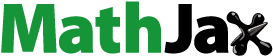
ABSTRACT
In this work we investigate the possible job-creation impact of artificial intelligence (AI) technologies, focusing on the supply side, where the development of these technologies can be conceived as product innovations in upstream sectors. The empirical analysis is based on a worldwide longitudinal sample (obtained by merging the EPO PATSTAT and BvD-ORBIS databases) of more than 3,500 front-runner companies that patented AI-related inventions over the period 2000–2016. Based on system GMM estimates of dynamic panel models, our results show a positive and significant impact of AI patent families on employment, supporting the labour-friendly nature of AI product innovation.
I. Introduction
Artificial Intelligence (AI) typically refers to the capability of a system to perform human-like cognitive functions (learning, understanding, reasoning, and interacting) with the aim of obtaining rational outcomes (Ertel Citation2018; Russell and Norvig Citation2016).
Indeed, the recent application of AI technologies in production has raised fears of a new wave of ‘technological unemployment’ (for a historical analysis of labour-saving innovations, see Staccioli and Virgillito Citation2021; for a survey, see Calvino and Virgillito Citation2018; for a theoretical framework, see Acemoglu and Restrepo Citation2018). In this vein, according to Brynjolfsson and McAfee (Citation2014), at the root of current employment problems lies a ‘Great Restructuring’ which is having an ever-bigger impact on jobs and skills (see also Frey and Osborne Citation2017; Arntz, Gregory, and Zierahn Citation2017; Graetz and Michaels Citation2018; Acemoglu and Restrepo Citation2020).
However, if we adopt a Schumpeterian perspective (see Schumpeter Citation1912), technological change cannot be reduced to labour-saving process innovation. Indeed, the extant literature only focuses on the possibly negative employment impact of AI and (particularly) robots conceived as process innovations, while there is no evidence so far about the possible positive employment effect of these new technologies, considered as product innovations in the supply sectors. This is unfortunate since product innovations are theoretically considered drivers of job creation (see Katsoulacos Citation1984; Vivarelli Citation1995; Edquist, Hommen, and McKelvey Citation2001), and several empirical studies have provided evidence in support of their labour-friendly nature (see Van Reenen Citation1997; Bogliacino and Pianta Citation2010; Bogliacino, Piva, and Vivarelli Citation2012; Van Roy, Vertesy, and Vivarelli Citation2018). In this framework, AI products not only entail the emergence of entire new sectors or a substantial expansion of existing onesFootnote1 but also the creation of brand-new employment opportunities such as those related to data processing, transactional procedures, customization, remote collaboration, and so on.
The aim of this study is indeed to assess the possible job-creation impact of AI technologies, focusing on the providers of the new knowledge base.
II. Methodology, data, and variables
Methodology
Similarly to prior relevant microeconometric literature (see Van Reenen Citation1997; Lachenmaier and Rottmann Citation2011; Bogliacino, Piva, and Vivarelli Citation2012; Van Roy, Vertesy, and Vivarelli Citation2018), we derive our empirical specification from a stochastic version of a dynamic standard labour demand, augmented with an innovation proxy:
Lower case letters denote natural logarithms, l corresponds to labour, y to output, w to wages, and I to gross investments; we use AI and non-AI patent families (Pat_(i,t)^(AI) and Pat_(i,t)^(Non-AI)) as a measure of innovation. Lastly, μ is an unobserved firm-specific and time-invariant effect and the usual error term. It is important to include non-AI technologies in model specifications in order to isolate the specific employment impact of AI technologies. Moreover, their exclusion may generate an omitted variable issue potentially leading to a spurious upward bias of the effects associated with AI variables arising from the positive correlation between AI and (omitted) non-AI variables (equal to 0.35 for patent families and 0.18 for patent family size in our sample).
Dynamic labour demand specifications as in Equation 1 suffer from simultaneity and endogeneity problems, which may lead to biased estimations. To tackle both problems, we use a system GMM approach as developed by Blundell and Bond (Citation1998; Citation2000). Since possible problems of endogeneity are not confined to the lagged dependent variable, all the explanatory variables are considered to be potentially endogenous and instrumented when needed. As a high instrument count may imply a downward bias in the two-step system GMM standard errors (see Roodman Citation2009b, pp. 140–141), we use the one-step system GMM estimator. The lag limits of the instruments were chosen both to satisfy the outcomes of the autocorrelation tests and to limit instrument proliferation, as highlighted in Roodman (Citation2009a, Citation2009b).
Data
To identify AI patents, we rely on Damioli et al. (Citation2021), who follow a comprehensive, keyword-based approach consisting of the search for specific terms in the title or the abstract of patents.Footnote2
Text-mining searches following the methodology of Van Roy, Vertesy, and Damioli (Citation2020) have been conducted to retrieve the keywords in either the patent title or the abstract of the Spring 2018 edition of the Worldwide Patent Statistical Database (PATSTAT) released by the European Patent Office (EPO). Containing bibliographical and legal event patent data from around 100 million patent filings covering more than 90 issuing authorities, including all the major offices, the EPO’s PATSTAT has become a point of reference in the field of patent statistics. We grouped retrieved patents in patent families based on PATSTAT extended family identifiers. A patent family is a collection of patent applications covering the same or similar technical content. The use of patent families therefore ensures avoiding double counting of the same or similar inventions filed in different patent offices across countries. We then obtained key company characteristics of AI patent applicants through the BvD-ORBIS databases.
After excluding observations with missing values and outliers in both levels and growth rates, our final dataset covers 3,510 firms (resulting in 26,137 observations) active in AI patenting over the years 2000–2016. It provides worldwide coverage and includes firms belonging to manufacturing and service sectors.
Variables
Our dependent variable is the number of employees in head counts. Explanatory variables include firm turnover, labour cost per employee, and gross investments measured as the annual change in fixed assets. We expect a positive impact on labour demand of turnover and gross investments, and a negative impact of labour cost. The models also control for industry-, year-, and country-specific differences in employment dynamics.
The key explanatory variable of interest is the number of AI patent families filed by a firm in a year, which measures the development and adoption of AI technology. In order to isolate the distinctive impact of AI technologies, we also take into account innovative efforts in non-AI related fields through a number of non-AI patent families.
Since patents can differ in both economic and technological values, other indicators have been proposed to correct for the quality of patents, such as forward citation-weighted patents and family size (Harhoff, Scherer, and Vopel Citation2003; Hall, Jaffe, and Trajtenberg Citation2005; Van Roy, Vertesy, and Vivarelli Citation2018). Weighting AI patents with forward citations is particularly controversial in the present setting since the take-off of AI technologies has occurred recently (Cockburn, Henderson, and Stern Citation2019). We therefore use patent family size, which is the number of countries in which an invention is protected by a patent family. Given the huge costs of acquiring patents in multiple jurisdictions, patent family size is often used to approximate the value that applicants attribute to the invention (see Harhoff, Scherer, and Vopel Citation2003; Lanjouw and Schankerman Citation2004). A firm-level measure of patent family size was computed by taking the average size of all applications filed by a company in a given year, separately for AI and non-AI patents, to provide a quality-augmented measure of innovation efforts of a firm.
in the Appendix reports the summary statistics of the dependent and explanatory variables used in the estimations. Firms in the sample report an average of over 4,200 employees due to the presence of big corporations (by contrast the median equals 99 employees). The development of AI technologies is much lower than the development of their non-AI counterparts: on average, each firm files approximately 1 AI patent family and 100 non-AI patent families every 3 years.
III. Results
reports the estimation coefficients in seven model specifications, in which AI and non-AI variables are included separately and together. The outcomes of the tests on autocorrelation dynamics are reassuring. With regard to the Hansen test on adequate instruments, the null hypothesis is rejected. Blundell and Bond (Citation2000) and Roodman (Citation2009a, Citation2009b) demonstrated that the Hansen test tends to over-reject the null in large samples. Therefore, the model was re-estimated on random sub-samples comprising 10% of the baseline observations and the null was never rejected.Footnote3
Table 1. Results from GMM-SYS analysis. Notes: one-step GMM robust standard errors are reported in parentheses. All variables are taken in log. *** p < 0.01, ** p < 0.05, * p < 0.1. For the level equation, we used differenced values of the explanatory variables as instruments, i.e. thrice-lagged differences in labour demand, AI and non-AI patent families, gross investments, and cost of labour. The level equations also include a set of sector, country, and year dummies. In the equations in differences, we employed thrice-lagged values of the above-mentioned right-hand side variables as instruments. The lag limits of the instruments were chosen both to satisfy the outcomes of the autocorrelation tests and to limit instrument proliferation, as highlighted in Roodman (Citation2009a, Citation2009b).
The magnitude and significance of the coefficients remain reassuringly unchanged in all specifications. Lagged employment is highly significant: unsurprisingly, labour demand is persistent and autoregressive, confirming its path dependency. With regard to the control variables, results are in line with prior studies (Van Reenen Citation1997; Bogliacino, Piva, and Vivarelli Citation2012; Van Roy, Vertesy, and Vivarelli Citation2018): the positive effect of turnover is substantial and highly significant, while the effect of gross investments is more contained, but still significant at the 5% level; finally, as expected, labour cost significantly inhibits labour demand.
Regarding our key innovation variables, positive and highly significant coefficients of AI and non-AI patent families are detected: they both imply a similarly moderate employment short-term elasticity of about 3–4%. The positive effect of AI technologies in models (6) and (7), which control for non-AI patents, indicates that the labour friendly nature of AI technologies is additional to other (non-AI) firm innovation activities. As such, this finding confirms the employment-friendly nature of product innovation in general, and provides novel evidence for the emerging AI technologies. In particular, when patent family size is used, only AI innovations imply a significant positive effect on employment.
IV. Conclusions
In contrast with the extant literature solely devoted to assessing the possible labour-saving impact of automation in the user sectors, this article investigates the possible labour-friendly nature of AI technologies, seen as product innovations in the supply industries.
Our estimates support this hypothesis. Moreover, the positive employment impact of AI is larger and more significant when compared to the job creation effect of other innovation activities. However, the labour-friendly employment effect of patenting in AI technologies is small in magnitude (the estimated elasticity being equal to 3–4%) and is unlikely to be able to compensate for the possible labour-saving impact in the downstream user industries (see the studies cited in Section 1).
Obviously, our findings cannot be generalized at a more aggregate and macroeconomic level; further research is needed to assess the employment impact of the new knowledge base at the level of the entire economy, taking into account innovators, non-innovators, and user firms. Finally, a further avenue of future research should focus on the possible role of SMEs in labour-friendly AI product innovation, a focus that is beyond the scope of the present contribution.
Disclaimer statement
The scientific output expressed here does not imply a policy position of the European Commission or the International Telecommunication Union and their Member States. Neither the European Commission nor any person acting on behalf of the Commission is responsible for the use that might be made of this study.
Acknowledgments
We are very grateful to two anonymous referees for extensive comments that helped to improve the article considerably. We also thank the participants of the Workshop ‘The Economics and Management of AI Technologies’ (Bureau d’Economie Théorique et Appliquée, Université de Strasbourg) and of the GLO Virtual Seminar. Giacomo Damioli acknowledges the financial support from the European Union’s Horizon 2020 Framework Programme under the ‘Innova Measure IV’ project (Grant agreement no. 857088). Marco Vivarelli acknowledges support by the Italian Ministero dell’Istruzione, dell’Università e della Ricerca (PRIN-2017, project 201799ZJSN: ’Technological change, industry evolution and employment dynamics’; principal investigator: Marco Vivarelli).
Disclosure statement
No potential conflict of interest was reported by the author(s).
Correction Statement
This article has been republished with minor changes. These changes do not impact the academic content of the article.
Additional information
Funding
Notes
1 Think, for instance, of the electronic industry where robots are produced, or scientific services where AI algorithms are conceived.
2 An analogous approach to selecting AI patents was adopted by Cockburn, Henderson, and Stern (Citation2019).
3 Results are available upon request.
References
- Acemoglu, D., and P. Restrepo. 2018. “The Race between Man and Machine: Implications of Technology for Growth, Factor Shares, and Employment.” American Economic Review 108 (6): 1488–1542. doi:10.1257/aer.20160696.
- Acemoglu, D., and P. Restrepo. 2020. “Robots and Jobs: Evidence from US Labor Markets.” Journal of Political Economy 128 (6): 2188–2244. doi:10.1086/705716.
- Arntz, M., T. Gregory, and U. Zierahn. 2017. “Revisiting the Risk of Automation.” Economics Letters 159: 157–160. doi:10.1016/j.econlet.2017.07.001.
- Blundell, R., and S. Bond. 1998. “Initial Conditions and Moment Restrictions in Dynamic Panel Data Models.” Journal of Econometrics 87: 115–143. doi:10.1016/S0304-4076(98)00009-8.
- Blundell, R., and S. Bond. 2000. “GMM Estimation with Persistent Panel Data: An Application to Production Functions.” Econometrics Reviews 19: 321–340. doi:10.1080/07474930008800475.
- Bogliacino, F., and M. Pianta. 2010. “Innovation and Employment: A Reinvestigation Using Revised Pavitt Classes.” Research Policy 39 (6): 799–809. doi:10.1016/j.respol.2010.02.017.
- Bogliacino, F., M. Piva, and M. Vivarelli. 2012. “R&D and Employment: An Application of the LSDVC Estimator Using European Data.” Economics Letters 116 (1): 56–59. doi:10.1016/j.econlet.2012.01.010.
- Brynjolfsson, E., and A. McAfee. 2014. The Second Machine Age: Work, Progress, and Prosperity in a Time of Brilliant Technologies. New York: W.W. Norton.
- Calvino, F., and M. E. Virgillito. 2018. “The Innovation Employment Nexus: A Critical Survey of Theory and Empirics.” Journal of Economic Surveys 32: 83–117. doi:10.1111/joes.12190.
- Cockburn, I., R. Henderson, and S. Stern. 2019. “The Impact of Artificial Intelligence on Innovation.” In The Economics of Artificial Intelligence: An Agenda, edited by A. Agrawal, J. Gans, and A. Goldfarb. University of Chicago Press and NBER.
- Damioli, G., V. Van Roy, and D. Vertesy. 2021. “The Impact of Artificial Intelligence on Labor Productivity.” Eurasian Business Review 11 (1): 1–25. doi:10.1007/s40821-020-00172-8.
- Edquist, C., L. Hommen, and M. McKelvey. 2001. Innovation and Employment: Product versus Process Innovation. Cheltenham: Elgar.
- Ertel, W. 2018. Introduction to Artificial Intelligence: Undergraduate Topics in Computer Science. New York: Springer International Publishing.
- Frey, C., and M. Osborne. 2017. “The Future of Employment: How Susceptible are Jobs to Computerisation?” Technological Forecasting and Social Change 114: 254–280. doi:10.1016/j.techfore.2016.08.019.
- Graetz, G., and G. Michaels. 2018. “Robots at Work.” The Review of Economics and Statistics 100 (5): 753–768. doi:10.1162/rest_a_00754.
- Hall, B., A. Jaffe, and M. Trajtenberg. 2005. “Market Value and Patent Citations.” RAND Journal of Economics 16–38.
- Harhoff, D., F. M. Scherer, and K. Vopel. 2003. “Citations, Family Size, Opposition and the Value of Patent Rights.” Research Policy 32 (8): 1343–1363. doi:10.1016/S0048-7333(02)00124-5.
- Katsoulacos, Y. S. 1984. “Product Innovation and Employment.” European Economic Review 26: 83–108. doi:10.1016/0014-2921(84)90023-0.
- Lachenmaier, S., and H. Rottmann. 2011. “Effects of Innovation on Employment: A Dynamic Panel Analysis.” International Journal of Industrial Organization 29 (2): 210–220. doi:10.1016/j.ijindorg.2010.05.004.
- Lanjouw, J., and M. Schankerman. 2004. “Patent Quality and Research Productivity: Measuring Innovation with Multiple Indicators.” Economic Journal 114 (495): 441–465. doi:10.1111/j.1468-0297.2004.00216.x.
- Roodman, D. 2009a. “How to Do Xtabond2: An Introduction to “Difference” and “System” GMM in Stata.” Stata Journal 9: 86–136. doi:10.1177/1536867X0900900106.
- Roodman, D. 2009b. “A Note on the Theme of Too Many Instruments.” Oxford Bulletin of Economics and Statistics 71: 135–158. doi:10.1111/j.1468-0084.2008.00542.x.
- Russell, S., and P. Norvig. 2016. Artificial Intelligence: A Modern Approach. Malaysia: Pearson Education Limited.
- Schumpeter, J. A. 1912. The Theory of Economic Development. (Mass.) Engl. Ed. 1968, Cambridge: Harvard University Press.
- Staccioli, J., and M. E. Virgillito. 2021. “Back to the Past: The Historical Roots of Labor-saving Automation.” Eurasian Business Review 11 (1): 27–57. doi:10.1007/s40821-020-00179-1.
- Van Reenen, J. 1997. “Employment and Technological Innovation: Evidence from U.K. Manufacturing Firms.” Journal of Labor Economics 15 (2): 255–284. doi:10.1086/209833.
- Van Roy, V., D. Vertesy, and G. Damioli. 2020. “AI and Robotics Innovation.” In Handbook of Labor, Human Resources and Population Economics, edited by K. Zimmermann, 1–35.
- Van Roy, V., D. Vertesy, and M. Vivarelli. 2018. “Technology and Employment: Mass Unemployment or Job Creation? Empirical Evidence from European Patenting Firms.” Research Policy 47 (9): 1762–1776. doi:10.1016/j.respol.2018.06.008.
- Vivarelli, M. 1995. The Economics of Technology and Employment: Theory and Empirical Evidence. Elgar, Cheltenham. (reprinted 1997).
Appendix
Table A1. Definition and summary statistics of the variables used in analysis. Notes: the sample includes 26,137 observations and 3,510 firms. The variable definition describes how variables are used in the empirical models. All variables are defined at firm-year level. The summary statistics are expressed as follows: employment is the number of employees; turnover, cost of labour per employee, and fixed assets are expressed in EURs; gross investments are shown as yearly percentage changes.