant locations .
2.2. Methods
2.2.1. Kernel density
The Kernel Density Analysis method is used to visualize the quantity of POIs within individual measurement areas and the degree of clustering of different points of interest within these areas. It involves performing a search within a circular area with a fixed radius centered around a particular grid cell to calculate the cumulative density value for each grid cell (Yang et al. Citation2019).
represents the kernel density value,
is the search radius,
represents the coordinates of point
within the search radius,
is the number of point features within the search radius, and
represents the coordinates of the center of the raster grid cell being calculated.
2.2.2. Standard deviation ellipse
The standard deviation ellipse method can identify the dispersion and distribution orientation of point features. By analyzing the area of the ellipse curve, the standard distances along the x-axis and y-axis, and the rotation angle, one can observe the central tendency, clustering degree, and directional trends of the research object. The major axis represents the direction of the most frequent spatial distribution, while the minor axis represents the direction of the least frequent spatial distribution. A smaller ellipse area indicates higher clustering, whereas a larger area suggests lower clustering (Duman et al. Citation2023).
xi and yi represent the coordinates of data point i, is the mean center of the data distribution, and n equals the total number of data points.
2.2.3. Spatial autocorrelation analysis
Spatial autocorrelation analysis can explain the structural morphology of point features in space and test the spatial correlation between neighborhoods, i.e., whether there is spatial clustering. Spatial autocorrelation includes two components: global autocorrelation and local autocorrelation. Global spatial autocorrelation measures the degree of spatial dependence in the distribution of restaurants within the main urban area of Shanghai and often uses Moran’s I as a measurement index. Local spatial autocorrelation describes the similarity between a particular area and its neighbors, indicating the extent to which each local unit conforms to the overall trend (including direction and magnitude) and reflects spatial heterogeneity. Local Moran’s I is commonly used to represent this (Siu and Wong Citation2004).
The formula for calculating global autocorrelation Moran’s I is as follows:
In this context, I represents the Moran’s I index, where a value greater than 0 indicates positive spatial autocorrelation, signifying clustering of point features within the neighborhood, while a value less than 0 indicates negative spatial autocorrelation. n represents the number of point features in the spatial domain, xi represents the attribute values of spatial units, xj represents the mean value of all attribute values, and Wij represents the spatial weighting values.
Moran’s I:
Local Moran’s I:
2.2.4. Gradient Boosting Decision Tree (GBDT)
GBDT is a method that uses decision trees as base models and iteratively improves predictions by minimizing the loss function through gradient boosting. Compared to traditional generalized linear regression models, the GBDT model, as a non-parametric model, can estimate nonlinear relationships with irregular shapes within a single model. It more accurately characterizes the multivariate linear impact of independent variables on the dependent variable and can handle multicollinearity issues that are difficult to address in linear regression. Additionally, the GBDT model has the advantage of flexibly handling missing values and outliers. Therefore, based on these features, this study utilizes the GBDT model to estimate the nonlinear effects and threshold effects of population, economy, transportation, and environmental variables on restaurant density, determining the rational configuration of the catering industry’s supporting environment, and providing a methodological basis for fine-grained planning policy evaluation (Huan et al. Citation2023; C. Li, Zhao, and Hou Citation2023).
Initialize model:
For each round::
Calculate the negative gradient:
Fit a regression tree to fit the negative gradient:
Update the model:
L is the loss function, F(x) is the current model output, h(x) is the regression tree, v is the learning rate, M is the number of iterations, and N is the number of samples.
2.2.5. Spatial econometric models
When observed values in a particular region show a correlation with the observed values in other regions, it is necessary to introduce the interaction effect between regions into the model. This is achieved by adjusting the general regression model using a spatial weight matrix (Yang, C et al., 2023). Given the spatial heterogeneity and clustering of restaurant spatial distribution, this study compares and selects the optimal model from Ordinary Least Square (OLS), Spatial Lag Model (SLM), and Spatial Error Model (SEM) within spatial regression models. Subsequently, it conducts regression analysis on regional factors that affect the concentration of the restaurant industry.
Using the fishnet tool in ArcGIS, a 1000 m *1000 m spatial grid is constructed, with each grid unit considered as a unit of analysis. Independent variables, such as restaurant density within each 1 km2, and dependent variables representing various influencing factors, are linked to these grid units through a spatial join operation. Geoda software is then used for spatial regression analysis, producing a correlation coefficient table between restaurant distribution density and the various influencing factors.
(1) OLS (Ordinary Least Square):
This model analyzes the correlation between two or more variables to determine the degree of closeness between independent and dependent variables using numerical modeling or fitting methods. OLS is the most basic and commonly used regression model (Mohammad et al. Citation2023). Its general form is as follows:
Where: y is the dependent variable. x is the independent variable. β₀ is the intercept parameter. β₁ is the slope parameter. ε is the random error vector.
(2) Spatial Lag Model (SLM)
The Spatial Lag Model (SLM) is used to describe the spatial correlation between dependent variables, specifically investigating whether the values of a dependent variable in one geographical area influence the values of the same variable in neighboring areas through a spatial contagion mechanism (Shu et al. Citation2023). The general form of this model is as follows:
Where: y is the dependent variable. ρ is the spatial regression coefficient. W is the n*n spatial weight matrix. Wy is the spatial lag of the dependent variable based on the spatial weight matrix W. x is the n*k exogenous explanatory variable matrix. β represents the impact of explanatory variables on the dependent variable. ε is the random error vector.
Spatial Error Model (SEM)
This model assumes that the errors of the model are spatially correlated, typically taking the general form:
y represents the dependent variable. x is the n * k matrix of exogenous explanatory variables. β reflects the coefficients that indicate the impact of explanatory variables on the dependent variable. ε is the vector of random errors. λ represents the spatial error coefficients for the dependent variable vector. W is the n * n spatial weight matrix. μ is the vector of normally distributed random errors (Yoo, Browning, and Minesinger Citation2018).
2.3. Data sources and preprocessing
2.3.1. Data sources
POI data include information such as facility name, category, address, latitude, and longitude, reflecting the spatial distribution of geographic elements, with characteristics of rich data volume and accurate geographic information. The POI data collected in this study all come from Gaode Maps(https://www.amap.com.) (Liu et al. Citation2023), collected before COVID-19 (2016 and 2018) and after COVID-19 (2020 and 2022). By running the source code in Python software to obtain the location coordinates of POI data, the obtained location information is then imported into ArcGIS software to extract the distribution of restaurant locations in point form. Through data cleaning and screening, incomplete, duplicate, or inaccurately located data were removed. A total of 60,668 valid restaurant POI data were obtained in 2016, 50626 in 2018, 43941 in 2020, and 40,630 in 2022 . The other POI data cover Transportation Facility Services, Retail Services, Educational and Cultural Services, Healthcare Services, Mixed-use Development, and Attraction as primary categories. Traffic and environmental POI data are sourced from Gaode Maps, population data are from the WorldPop project (https://www.worldpop.org.) (H. Huang et al. Citation2024), house price data are from historical data on Beike (https://m.bj.ke.com/.) (A. Wang, Hussain, and Yan Citation2024), and GDP spatial distribution grid data and road network data are sourced from the Data Center for Resources and Environmental Sciences, Chinese Academy of Sciences (http://www.resdc.cn/.) (Xu et al. Citation2022).
Figure 3. Spatial distribution of restaurants in the main urban area of Shanghai in 2016, 2018, 2020, and 2022.
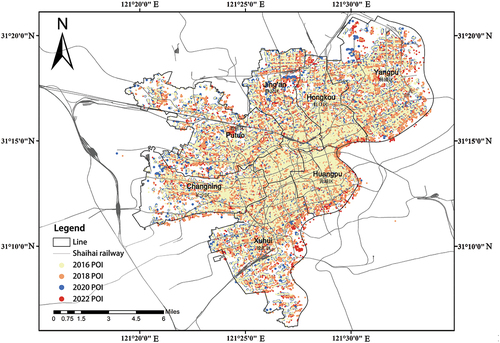
Referencing existing methods for selecting influencing factors and considering the spatial distribution characteristics of restaurant spaces in Shanghai’s main urban area, this study selects 13 independent variables from the four dimensions of population, economy, transportation, and environment, for correlation analysis with restaurant density. Considering the modifiable areal unit problem (MAUP), the fishnet tool in ArcGIS is used to construct square grids with a side length of 1 km as the analysis unit. Using spatial correlation and other methods, the number of restaurants (dependent variable Y) and other indicators (independent variables X1–X13) within each grid are counted to better represent the heterogeneity within the space. The specific grid quantification method is shown in the and .
Table 1. Variable design for restaurant spatial distribution regression model.
2.3.2. Descriptive statistics of the data
The descriptive statistics of the data indicate consistent frequencies without any significant outliers. The table presents the descriptive statistical analysis results of all driving factors after preprocessing. To verify the existence of linear relationships between variables and the absence of multicollinearity, Pearson correlation tests and multicollinearity diagnostics were conducted in this study. The results indicate that the Pearson correlation coefficients are non-zero , and the Variance Inflation Factor (VIF) values for the independent variables are all less than 10. This suggests that there are linear relationships between the independent variables and no multicollinearity issues, making them suitable for use in spatial regression models .
Table 2. Descriptive statistics of the data.
3. Results and discussion
3.1. Evolution characteristics of restaurant spatial patterns in shanghai’s central urban area
3.1.1. Changes in restaurant quantity
The graph indicates a continuous decline in the number of restaurants in the central urban area of Shanghai from 2016 to 2022 , representing a decrease of approximately one-third. The period before the COVID-19 pandemic saw the highest number of restaurant closures, marking the most severe period in nearly a decade, with a relatively smaller decline observed after the pandemic. Therefore, while the COVID-19 outbreak hindered the development of the catering industry, it was not the primary reason for restaurant closures. At the regional level, the trends in changes in the number of restaurants across districts from 2016 to 2022 were consistent with the overall trend, which we attribute to two main factors. First, the rise of the online food delivery industry significantly affected the offline catering market (Thompson Citation2010). The development of online food delivery services in Shanghai experienced a boom between 2015 and 2016, with major delivery platforms (e.g., Meituan and Ele.me) expanding rapidly. Subsequently, the market became saturated, and the fierce competition created by online food delivery services led to numerous offline restaurants closing. Second, the implementation of Shanghai’s shantytown renovation projects contributed to changes in commercial land use. From 2015 to 2018, Shanghai witnessed the most rapid progress in shantytown renovation projects, with many old housing units being renovated or demolished. This led to changes in commercial land use, thereby restricting restaurant expansion (X. Li, Kleinhans, and van Ham Citation2018). The number of restaurants did not decrease significantly after the COVID-19 outbreak for two reasons. First, with the relaxation of COVID-19 control measures and economic recovery, the public demand for catering services gradually recovered, prompting many restaurants to reopen. Second, the Shanghai municipal government implemented a series of policies, including financial subsidies, rent reductions, and tax incentives to support the development of the catering industry following the COVID-19 outbreak (Zhou et al. Citation2023). In summary, while the pandemic affected the catering industry, its extent was far less significant than the effects of urban land planning adjustments and online food delivery platforms.
3.1.2. Changes in restaurant kernel density
After the outbreak of COVID-19, there was no significant change in the spatial distribution of restaurants in the central urban area of Shanghai, which consistently exhibited a pattern of “single core surrounded by multiple cores.” The main core areas were concentrated in the southern parts of Huangpu and Jing’an districts, with the Bund and surrounding commercial areas serving as the main core areas, highly contiguous with Nanjing West Road, Jing’an Temple, and Dapuqiao. Sub-core areas emerged in various districts, such as Xujiahui and Huaihai Middle Road in the northern part of Xuhui District, Huayang Road and Tianshan Road in the eastern part of Changning District, Changshou Road and North Zhongshan Road in the eastern and southern parts of Putuo District, Sichuan North Road and Jiaxing Road in the southeastern part of Hongkou District, and the Wujiaochang area in the central part of Yangpu District. After the COVID-19 outbreak, the phenomenon of contiguous restaurants in Huangpu District became more pronounced. Restaurants in Xuhui District gradually became sparser from north to south, and the restaurant cluster area in Changning District shifted from the east side of the Beixinjing Street to the west side. In Jing’an District, restaurant cluster areas appeared for the first time in the southern parts of Pengpu Town and Daning Street. In Putuo District, restaurant cluster areas shifted from east to west. There were no significant changes in Hongkou and Yangpu districts, but there was a trend of outward expansion .
3.1.3. Spatial autocorrelation and standard deviation ellipse results
The results of spatial autocorrelation indicate a significant positive spatial correlation in the spatial distribution of restaurants in the central urban area of Shanghai from 2016 to 2022. The Moran’s I indices for each year were 0.806, 0.8175, 0.8200, and 0.8159, respectively . All of these values passed the significance test at the 0.01 level, with standardized Z-values exceeding the critical values at the 0.05 significance level. Scatter plots of Moran’s indices and LISA cluster maps revealed no significant changes in the distribution of restaurant hotspots and cold spots before and after COVID-19. The hotspots consistently clustered within the primary agglomeration core centered around Nanjing East Road, with the radiation range gradually expanding over time. As time progressed, the agglomeration effect in the restaurant industry became more pronounced, gradually forming multiple secondary agglomeration centers in financial centers such as Lujiazui Financial and Trade Zone and commercial districts like Xujiahui .
Figure 8. Hotspot and Coldspot Analysis of Restaurants’ Spatial Distribution in the Central Urban Area of Shanghai in 2016, 2018, 2020, and 2022.
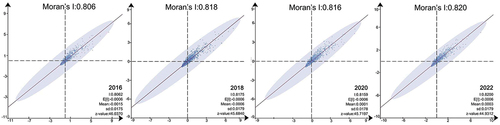
Figure 9. (a)(b)(c)(d) Moran’s I Index of Restaurants in the Central Urban Area of Shanghai in 2016, 2018, 2020, and 2022.
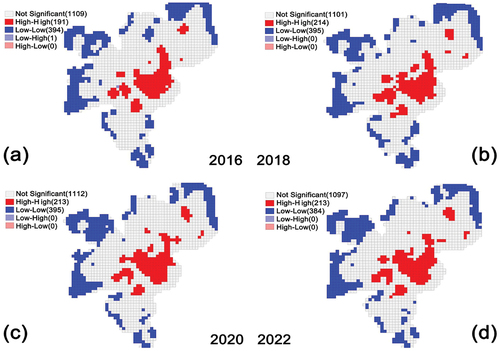
The spatial distribution of restaurants exhibits a noticeable directional pattern, mainly oriented in the southwest to northeast direction, with significant disparities between the long and short axes, indicating a strong spatial distribution orientation. This distribution pattern aligns well with the planned distribution of urban commercial centers and activity centers outlined in the “Shanghai Commercial Store Layout Plan (2013–2020).” There was no displacement or alteration observed after COVID-19. The values of kurtosis for restaurant distribution in different years indicate that 2018 had the highest kurtosis value, suggesting a stronger directional tendency and dispersion. In contrast, the years 2016, 2020, and 2022 returned to a stable state. Therefore, it can be anticipated that as the economy gradually recovers, the urban functions of Shanghai will quickly rebound and maintain the existing layout and format and .
Figure 10. (a)(b) Directional Distribution Analysis of Restaurants in the Central Urban Area of Shanghai in 2016, 2018, 2020 2022.
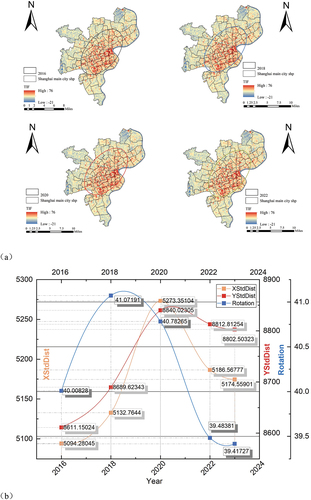
Table 3. Standard deviation elliptical attribute analysis of restaurants in the central urban area of Shanghai in 2016, 2018, 2020, and 2022.
3.2. Changes in the contribution rank and threshold effect of driving factors for the spatial distribution of catering industry in shanghai’s main urban area
3.2.1. Changes in the contribution rank of driving factors
GBDT is a typical machine learning method. In this study, relationship dependency plots (PDP) were created using packages such as scikit-learn, matplotlib, and statsmodels in Python to illustrate the nonlinear relationships between variables. By estimating the marginal effects of each independent variable on the fitted results and based on the distribution of fitted values for all individual samples, marginal variations of fitted intervals with two times standard errors were plotted for each independent variable. To prevent overfitting, this study also employed a 5-fold cross-validation method for validation assessment. During the parameter tuning process, the learning rate was set to 0.1, the number of decision trees was set to 100, and the tree depth was set to 3 based on the minimum Root Mean Square Error (RMSE). The iteration was set to 100. At this point, the mean square error of the training set and testing set before COVID-19 was 863.7 and 455.1, respectively, with an R-squared value of 0.7702. After COVID-19, the mean square error of the training set and testing set were 607.6 and 184.9, respectively, with an R-squared value of 0.776. This study also compared the regression fit of the GBDT and Ordinary Least Squares (OLS) models. The final R-squared values for the OLS regression were 0.757 and 0.722, respectively, which were lower than those of the GBDT model, indicating a higher fit of the GBDT model compared to the OLS model .
Table 4. Importance of the influencing factors on the spatial distribution of restaurants.
The results of the GBDT model show that the contribution rank of influencing factors before the outbreak of COVID-19 is as follows: mall(x8) > residential(x12) > office(x9) > hospital(x11) > attraction(x13) > gdp(x2) > parking(x6) > subway(x5) > road(x7) > bus(x4) > population(x1) > house price(x3) > school(x10) . After the outbreak of COVID-19, the contribution rank of influencing factors changes to: parking(x6) > mall(x8) > office(x9) > residential(x12) > road(x7) > hospital(x11) > gdp(x2) > school(x10) > population(x1) > house price(x3) > bus(x4) > subway(x5) > attraction(x13) .
The importance ranking results show that, after the COVID-19 outbreak, the target market factors continued to be the most important factors influencing the spatial distribution of restaurants. This indicates that shopping malls, office buildings, and residential communities with sufficient consumer groups will always be popular areas for restaurant location selection during post-COVID-19. These areas are characterized by high foot traffic, a broad potential customer base, convenient transportation, and strong social interactions, making them prone to restaurant clustering. As COVID-19 ended and the economy recovered, these areas were among the first to see a rebound in dining, indicating that restaurants in these areas have better resilience and recovery capabilities.
With the COVID-19 outbreak, the importance of certain factors has significantly increased, such as parking (x6), school proximity (x10), and road density (x7) First, regarding parking (x6), following the COVID-19 outbreak, parking facilities have emerged as the most important driving factor. This shift is attributed to changes in people’s travel behavior due to COVID-19, with individuals preferring private over public transportation to reduce contact with others. Consequently, parking facilities have become the preferred choice for both consumers and restaurant owners. Second, for school proximity (x10), although schools are not the primary determinant of restaurant spatial distribution, their importance has increased post-COVID-19. This indicates that restaurants near schools have stronger resilience and recovery capabilities than those in other areas. This could be because of the relatively lower rental costs in areas surrounding schools, which reduces operational expenses for restaurants. Additionally, schools provide a more stable and consistent customer base with higher foot traffic, thereby enabling restaurants in these areas to quickly reopen after the COVID-19 pandemic ended. Third, regarding road density (x7), during the COVID-19 period, the restaurant industry faced severe challenges. Areas with high road network density offer more commercial opportunities and potential customers. Therefore, restaurant owners under business survival pressures were more inclined to choose these areas to enhance their chances of survival.
With the COVID-19 outbreak, the importance of certain factors has diminished, such as attractions (x13), subway proximity (x5), and hospital proximity (x11). First, for attractions (x13), their importance has shown the largest decline because it is the least important factor among all driving factors in the post-COVID-19 era. The COVID-19 outbreak has strongly affected the tourism industry, with many attractions closing or limiting the number of visitors. Consequently, tourist traffic has significantly reduced, leading to the closure of numerous restaurants around attractions post-COVID-19. Second, regarding subway proximity (x5), areas near subway stations often have high rental costs and are dominated by fast-food outlets with lower average consumer spending. Therefore, sufficient foot traffic is essential to ensure smooth business operations in these areas. However, following the COVID-19 outbreak, policy regulations and consumer fears about infectious diseases led to a sharp decline in foot traffic. Consequently, many businesses have closed because of their inability to afford the operational costs. In addition, restaurants near subway stations often attract random consumption, leading to lower customer loyalty, which contributes to the closure of many restaurants. Three, concerning hospital proximity (x11), the importance of hospitals has decreased post-COVID-19, partly because of the reduced medical demand and partly because of changes in restaurant operators’ safety awareness. Through the experience of the COVID-19 outbreak, restaurant operators have realized that, while areas around hospitals may have sufficient foot traffic, they are not as resilient to public health crises as shopping malls or residential areas. Instead, they are likely to become areas of heightened control and risk during public health crises. Consequently, restaurant operators are more cautious when choosing locations near hospitals.
3.2.2. Threshold values of influencing factors on restaurant spatial distribution in Shanghai’s main urban area
The dependency plots (PDP) of the GBDT model results illustrate the non-linear relationship between restaurant density and independent variables, while controlling for other variables. The results indicate that factors such as population, economy, transportation, and environment exhibit non-linear relationships with restaurant density, accompanied by threshold effects .
Figure 13. (a) The dependency plots (PDP) of the GBDT model results before the outbreak of COVID-19; (b) The dependency plots (PDP) of the GBDT model results after the outbreak of COVID-19.
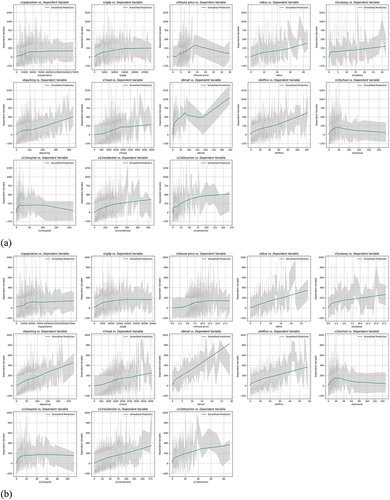
Figure 14. (a) Scatter plots for the years 2016 and 2018 before the COVID-19; (b) Scatter plots for the years 2020 and 2022 after the COVID-19.
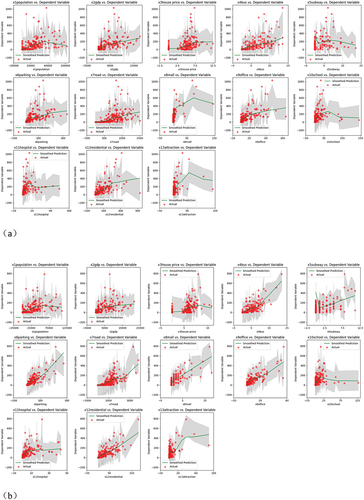
Population Variable: There exists a non-linear positive correlation and threshold effect between population and restaurant density. Based on the average effect of the model results, an increase in population density within the range of 0–50000 people/km2 corresponds to an increase in restaurant density. However, beyond this range, further increases in population density no longer have a significant impact on restaurant numbers. This suggests that population density within a certain range effectively facilitates the expansion of restaurants. Once the population density exceeds 50,000 people/km2, the number of restaurants reaches saturation point relative to market demand. Further increases in population do not significantly increase the number of restaurants. At this stage, factors such as market competition, land costs, and policy restrictions exert a greater influence on restaurant location selection .
Economic Indicators: (1) GDP: There exists a non-linear positive correlation between GDP and restaurant density. Pre-COVID-19, an increase in GDP within the range of 0–15 billion yuan/km2 corresponds to an increase in restaurant density. Post-COVID-19, the threshold range expands to 0–20 billion yuan/km2. Beyond this range, further increases in GDP no longer have a significant impact on restaurant numbers. The expansion of the threshold range indicates that the influence of the economy on restaurant density growth becomes more extensive, and the sensitivity of restaurant density growth to economic activities increases. (2) House Price: Within the range of 20,000–130000 yuan/m2, restaurant density significantly increases with rising house prices. Pre-COVID-19, when house prices exceed 130,000 yuan/m2, the number of restaurants significantly decreases. Post-COVID-19, when house prices exceed 130,000 yuan/m2, the number of restaurants no longer decreases with rising house prices; instead, it remains relatively stable. This indicates a weakened sensitivity of restaurant density to house prices after COVID-19, and restaurants in high-priced areas still possess resilience to risks and can adapt to market changes and uncertainties. This is because restaurants in high-priced areas have relatively stable and loyal high-income consumer groups who are capable and willing to consume normally even during economic downturns. Additionally, high-priced areas are mainly concentrated in commercially vibrant districts such as Xuhui, Jing’an, and Huangpu, which are key targets for government support policies such as tax relief or rent reduction post-COVID-19. Policy intervention has facilitated the rapid recovery of commercial activities in these areas .
Transportation Indicators: The non-linear positive correlation between transportation factors and restaurant numbers shows no significant change, but it is evident that post-COVID-19, transportation factors have generally strengthened their influence on restaurant spatial distribution. The number of restaurants continues to increase with the increasing density of bus stops, subway stations, parking lots, and roads, without a clear threshold upper limit. Specifically, the slope of the parking lot quantity has increased post-COVID-19, indicating that parking lots have become an important consideration factor for restaurant location selection in the Post-COVID era .
Target Market: There were notable changes in malls and hospitals within the target market, while other variables showed no significant variations. Before COVID-19, the number of malls exhibited a negative correlation with restaurant density within the range of 75–190 per km2, indicating a decrease in restaurant numbers with an increase in mall quantity. However, post-COVID-19, restaurant numbers consistently increased with the increase in mall quantity. This phenomenon may be attributed to intensified competition and shopping district effects. When the number of malls in a certain area reaches a certain level, competition among restaurants becomes fiercer, leading some to opt for closure or relocation to avoid competition (Y. Li, Yao, et al. Citation2022). However, the outbreak of COVID-19 has propelled changes in consumer patterns and demands, with more people opting for online shopping and food delivery services. In this scenario, restaurants may be more influenced by online platforms and less affected by the quantity of malls. Additionally, some restaurants have developed new service models and online sales channels to cope with the challenges posed by COVID-19, thereby reducing their dependence on malls and gradually weakening the impact of peer competition. The trends for schools and hospitals are more consistent, exhibiting clear thresholds. Before COVID-19, restaurant numbers increased with the increase in school quantity within the range of 0–40 per km2, slowly decreasing beyond this threshold. However, post-COVID-19, the threshold decreased to 20, with restaurant numbers decreasing once school quantity exceeded 20 per km2. The reduction in the threshold may be attributed to changes in commercial activities and consumption habits around schools post-COVID-19, such as a decrease in the number of students due to the outbreak, thereby affecting pedestrian flow and consumption demand around schools. The decrease in demand for commercial activities led to a reduction in the threshold for restaurant density. For hospital density within the range of 0–10 per km2, restaurant numbers consistently increased significantly with the increase in hospital quantity, but beyond this range, an increase in hospital quantity no longer had a significant impact on restaurant density. The most significant increase in restaurants occurred within the range of 0–40 attractions per km2. This may be because within this range, the layout of restaurants becomes denser, facilitating the formation of relatively independent commercial areas and thereby promoting interaction and growth among them .
3.3. Varying correlations between restaurant density in the main urban area of Shanghai and driving factors
To determine the most suitable spatial econometric model, this study employed Lagrange multiplier tests proposed by L. Anselin, including LM-Error and LM-Lag, as well as robust versions of these tests (Robust LM-Error and Robust LM-Lag), to select the optimal spatial econometric model. If both LM-lag and Robust LM-lag tests yield p-values <0.05, it indicates significant spatial lag effects, prompting the selection of the Spatial Lag Model (SLM). If both LM-Error and Robust LM-Error tests yield p-values <0.05, it suggests significant spatial error correlation, leading to the choice of the Spatial Error Model (SEM). If both tests are significant, it indicates the presence of both spatial lag effects and spatial error correlation, warranting the Spatial Durbin Model. If neither test is significant, the Ordinary Least Squares (OLS) model is chosen. Upon examination, the LM-Error test and Robust LM-Error test for the years 2016–2022 both yielded p-values significant at the 5% level, thus leading to the selection of the SEM model .
Table 5. LM Test Results for the Model.
The results of the spatial error model from reveal that:
Table 6. SEM Regression Analysis Results of Driving Factors from 2016–2022.
The coefficient of the spatial error term is significantly positive, indicating a notable positive spatial correlation in the distribution of restaurants, with this correlation stemming from the error term. This suggests a certain level of interdependence in the spatial distribution of restaurants in the central urban area of Shanghai, potentially influenced by spillover effects from factors such as population, economy, and environment.
In terms of significance, the results of the spatial lag model are consistent with those of the Gradient Boosting Decision Tree (GBDT) model, indicating that favorable transportation conditions and a stable consumer market are crucial factors determining the spatial distribution of restaurants. As one of the most developed economic regions in China, Shanghai’s central urban area has the highest private car ownership rates. Therefore, whether a restaurant has independent parking facilities becomes a crucial consideration for diners. Additionally, after the COVID-19 outbreak, heightened safety awareness among residents led to an increased emphasis on social distancing (Maulana et al. Citation2022). With more people opting to drive themselves post-COVID-19, parking convenience has become a primary consideration in restaurant location selection. Shopping malls, office buildings, and residential areas provide a stable customer base. For instance, shopping malls and office buildings serve as places for shopping and work, whereas people live in residential areas. Opening restaurants in these areas makes it easier to reach potential customers.
In terms of positive and negative correlations, only x3 (house price) consistently exhibited a negative correlation with restaurant quantity. This is because high housing prices imply high rents, which increases the operational costs of restaurants, making it difficult to form restaurant clusters. Restaurant quality and x7 (road density) had a negative correlation in 2016, but a positive correlation in other periods, which may be related to the enactment of the “Shanghai Urban Renewal Measures” in 2015. This policy changed the direction and focus of urban development in Shanghai’s central urban area, affecting land use and planning strategies related to the commercial land and transportation layout. The “Urban Renewal Measures” strengthened commercial land planning and restrictions to emphasize urban spatial order, urban image shaping, and environmental protection, thereby limiting commercial land development and restaurant construction. This resulted in a negative correlation between road density and restaurant quantity in 2016. The negative correlation between x11 (hospital proximity) and restaurant quantity in 2016 and the positive correlation in other years may be attributed to the distribution of hospitals in Shanghai’s central urban area in 2016, primarily in bustling areas such as the Huangpu, Xuhui, and Changning Districts. Although these areas have convenient transportation, dense populations, and well-developed facilities, they also have high commercial rents, making it difficult for them to become popular restaurant locations. However, after 2016, medical facilities in Shanghai’s central urban area began to expand outward, covering a wider space and gradually including areas with lower rental costs. Therefore, restaurant quantity has been positively correlated with hospitals since 2016. The negative correlation between x10 (school proximity) and restaurant quantity during the initial stage of the COVID-19 outbreak (2020) had become positive by 2022, indicating that although restaurants near schools were initially affected during the early stages of the COVID-19 outbreak, leading to temporary closures, they were able to quickly and flexibly adjust to cope with the crisis, demonstrating good resilience (Mitra et al. Citation2023). Furthermore, x13 (attractions) exhibited a positive correlation with restaurants only in 2016, whereas negative correlations can be observed in the other years. In 2015, the Shanghai government launched a series of measures to support the construction of attractions to improve the city’s image and living environment. Therefore, the number of attractions in Shanghai peaked in 2016. To meet the dining needs of those visiting attractions, the government actively encouraged the development of the restaurant industry around attractions. However, owing to factors such as remote locations, inconvenient transportation, low consumption return rates, and reduced government subsidies, areas near attractions ceased to be popular restaurant locations after 2016, resulting in negative correlations in subsequent years.
To assess the robustness of the spatial error model above, this study replaced the spatial weighting matrix (geographical distance-weighted matrix) for verification. By calculating the surface distance based on the latitude and longitude of each fishing net’s center point, it was found that the farther the distance, the smaller the spatial weight coefficient, indicating weaker spatial correlation. The results of the spatial error model under the geographical weighting matrix were not significantly different from those under the aforementioned Queen adjacency matrix, suggesting that the estimation results of the model are robust. Therefore, the conclusions drawn above possess a high level of credibility.
4. Conclusion and suggestions
The restaurant industry, as a vital component of the commercial space, largely reflects trends in urban functional space expansion. The restaurant industry’s clustering and diffusion directions are closely coupled with commercial functional areas and urban expansion. This study utilized spatial big data to quantitatively depict the distribution pattern of the restaurant industry in Shanghai’s main urban area before and after the COVID-19 pandemic. The effects of multiple factors, such as population, economy, transportation, and the environment were analyzed, providing helpful insights for understanding the changes in the spatial pattern of the restaurant industry in response to public health crises within the city. This research also provides insights into restaurant location selection, urban commercial space layout, and resident consumption behaviors. The main conclusions are described below.
First, the COVID-19 outbreak resulted in significant challenges for the restaurant industry; however, its impact pales in comparison to the effects of urban land planning adjustments and the increase in online delivery platforms. In the post-COVID era, the restaurant industry in Shanghai’s main urban area will continue to maintain a pattern focused on “the Bund and surrounding commercial districts as the main core” and “an outward radial distribution from southwest to northeast.”
Second, changes in the ranking of contributing factors indicate that, in the post-COVID era, the target market remains a decisive factor in restaurant site selection. The areas surrounding shopping malls, offices, and residential areas continue to attract many restaurants. Regarding transportation conditions, the availability of parking spaces has become a top priority for restaurant operators and consumers. Areas with high school and road densities will emerge as popular locations for restaurant site selection in the future because of their high economic resilience and resistance to pressure during the COVID-19 pandemic. The trend of restaurants clustering around attractions, subway stations, and hospitals has weakened in the post-COVID era owing to the difficulties they face in quickly adapting to market changes and uncertainties.
Third, the threshold effect results of the influencing factors indicate that the development of the restaurant industry can be effectively promoted within certain ranges of population, economy, transportation, and environmental conditions. In the post-COVID era, it is most beneficial for restaurants to cluster when the population density ranges from 0 to 50,000 people/km2, GDP ranges from 0 to 20, billion yuan/km2, house prices range from 20,000 to 130,000 ten thousand yuan/m2, the number of schools ranges from 0 to 20 per km2, hospital density ranges from 0 to 10 per km2, and the number of attractions ranges from 0 to 40 per km2. Transportation conditions have no apparent threshold, with more developed transportation systems being more conducive to the development of the restaurant industry.
Fourth, regarding the positive and negative relationships between the influencing factors and restaurant density, high-priced areas and those around attractions have been less likely to attract a large number of restaurants in the post-COVID era, although restaurants in high-priced areas still have a certain level of risk resilience. Although restaurants may continue to cluster around hospitals in the post-COVID era, the intensity of this trend is expected to diminish.
Regarding the development of the catering industry in the main urban area of Shanghai in the Post-COVID era, this study proposes the following suggestions:
Deeply explore the target market and flexibly adjust site selection strategies. In the Post-COVID era, a large number of restaurants will still gather near shopping malls, office buildings, and residential areas. Therefore, urban planners should thoroughly understand the development potential of each area, analyze the post-normalization consumer demands and preferences after COVID-19, and layout different types of catering businesses targeting different demographics and consumer habits to meet market demands (Makona et al. Citation2023).
Emphasize the enhancement of public health awareness to meet customer parking needs. In the Post-COVID era, the concept of a healthy lifestyle has become deeply ingrained in society, with residents paying increased attention to safe social distancing and personal hygiene (Planinc and Kukanja Citation2022). As a result, self-driving and food safety will become primary concerns for residents. This necessitates that urban planners, when designing commercial spaces, comprehensively consider factors such as restaurant seating capacity, foot traffic, and surrounding transportation conditions. Ample parking areas should be allocated or existing parking facilities should be prioritized to reduce construction costs. Additionally, public health authorities should enforce strict food safety regulations. The heightened demand for food safety among residents during the COVID-19 period underscores the necessity for stringent food safety management in the Post-COVID era to facilitate the recovery of the catering industry.
Strengthening planning support for areas around schools, reasonably controlling the density of restaurants around tourist attractions, subway stations, and hospitals, to avoid excessive concentration of resources and waste (Ardestani et al. Citation2024). More commercial land should be provided for areas around schools, optimizing transportation networks, improving infrastructure, and enhancing regional competitiveness. Considering that restaurants around schools are often mid- to low-end establishments, the government should provide support through policies such as tax reductions and exemptions to help the restaurant industry recover vitality quickly. In the future, encouragement should be given to the diversified development of restaurants around schools, with strengthened regulation and guidance to elevate the overall industry standards, attract mid- to high-end restaurant brands, and enhance local consumption levels. Additionally, for low-end restaurants willing to improve, local governments should offer entrepreneurial support and training opportunities to promote transformation and upgrading.
Support innovative operations and drive digital transformation. To proactively address the COVID-19 crisis, the restaurant industry should adapt to the trend of online consumption, expand the delivery market, and adopt innovative business models (Fainshtein et al. Citation2023). This includes promoting food delivery services, advocating for contactless dining methods, accelerating the process of retailing semi-finished food products, and encouraging direct supply from farms. During the COVID-19 pandemic, the delivery business was less impacted compared to dine-in services and recovered more quickly. Therefore, restaurant enterprises should seize the opportunity to expand into the delivery market, creating additional profit opportunities for the industry. Simultaneously, they should digitally upgrade dine-in services by promoting smart ordering systems and implementing online reservation systems. This proactive approach will prepare the restaurant industry to withstand potential future risks.
This study has its limitations: (1) While multiple years of data were selected for analysis, the time span may not be sufficient to capture long-term changes. (2) The spatial scale of the study was limited to the central urban area of Shanghai, potentially overlooking more localized spatial variations. (3) Different types and scales of restaurant businesses may have diverse spatial distributions and influencing factors. Especially in the context of the interplay between consumer economics, the internet, and COVID-19, residents’ dining preferences exhibit variations, making the factors considered in restaurant location selection more complex. However, This study adopts an environmental economics perspective to explore the effects of a public health crisis, particularly the COVID-19 pandemic, on the urban restaurant industry. It provides a novel perspective and methodology for understanding the effects of public health events on economic behavior and urban spatial layouts. Additionally, this study innovatively integrates various methods such as geographic information technology, machine learning, and spatial econometric modeling to conduct in-depth quantitative analyses of urban spatial distribution characteristics and their driving factors. This study contributes new analytical tools and methodologies to the field of environmental economics. Finally, this study presents predictions and recommendations for development trends in the restaurant industry in Shanghai’s main urban area and its spatial layout in the post-COVID era. This study aimed to integrate the impact of public health events on the urban restaurant industry into the research scope of environmental economics, offering new insights and research directions for scholars in this field.
Disclosure statement
No potential conflict of interest was reported by the author(s).
Data availability statement
The data presented in this study are available on request from the author.
Additional information
Funding
Notes on contributors
DanDan Shao
Dandan Shao is a Ph.D. candidate at the Graduate School of Environmental Studies, Seoul National University. Her primary research interests include urban design, urban renewal, and the study of urban lifestyles.
KyungJin Zoh
KyungJin Zoh is a professor at the Graduate School of Environmental Studies, Seoul National University. He earned his Ph.D. in Urban and Regional Planning from the University of Pennsylvania. His main research areas are landscape design and theory.
Yanzhao Xie
Yanzhao Xie is a lecturer at the School of Computer Science and Cyber Engineering, Guangzhou University. His research focuses on hashing encoding with machine learning, deep learning, and related fields.
References
- Acuto, M., S. Larcom, R. Keil, M. Ghojeh, T. Lindsay, C. Camponeschi, and S. Parnell. 2020. “Seeing COVID-19 Through an Urban Lens.” Nature sustainability 3 (12): 977–978.
- Ahmed, S. A., H. N, and H. N. 2023. “Land Use and Land Cover Classification Using Machine Learning Algorithms in Google Earth Engine.” Earth Science Informatics 16 (4): 3057–3073. https://doi.org/10.1007/s12145-023-01073-w.
- Ardestani, Z. A., M. Panahi, A. H. Ebrahimi, and E. Parvizi. 2024. “How Urban Shopping Malls in Tehran Have Changed in the Post‐COVID Era.” Regional Science Policy & Practice 16 (2): 12628. https://doi.org/10.1111/rsp3.12628.
- Baek, C., P. B. McCrory, T. Messer, and P. Mui. 2021. “Unemployment Effects of Stay-At-Home Orders: Evidence from High-Frequency Claims Data.” Review of Economics and Statistics 103 (5): 979–993.
- Bao, W., A. Gong, Y. Zhao, S. Chen, W. Ba, and Y. He. 2022. “High-Precision Population Spatialization in Metropolises Based on Ensemble Learning: A Case Study of Beijing, China.” Remote Sensing 14 (15): 3654. https://doi.org/10.3390/rs14153654.
- Boissay, F., and P. Rungcharoenkitkul. 2020. Macroeconomic Effects of COVID-19: An Early Review. BIS Bulletins 7: Bank for International Settlements.
- Brizek, M. G., R. E. Frash, B. M. McLeod, and M. O. Patience. 2021. “Independent Restaurant Operator Perspectives in the Wake of the COVID-19 Pandemic.” International Journal of Hospitality Management 93:102766. https://doi.org/10.1016/j.ijhm.2020.102766.
- Carmona, M. 2021. Public Places Urban Spaces: The Dimensions of Urban Design. Routledge. https://doi.org/10.4324/9781315158457.
- Carpio, A., R. Ponce-Lopez, and D. F. Lozano-García. 2021. “Urban Form, Land Use, and Cover Change and Their Impact on Carbon Emissions in the Monterrey Metropolitan Area, Mexico.” Urban Climate 39:100947. https://doi.org/10.1016/j.uclim.2021.100947.
- Duman, Z., X. Mao, B. Cai, Q. Zhang, Y. Chen, Y. Gao, and Z. Guo. 2023. “Exploring the Spatiotemporal Pattern Evolution of Carbon Emissions and Air Pollution in Chinese Cities.” Journal of Environmental Management 345:118870. https://doi.org/10.1016/j.jenvman.2023.118870.
- Fainshtein, E., V. Chkoniya, E. Serova, and P. Vorobyev. 2023. “Sustainable Social Systems: Innovative Service Implications in the Restaurant Business in the Post-COVID Era with Digital Transformation Strategies.” Sustainability 15 (19): 14539. https://doi.org/10.3390/su151914539.
- Furuhashi, K., and T. Nakaya. 2023. “Investigating the Effects of Parameter Tuning on Machine Learning for Occupant Behavior Analysis in Japanese Residential Buildings.” Buildings 13 (7): 1879. https://doi.org/10.3390/buildings13071879.
- Gómez, J. A., J. E. Patiño, J. C. Duque, and S. Passos. 2020. “Spatiotemporal Modeling of Urban Growth Using Machine Learning.” Remote Sensing 12 (1): 109. https://doi.org/10.3390/rs12010109.
- He, H., and L. Harris. 2020. “The Impact of COVID-19 Pandemic on Corporate Social Responsibility and Marketing Philosophy.” Journal of Business Research 116:176–182. https://doi.org/10.1016/j.jbusres.2020.05.030.
- Hoernke, K., N. Djellouli, L. Andrews, S. Lewis-Jackson, L. Manby, S. Martin, and C. Vindrola-Padros. 2021. “Frontline Healthcare workers’ Experiences with Personal Protective Equipment During the COVID-19 Pandemic in the UK: A Rapid Qualitative Appraisal.” British Medical Journal Open 11 (1): e046199. https://doi.org/10.1136/bmjopen-2020-046199.
- Huan, Y., L. Song, U. Khan, and B. Zhang. 2023. “Stacking Ensemble of Machine Learning Methods for Landslide Susceptibility Mapping in Zhangjiajie City, Hunan Province, China.” Environmental Earth Sciences 82 (1): 35. https://doi.org/10.1007/s12665-022-10723-z.
- Huang, H., J. Huang, B. Chen, X. Xu, and W. Li. 2024. “Recognition of Functional Areas in an Old City Based on POI: A Case Study in Fuzhou, China.” Journal of Urban Planning and Development 150 (1): 04024001. https://doi.org/10.1061/JUPDDM.UPENG-4593.
- Huang, T., Y. Zhang, S. Li, G. Griffiths, M. Lukac, H. Zhao, and J. Zhu. 2023. “Harnessing Machine Learning for Landscape Character Management in a Shallow Relief Region of China.” Landscape Research 48 (8): 1019–1040. https://doi.org/10.1080/01426397.2023.2241390.
- Jamali, A. 2021. “Land Use Land Cover Modeling Using Optimized Machine Learning Classifiers: A Case Study of Shiraz, Iran.” Modeling Earth Systems and Environment 7 (3): 1539–1550. https://doi.org/10.1007/s40808-020-00859-x.
- Jeong, M., K. Kim, F. Ma, and R. DiPietro. 2022. “Key Factors Driving customers’ Restaurant Dining Behavior During the COVID-19 Pandemic.” International Journal of Contemporary Hospitality Management 34 (2): 836–858. https://doi.org/10.1108/IJCHM-07-2021-0831.
- Li, C., J. Zhao, and W. Hou. 2023. “Nonlinear Effects of Landscape Patterns on Ecosystem Services at Multiple Scales Based on Gradient Boosting Decision Tree Models.” Remote Sensing 15 (7): 1919. https://doi.org/10.3390/rs15071919.
- Li, X., R. Kleinhans, and M. van Ham. 2018. “Shantytown Redevelopment Projects: State-Led Redevelopment of Declining Neighbourhoods Under Market Transition in Shenyang, China.” Cities 73:106–116. https://doi.org/10.1016/j.cities.2017.10.016.
- Li, Y., Y. H. Chiu, Y. Li, T. Y. Lin, and Y. N. Lin. 2022. “The Influence of the Internet on Catering and Accommodation Industry Efficiency.” Economic Research-Ekonomska Istraživanja 35 (1): 949–970. https://doi.org/10.1080/1331677X.2021.1952087.
- Li, Y., P. Yao, S. Osman, N. Zainudin, and M. F. Sabri. 2022. “A Thematic Review on Using Food Delivery Services During the Pandemic: Insights for the Post-COVID-19 Era.” International Journal of Environmental Research and Public Health 19 (22): 15267. https://doi.org/10.3390/ijerph192215267.
- Liu, B., S. Zheng, L. Zhang, J. Liu, T. Fu, R. Hao, and M. Yin. 2023. “Identification and Analysis of Potential Open-Sharing Subjects of Unit-Affiliated Green Spaces in Shanghai Based on POI Data.” The Land 12 (12): 2162. https://doi.org/10.3390/land12122162.
- Makona, A., R. Elias, V. Makuya, and I. A. Changalima. 2023. “Does Innovation Ambidexterity Influence Restaurant Economic Performance in the Post-COVID-19 Era? The Mediating Effect of Customer Orientation.” Cogent Business & Management 10 (2). https://doi.org/10.1080/23311975.2023.2242164.
- Malki, Z., E. S. Atlam, A. E. Hassanien, G. Dagnew, M. A. Elhosseini, and I. Gad. 2020. “Association Between Weather Data and COVID-19 Pandemic Predicting Mortality Rate: Machine Learning Approaches.” Chaos, Solitons & Fractals 138:110137. https://doi.org/10.1016/j.chaos.2020.110137.
- Matsenko, O. M., O. V. Kubatko, V. Bardachenko, and K. Demchuk. 2021. “Transformation of the Restaurant Business as a Result of the COVID-19 Pandemic.” Improving the Security of Service and Maintaining the Health of Human Capital 2 (3): 27–38. https://doi.org/10.21272/hem.2021.3-03.
- Maulana, N., R. D. Astuti, H. B. Sukamdani, and P. Tjiptoherijanto. 2022. “Risk Perception in the Post COVID-19 Pandemic Era: An Analysis of Tourist Accommodation and Travel Behavior in the New Normal Era.” Sustainability 14 (22): 14758. https://doi.org/10.3390/su142214758.
- Mitra, P., Y. Zhang, B. K. Mitra, and R. Shaw. 2023. “Assessment of Impacts and Resilience of Online Food Services in the Post-COVID-19 Era.” Sustainability 15 (17): 13213. https://doi.org/10.3390/su151713213.
- Mohammad, N. S., A. R. Abdul Rasam, R. Ghazali, R. Idris, and R. Abu Bakar. 2023. “Spatial Clustering Phenomena of COVID-19 Cases in Selangor: A Hotspot Analysis and Ordinary Least Squares Method.” International Archives of the Photogrammetry, Remote Sensing and Spatial Information Sciences 48:237–243. https://doi.org/10.5194/isprs-archives-XLVIII-4-W6-2022-237-2023.
- Moon, J., and S. L. Han. 2016. “Analysis of the Influence of MERS Epidemic on Retailing Industry of Seoul City.” Journal of Channel and Retailing 21 (1): 129–152. https://doi.org/10.17657/jcr.2016.01.31.6.
- Mueller, J. T., K. McConnell, P. B. Burow, K. Pofahl, A. A. Merdjanoff, and J. Farrell. 2021. “Impacts of the COVID-19 Pandemic on Rural America.” Proceedings of the National Academy of Sciences 118 (1): 2019378118. https://doi.org/10.1073/pnas.2019378118.
- Muller, C. C., and C. Inman. 1994. “The Geodemographics of Restaurant Development.” Cornell Hotel and Restaurant Administration Quarterly 35 (3): 88–95. https://doi.org/10.1177/001088049403500319.
- Neise, T., P. Verfürth, and M. Franz. 2021. “Rapid Responding to the COVID-19 Crisis: Assessing the Resilience in the German Restaurant and Bar Industry.” International Journal of Hospitality Management 96:102960. https://doi.org/10.1016/j.ijhm.2021.102960.
- Pak, A., O. A. Adegboye, A. I. Adekunle, K. M. Rahman, E. S. McBryde, and D. P. Eisen. 2020. “Economic Consequences of the COVID-19 Outbreak: The Need for Epidemic Preparedness.” Frontiers in Public Health 8:546036. https://doi.org/10.3389/fpubh.2020.00241.
- Planinc, S., and M. Kukanja. 2022. “Restaurant Customers’ Quality Expectations: A Pre-And Post-COVID-19 Pandemic Comparison.” Tourism: An International Interdisciplinary Journal 70 (2): 168–182. https://doi.org/10.37741/t.70.2.2.
- Prayag, G., M. Landré, and C. Ryan. 2012. “Restaurant Location in Hamilton, New Zealand: Clustering Patterns from 1996 to 2008.” International Journal of Contemporary Hospitality Management 24 (3): 430–450. https://doi.org/10.1108/09596111211217897.
- Qiu, R. T., J. Park, S. Li, and H. Song. 2020. “Social Costs of Tourism During the COVID-19 Pandemic.” Annals of Tourism Research 84:102994. https://doi.org/10.1016/j.annals.2020.102994.
- Remuzzi, A., and G. Remuzzi. 2020. “COVID-19 and Italy: What Next?” The Lancet 395 (10231): 1225–1228. https://doi.org/10.1016/S0140-6736(20)30627-9.
- Rizou, M., I. M. Galanakis, T. M. Aldawoud, and C. M. Galanakis. 2020. “Safety of Foods, Food Supply Chain and Environment within the COVID-19 Pandemic.” Trends in Food Science & Technology 102:293–299. https://doi.org/10.1016/j.tifs.2020.06.008.
- Sadique, M. Z., W. J. Edmunds, R. D. Smith, W. J. Meerding, O. De Zwart, J. Brug, and P. Beutels. 2007. “Precautionary Behavior in Response to Perceived Threat of Pandemic Influenza.” Emerging Infectious Diseases 13 (9): 1307. https://doi.org/10.3201/eid1309.070372.
- Shen, J. 2020. “Covid-19 and Inter-Provincial Migration in China.” Eurasian Geography and Economics 61 (4–5): 620–626. https://doi.org/10.1080/15387216.2020.1820355.
- Shu, Y., J. Liang, Y. Rong, Z. Fu, and Y. Yang. 2023. “A More Accurate Estimation with Kernel Machine for Nonparametric Spatial Lag Models.” Spatial Statistics 58:100786. https://doi.org/10.1016/j.spasta.2023.100786.
- Siu, A., and Y. R. Wong. 2004. “Economic Impact of SARS: The Case of Hong Kong.” Asian Economic Papers 3 (1): 62–83. https://doi.org/10.1162/1535351041747996.
- Song, H. J., J. Yeon, and S. Lee. 2021. “Impact of the COVID-19 Pandemic: Evidence from the US Restaurant Industry.” International Journal of Hospitality Management 92:102702. https://doi.org/10.1016/j.ijhm.2020.102702.
- Stobart, A., and S. Duckett. 2022. “Australia’s Response to COVID-19.” Health Economics, Policy and Law 17 (1): 95–106. https://doi.org/10.1017/S1744133121000244.
- Taherdoost, H. 2023. “Enhancing Social Media Platforms with Machine Learning Algorithms and Neural Networks.” Algorithms 16 (6): 271. https://doi.org/10.3390/a16060271.
- Thompson, G. M. 2010. “Restaurant Profitability Management: The Evolution of Restaurant Revenue Management.” Cornell Hospitality Quarterly 51 (3): 308–322. https://doi.org/10.1177/1938965510368653.
- Tian, C., W. Luan, S. Li, Y. Xue, and X. Jin. 2021. “Spatial Imbalance of Chinese Seafood Restaurants and Its Relationship with Socioeconomic Factors.” Ocean & Coastal Management 211:105764. https://doi.org/10.1016/j.ocecoaman.2021.105764.
- Vaz, E. 2021. “COVID-19 in Toronto: A Spatial Exploratory Analysis.” Sustainability 13 (2): 498. https://doi.org/10.3390/su13020498.
- Walter, U., D. K. Müller, and A. Långvall. 2022. “The Spatial Distribution of Gourmet Restaurants.” Scandinavian Journal of Hospitality and Tourism 22 (4–5): 285–302. https://doi.org/10.1080/15022250.2022.2112412.
- Wang, A., S. Hussain, and J. Yan. 2024. “Macroeconomic Drivers Effect on Housing Sale Prices in China.” International Journal of Housing Markets and Analysis. https://doi.org/10.1108/IJHMA-12-2023-0182.
- Wang, L., Z. Q. Lin, and A. Wong. 2020. “Covid-Net: A Tailored Deep Convolutional Neural Network Design for Detection of COVID-19 Cases from Chest X-Ray Images.” Scientific Reports 10 (1): 19549. https://doi.org/10.1038/s41598-020-76550-z.
- Wong, A., C. Zoller, A. Fouda, and F. Paolucci. 2024. “Are We Past the COVID-19 Pandemic? Insights from Singapore.” Health Policy and Technology 13 (1): 100779. https://doi.org/10.1016/j.hlpt.2023.100779.
- Wu, K., C. Xu, J. Yan, F. Wang, Z. Lin, and T. Zhou. 2023. “Error-Distribution-Free Kernel Extreme Learning Machine for Traffic Flow Forecasting.” Engineering Applications of Artificial Intelligence 123:106411. https://doi.org/10.1016/j.engappai.2023.106411.
- Wu, M., T. Pei, W. Wang, S. Guo, C. Song, J. Chen, and C. Zhou. 2021. “Roles of Locational Factors in the Rise and Fall of Restaurants: A Case Study of Beijing with POI Data.” Cities 113:103185. https://doi.org/10.1016/j.cities.2021.103185.
- Xu, G., Y. Jiang, S. Wang, K. Qin, J. Ding, Y. Liu, and B. Lu. 2022. “Spatial Disparities of Self-Reported COVID-19 Cases and Influencing Factors in Wuhan, China.” Sustainable Cities and Society 76:103485. https://doi.org/10.1016/j.scs.2021.103485.
- Yang, J., J. Zhu, Y. Sun, and J. Zhao. 2019. “Delimitating Urban Commercial Central Districts by Combining Kernel Density Estimation and Road Intersections: A Case Study in Nanjing City, China.” ISPRS International Journal of Geo-Information 8 (2): 93. https://doi.org/10.3390/ijgi8020093.
- Yoo, J., J. Browning, and K. Minesinger. 2018. “Open Space Premium Near Commercial Zones–A Case Study in the City of Corona, California.” International Journal of Urban Sciences 22 (3): 322–333. https://doi.org/10.1080/12265934.2017.1407252.
- Yuan, Y., Q. Wang, and X. T. Yang. 2021. “Traffic Flow Modeling with Gradual Physics Regularized Learning.” IEEE Transactions on Intelligent Transportation Systems 23 (9): 14649–14660. https://doi.org/10.1109/TITS.2021.3131333.
- Zhou, L., W. Xiao, Z. Zheng, and H. Zhang. 2023. “Commercial Dynamics in Urban China During the COVID-19 Recession: Vulnerability and Short-Term Adaptation of Commercial Centers in Shanghai.” Applied Geography (Sevenoaks, England) 152:102889. https://doi.org/10.1016/j.apgeog.2023.102889.