ABSTRACT
Resources for development are used efficiently when social programs help to promote at the same time the sustainability of grass-root financial associations at the bottom of the pyramid. This study applies machine-learning to a worldwide database of grass-root associations in order to identify which social programs are good predictors of financial returns in the groups. The results indicate that education, income-generating activities and health programs are the most frequent programs provided by development agencies. Business training is not the most frequent intervention applied to grass-root associations, but it is in fact the most important social program to encourage financial sustainability, particularly after a development agency stops working with a group and leaves the community. Theoretical and practical implications of the findings are discussed.
1. Introduction
Financial access to low-income households in developing countries tends to be provided by micro-finance institutions. In contrast to this institutional approach, in grass-root finance individuals living in impoverished communities create a group and start to accumulate their savings into a fund, which is later used to provide small loans to themselves.
Grass-root groups receive different names, like inter alia savings groups (Allen and Panetta Citation2010), self-help groups (Venkatraja Citation2019), rotating savings and credit associations or accumulating savings and credit associations (Bouman Citation1995). Greaney et al. (Citation2016) and Burlando and Canidio (Citation2017) estimate that over 100 million persons in 10.5 million households participate in grass-root financial groups worldwide.
Studying the dynamics of informal grass-root finance is extremely important due to the recent evidence that expanding the access to formal savings and loans will not be enough to broaden financial access to the poor (Dupas et al. Citation2018). Grass-root finance arises at the bottom of the pyramid and reaches the poorest population in developing countries, who do not have access to formal financial services and rely exclusively on grass-root finance to meet their needs (Burlando and Canidio Citation2017).
International donors and development agencies have recognized the relevance of grass-root finance for poverty reduction. These organizations work with grass-root groups as a platform to provide communities with sustainable development programs. The agencies help a community to organize a financial group, and then provide a development service to the group, like entrepreneurship, agriculture, adaptation to climate change, health and sanitation, or programs of literacy, education and women empowerment.
Examples of grass-root financial associations supported by development agencies are the Village Savings and Loan Association (VSLA) promoted by CARE International or the Savings for Changes (SfC) model supported by Oxfam and Freedom from Hunger (Le Polain et al. Citation2018). During the implementation of social and financial programs, development agencies work closely with donors like the Inter-American Development Bank or the Barclays Corporation (Flynn and Sumberg Citation2017).
Given the wide variety of sustainable development programs that agencies and donors can provide to grass-root groups, the question arises as to which program helps to promote both the social and financial sustainability of a group.
A group is financially sustainable when generates returns during the process of savings accumulation and loan provision. Groups that generate returns will have incentives to keep operating over time and thus will continue providing financial access to bottom-of-the-pyramid (BoP) individuals. Groups that are financially sustainable may also opt to maintain their social programs alive, even after the development organization leaves the community.
This study uses machine-learning methods to identify which social development programs are the best predictors of financial returns generated by grass-root associations. Text-mining and random forests are applied to the Savings Groups Information Exchange (SAVIX), a database with information of more than 250000 grass-root financial associations worldwide.
The results indicate that education, income-generating activities and health are the most frequent development programs provided to grass-root groups. Training to create small retail businesses and health interventions are provided less frequently, but interestingly these interventions are in fact the ones that boost the profit-generating capacity of grass-root groups, particularly business training in graduated groups that are no longer supervised by a development agency.
In the practice, the results imply that donors and development agencies that look to achieve social targets but also want to support financial sustainability can prioritize the provision of health programs and business training to grass-root groups. In combination with social interventions, encouraging groups to ‘graduate’ and become an autonomous and unsupervised association further enhances financial sustainability.
The findings also contribute to the development literature by suggesting that grass-root business stimulated by social interventions can be thought as a new paradigm of sustainable business, complementary to social enterprises and corporate social responsibility.
Next section describes the methods and data used in the study. Section 3 details the results and Section 4 concludes by discussing practical implications and contributions, as well as the links of the findings with the Sustainable Development Goals (SDGs).
2. Methods and data
Machine learning is applied to the SAVIX database in order to identify which sustainable development programs are the best predictors of the profit-generating capacity of grass-root financial organizations across the world.
The SAVIX database has information of 250000 grass-root financial groups in 52 countries around the world (). The group-level data of the SAVIX is collected in the field through an online system, the Savings Groups Management Information System (MIS), which is supported by the Bill & Melinda Gates Foundation, CARE, Catholic Relief Services, Oxfam America and Plan International.
Figure 1. Geographical distribution of grass-root financial groups in the SAVIX database. Half of the groups in the database are located in eight African countries: Uganda (22702 groups), Tanzania (21374 groups), Mali (21021 groups), Burkina Faso (13680 groups), Ghana (12337 groups), Mozambique (10244 groups), Senegal (10148 groups) and Kenya (8906 groups).
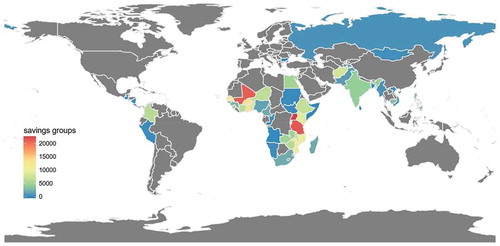
Machine-learning are supervised, semi-supervised or unsupervised algorithms that allow to make predictions and create data-driven knowledge from a database. Applications of machine-learning in sustainable development are promoted by the United Nations Data Revolution Group (United Nations, Citation2014). Recent applications of machine-learning include the identification of harmful environmental impacts caused by unsustainable business (Can and Alatas, Citation2017) and the application of machine-learning for monitoring the SDG indicators (Holloway et al. Citation2018).
In this study, two common machine learning methods are applied to the SAVIX: text-mining and random forests.
Text-mining is an unsupervised process that seeks to extract useful information and identify patterns in textual data (Feldman and Sanger Citation2007). The text-mining implementation in this study transforms the unstructured information of social interventions in the SAVIX into a corpus, i.e. a collection of writing data about sustainable development programs. The corpus is processed and summarized into a matrix of tokens, which is analyzed to find word frequencies and patterns. The text-mining results are displayed with word-clouds, where the word size denotes the frequency of a word in the corpus – see Weiss et al. (Citation2015), Vijayarani et al. (Citation2015) or Zhou et al. (Citation2016).
Random forest are supervised algorithms that fit decision-trees to random subsets of training data and use the combined result – the forest – for prediction (Breiman Citation2001). Decision-trees split the dataset into smaller subsets, with the aim of increasing the predictive power of the model for the target variable (Genuer et al. Citation2017). In this study, the individual predictions from the trees estimated with the SAVIX are combined into a final prediction of financial returns obtained by the groups. The importance of each variable for returns is calculated with node impurity – a measure of the splits that have a high inter-node variance and a small intra-node variance – and with the increase in the mean squared error of predictions (see Gregorutti et al. Citation2017).
A final decision-tree is estimated with the full sample (train and test) in order to find out which development programs are the best predictors of the profit-generating capacity of grass-root financial associations.
3. Results
The SAVIX data shows that grass-roots finance is provided to the poorest population living in rural communities and urban slums in developing countries. In the database, 65% of the groups operate in rural regions, 33% in urban slums and only 2% in urban regions. The average amount of savings per member in the grass-root groups is 17.5 USD, with a median of 9.5 USD. The value of loans provided to the members is on average only 12 USD, with a median of 6 USD (). The low values of savings and loans show that grass-root finance is a source of financial services for the extreme poor in the BoP.
Table 1. Descriptive statistics of the variables in the SAVIX database.
Despite the low values of savings and loans in grass-roots associations, these groups have on average returns on savings (ROS) equal to 45%, with a median of 36% (). Allen and Panetta (Citation2010) explain that the high financial returns of grass-roots associations are the consequence of groups charging monthly interest rates ranging from 5 to 10 percent. Guha and Gupta (Citation2005) add that because members must repay the loan and pay interests, as well as keep contributing with their savings, a surplus that boosts returns arises naturally in grass-root associations.
Figure 2. Left: Histogram of returns on savings (ROS) of grass-root groups in the SAVIX. Right: Scatterplot of the ROS in the trained and test samples used for cross-validation in the random forest.
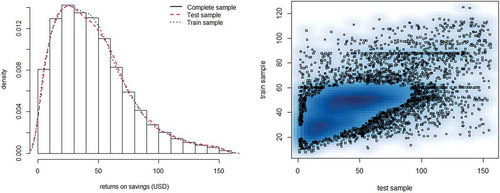
In the SAVIX, 57863 grass-root groups have records of having received a social intervention from a development agency. The text-mining of the corpus of development interventions indicates that the most frequent programs provided to grass-root groups are related to education, income-generating activities and health (, ). Business training is not among the most frequent interventions applied in grass-root financial associations.
Table 2. Text-mining results for the 12 more frequent words in the records of development services offered to grass-root financial associations in the SAVIX. The textual records of development programs in the SAVIX database are written in English, French and Portuguese. The texts in French and Portuguese were translated to English. IGA: income-generating activities.
Figure 3. Text-mining of the sustainable development programs provided to grass-root associations in the SAVIX. The size of the words represents the frequency of the records (higher frequency, higher size). As part of the text-mining exercise, the records of development programs in the SAVIX were transformed into a lower-case corpus and were cleaned from special characters, English stop-words, punctuations, extra white spaces and numbers.
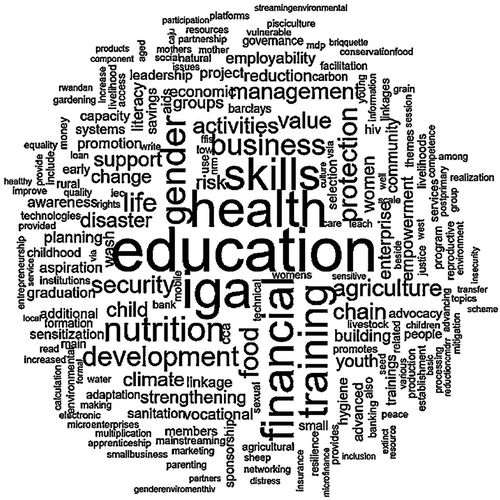
One-hot encoding was used to translate the corpus to a binary matrix of development programs. The matrix was included in the random-forest model to estimate which social interventions predict higher returns. In order to control for other variables that could affect profit generation, group-level characteristics and macro-economic variables were added as controls in the random forests.Footnote1
The number of variables that are randomly selected for splitting at each node of the trees are selected with cross-validation.Footnote2 In the cross-validation, the database is randomly divided in a validation and a train set, with 65% of the data in the train set. The minimum value of both the root mean squared error (RMSE) and the mean absolute prediction error (MAPE) is obtained with 5 splitting variables (). Five splitting variables also maximize the correlation of predictions in the test set against the estimations of ROS in the train set ( and ).Footnote3
Table 3. Cross-validation results to select the number of splitting variables in the models of random forests.
illustrates the results about the importance of each variable for profit generation obtained with a random forest that has 5 splitting variables at each node.
Figure 4. Results of the random forests. The importance of each variable for the generation of returns in grass-root financial associations is measured with the increase in the mean squared error of prediction (IncMSE, left) and the increase in node impurity (IncNodePurity, right). IncMSE is the error of prediction caused by a specific variable being excluded from the model. In the case of IncNodePurity, the most relevant variables have a higher inter node variance and a smaller intra node variance.
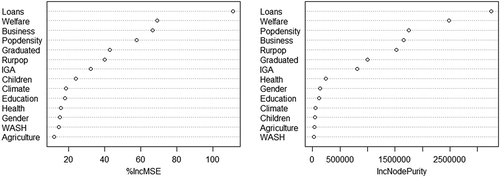
The highest increase in MSE and node impurity is obtained for the variable loans per member, indicating that loans are the main predictor of returns in grass-root financial associations. This result is expected, because loan allocation is the main channel of profit generation in grass-root associations. The fact that loans are identified as the best predictor of profit generation supports the ability of the machine-learning algorithm to truly detect predictors of financial returns.
The welfare fund is the second most important factor that predicts higher returns in grass-root financial associations (). While the purpose of the welfare fund is to offer grants or interest-free loans to cover emergencies and life-cycle events, Maliti (Citation2017) found that groups in Tanzania appeal to the welfare fund for loan repayment, a deviation from the original purpose of the welfare fund that can increase returns.
In relation to development interventions, agricultural programs and training in WASH (water, sanitation and hygiene) have the lowest effect on financial returns (), while in contrast development programs for the creation of small retail businesses have the highest predictive power for the generation of returns in grass-root financial associations.
Similar results are obtained with the final decision-tree estimated with the full dataset (). Graduated grass-root groups that receive business training (1149 groups) have a median of ROS equal to 99%, with an interquartile range of 37% to 126% (Node 15, ). Groups that receive business training but are still supervised by a development agency have a median of ROS equal to 75%, if the group has a welfare fund equal or higher than 1.24 USD (Node 14, ). Groups that received business training but are still under supervision and have a smaller amount of money in the welfare fund (less than 1.24 USD per member) have a median of ROS equal to 48% (Node 13, ).
Figure 5. Decision tree of sustainable development programs offered to grass-root financial associations. In the graph, n is the number of grass-root groups in each node of the tree.
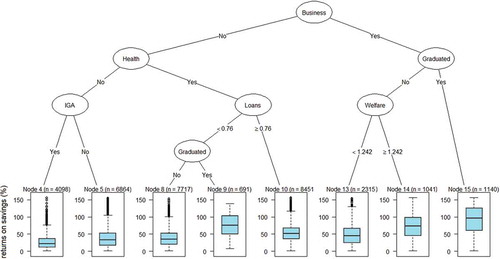
Health development programs are the second-best predictor of returns in grass-root financial associations, when the groups manage to provide loans to their members. Graduated groups that have received training in health and at the same time allocate loans have an average rate of returns equal to 75% (Node 9, ).
If no health or business programs are offered to the groups, social programs aimed to promote income generating activities can also boost the financial sustainability of the groups (, Nodes 4 and 5), but on a lower magnitude compared to groups that receive business training and are no longer under the active supervision of a development agency.
4. Discussion
Higher returns in grass-root financial associations ensure sustainable finance and the continuity of business and social development interventions. Groups with higher profits will have more incentives to keep operating over time, and thus will keep providing informal financial services to the poor. Likewise, financially sustainable groups will keep supplying social development programs to vulnerable populations for longer periods of time.
The machine-learning results of this study show that the most frequent development interventions applied to grass-root financial associations are related to education, income-generating activities and health. Business training is not the most frequent development program offered to grass-root associations, but it is in fact the most important predictor of financial returns, particularly for graduated groups already trained by development organizations.
In the practice, the findings imply that international donors and non-governmental organizations interested in both social and financial sustainability can make an efficient use of scarce resources by implementing interventions of health and business training, because these programs will simultaneously promote both financial and social welfare of low-income individuals participating in grass-root associations. The results also indicate that the staff hired by development organizations must provide enough training to allow groups to achieve graduation. The training staff needs to encourage leadership and trust within a group, as well as proactive membership and the ability and motivation to follow group rules (Delany and Storchi Citation2012).
The findings of the study also contribute to the development literature on sustainable finance by suggesting that grass-root business stimulated by social interventions can be thought as a new paradigm of sustainable businesses at the BoP, complementary to the traditional paradigms of social enterprises (Hossain et al. Citation2017) and corporate social responsibility (Kolk Citation2016).
In the case of corporate social responsibility, Hoque et al. (Citation2018) suggest that – both in developed and developing countries – this paradigm tends to be a voluntary philanthropic fashion not necessarily focused on improving social well-being but rather aimed to build public image and enhance business profit. The businesses stimulated by grass-root financial organizations are on the contrary created and owned by the members, and thus are primarily interested in improving the lives of the participants.
Social enterprises on the other hand can be imposed on the poor by governments and developing agencies. In contrast to this centralized and authoritarian business model, grass-root businesses spawned by grass-root financial associations answer to the necessities, potentialities and expectations of the members. Social enterprises also tend to prioritize social value over economic value (Seelos and Mair Citation2005), while in contrast the small businesses promoted by grass-roots groups focus on both, social and economic value.
Business creation through grass-root finance is hence a community model that helps households to smooth consumption and also aids individuals to self-finance their productive investments in human and business capital (Karlan and Zinman, Citation2014). In the businesses created through sustainable grass-root financial organizations, individuals raise capital from their community and invest their money locally. Since grass-root businesses are locally owned and locally financed, wealth-creation remains in the community.
Finally, this study has also implications for the efficient allocation of resources of governments, donors and development agencies interested in achieving the SDGs. The development programs promoted through grass-root financial organizations are linked to the SDGs 1 (no poverty), 2 (zero hunger), 3 (health), 4 (education), 5 (gender equality), 6 (water and sanitation), 8 (decent work and economic growth) and 13 (climate action). The results show that grass-root groups that receive health interventions and business training can achieve simultaneously multiple targets of the SDGs 8, 3 and 1.
Grass-root financial groups can also be a platform to implement other development interventions, as for example programs to reduce psychological or physical aggression to children (SDG 16) or projects to provide sources of renewable energy (SDG 7)Footnote4. Future studies can explore the simultaneous social and financial impact of these interventions, in order to improve the efficient use of resources for development and create insights about additional opportunities to make a stronger impact on the well-being of people around the world.
Acknowledgments
The author would like to thank Roy Mersland, Bert D’Espallier, Linda Nakato and Annikka Berridge.
Disclosure statement
No potential conflict of interest was reported by the author.
Additional information
Funding
Notes
1. The number of loans per member is included as a control covariate of operating efficiency and the status of the group – supervised against graduated – is used to account for the stage of agency monitoring (Ledgerwood et al. Citation2013). The welfare fund of a group was included as a control because the social fund can act as a collateral mechanism to cope with risks. Population density and the percentage of rural population of a country are included as macroeconomic controls because in less populated rural areas transport costs limit the possibilities of members attending meetings, thus increasing the chances of members not contributing with their savings and/or not paying their debts to the group (Christensen Citation1993).
2. Nicodemus and Shugart (Citation2007) and Strobl et al. (Citation2008) highlight that the ability of random forests to detect influential predictor variables depends on the number of selected splitting variables.
3. Out-of-bag (OOB) errors are used to validate the random forest model. The OOB error is the average error calculated using bootstrapped predictions from the trees with a specific number of splitting variables randomly permuted during the estimation of the forest (Gregorutti et al. Citation2017). An OOB error of 601.56 is obtained with 4 splitting variables, 594.91 with 5 splitting variables and 597.26 with 6 splitting variables.
4. ARED (African Renewable Energy Distributor) for example, is a micro-franchising business at the BoP that offers solar-powered mobile kiosks in Africa (Gabriel and Kirkwood Citation2016). This business model can be combined with grass-root finance to achieve simultaneously multiple goals of the SDGs 7, 8 and 1.
References
- Allen H, Panetta D. 2010. Savings groups: what are they? Technical Report. SEEP Network.
- Bouman F.J. 1995. Rotating and accumulating savings and credit associations: A development perspective. World Dev. 23(3):371–384.
- Breiman L. 2001. Random forests. Mach Learn. 45:5–32.
- Burlando A, Canidio A. 2017. Does group inclusion hurt financial inclusion? Evidence from ultra-poor members of Ugandan savings groups. J Dev Econ. 128:24–48.
- Can U, Alatas B. 2017. Big social network data and sustainable economic development. Sustainability. 9(11):2027.
- Christensen G. 1993. The limits to informal financial intermediation. World Dev. 21:721–731.
- Delany A, Storchi S. 2012. SaveAct savings and credit groups and small enterprise development. South Africa: FinMark Trust.
- Dupas P, Karlan D, Robinson J, Ubfal D. 2018. Banking the unbanked? evidence from three countries. Am Econ J: Appl Econ. 10:257–297.
- Feldman R, Sanger J. 2007. The text mining handbook: advanced approaches in analyzing unstructured data. New York, NY: Cambridge university press.
- Flynn J, Sumberg J. 2017. Youth savings groups in Africa: they’re a family affair. Enterp Dev Microfinance. 28(3):147–161.
- Gabriel CA, Kirkwood J. 2016. Business models for model businesses: lessons from renewable energy entrepreneurs in developing countries. Energy Policy. 95:336–349.
- Genuer R, Poggi J-M, Tuleau-Malot C, Villa-Vialaneix N. 2017. Random forests for big data. Big Data Res. 9:28–46.
- Greaney BP, Kaboski JP, Van Leemput E. 2016. Can self-help groups really be self-help? Rev Econ Stud. 83(4):1614–1644.
- Gregorutti B, Michel B, Saint-Pierre P. 2017. Correlation and variable importance in random forests. Stat Comput. 27:659–678.
- Guha S, Gupta G. 2005. Microcredit for income generation: the role of RoSCA. Econ Polit Wkly. 40(14):1470–1473.
- Holloway J, Mengersen K, Helmstedt K. 2018. Spatial and machine learning methods of satellite imagery analysis for sustainable development goals. 16th Conference of the International Association of Official Statisticians (IAOS); Sep 19–21; Paris, France.
- Hoque N, Rahman ARA, Molla RI, Noman AHM, Bhuiyan MZH. 2018. Is corporate social responsibility pursuing pristine business goals for sustainable development? Corporate Social Resp Environ Manage. 25:1130–1142.
- Hossain S, Saleh MA, Drennan J. 2017. A critical appraisal of the social entrepreneurship paradigm in an international setting: a proposed conceptual framework. Int Entr Manage J. 13:347–368.
- Karlan Ratan L, Zinman J. 2014. Savings by and for the poor: A research review and agenda. Rev Income Wealth. 60:36–78.
- Kolk A. 2016. The social responsibility of international business: from ethics and the environment to CSR and sustainable development. J World Bus. 51:23–34.
- Le Polain M, Sterck O, Nyssens M. 2018. Interest rates in savings groups: thrift or threat? World Dev. 101:162–172.
- Ledgerwood J, Earne J, Nelson C. 2013. The new microfinance handbook: a financial market system perspective. NW Washington, DC: The World Bank.
- Maliti E. 2017. Deviation of community savings groups from their apparent methodology: lessons from the field. Int J Soc Econ. 44:326–336.
- Nicodemus K, Shugart Y. 2007. Impact of linkage disequilibrium and effect size on the ability of machine learning methods to detect epistasis in case-control studies. In Genetic Epidemiology. 31(6):611–611.
- Seelos C, Mair J. 2005. Social entrepreneurship: creating new business models to serve the poor. Bus Horiz. 48:241–246.
- Strobl C, Boulesteix A.L, Kneib T, Augustin T and Zeileis A. 2008. Conditional variable importance for random forests. BMC Bioinformatics. 9(1):307.
- United Nations Secretary-General’s Independent Expert Advisory Group on a Data Revolution for Sustainable Development 2014. A world that counts mobilizing the data revolution for sustainable development. Technical Report, Data Revolution Group.
- Venkatraja B. 2019. Are SHGs catalysts of inclusive sustainable rural development? Impact assessment of SKDRDP interventions in India. Int J Sustainable Dev World Ecol. 26(4):302–312.
- Vijayarani S, Ilamathi MJ, Nithya M. 2015. Preprocessing techniques for text mining-an overview. Int J Comput Sci Commun Networks. 5:7–16.
- Weiss SM, Indurkhya N, Zhang T. 2015. Fundamentals of predictive text mining. London: Springer.
- Zhou Y, Tong Y, Gu R, Gall H. 2016. Combining text mining and data mining for bug report classification. J Software: Evol Process. 28:150–176.