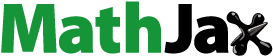
ABSTRACT
This study proposes a novel approach by combining the first differenced generalized method of moments method (DIF-GMM) to remove the estimates bias in the dynamic panel data model and the least trimmed squares (LTS) to control outlier influence. The combination of these two methods is referred to as DIF-GMM+LTS. We apply this approach to examine the influence of outliers on the effect of financial development on economic growth. Our results show a counter-intuitive evidence that the bank development negatively affects economic growth when the outlier influence is ignored. However, the bank development exhibits a positive influence on economic growth once the proposed approach DIF-GMM+LTS is adopted. Also, stock market development shows a positive effect on economic growth regardless of the outliers.
I. Introduction
Despite the complex data structure of dynamic panels, a vast majority of literature focuses on the empirical results without considering outlier influence (Kiviet Citation2020), which may produce the biased or non-robustness results.
To get precise and robust results, many researchers simply remove a certain extreme percentage of observations before or after the estimation, and use the winsorization method. These extreme values usually be treated as x- or y-outliers but not as regression outliers (Rousseeuw and Leroy Citation1987). Removing x- and y-outliers may not eliminate the regression outliers, thereby cause a biased estimation Shen, Luo, and Huang (Citation2015) found that winsorization may excessively or inadequately adjust for the outlier effect and suggested the use of MM (Yohai Citation1987). Raymaekers and Rousseeuw (Citation2021) proposed the robust estimator could deal with the outliers precisely by lots of methods using data transformations. However, some outlying extreme observations could not be effectively identified.
Other extreme observations treated as x- or y-outliers but no regression outliers could not be deleted, otherwise it will enhance outlier influence on robust statistics. The crux of the robust theory is the approximate character of the strict parametric model. Comparing with many studies following the probabilistic reduction approach in search for the distribution , in robust statistics the distribution of the complete data is represented by
.Footnote1 Conventionally,
reflects the central model distribution whereas outliers are generated by the distribution
. To provide a global measure of the sensitivity of an estimator to outlying observations, the ideas of breakdown point and influence function are introduced to the robustness of an estimator for the argument that outliers corrupt classical estimation.Footnote2 Considering the susceptibility of classical estimators to outliers in dynamic panel data model, new robust estimators developed in robust statistics are to trace outliers and diminish their influence on statistical inference.
Unfortunately, the widely used DIF-GMM has been found to have poor precision in simulation studies. Lagged levels of the series provide weak instruments for first differences in this case Dhaene and Zhu (Citation2017) proposed globally robust estimators that were based on the median ratios of the first- or higher-order differences of the dependent variable. The main shortcomings of these methods follow the fixed number of the differences and ratios.
This study incorporates the least trimmed squares (LTS) to control outlier influence in the DIF-GMM framework, which is referred to as DIF-GMM+LTS. According to Shen et al. (Citation2018), we select LTS to consider outlier influence. DIF-GMM optimally exploits all the linear moment restrictions that follow from the assumption of no serial correlation in the errors, and no strictly exogenous variables. These features make LTS be easy and feasible to combine with DIF-GMM.
The paper is organized as follows. Section II discusses the dynamic panel considering the outliers. Section III contains the application to the effect of financial development on economic growth and Section IV concludes.
II. Estimation
Considering outliers: DIF-GMM estimator
We consider the dynamic panel data model with independent variables:
where is a
vector,
are not serially correlated. The DIF-GMM estimator has exploited optimally all the linear moment restrictions with some specifications based on unbalanced panel data.
Weak instruments are common in economic studies using instrumental variables to make causal inferences from observational studies. In the econometric literature, it is well established that bad outliers (×- and y-outliers) can generate the weak instruments (Desbordes and Verardi Citation2012) and good leverage points (mistakenly defined as x- or y-outliers) can provide the strong instruments (Ontiveros and Verardi Citation2012). Hence, we define outliers as the regression outliers to get more good leverage points.Footnote3
Removing outliers: LTS method based on panel data
After removing outliers,Footnote4 precise results can be obtained. Consider the dynamic panel data model (1), we define
where is the number of observations that are not trimmed from the data set,
denotes the residual of the t-th observation from the NT-sample, and
,
Thus, the LTS minimizes the sum of the squared residuals with the smallest h, and the maximum possible value for the breakdown point,
, [.] denotes the integer, in which the LTS estimator reaches the maximal possible value for the breakdown point. This breakdown point minimizes the trimmed sum of the squared residuals.
Dynamic panel data model with the consideration of outliers: DIF-GMM+LTS
We select DIF-GMM to incorporate LTS for two reasons. First Čížek and Aquaro (Citation2018), found plenty of moment conditions might increase estimation bias due to outliers, so we chose DIF-GMM estimator just because it does not make use of all available moment conditions. Second, the DIF-GMM can incorporate LTS more easily than others approaches. Our DIF-GMM+LTS method includes six steps.
Step 1. Conduct based on the full sample set
without considering outlier influence, then generate the resulting residuals series
.
Step 2. Calculate based on the stochastic subset
from
, subscript (1) denotes the first iteration of the estimator.
Step 3. Sort in an ascending order, yielding permutation g for which
where denotes the residual of the lth observation of the full set
. The former
observations
are assembled in the new subset
.
Step 4. Similarly compute , where subscript (2) denotes the second iteration of the estimator based on subset
. The first round of estimates for DIF-GMM+LTS is obtained by repeating these iteration processes,Footnote5
where subscript (FINAL) denotes the final iteration of the estimator, and .
Step 5. Define the standardized residuals that exceed 2.5 as the outliers and remove them in original sample in view of residuals (see Step 4).
Step 6. Obtain DIF-GMM+LTS final estimators based on the remaining sample and DIF-GMM estimator.
III. An application
In this section, we apply the strategy for estimation and testing outlined earlier to a model of bank development and stock market development on economic growth, using panel data for a sample of 48 countries (See Appendix) for the period 1988–2020. According to Shen et al. (Citation2018), now we consider the same model
where the dependent variable GROWTH is proxied by the logarithm of real per capita GDP, is an unobserved country-specific effect, whereas
is the error term,Footnote6 The bank development is proxied by the claims on the private sector by banks divided by GDP (LENDING), and the stock market development is proxied by the ratio of market capitalization divided by GDP (MKTCAP).
denotes the vector of controlled variables and includes two variables, which are government consumption expenditure divided by GDP (GCONSUMP) and investment divided by GDP (INVESTMENT).
The results of the left of , are quite similar in all cases. The bank development has negative significantly effect on economic growth whereas the stock market development has positive significantly effect on economic growth. This result contradicts the well-known expectation that bank development should increase growth in the current year. The negative effect about bank development is also found in Shen et al. (Citation2018). This present study differs from others by arguing that the outliers may create the negative effect.
Table 1. Effect of financial development on economic growth: DIF-GMM.
The right of presents the estimated results using DIF-GMM+LTS (i.e. considering the outliers). The assumption of exogenous or endogenous variables in column (1)-(4) from the left of are the same as the right. Hence, once outlier influence is considered, both the bank development and the stock market development have positive influence on economic growth. The positive results are consistent with those of Shen et al. (Citation2018) and Noor Zahirah and Mehmet (Citation2020). The dramatic change in sign of coefficient of proxy for the bank development suggests that the existence of outliers may account for the statistically negative coefficients in the literature. Specially, the similar results of GCONSUMP without and with considering outliers can also be found in Noor Zahirah and Mehmet (Citation2020) with considering bad and good institutions respectively. Comparing with LSDVC+LTS estimator mentioned in Shen et al. (Citation2018) to consider outlier influence, we provide DIF-GMM+LTS estimator, which has taken additional account of endogeneity, find the same result that financial development has positive significantly effect on economic growth.
IV. Conclusion
In this paper, we have discussed the estimation of dynamic panel data models by the generalized method of moments. We focus on models with predetermined but not strictly exogenous explanatory variables in which identification results from lack of serial correlation in the errors.
This study presents that outlier influence may cause the mixed results about the effect of financial development on economic growth, particularly when financial development is measured as the bank development by the dynamic panel data model. We show how to incorporate DIF-GMM and LTS to consider outlier influence in panel data. Our results show that the bank development negatively affects economic growth when the outlier influence is ignored. However, once the DIF-GMM+LTS is adopted to control the outliers, the negative influence becomes positive. Moreover, stock market development shows a positive effect on economic growth regardless of the outliers. Future studies can consider different outlier elimination methods while considering the dynamic panel data model.
Disclosure statement
No potential conflict of interest was reported by the authors.
Correction Statement
This article has been republished with minor changes. These changes do not impact the academic content of the article.
Additional information
Funding
Notes
1 We suppose that a fraction of the data obeys
but the rest
of the data are generated by an unknown distribution
.
2 Classical estimators such as OLS, GLS, 2SLS, and GMM have a breakdown point of zero, whereas LTS estimator has a higher breakdown point of 50% when the best robustness properties are achieved. The other concept influence function which is equal to the derivative with respect to
of the probability limit of the estimator when
puts mass one at point
. Specially, the usual GMM has bounded influence function by replacing the usual GMM defining sample moment condition by a robust empirical moment restriction.
3 Fajeau (Citation2021) demonstrated that the threshold conclusion required a peculiar methodological setup relying on extensive use of either irrelevant or weak instruments, which might result in spurious threshold regressions overfitting a few outliers.
4 Rousseeuw and Leroy (Citation1987) proposed LTS method that recognized observations whose standard deviation exceeded 2.5 as the outliers.
5 In practice, only finite amounts of h-subsets are present; thus, index m must exist, such that . Hence, convergence is always reached after some finite steps.
6 We assume that the error term is not serially correlated, and that the explanatory variables
and
are weakly exogenous.
References
- Arellano, M., and S. Bond. 1991. “Some Tests of Specification for Panel Data: Monte Carlo Evidence and an Application to Employment Equations.” The Review of Economic Studies 58 (2): 277–297.
- Čížek, P., and M. Aquaro. 2018. “Robust Estimation and Moment Selection in Dynamic Fixed-Effects Panel Data Models.” Statistics and Computing 33 (2): 675–708. doi:10.1007/s00180-017-0782-7.
- Desbordes, R., and V. Verardi. 2012. “A Robust Instrumental-Variables Estimator.” The Stata Journal 12 (2): 169–181.
- Dhaene, G., and Y. Zhu. 2017. “Median-Based Estimation of Dynamic Panel Models with Fixed Effects.” Computational Statistics & Data Analysis 113: 398–423.
- Fajeau, M. 2021. “Too Much Finance or Too Many Weak Instruments?” International Economics 165: 14–36.
- Kiviet, J. F. 2020. “Microeconometric Dynamic Panel Data Methods: Model Specification and Selection Issues.” Econometrics and Statistics 13: 16–45.
- Noor Zahirah, M. S., and A. Mehmet. 2020. “Do Government Expenditures and Institutions Drive Growth? Evidence from Developed and Developing Economies.” Studies in Economics and Finance 38 (2): 400–440.
- Ontiveros, D. U., and V. Verardi. 2012. “Supposedly Strong Instruments and Good Leverage Points.” No 1203, Working Papers from University of Namur, Department of Economics.
- Raymaekers, J., and P. J. Rousseeuw. 2021. “Transforming Variables to Central Normality.” Machine Learning 5: 1–24.
- Rousseeuw, P., and A. Leroy. 1987. Robust Regression and Outlier Detection. New York: Wiley.
- Shen, C., X. Fan, D. Huang, H. Zhu, and M. Wu. 2018. “Financial Development and Economic Growth: Do Outliers Matter?” Emerging Markets Finance and Trade 54 (13): 2925–2947.
- Shen, C., F. Luo, and D. Huang. 2015. “Analysis of Earnings Management Influence on the Investment Efficiency of Listed Chinese Companies.” Journal of Empirical Finance 12 (34): 60–78.
- Yohai, V. J. 1987. “High Breakdown-Point and High Efficiency Robust Estimates for Regression.” Annals of Statistics 15 (20): 642–656.
Appendix
Our sample contains 48 countries in 18 different regions, including 9 Latin American countries (Argentina, Brazil, Chile, Colombia, Ecuador, Mexico, Peru, Uruguay, Venezuela), 1 Australian country (Australia), 3 Central European countries (Austria, Germany, Switzerland), 3 West European countries (Belgium, France, Ireland), 1 North American country (Canada), 4 North European countries (Finland, Norway, Sweden, Denmark), 1 North African country (Egypt), 2 South European countries (Greece, Italy), 2 South Asian countries (India, Pakistan), 8 East Asian countries (Indonesia, Philippines, Singapore, Hong Kong SAR (China), Thailand, Japan, Korea, Malaysia), 2 West Asian countries (Israel, Turkey), 1 North Arab country (Jordan), 4 sub-Saharan African countries (Kenya, Nigeria, South Africa, Zimbabwe), 2 Northwest European countries (Netherlands, UK), 1 Oceanian country (New Zealand), 2 Southwest European countries (Portugal, Spain), 1 Central North-American country (U.S.A.), 1 South-central Asian country (Sri Lanka).