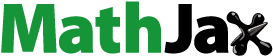
ABSTRACT
Foreign direct investment (FDI) plays an ever-increasing role in the world economy; however, empirical research on many aspects of FDI is hindered by data problems. New data on FDI stocks, broken down according to the nationality of the ultimate owner company, are available for a few countries. Based on the example of three Central and Eastern European countries, we show that these new data give better results when analysing the main drivers of bilateral FDI, than the up till now available FDI data, which were broken down according to the nationality of the immediate investor firm. Furthermore, we present evidence on the main drivers of FDI into three former transition economies: distance, relative country size and trade costs.
I. Introduction
There is a wealth of literature on foreign direct investment (FDI). They focus mainly on the direction and driving forces of FDI flows and their impact on the home and host economies (Li, Liao, and Sun Citation2018). Data problems and the impact of data selection on study results were often underlined as important barriers to this analysis (Iwasaki and Tokunaga Citation2014). Up till now, one approach to overcome this problem was to use detailed firm-level data (El-Sahli, Gullstrand, and Olofsdotter Citation2018). We investigate whether new FDI stock data based on the principle of ultimate beneficiary owner’s nationality as opposed to the immediate or direct owner of FDI perform better in analysing the most important factors affecting bilateral FDI stocks.
We selected three Visegrad countries (the Czech Republic, Hungary and Poland) as a case study for our analysis. FDI had an important role for these countries in their transition process and later on in their convergence process with developed economies. After a long period of isolation during the planned economy, these countries opened up to FDI more or less abruptly as their transition to market economy started. Thus, they became interesting ‘laboratories’ (Meyer and Peng Citation2016) for economic and business analysis, resulting in a set of investigations about the various aspects of FDI. Their similarity due to their common economic history, structure, geographic location and size provides us with a relatively homogeneous group of countries.
Our main aim is to show on a sample of three Central European countries that the new FDI data provide better results when analysing the main drivers of bilateral FDI, than the up till now available FDI data, which were broken down according to the nationality of the immediate investor firm.
II. Methods and data
Our analysis is based on the knowledge-capital model of multinational activity (Markusen Citation2002) which explains the co-existence of horizontal and vertical FDI in a general equilibrium framework and takes into account both home and host countries’ characteristics and the increased international fragmentation of production. Following earlier studies (e.g. Perez, Brada, and Drabek Citation2012), we estimate the following benchmark models.
The independent variables are the following: FACTOR1 represents the factor endowment differences, using the absolute value of the differences between home and host country per capita GDP. ADJ takes the value of 1 if the host and home countries have a common border and 0 otherwise. DIST is the distance between the respective capital cities. SUM controls for relative country size, following Egger and Winner (Citation2006), and GDP2 is that of the home country. TCHOST is the trade cost of the host country, proxied by imports in % of GDP. TCHOME is the trade costs of the home country, proxied by balance of goods and services in % of GDP. FACTOR2 represents the difference in skill endowments, by using the difference between the Human Development Index of home and host countries, following Perez, Brada, and Drabek (Citation2012).
We use a newly available data set on FDI stocks for the year 2016, broken down according to the nationality of the ultimate owner company. In tracing the ‘real origin’ of FDI in a host country, a new opportunity is provided by these data, which should be compiled by the national banks according to the latest balance of payments manual (BPM6) and the new benchmark definition of FDI (BMD4) (IMF Citation2009; OECD Citation2015). The new methodology complies with the reality of FDI, where the ultimate controlling investor of an investment project may differ from the direct investor and thus also their nationalities differ. Multinationals increasingly use their foreign subsidiaries for channelling FDI to third (or fourth, fifth and so on) countries, for various reasons, among which tax optimization through using tax havens (Bojnec and Fertő Citation2018) or tax-friendly developed countries clearly stands out. Further reasons include organizational ones or higher familiarity of subsidiary staff with the new location of FDI in a third country.
Two FDI data sets are available for each country: one based on the nationality of the immediate investor and another one based on the nationality of the ultimate investor. The task of tracing the ultimate investor multinational company is in certain cases problematic and requires substantial knowledge and resources, even if there are detailed guidelines published on identifying them (OECD Citation2015). That may be the reason why up until now only a few national banks published two data sets. From the OECD, FDI position (stock) data were available at the time of our research for 17 countries, including our sample countries.
We use the latest available data (2016) for the three selected economies. (At present, the data are available for maximum four years: 2014–2015–2016 and for a few countries for 2017. As of 6 July 2019, 17 countries present their data at the OECD website: https://stats.oecd.org/Index.aspx?QueryId=64220: FDI positions by partner country BMD4: Inward FDI by immediate and by ultimate investing country.) For the Czech Republic, we use bilateral FDI stock data for 45 countries, for Hungary, 56 countries and for Poland 45 countries, where data are different from zero and contain the most important investing countries. Our sample covers more than 95% of the total FDI stock (both immediate and ultimate) of these countries. Note that the two FDI data sets are rather similar (correlation coefficient of 0.732 and Spearman correlation coefficient of 0.727), but there are some important differences between them. We use OLS regression with heteroscedastic robust SEs.
shows that there is a clear dominance of one investor country according to final investors (in this case Germany). According to direct investors, there is still a dominant investor, but the distribution among investing countries is more even. Furthermore, small final investors compared to direct ones are more numerous in the Czech Republic and Hungary, while the case is the opposite in Poland.
Figure 1. Differences between FDI stock data according to the nationality of the direct and ultimate investor.
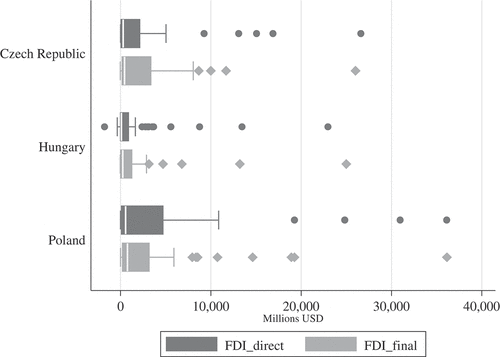
Our results are mixed in terms of reinforcing the basic model (). For KK1 model, five variables are significant with ultimate FDI data, three of them: SK1, DIST and SUM with the expected sign. With immediate FDI data, there are only three significant variables, two of them with the expected sign. For the augmented KK2 model, the ultimate FDI yielded in a higher number of significant variables compared to the immediate FDI one, with the interaction effect of Sk2difgdp and Tchostsk2 significant and positive with ultimate FDI data.
Table 1. Results of OLS regressions.
Our results suggest that ultimate FDI data perform basically better in each case compared to when FDI data according to the nationality of the immediate owners are used. This is understandable, as many multinationals ‘sandwich’ a subsidiary in third (fourth, etc.) countries between the parent and the final destination country of the direct investment, introducing substantial distortion in the country composition of inward FDI stock according to the immediate investor’s nationality in a given economy. This distortion is corrected to a significant extent by the new BPM6/BMD4 FDI data.
Furthermore, distance is an important factor influencing FDI between a Visegrad country and the individual investor countries, performing well with both ultimate and immediate investors. This is in line with the results of previous studies (e.g. Brenton, Di Mauro, and Lücke Citation1999). Relative country size performs well as an explanatory factor of bilateral FDI with ultimate FDI data indicating the importance of ‘similarity’ in FDI flows. Trade costs, especially those of the home country and the host country with ultimate investors, are significant. This suggests that while both horizontal and vertical FDI are present in the region, the latter dominates. Relative factor endowment differences, however, gave ambiguous results: their nonexpected signs may indicate the changing position of the three countries in this respect as a result of their increasing wage levels. Surprisingly, common border is not significant, offering various explanations. First, Germany is the leading investor in Hungary, without a common border between the two countries, second, in the case of ultimate FDI data, US, and in the case of direct data, countries with beneficial tax regulations (Netherlands, Luxemburg, Ireland) but without common border with the analysed countries are the leading investors. Furthermore, intraregional FDI has remained low so far.
III. Conclusion
We show that new FDI data, broken down according to the nationality of the ultimate investor company, give better results when analysing the main drivers of bilateral FDI data, than the up till now available FDI data according to the nationality of immediate investor firms. Furthermore, we show that distance, relative country size and trade costs are the main drivers of FDI into three former transition economies.
Further research can expand the country group analysed to include not only former transition economies but other OECD countries as well. When data for further years will be available, a panel data analysis may yield better results allowing to analyse the dynamics of FDI and the impacts of changes in the institutional environment onFDI. Furthermore, the role of intermediary countries can be analysed: what are the main determining factors (besides tax optimization) in the selection of intermediary third countries.
Disclosure statement
No potential conflict of interest was reported by the authors.
References
- Bojnec, S., and I. Fertő. 2018. “Globalization and Outward Foreign Direct Investment.” Emerging Markets Finance and Trade 54 (1): 88–99. doi:10.1080/1540496X.2016.1234372.
- Brenton, P., F. Di Mauro, and M. Lücke. 1999. “Economic Integration and FDI: An Empirical Analysis of Foreign Investment in the EU and in Central and Eastern Europe.” Empirica 26 (2): 95–121. doi:10.1023/A:1007006429600.
- Egger, P., and H. Winner. 2006. “How Corruption Influences Foreign Direct Investment: A Panel Data Study.” Economic Development and Cultural Change 54 (2): 459–486. doi:10.1086/497010.
- El-Sahli, Z., J. Gullstrand, and K. Olofsdotter. 2018. “Exploring Outward FDI and the Choice of Destination: Evidence from Swedish Firm-level Data.” Applied Economics Letters 25 (17): 1222–1225. doi:10.1080/13504851.2017.1412068.
- IMF. 2009. Balance of Payments and International Investment Position Manual, Sixth ed. (BPM6), Washington DC: International Monetary Fund.
- Iwasaki, I., and M. Tokunaga. 2014. “Macroeconomic Impacts of FDI in Transition Economies: A Meta-Analysis.” World Development 61: 53–69. doi:10.1016/j.worlddev.2014.03.022.
- Li, B., Z. Liao, and L. Sun. 2018. “Evolution of FDI Flows in the Global Network: 2003–2012.” Applied Economics Letters 25 (20): 1440–1446. doi:10.1080/13504851.2018.1430306.
- Markusen, J. R. 2002. Multinational Firms and the Theory of International Trade. Cambridge, MA: MIT Press.
- Meyer, K. E., and M. W. Peng. 2016. “Theoretical Foundations of Emerging Economy Business Research.” Journal of International Business Studies 47 (1): 3–22. doi:10.1057/jibs.2015.34.
- OECD. 2015. Measuring International Investment by Multinational Enterprises. Implementation of the OECD’s Benchmark Definition of Foreign Direct Investment. 4th ed. Paris: OECD.
- Perez, M. F., J. C. Brada, and Z. Drabek. 2012. “Illicit Money Flows as Motives for FDI.” Journal of Comparative Economics 40 (1): 108–126. doi:10.1016/j.jce.2011.03.007.