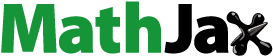
ABSTRACT
Political actors participate in policy debates as an advocacy strategy to influence political opponents and public opinion. They often engage in cross-sectoral advocacy by participating in multiple adjacent debates. To investigate what factors influence cross-sectoral advocacy in policy debates, we examined advocacy coalitions in two health policy debates in the UK – the regulation of sugar-sweetened beverages and alcoholic drinks. We predicted cross-sectoral advocacy efforts across 237 actors, such as health charities, manufacturers, or retailers, as a function of their belief system alignment relative to their advocacy coalition. In a methodological innovation, Bayesian item response models were applied to policy beliefs stated publicly by actors in eleven newspapers to measure the ideological positions of actors and beliefs relative to their advocacy coalition. Extreme belief alignment relative to one’s coalition, a small spread of stated beliefs, and a strong engagement were found to explain cross-sectoral advocacy.
Introduction
Political actors often have a vested interest in specific policies. To achieve their policy goals, they engage in advocacy. Advocacy efforts can take different forms, such as lobbying or engagement in policy debates. When actors participate in policy debates, they try to sway public opinion or influence their political opponents (Fischer & Forester, Citation1993; Jenkins-Smith et al., Citation1991).
Each actor holds a complex system of policy beliefs. They feed into an actor’s decision on what to contribute to a policy debate, when, and how (Leifeld, Citation2017; Sabatier, Citation1988, Citation1998). Policy beliefs also guide actors’ coordination in policy networks in the pursuit of policy objectives (Henry et al., Citation2011; Ingold, Citation2011). Within each policy subsystem, actors can be grouped empirically into advocacy coalitions by the extent of overlap in their belief systems (Sabatier, Citation1988, Citation1998).
Actors who engage in policy debates can draw on different kinds of resources in their pursuit of policy objectives. Such resources include money, connections to journalists and other actors, a central position in the policy network, formal authority, membership in policy forums, and reputation (Ingold & Leifeld, Citation2016). Another resource they can use is cross-sectoral advocacy – where actors engage in multiple related but distinct debates – for example in the regulation of different harmful products. In this contribution, we aim to explain why some actors engage in cross-sectoral advocacy efforts and make use of this resource while others commit to a single policy debate.
There are several reasons for engaging in cross-sectoral advocacy: First, actors can use connections to other subsystems to develop a better understanding of the effectiveness of their arguments or frames. Cross-sectoral advocacy will therefore give actors an informational advantage to position themselves strategically in each of the debates (Brandenberger et al., Citation2021). Second, actors who reach across policies can more effectively tie different policy coalitions together. Doing so increases the network embeddedness of the coalitions with each other and thereby their information flows and shared strategies (Heaney & Leifeld, Citation2018; Junk, Citation2019; Mahoney, Citation2007). A third reason for using cross-sectoral advocacy is that actors who participate in multiple debates can find overarching, integrated solutions to policy problems (Candel, Citation2017). Such efforts are known as policy integration (Tosun & Lang, Citation2017; Trein et al., Citation2019). Fourth, cross-sectoral advocacy is a resource in pursuing policy objectives because it unites advocacy actors under a common umbrella of arguments and frames: It leads to more ‘frame bundling' and stronger integration and persuasiveness of arguments, which in turn increases the perceived cohesion of actors in a coalition (Leifeld & Haunss, Citation2012). Cross-subsystem involvement has been described in different contexts also under the labels boundary spanning (Brandenberger et al., Citation2021) and horizontal, or cross-boundary, policy entrepreneurship (Faling et al., Citation2019).
Although cross-sectoral advocacy can serve as a resource in the pursuit of policy objectives, not every political actor engages in it. But what explains this variation in cross-sectoral advocacy efforts? Currently, the drivers of this variation between actors are not well understood. A better understanding is required if we want interest intermediation to be pluralist, transparent, and equitable: If one coalition draws on such resources while another one loses out, it may lead to adverse outcomes like, in the extreme case, regulatory capture (Laffont & Tirole, Citation1991). In addition to improving democratic policy making, diagnosing differences in cross-sectoral advocacy should also matter for any particular policy subsystem in a normative way. Actors should learn to realise their cross-policy advocacy potential and exploit it to attain their policy goals (Faling et al., Citation2019).
One plausible explanation for the variation in cross-sectoral advocacy efforts lies in the way in which actors’ individual beliefs are aligned with the collective belief system of their coalition. A belief system consists of beliefs at different layers of generality. Each layer contains specific beliefs or frames actors can support or reject (Leifeld, Citation2013, Citation2017), such as different policy instrument preferences or arguments. By adopting some policy beliefs while not adopting or rejecting other beliefs, actors display a belief system alignment relative to the other actors in their coalition. Some actors display moderate overall positions while other actors are ideologically more extreme. For example, an industry actor with an extreme alignment relative to its industry-led coalition may reject all beliefs related to the regulation of a commodity and its alleged negative health consequences and only support self-regulation. In contrast, an industry actor with a moderate alignment may accept some kinds of regulation as long as there is no outright ban of a product and may acknowledge mixed scientific evidence on negative health consequences of the product. The relative belief alignment of an actor with its coalition may explain whether the actor engages in a single policy debate or cross-sectoral efforts – but there are multiple plausible ways in which this may happen. Actors with a more extreme alignment may have a stronger interest in the issue and thus engage in adjacent debates as well – or they may be more specialised and thus focus all their attention on the issue. Below, we will pick these theoretical mechanisms apart and provide a first empirical test future research can build on.
A complication arises in this theory test: There is no established way of measuring belief system alignment. The reason is that the position of the beliefs relative to one another in any policy debate is unknown and subjective. For example, within a coalition it is unclear and may be disputed whether the belief that a policy will improve population health is more, or less, extreme than the belief that the policy will improve public financial resources because the two beliefs are of a categorical nature. Would an actor adopting one or the other be more extreme? Only by considering the whole empirical belief system simultaneously can the latent ideological positions of the beliefs be determined – and consequently the alignment of the actors holding them be measured. To accomplish the measurement of belief system alignment, we propose employing item response theory, a family of measurement models from educational and psychological testing and with many existing applications to ideological scaling. Measuring belief system alignment in this way overcomes an obstacle often encountered in research on advocacy coalitions: Ideological heterogeneity of actors within coalitions is routinely ignored, and coalitions are treated as monolithic blocs (e.g., Cobb & Coughlin, Citation1998; Savell et al., Citation2016).
Against this backdrop, the present article makes three contributions. First, we propose a methodology for the systematic measurement of the relative ideological location of each belief and each actor toward each other in the collective belief system of a coalition, debate, or subsystem. The Bayesian item response model is applied to actors’ stated beliefs in press articles. This approach yields estimates of actors’ ideological positions on a latent left-right dimension (the actor’s ability parameter), relative positions of beliefs (the item difficulty parameter), and the discriminatory power of each belief for the coalition structure of the policy subsystem (the item discrimination parameter), which can be interpreted as a measure of the alignment of a belief with a coalition. We derive two measures of belief system alignment from these estimates: the relative location of an actor in the belief system of its coalition and the spread of an actor’s beliefs relative to the collective belief system.
Second, using these methods, we present a joint analysis of two health policies and the public debates surrounding their introduction: Minimum Unit Pricing for alcohol (MUP) and the Soft Drinks Industry Levy (SDIL). These policies were selected because they were both originally considered as policy interventions for introduction into the United Kingdom (UK) and because they would both reduce the harmful consumption of unhealthy products across the population by using fiscal measures – similarities that qualify them in principle for cross-sectoral advocacy (Buckton et al., Citation2019; Collin et al., Citation2017; Fergie et al., Citation2019; Savell et al., Citation2016). Manufacturers, retailers, think tanks, and associations were part of an informal advocacy coalition in the media trying to contain regulation, while an advocacy coalition of health charities and advocacy groups, government advisory bodies, professional associations, and scientists worked to expand regulation (see Cobb & Coughlin, Citation1998; Schaub & Metz, Citation2020; Tosun & Schaub, Citation2017 on policy containers and expanders). We find that, somewhat ironically, those seeking to contain policy find themselves engaging in more cross-sectoral advocacy efforts than those seeking to expand regulation. But the variation in cross-sectoral advocacy is not fully explained by containment.
Third, we therefore develop theoretical ideas on how the belief system alignment of an actor relative to its coalition may help explain whether the actor engages in cross-sectoral advocacy efforts. We exploit variation between actors to put these hypotheses to a first test. While a broader test across a range of sectors and policies will be needed eventually, the analysis provides an initial credibility test of the belief system alignment hypothesis.
Belief system alignment
Belief system alignment has two aspects: the location of an actor relative to its coalition and the spread of the actor’s beliefs around this location. We will consider each in turn below.
Because we are examining two similar policies, we assume that the policy debates can be broken down to a single left-right dimension. In the regulation of unhealthy commodities, the left-right scale can be interpreted as a dimension ranging from pro-health interests – trying to regulate products as rigorously as possible and claiming serious health hazards – to pro-industry interests, trying to avert regulation, insisting on their freedom to sell products as much as possible, and disputing scientific evidence on the harm of their products. There is an absolute zero position at the center of the dimension. Clustering of actor and belief positions on the left and on the right of the zero point marks the advocacy coalitions on each side. There are moderate actors and beliefs taking a middle-ground position on either side. There are also extreme actors and beliefs close to the end points of the scale on either side. The absolute distance from the zero point in the middle to each actor’s or belief’s estimated position indicates its extremeness or its location relative to its coalition, from moderate to extreme.
Extremeness of ideology
Holding an extreme ideological position within one’s coalition may affect cross-sectoral advocacy efforts in several ways. As either a positive or a negative association between the two seems plausible, we will formulate the association as two opposing working hypotheses (i.e., an undirected hypothesis with two underlying explanations): the policy specialisation hypothesis and the policy integration hypothesis.
One possibility is that actors with more extreme policy beliefs avoid cross-sectoral advocacy because they specialise in a narrowly defined issue and direct all their attention to the policy. A specialised actor may have vested interests in this one specific policy or in its containment. Its extreme beliefs reflect the stakes the actor faces. For example, a producer of one specific commodity may strongly prefer the containment of regulation of this commodity but may not care about other products. Because of these high stakes, the actor will be outspoken and display extreme policy beliefs in order to sway public opinion and its opponents in the debate. If the actor had a more diverse product portfolio, perhaps including healthier products, it would take a less extreme position on this one issue, but it would also try to participate in multiple debates around different kinds of food and divide its attention. For example, a producer of spirits is specialised, outspoken, and displays extreme policy beliefs around the regulation of alcoholic drinks but perhaps not about sugar-sweetened beverages – while a retailer can sell different products and may display more moderate beliefs on the regulation of each one of them. On the other end of the spectrum, a cancer charity is less specialised and possibly less ideologically extreme than an obesity- or addiction-specific advocacy group on each specific issue and may need to divide its attention to fulfill its mission. Hence more extreme policy beliefs may be associated with policy specialisation rather than cross-sectoral advocacy.
Another possibility is that extreme policy beliefs are associated with cross-sectoral advocacy and policy integration. Exhibiting strong policy views in a coalition might imply a desire to regulate (or fend off regulation) across multiple processes and find a unified solution. If more interests are at stake at once for an actor, the actor will more strongly support or oppose regulation and engage in multiple policy debates. Finding common, overarching regulatory frameworks for multiple products will motivate the most extreme health charities and the most extreme industry actors to invest in cross-debate advocacy because the payoffs of policy success are proportionally higher. Hence extreme policy beliefs can lead to policy integration through cross-sectoral advocacy.
Spread of an actor’s policy beliefs
In addition to the ideological location of an actor, the spread of an actor’s policy beliefs defines an actor’s belief system alignment with its coalition. An actor can display a narrowly defined ideological position (small spread) or a large uncertainty around the expected ideological position of the actor (large spread). Ideological spread is operationalised by the variance of the ideological position estimate of an actor in the Bayesian item response model. Like the extremeness of ideology, its spread may have a positive or a negative relationship with cross-sectoral advocacy. Three different underlying mechanisms may account for the positive or negative association: belief variance, self-contradictions, or engagement.
First, an actor may display a large or small variance in beliefs. For example, an actor can hold several extreme beliefs and several moderate beliefs. Holding a diverse portfolio of policy beliefs may be associated with cross-sectoral involvement because varied beliefs cover different cross-sectoral aspects of regulation. For example, a health charity may both state that regulation will improve population health and that the policy being debated needs to be part of a package of measures. Holding both beliefs rather than only the first one will increase the estimated positional variance and may at the same time indicate oversight of multiple sectors, such as alcohol and sugar regulation. Oversight of multiple sectors is also required from office-seeking politicians and parties, who seek public attention and try to generate votes by participating in policy debates. Such motivations would translate into a large spread of beliefs and engagement in cross-sectoral activity. Hence more belief variance should be associated with more cross-sectoral advocacy.
Second, a large belief spread can be caused by self-contradictions. They occur when an actor states both support and rejection of a policy belief, for instance in the news media. Actors may simply do so because beliefs may be intrinsically at odds across two policy sectors. For example, believing that there is enough medical evidence to support the regulation of alcohol may not necessarily imply believing that there is enough medical evidence to support regulation of sugar in beverages or vice-versa. If aggregated across debates, the actor will both support and reject the stance that there is enough scientific evidence for regulation of unhealthy commodities. Such seemingly inconsistent beliefs will increase the variance of beliefs and the spread of ideological position estimates. A larger spread of ideology may thus be associated with a greater engagement in cross-sectoral policy advocacy.
Third, a larger spread of policy beliefs may arise from superficial engagement with a policy. An actor who has a strong interest in (the containment of) a policy will hold, and publicly state, many policy beliefs. An actor whose interests are not as much at stake will hold some policy beliefs but will be uncertain on others and will refrain from stating positions on these beliefs publicly. The fewer policy beliefs the actor states, the more uncertain the actor’s position and the larger the variance of the positional estimate. Hence a lack of interest and low stakes imply a large ideological spread – and engagement with only one sector rather than cross-sectoral advocacy efforts.
Policy belief spread may therefore either increase or decrease cross-sectoral advocacy efforts. If the spread or uncertainty is primarily caused by belief variance or contradictory positions across debates, we expect that a larger spread of beliefs leads to more cross-sectoral advocacy efforts. If the ideological spread is caused by a lack of engagement and many unstated beliefs, we expect that it leads to less cross-sectoral advocacy. In the analysis below, we will first test the aggregate effect of the spread of ideology and then, in a separate model, the three possible mechanisms through which it may operate.
If belief spread operates through a lack of engagement with the policy, we should also expect this to intervene in the extremeness hypothesis. An actor who shows a large variance in estimated belief positions because of low engagement will unlikely hold extreme policy beliefs. If engagement matters, both extremeness of position and spread of policy beliefs should be associated with it.
Finally, we include several control variables. We account for policy expanders and containers (Cobb & Coughlin, Citation1998; Schaub & Metz, Citation2020; Tosun & Schaub, Citation2017) by controlling for whether an actor’s estimated policy position is in the PRO-regulation (expander) coalition and by controlling for specific actor types like manufacturers, retailers, etc. Finally, we control for the frequency with which actors state their beliefs because it may affect policy specialisation or integration as well as cross-sectoral advocacy.
Case study and data
National debates around two fiscal policies designed to address unhealthy commodity consumption and improve population health were selected for a joint analysis: Minimum Unit Pricing (MUP) for alcohol, introduced in Scotland (May 2018) and Wales (March 2020); and the Soft Drinks Industry Levy (SDIL), introduced across the UK in April 2018. Both controversial policies resulted in significant national-level debates with clear polarisation in stakeholder views. There is mounting evidence that the alcohol, ultra-processed food, and drink industries use similar strategies to the tobacco industry in efforts to undermine effective health policies (Freudenberg, Citation2014; Moodie et al., Citation2013 ). But unlike the tobacco industry, manufacturers of alcohol and ultra-processed food and drink products are often identified as potential partners in multi-sectoral health initiatives (Collin et al., Citation2017) – although these unhealthy commodity industries (UCIs) are increasingly identified as major drivers of non-communicable disease (NCD) epidemics (Freudenberg, Citation2014). The recent introduction of policies tackling the health harms caused by UCIs indicate a new willingness of governments to adopt a regulatory approach rather than relying on insufficient voluntary initiatives and positive nudge approaches to improve public health.
UCIs are known to use the mass media to try to persuade the public and policymakers to support or oppose new legislation. In the lead-up to the introduction of such legislation, the news media play a critical role in framing the issues, presenting opinion and attracting public and policy support (Hilton et al., Citation2020). As the advertising and marketing activities of UCI retailers and manufacturers have been curbed by regulation, media influence has become an increasingly important resource for UCIs. This is especially true in politically charged pricing policy debates, such as MUP for alcohol and SDIL, where industry efforts to disrupt the introduction of these pricing policies have been significant (Hilton et al., Citation2020).
Smith et al. (Citation2016) suggest examining comparisons of cross-policy advocacy efforts and the network of actors in them to elucidate interests, strategies, and actions that are common across industry sectors. Collin et al. (Citation2017) also suggest the importance of exploring the actions of key stakeholders working across different UCIs, drawing attention to large single corporations whose interests penetrate multiple industries.
Sharing of ideologies and policy beliefs across UCIs working to oppose legislation is also evident. Comparing alcohol and tobacco strategies and arguments, Savell et al. (Citation2016) suggest that there are several commonalities, including both sectors providing skewed interpretations of evidence while also promoting voluntary codes, based on establishing themselves as acting responsibly in relation to health. Petticrew et al. (Citation2017) have noted commonalities in use of ‘complexity' arguments across food, beverage, alcohol and gambling industry documents. Looking across separate studies of media representations of MUP and SDIL, commonalities are also evident in Buckton et al. (Citation2019) and Fergie et al. (Citation2019). However, systematic comparison of similarities and differences in belief systems of these policy problems, solutions, and impacts, as well as the determinants of cross-policy advocacy efforts, has not been undertaken – with one exception: Hilton et al. (Citation2020) compared the debates using network analysis – but without a focus on explaining cross-policy advocacy. Their comparison tentatively suggested ‘greater cross-sector collaboration among policy opponents than proponents' and ‘that, in seeking policy congruence, there may be a space for further cross-sector public health advocacy, by presenting arguments across policy debates in support of their counterparts.'
To investigate the belief systems and any resulting cross-policy advocacy activities across MUP and SDIL further, a media content analysis was conducted using eleven leading national UK newspapers (for more details, see Hilton et al., Citation2020). Each newspaper article was screened for instances where political actors were reported to support or reject policy beliefs – more specifically, secondary aspects in the terminology of the advocacy coalition framework. 63 policy beliefs were inductively identified in this way, and each actor’s stances on the beliefs were recorded. We call observations of actors referring to beliefs statements. For the MUP policy debate, 1924 statements by 152 individuals from 87 organisations (e.g., companies, parties, associations) were coded in 348 articles, covering May 2011 to November 2012. For the SDIL debate, 3883 statements by 214 individuals from 175 organisations were coded in 511 articles covering May 2015 to November 2016. About 29 policy beliefs were present in both debates while 17 were unique to MUP and another 17 were unique to SDIL. The manual coding was conducted using the software Discourse Network Analyzer. Two coders concurrently coded one debate each and synchronised their inductive codebook regularly and discussed any differences to ensure reliability. For each observation, the person, organisation, policy belief, and a binary agreement variable indicating support of, or opposition to, the belief by the actor was recorded. The result was a comprehensive national-level media dataset covering actors and the secondary-aspect layer of their belief systems, which, according to Sabatier (Citation1998), is the belief system layer that characterises coalitions within policy processes (as opposed to the wider subsystem).
Methods
The dataset was recoded into an binary matrix with belief categories
as column labels and actors
as row labels, with zeros denoting more instances of rejection of a belief by the actor than instances of support and ones denoting more support than rejection. Actor-belief combinations that did not occur empirically were coded as missing values, NA, which effectively increased the uncertainty around the estimation of an actor’s position, leading to a higher spread of the actor’s belief system around the estimated ability parameter.
With this data structure, a one-dimensional item response model was estimated using Gibbs sampling (Albert, Citation1992), as implemented in the MCMCpack R package by Martin et al. (Citation2011). Item response theory was originally designed to estimate the ability of students and the difficulty of a test question and its ability to discriminate between good and poor students (Albert, Citation1992). Item response models are routinely used in political science to scale legislators and parties on a latent ideological left-right dimension using roll-call voting records (Clinton et al., Citation2004). Däubler and Benoit (Citation2017) demonstrated the efficacy of IRT models in manual content analysis of ideological statements in party manifestos. The item response model can be expressed as an overparameterised probit model of the form
where index
denotes items (= beliefs), index
denotes individuals (= actors),
is the probability of individual
to get item
right (i.e., to have a one, rather than zero, in cell
of the matrix),
is the standard normal cumulative distribution function (the inverse link function of the probit model),
is the (latent) difficulty of the item,
is the ability of the individual, and
is the ability of the item to discriminate between individuals’ abilities. In the product, the item discrimination parameter ‘modifies' the ability of the individual. The joint likelihood for all observations in
is the product of the individual probabilities over all
and
indices. While the MLE can be obtained in the E-M algorithm by making additional assumptions to ensure identifiability, Bayesian estimation using Gibbs sampling is nowadays the standard approach to estimation, with identification by drawing from a multivariate normal distribution for the individual and item parameters and adding constraints for avoiding position flipping along the MCMC chain (Martin et al., Citation2011). The IRT models were estimated with 3.5 million iterations, of which the first 500,000 were discarded as burn-in, and samples were drawn from the chain with a thinning factor of 200. The result was a sample of 17,500 iterations.
The resulting ability parameters for the actors and item discrimination parameters
for the beliefs were plotted in descending order along with their highest posterior density (HPD) intervals as a measure of the spread around estimated positions. The spread around a point estimate (the mean posterior density) can be due to the uncertainty arising from unstated beliefs (NAs), self-contradictions on beliefs, and actual variance in beliefs (e.g., actors adopting beliefs with different ideological positions). The ability parameters for the actors represent their ideal points (i.e., locations), with values below zero indicating classification into the PRO-regulation coalition and above zero ANTI-regulation. The absolute mean value of the ability parameter,
, was then used to measure the distance from the center of the ideological scale, which is a measure of the extremeness of an actor’s position in their coalition. High values represent extreme positions, and low values represent moderate positions. The spread around an actor’s ideal point was measured by subtracting the 2.5% HPD value from the 97.5% HPD value, effectively measuring how clear or how vague the stated position of an actor was.
Both quantities, extremeness and spread, along with control variables for frequency of belief statements, a dummy variable for positive versus negative ideological positions (= ANTI or PRO coalition), and actor types were then used to explain cross-policy advocacy using a logistic regression model of the form:
where integration refers to policy integration or cross-policy advocacy (1 if an actor is active in both policy debates or 0 if only in one); RET = Retailers; MAN = Manufacturers and associated industries; POL = Political parties and government actors; and CHA = Health charities and advocacy organisations.
Three separate models were estimated for joint, mixed, and separate versions of the dependent variable. The joint model used estimates from a joint item response model of all actors and beliefs across the two debates. The advantages are direct comparability of all measures and high statistical power because the full set of information went into the estimation of positions. The separate model used parameters estimated either from the MUP or the SDIL debate for each actor, depending on where the actor was more active, to predict the actor’s secondary activity in the respective other policy debate. The advantage is a slightly clearer causal direction (though all analyses presented here can only establish associations, not causality, due to the lack of a temporal perspective and a causal research design); the disadvantage is lower comparability and statistical power as the two debates were estimated separately and observations from each debate could not inform inference on the belief system in the other debate, hence leading to lower accuracy in estimates due to less information. The mixed model takes a middle ground: Estimates from either MUP or SDIL were employed for observations that participated in only one debate while joint estimates were used for all remaining data points.
The spread variable resulted directly from the estimated IRT model, which accounted for the whole belief system and actors’ empirical positions on these beliefs. While it was moderately correlated with the extremeness of actors’ positions
, extremeness of position was controlled for with
, hence both effects could be effectively separated in the model.
In order to pinpoint the precise mechanisms driving the effect of belief spread, a fourth model was estimated. It included all model terms of the mixed model (the best-fitting model among the three) and three additional mechanisms in lieu of belief spread: For each actor, we included (a) the standard deviation of the estimated discrimination parameters of the beliefs the actor adopted, weighted positively or negatively depending on whether the actor supported of rejected the belief (thus measuring actual belief variance); (b) the number of beliefs over which the actor did not express opinions (i.e., NAs) as a measure of engagement, where many unstated beliefs were equivalent to low engagement; and (c) the number of beliefs over which the actor held contradictory beliefs, i.e., made at least one supportive and one critical statement. These three mechanisms were tested to unpack the underlying factor(s) of the belief spread hypothesis as outlined above.
Finally, the best-fitting logit model (among the first three) was used to predict probabilities for selected scenarios to aid model interpretation.
Descriptive results
shows the estimated ability parameters and their 95% HPD intervals for the most active actors (those who make at least ten statements). filters these positions by actor type. There is strong clustering by actor type, with retailers, think tanks, and manufacturers and associated industries being firmly rooted in the ANTI-regulation coalition and academics, health charities and advocacy groups as well as health professionals and government health advisors being predominantly located in the PRO-regulation coalition. Political parties and government agencies are spread across the two coalitions. The opposing positions of health charity/advocacy groups and manufacturers/associated industries underline the inherent policy conflict between public health advocates and industrial representatives. Both sides were additionally supported by scientific or technical expertise of organisations such as the British Medical Association (BMA) for the PRO-regulation coalition and think tanks/research analysts like the Institute of Economic Affairs for the ANTI-regulation coalition (Sabatier, Citation1988). The leading policy opponents principally were industry associations like the Wine and Spirit Trade Association and the Scotch Whisky Association for the MUP debate and the British Soft Drinks Association and the Food and Drink Federation for the SDIL debate. The PRO-regulation coalition was led by the Scottish Government (in the case of MUP) along with health advisors and advocacy groups like Action on Sugar and Alcohol Concern.
shows the estimated discrimination parameters for policy beliefs occurring in both debates. The positions are highly correlated between the two debates , therefore notably underlining ideological belief similarities in the MUP and SDIL debates that may serve as common ideological background for actors to participate in both debates. On the extreme PRO end of the scale, beliefs such as ‘Policy is supported by evidence' and ‘Responsibility deals with the industry are ineffective' can be found, while the extreme ANTI end of the scale features beliefs like ‘Policy is illegal' or ‘Policy will damage the wider economy'.
summarises the contentiousness of the beliefs using simple counts, expressed as a percentage (i.e., not based on the scaling). The most contested beliefs revolved around whether a policy would reduce the consumption of the commodity and whether legislation was indeed needed.
Inferential results
presents the coefficients and standard errors of the three logit models. The Mixed model shows a slightly better model fit in terms of the AIC and log likelihood than the other two models, hence the remaining discussion and interpretation will focus primarily on this model.
Table 1. Logistic regression models of cross-sectoral advocacy, with three types of aggregation for the ideology variables.
Extremeness of ideology (the absolute value of the ability parameter) shows a positive and significant (at the 95% level) effect on cross-sectoral advocacy in all three initial models. In absolute terms, the extremeness of the ideology coefficient of means that a one-point move away from the zero line on the ideological scale in either direction towards the extreme ends increases the log-odds of being active in both debates, rather than only one of them, by
. The MUP ability scale ranges from
to
, the SDIL ability scale from
to
, and the joint scale from
to
. The standard deviation of the joint ability parameter of actors is
(or
for MUP and
for SDIL). Moving away from the ideological zero point by one standard deviation (
) towards the extreme poles would consequently entail an increase in the log-odds of policy integration by
, or an increase of
in the odds of engaging in both sectoral debates. Moving from the average position in the PRO coalition on the left of the scale (mean of the PRO coalition
on the joint scale) to its extreme end (
) would hence imply a more than five-fold increase in the odds of being engaged both debates (
), and going from the average position in the ANTI coalition on the right of the scale (mean of the ANTI coalition
) to its extreme end (
) would roughly imply a more than ten-fold increase in the odds of cross-sectoral engagement (
), controlling for the extent to which actors are engaged and the camp they are in. In conclusion, we reject the null hypothesis that an tor’s ideological position within a coalition is not associated with cross-sectoral advocacy in favor of the alternative hypothesis that policy actors with more extreme positions within their coalition tend to engage in cross-sectoral advocacy efforts.
The spread of an actor’s ideology as measured by the size of the actor’s 95% HPD interval has a negative coefficient of in the Mixed model at a significance level of 99.9%. To put this into context, the smallest recorded HPD interval has a size of
and the largest one
in the Mixed model, with a mean of
, a median of
, and a standard deviation of
. Hence an increase of one standard deviation in the size of one’s interval is associated with a 90% decrease (
) in the odds of being involved in both policy debates rather than only one. Thus, the null hypothesis that the spread of the ability HPD does not systematically covary with cross-sectoral advocacy can be rejected with high confidence in favour of the alternative hypothesis stating that belief spread leads to lower chances of cross-sectoral advocacy.
Whether an actor is in the PRO or ANTI coalition does not make a discernible difference for cross-sectoral advocacy. Neither does the activity of an actor in terms of statement frequency. However, certain actor types exhibit more (or less) cross-sector advocacy than others: Retailers/retail associations are among the most consistent opponents of regulation, and they show a strong tendency for cross-sectoral advocacy efforts as they have an interest in both products. Although manufacturers and associated industries and associations are also in the ANTI coalition, they do not show an elevated tendency to engage in cross-sectoral activity as they have an interest in a specific product. To the contrary, they seem less inclined to participate in both sectors than other actors (with a borderline-significant result). In the PRO coalition, the main group of proponents of the regulation of unhealthy commodities, the health charities and advocacy groups, do not show a statistically detectable association with cross-sector advocacy efforts. Finally, one would expect political parties and government actors, who were divided over the issue, to engage in both policy debates as they have a mediating position between societal interests in pluralist and corporatist interest intermediation. Indeed, there is a positive and significant effect at the 95% confidence level in the Joint model, 90% level in the Mixed model, and at the 99% level in the Separate model, with coefficients ranging from to
.
To aid interpretation of these effects, presents predicted probabilities for nine scenarios, with average/typical values on all variables unless otherwise stated. Retailers in the ANTI coalition with extreme ideology (i.e., the maximum value) and a low belief spread (i.e., the empirically observable minimum of the HPD interval sizes of all actors) are almost certain to engage in both policy debates. Moving from extreme and consistent beliefs to average positions within their coalition and an average spread brings the probability of engaging in both debates down to around 0.4, which is still larger on average (though not significantly so) than the baseline probability of , as eleven per cent of all actors engaged in both debates. Manufacturers and associated industries with extreme ideologies and low spread also show a high probability (around 95%) of being active in both debates, but as soon as their ideological position and spread are changed to average within-coalition levels, their probability of cross-sectoral activity goes down to close to zero. This supports the results from the regression table that manufacturers and associated industries per se do not show a significant effect, and it is really the position and spread that drive the high probability for this group. The average probability in the ANTI group for any actor is in the low single-digit range, and the average probability in the PRO camp is lower than
, confirming that coalition membership per se is not responsible for the variation. Health charity and advocacy groups in the PRO coalition, even when they have an extreme position and low ideological spread, have a very small probability of cross-sectoral advocacy engagement. This is noteworthy for two reasons. First, the two main hypotheses seem to hold irrespective of coalition or actor type unless the actor is a health charity or advocacy group, in which case belief alignment is not able to motivate actors to engage in both debates at all. Second, this result speaks to the isolation of actors in the PRO coalition observed elsewhere (Hilton et al., Citation2020). Health charities and advocacy groups missed out on the potential to mobilise across debates, despite presumably firm beliefs in their cause. Finally, political parties and government actors show more cross-sectoral coordination than other actor types, but the last two scenarios show that this is driven to a large extent by the two main variables, belief extremeness and spread. With average beliefs and spread within their respective coalitions, their probability of cross-policy activity is rather close to zero.
Figure 5. Predicted probabilities of cross-policy advocacy for nine hypothetical scenarios, based on the second model (‘Mixed'). Point prediction marked by a red cross, with the 95% confidence interval represented by error bars.
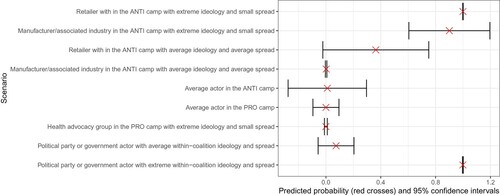
In an additional model specification reported in the last column of , we tested whether the composite variable of ideological spread could be further unpacked into constituent factors, following the discussion of three underpinning mechanisms above. Ideological spread affects cross-sectoral advocacy most systematically through the number of unstated beliefs, which is the only significant predictor among the three. Actors who specialise on a few selected beliefs in the policy debate are estimated to have a large ideological spread by the IRT model because their belief portfolio leaves much room for speculation about the beliefs they do not reference. In line with the hypothesis, such lack of engagement leads to a lower propensity to engage in cross-sectoral activities and an exclusive focus on a single policy debate. As hypothesised, a lack of engagement through the number of unstated beliefs is also correlated with the extremeness of one’s ideological position; hence the number of unstated beliefs acts as a mediator both for spread and extremeness, which becomes apparent by the now insignificant coefficient for extremeness of beliefs. Were this uncertainty in the posterior distribution factored directly into the estimation of the effect of extremeness on cross-sectoral advocacy, instead of using the posterior mean as a point estimate, then this would consequently lead to an insignificant result for extremeness. Actors who hold extreme beliefs state their beliefs explicitly and engage in cross-sectoral advocacy. Actors who hold moderate beliefs engage less and show a lower probability of cross-sectoral advocacy. Controlling for engagement thus renders the extremeness of ideology over and above engagement unimportant. The variance of the beliefs that are indeed stated, as measured through the standard deviation of the positively or negatively weighted discrimination parameters of stated beliefs per actor, is not a significant mediator of the effect of spread. Neither is the number of beliefs over which actors contradict themselves. Thus, extreme beliefs and low spread in expressed beliefs jointly determine cross-sectoral advocacy efforts. They are jointly mediated by lack of engagement.
Conclusion
Explanations of cross-sectoral advocacy have been somewhat opaque in the literature on policy processes and advocacy. The empirical evidence in this study suggests that belief system alignment is associated with such efforts. This speaks to the literature on advocacy coalitions (Sabatier, Citation1998) and policy entrepreneurship across boundaries (Faling et al., Citation2019). Extreme and well-defined ideological positions relative to one’s coalition are associated with more cross-sectoral advocacy efforts across similar policy debates. This is irrespective of frequency of involvement and coalition membership but may hold more for some actor types (here: retailers, manufacturers) than others (health charities and advocacy groups). Future research will need to replicate this finding in other contexts and case studies to find out what kinds of actor types demonstrate this behaviour (or absence of behaviour) systematically.
More research is also needed regarding the causal direction of the relationships documented here. Theoretically, it may be more plausible that belief system alignment and engagement cause cross-sectoral advocacy than that positions and ideological uncertainty are caused by cross-sectoral policy debate participation. This reverse causal path should be either confirmed or eliminated in future research. While the observations on both beliefs and cross-sectoral advocacy are temporal over the course of several years, the observations had to be aggregated into a cross-sectional dataset to permit ideological scaling. It may be possible to obtain temporally smoothed ideological positions with temporal variation to construct a panel or event history dataset, but the obtained estimates would likely be much less precise and might fail to permit inference on the role of relative position and spread.
The literature on policy containers and expanders posits that those wishing to contain a policy try to sweep the policy under the rug by also containing actor involvement in the debate (e.g., Schaub & Metz, Citation2020). Ironically, policy containers seem to be precisely the ones who engage in multiple sectoral debates to attain this goal.
The methodological approach taken in this article is novel in the field of advocacy research and policy processes. The application of IRT to the coalition structure in policy debates permits simultaneous estimation of the belief system position of actors and beliefs relative to each other as well as the discriminatory power of policy beliefs for coalition membership. This approach not only lends itself to explaining advocacy behaviour but also allows principled description of coalitions in a policy debate by reverting to the belief categories of which advocacy coalitions are composed. The approach can be applied to media text data (like in this analysis) or any other text data exhibiting actors’ positions on relevant policy beliefs, with the caveat that each type of source document may only depict a certain ‘arena' of the entire underlying actor and belief constellation. The method complements approaches stressing the complexity and higher-order dimensionality of subsystems and coalitions, such as discourse network analysis (Leifeld, Citation2013; Citation2017), by offering a scaling solution for policy debates, which can be intrinsically one-dimensional (though this may depend on the type of belief measured and is ultimately an empirical question). We recommend this approach over more complex approaches like discourse network analysis when it is clear that the ideological spectrum is one-dimensional (e.g., a debate around a single policy), while discourse network analysis is more appropriate for the analysis of whole subsystems with multiple policies or cleavage lines, as in Leifeld (Citation2013), or policy conflicts with multiple non-opposite policy options on the table.
Finally, the case examined here suggests a significant unrealised potential for cross-sectoral policy advocacy efforts by public health charities and advocacy groups. Their opponents in the conflict around unhealthy commodity regulation already leverage cross-policy synergies as a strategic resource to strengthen their advocacy efforts, and the PRO-regulation camp would do well to adopt the same strategy in the interest of public health. However, future research is needed to evaluate how much more effective cross-sectoral advocacy really is compared to policy specialisation in a single debate.
Supplemental Materials
Download Zip (164.8 KB)Acknowledgments
The authors would like to thank three anonymous reviewers for helpful feedback. SH, GF and CB were supported by UK Medical Research Council and Chief Scientist Office core funding as part of the MRC/CSO Social and Public Health Sciences Unit Policy programme (2015-2020), MC_UU_12017/15; SPHSU15; and Complexity in Health programme (2020-2025), MC_UU_00022/1; SPHSU16.
Disclosure statement
No potential conflict of interest was reported by the author(s).
Additional information
Notes on contributors
Philip Leifeld
Philip Leifeld is a Professor of Comparative Politics in the Department of Government at the University of Essex, UK.
Tim Henrichsen
Tim Henrichsen is a Postdoctoral Researcher in the Department of Politics and International Studies at the University of Warwick, UK.
Christina Buckton
Christina Buckton is a Research Assistant in the MRC/CSO Social & Public Health Sciences Unit at the University of Glasgow, UK.
Gillian Fergie
Gillian Fergie is a Postdoctoral Research Associate in the MRC/CSO Social & Public Health Sciences Unit at the University of Glasgow, UK.
Shona Hilton
Shona Hilton is a Professor of Public Health Policy and Deputy Director of the MRC/CSO Social & Public Health Sciences Unit at the University of Glasgow, UK.
References
- Albert, J. H. (1992). Bayesian estimation of normal ogive item response curves using Gibbs sampling. Journal of Educational Statistics, 17(3), 251–269. https://doi.org/https://doi.org/10.3102/10769986017003251
- Brandenberger, L., Ingold, K., Fischer, M., Schläpfer, I., & Leifeld, P. (2021). Boundary spanning through engagement of policy actors in multiple issues. Policy Studies Journal. Forthcoming. https://doi.org/https://doi.org/10.1111/psj.12404
- Buckton, C. H., Fergie, G., Leifeld, P., & Hilton, S. (2019). A discourse network analysis of UK newspaper coverage of the “sugar tax” debate before and after the announcement of the Soft Drinks Industry levy. BMC Public Health, 19(1), 1–14. https://doi.org/https://doi.org/10.1186/s12889-019-6799-9
- Candel, J. J. (2017). Holy grail or inflated expectations? The success and failure of integrated policy strategies. Policy Studies, 38(6), 519–552. https://doi.org/https://doi.org/10.1080/01442872.2017.1337090
- Clinton, J., Jackman, S., & Rivers, D. (2004). The statistical analysis of roll call data. American Political Science Review, 98(2), 355–370. https://doi.org/https://doi.org/10.1017/S0003055404001194
- Cobb, R. W., & Coughlin, J. F. (1998). Are elderly drivers a road hazard? Problem definition and political impact. Journal of Aging Studies, 12(4), 411–427. https://doi.org/https://doi.org/10.1016/S0890-4065(98)90027-5
- Collin, J., Hill, S. E., Eltanani, M. K., Plotnikova, E., Ralston, R., & Smith, K. E. (2017). Can public health reconcile profits and pandemics? An analysis of attitudes to commercial sector engagement in health policy and research. PloS ONE, 12(9), e0182612. https://doi.org/https://doi.org/10.1371/journal.pone.0182612
- Däubler, T., & Benoit, K. (2017). Estimating better left-right positions through statistical scaling of manual content analysis. Unpublished Manuscript. https://pdfs.semanticscholar.org/9eb7/6a90e29fe43db2c4192ef2df5f4d73aa0567.pdf (accessed 9 March 2021).
- Faling, M., Biesbroek, R., Karlsson-Vinkhuyzen, S., & Termeer, K. (2019). Policy entrepreneurship across boundaries: A systematic literature review. Journal of Public Policy, 39(2), 393–422. https://doi.org/https://doi.org/10.1017/S0143814X18000053
- Fergie, G., Leifeld, P., Hawkins, B., & Hilton, S. (2019). Mapping discourse coalitions in the minimum unit pricing for alcohol debate: A discourse network analysis of UK newspaper coverage. Addiction, 114(4), 741–753. https://doi.org/https://doi.org/10.1111/add.14514
- Fischer, F., & Forester, J. (1993). The Argumentative Turn in Policy Analysis and Planning. Duke University Press.
- Freudenberg, N. (2014). Lethal but Legal: Corporations, Consumption, and Protecting Public Health. Oxford University Press.
- Heaney, M. T., & Leifeld, P. (2018). Contributions by interest groups to lobbying coalitions. Journal of Politics, 80(2), 494–509. https://doi.org/https://doi.org/10.1086/694545
- Henry, A. D., Lubell, M., & McCoy, M. (2011). Belief systems and social capital as drivers of policy network structure: The case of california regional planning. Journal of Public Administration Research and Theory, 21(3), 419–444. https://doi.org/https://doi.org/10.1093/jopart/muq042
- Hilton, S., Buckton, C. H., Henrichsen, T., Fergie, G., & Leifeld, P. (2020). Policy congruence and advocacy strategies in the discourse networks of minimum unit pricing for alcohol and the soft drinks industry levy. Addiction, 115(12), 2303–2314. https://doi.org/https://doi.org/10.1111/add.15068
- Ingold, K. (2011). Network structures within policy processes: Coalitions, power, and brokerage in Swiss climate policy. Policy Studies Journal, 39(3), 435–459. https://doi.org/https://doi.org/10.1111/j.1541-0072.2011.00416.x
- Ingold, K., & Leifeld, P. (2016). Structural and institutional determinants of influence reputation: A comparison of collaborative and adversarial policy networks in decision making and implementation. Journal of Public Administration Research and Theory, 26(1), 1–18. https://doi.org/https://doi.org/10.1093/jopart/muv041
- Jenkins-Smith, H. C., St. Clair, G. K., & Woods, B. (1991). Explaining change in policy subsystems: Analysis of coalition stability and defection over time. American Journal of Political Science, 35(4), 851–880. https://doi.org/https://doi.org/10.2307/2111497 .
- Junk, W. M. (2019). When diversity works: The effects of coalition composition on the success of lobbying coalitions. American Journal of Political Science, 63(3), 660–674. https://doi.org/https://doi.org/10.1111/ajps.12437
- Laffont, J. J., & Tirole, J. (1991). The politics of government decision-making: A theory of regulatory capture. The Quarterly Journal of Economics, 106(4), 1089–1127. https://doi.org/https://doi.org/10.2307/2937958
- Leifeld, P. (2013). Reconceptualizing major policy change in the advocacy coalition framework: A discourse network analysis of German pension politics. Policy Studies Journal, 41(1), 169–198. https://doi.org/https://doi.org/10.1111/psj.12007
- Leifeld, P. (2017). Discourse network analysis: Policy debates as dynamic networks. In J. N. Victor, A. H. Montgomery, & M. N. Lubell (Eds.), The Oxford Handbook of Political Networks (pp. 301–325). Oxford University Press.
- Leifeld, P., & Haunss, S. (2012). Political discourse networks and the conflict over software patents in Europe. European Journal of Political Research, 51(3), 382–409. https://doi.org/https://doi.org/10.1111/j.1475-6765.2011.02003.x
- Mahoney, C. (2007). Networking vs. allying: The decision of interest groups to join coalitions in the US and the EU. Journal of European Public Policy, 14(3), 366–383. https://doi.org/https://doi.org/10.1080/13501760701243764
- Martin, A. D., Quinn, K. M., & Park, J. H. (2011). MCMCpack: Markov chain Monte Carlo in R. Journal of Statistical Software, 42(9), 1–21. https://doi.org/https://doi.org/10.18637/jss.v042.i09
- Moodie, R., Stuckler, D., Monteiro, C., Sheron, N., Neal, B., Thamarangsi, T., Lincoln, P., & Casswell, S. (2013). Profits and pandemics: Prevention of harmful effects of tobacco, alcohol, and ultra-processed food and drink industries. The Lancet, 381(9867), 670–679. https://doi.org/https://doi.org/10.1016/S0140-6736(12)62089-3
- Petticrew, M., Shemilt, I., Lorenc, T., Marteau, T. M., Melendez-Torres, G. J., O'Mara-Eves, A., Stautz, K., & Thomas, J. (2017). Alcohol advertising and public health: Systems perspectives versus narrow perspectives. Journal of Epidemiology and Community Health, 71(3), 308–312. https://doi.org/https://doi.org/10.1136/jech-2016-207644
- Sabatier, P. A. (1988). An advocacy coalition framework of policy change and the role of policy-oriented learning therein. Policy Sciences, 21(2-3), 129–168. https://doi.org/https://doi.org/10.1007/BF00136406
- Sabatier, P. A. (1998). The advocacy coalition framework: Revisions and relevance for Europe. Journal of European Public Policy, 5(1), 98–130. https://doi.org/https://doi.org/10.1080/13501768880000051
- Savell, E., Fooks, G., & Gilmore, A. B. (2016). How does the alcohol industry attempt to influence marketing regulations? A systematic review. Addiction, 111(1), 18–32. https://doi.org/https://doi.org/10.1111/add.13048
- Schaub, S., & Metz, F. (2020). Comparing discourse and policy network approaches: Evidence from water policy on micropollutants. Politics and Governance, 8(2), 184–199. https://doi.org/https://doi.org/10.17645/pag.v8i2.2597
- Smith, K., Dorfman, L., Freudenberg, N., Hawkins, B., Hilton, S., Razum, O., & Weishaar, H. (2016). Tobacco, alcohol, and processed food industries–why do public health practitioners view them so differently? Frontiers in Public Health, 4(64), 1–3. https://doi.org/https://doi.org/10.3389/fpubh.2016.00064
- Tosun, J., & Lang, A. (2017). Policy integration: Mapping the different concepts. Policy Studies, 38(6), 553–570. https://doi.org/https://doi.org/10.1080/01442872.2017.1339239
- Tosun, J., & Schaub, S. (2017). Mobilization in the European public sphere: The struggle over genetically modified organisms. Review of Policy Research, 34(3), 310–330. https://doi.org/https://doi.org/10.1111/ropr.12235
- Trein, P., Meyer, I., & Maggetti, M. (2019). The integration and coordination of public policies: A systematic comparative review. Journal of Comparative Policy Analysis, 21(4), 332–349. https://doi.org/https://doi.org/10.1080/13876988.2018.1496667