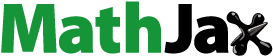
ABSTRACT
In all European countries unemployed persons face a high risk of relative poverty, but poverty rates vary greatly among EU countries. We analyse to what extent these differences could be explained by a different composition of the unemployed or by differences in the national income distribution functions. Our results indicate that the effects of individual characteristics on the poverty risk are roughly comparable between countries, but the composition of the unemployed is very different, which explains on average half of the cross-country differences in poverty rates.
I. Introduction
The relative risk of poverty, defined by living below the poverty line of 60% of the median disposable income, is one of the leading social indicators within the European Union. In all countries, unemployed persons face a particularly high risk of relative poverty since they are lacking earned income. However, there is also substantial cross-country heterogeneity. In 2013, the poverty rate of the unemployed amounted to around 70% in Germany, whereas it was below 30% in Denmark and 47% in the EU-28. Several factors contribute to these cross-country differences, e. g. differences in national income protection systems. Apart from redistribution, the structure of households and the distribution of individual characteristics play a major role. The present note provides evidence that differences in the composition of the unemployed can explain to a significant extent cross-country differences in poverty rates of the unemployed.
II. Data and methodology
We use the cross-sectional component of the Scientific Use File (SUF) of EU-SILC 2014 (European Union Statistics on Income and Living Conditions) provided by Eurostat (Eurostat Citation2013).Footnote1 EU-SILC is based on yearly national representative surveys of private households in the 28 EU member states. We calculate the national poverty threshold as 60% of the median of the equivalized disposable income of the total population, using total disposable income provided in EU-SILC and the modified OECD equivalence scale. In accordance with the Eurostat statistics on poverty and social exclusion, we classify someone as unemployed if (s) he was unemployed for more than six months during the reference year, which is the preceding year (2013).
We focus on six European countries: Belgium (BE), Germany (DE), Spain (ES), Sweden (SE), Slovakia (SK) and the United Kingdom (UK). These countries were selected for two reasons: First, they differ with regard to the poverty risk of the unemployed, with very high (DE, UK), close to the EU-28 average (SK, ES) and low (BE, SE) poverty rates (see ).Footnote2 Second, the six countries represent a variety of welfare state types, according to the well-known welfare state typology of Esping-Andersen (Citation1990) and later extensions and modifications of this typology.Footnote3 Sweden represents the ‘universalist’ social democratic welfare state model, Germany and Belgium stand for the ‘continental’ model, the UK comes close to the ‘liberal’ model, Spain represents the Mediterranean ‘rudimentary’ welfare state type and Slovakia belongs to the heterogeneous group of post-socialist welfare states.
Table 1. Poverty rates by country, 2013.
Table 2. Decomposition of cross-country poverty rate-differences.
Our sample comprises 124,680 observations with 7,503 unemployed respondents. We keep only observations with positive household incomes and valid values for the control variables considered in the analysis, which leaves us with 6,830 observations of unemployed individuals.Footnote4
We built on Biewen and Jenkins (Citation2005) and estimate a parametric specification of the distribution of the net household equivalence income for households with at least one unemployed person for each country, based on a Singh–Maddala (SM) distribution function (Bandourian Citation2003):
where ,
.Footnote5 in the Appendix shows a good fit between the sample and the theoretically expected quantiles, especially around the poverty lines (vertical lines), indicated by the closeness of the points to a 45
-line which stands for a perfect fit.
Figure A1. Quantile–quantile-plots for equivalent disposable income of the unemployed.
Source: Own calculation based on EU-SILC 2014
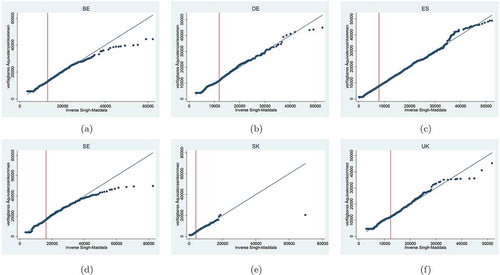
Next, we estimate the distribution models for each country conditional on individual and household characteristics. We choose household composition, the age of the unemployed, education and an indicator for a low work intensity as explanatory variables. Low work intensity means that the working age members in the household worked less than 20% of their potential working time during the reference year. in the Appendix shows a large variation of these variables across countries.
Last, we decompose poverty rate differences between two countries and
:
or
where the aggregate poverty rate is
with the poverty function of the subpopulation with the characteristics
, the poverty line
and the population distribution function
. The first difference in Equation (2),
, describes differences in the aggregated poverty rates due to cross-national differences in the poverty functions. The second difference
describes the difference due to a different composition of the unemployed in both countries. Alternatively, poverty rate differences could be decomposed into these two contributions in a reverse order:
We calculate all components of Equations (3) and (5) for all differences between the six countries, i. e. for 15 country pairs, whereas is the (weighted) distribution of the characteristics of unemployed below the poverty line in each country. We present the results as weighted average of both approaches shown in Equations (3) and (5) (Biewen and Jenkins Citation2005):
III. Estimation results
shows in the third row the estimated poverty risk for a reference household. The reference household is a low-qualified unemployed aged between 30 and 49 years, living in a household with two or more adults and with high work intensity. The rows below give the estimation results for the effect of a change of a characteristic on the poverty risk in percentage points, holding the other covariates constant. Living alone or as a lone parent leads to an increase in all countries, with the notable exception of Germany and the UK in the case of single parents. Age has, on the whole, only a moderate influence. A higher skill level is associated with a reduction of poverty rates, as could be expected. The work intensity of the household has by far the strongest effect in all countries. Low work intensity lifts the risk by more than 30 percentage points everywhere, and by even more than 60 percentage points in Slovakia. In Germany, the share of unemployed persons living in households with low work intensity is particularly high (see ), whereas it is lowest in Slovakia. This is closely linked to the respective share of persons living alone, because single-person households mostly have a low work intensity.
The results of the decomposition analysis shown in reveal that about half of the cross-country poverty differences are on average due to composition effects. It should be noticed that this result is based on an analysis considering only four, albeit important, characteristics. It is plausible that the proportion explained by differences in the composition increases if further variables could be included.
Furthermore, the results show the variations in the explanation of poverty between countries. The good performance of Belgium (BE) is mainly explained by the contribution of differences in the conditional poverty function. In Germany (DE), the country with the highest poverty risk, the high poverty risk for unemployed is to a significant extent due to an unfavourable population structure. For Spain (ES), the picture is more balanced and Sweden (SE), the country where unemployed face the lowest poverty risk, is characterised by a more favourable distribution of income compared to Germany, Spain, Slovakia and UK. For Slovakia (SK) the results show that differences in poverty rates are mostly explained by an unfavourable income distribution. The UK depicts a middle position, regarding the contribution of the composition and distribution effect.
IV. Conclusion
In this note, we have studied the influence of differences in the composition of the unemployed on differences in the poverty rate of the unemployed between six European countries. We find that most characteristics have the same effect on the poverty risk in all countries, albeit with a different intensity. The results of a decomposition analysis show that about half of the cross-country poverty differences are on average due to observed differences in the composition of the unemployed. Some countries have an advantage in terms of a favourable distribution of poverty-relevant characteristics among the unemployed, but also have a more unfavourable income distribution (Slovakia, partially Spain) or vice versa (Belgium, Sweden, UK). Germany however has both, an unfavourable structure of the unemployed and a high concentration of the unemployed around the poverty line.
Disclosure statement
No potential conflict of interest was reported by the authors.
Notes
1 The responsibility for all conclusions drawn from the data lies entirely with the authors.
2 Denmark, the country with the lowest poverty rate, could not be included because the sample size was too small for our estimations.
3 For a summary of these extensions and modifications, see Seeleib-Kaiser (Citation2008).
4 The indicator for the work intensity in the household, one of our control variables, is defined in the EU-SILC only for individuals below 60 years of age.
5 For the estimation of the distribution parameters, we use the Stata commands smfit written by Jenkins (Citation2004). Estimation results are shown in .
References
- Bandourian, R., J. McDonald, and R. Turley. 2003. “A Comparison of Parametric Models of Income Distribution across Countries and over Time.” Estadistica 55: 135–152.
- Biewen, M., and S. Jenkins. 2005. “A Framework for the Decomposition of Poverty Differencees with an Applicatin to Poverty Differences between Countries.” Empirical Economics 30: 331–358. doi:10.1007/s00181-004-0229-1.
- Esping-Andersen, G. 1990. The Three Worlds of Welfare Capitalism. Princeton: Princeton University Press.
- Eurostat. 2013. “EU-SILC 065 (2013 Operation). Description of Target Variables: Cross-Sectional and Longitudinal. 2013 Operation (Version May 2013).” European Commission. Directorate F: Social Statistics and Information Society. https://circabc.europa.eu/sd/a/cacec83d-9db2-4688-80cb-d2a9342eb93c/SILC065%20operation%202012%20VERSION%20MAY%202013.pdf
- Jenkins, S. P. 2004. Fitting Distributions by Maximum Likelihood. Berlin: Presentation at Second German Stata Users Group Meeting.
- Seeleib-Kaiser, M., ed. 2008. Welfare State Transformations. Comparative Perspectives. New York: Palgrave/Macmillan.
Appendix
Table A1. Estimates of SM-distribution parameters by country.
Table A2. Individual and household characteristics of the unemployed (per cent).
Table A3. Estimates of distribution parameters by country.