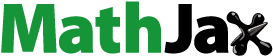
ABSTRACT
During the COVID-19 pandemic there were supply chain bottlenecks all over the world with regard to raw materials and intermediate products. In this article, we examine how these constraints affected labour market development. For an empirical panel analysis, we combine survey data and administrative labour market data for economic sectors in Germany. We find effects on unemployment that are noticeable but still relatively limited. The effect on short-time work, on the other hand, is revealed to be considerable. Whilst short-time work is traditionally imposed where there are slumps in demand, our results show that it is also used in the case of adverse supply shocks. While inflation is rising, this explains why the Phillips curve does not shift outward.
I. Introduction
The COVID-19 pandemic led to supply chain bottlenecks all over the world with regard to raw materials and intermediate products (e.g. Krolikowski and Naggert Citation2021). The Russia-Ukraine war is exacerbating this problem even further. In the literature, the topic of material shortages is not treated exhaustively, perhaps because considerable problems with the supply chain were a rare phenomenon themselves until recently (Wohlrabe Citation2021). However, the potential damage that such shortages can cause to the labour market and their relevance for the functioning of the economy are significant. To the best of our knowledge, this article is the first to demonstrate labour market effects of supply chain bottlenecks in the COVID-19 crisis.
We particularly examine how material shortages affect both short-time work and entries into and exits from unemployment. For an empirical panel analysis, we combine survey data and administrative labour market data for economic sectors in Germany. This allows for identification of bottleneck effects in a dynamic panel setting controlling for heterogeneity.
II. Data
We use data from the Ifo Institute’s surveys of the economic situationFootnote1 to determine the extent of the material bottlenecks for 24 economic sectors. Here, a quarterly survey is carried out to determine whether the production in the companies in the manufacturing industry is currently being hampered due to the lack of raw materials or semi-finished products (Wohlrabe Citation2021).
illustrates the average percentages of businesses that are affected by material bottlenecks for the period from April 2021 to January 2022, divided into economic sectors. Accordingly, it was the sectors involving mineral oil refining and electrical equipment manufacturing and, as a result of this, the areas concerning automotive and automotive parts manufacturing and other vehicle manufacturing which were particularly affected by the material shortage in that period.
Table 1. Share of businesses with material bottlenecks by sector in percent (average share for April 2021 to January 2022).
shows how the material bottlenecks have developed over time. It becomes clear that a bottleneck situation of this nature is without equal in the past 30 years. The share of businesses in the manufacturing industry whose production has been hampered by material bottlenecks reached an all-time high in October 2021 and has persistently remained at around the 70% mark since then. It is only in the main construction industry that the situation can be observed to have eased to some extent since it reached its peak in June 2021.
Figure 1. Share of businesses with material bottlenecks, January 1991 to January 2022 (in %).
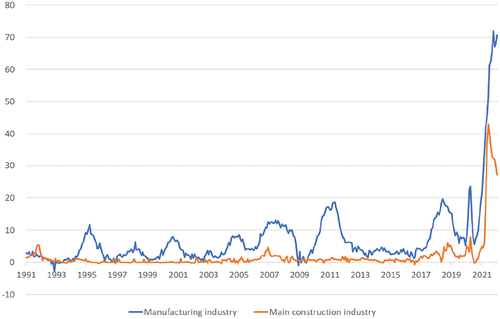
We obtain various variables from the German Federal Employment Agency statistics that have the same distinction according to economic sector and are seasonally adjusted in long time series. We use data on entries into unemployment from the primary labour market, exits from unemployment into the primary labour market and short-time work notifications.Footnote2 shows the course across all sectors used here over time. On aggregate, all indicators strongly worsen with the beginning of the pandemic and recover afterwards.
III. Method
We use the panel dimension across different sectors to estimate the effects of material bottlenecks on the labour market. The entries into unemployment, exits from unemployment and the short-time work notifications serve as independent variables, all of them logarithmised. We use time fixed effects to control macro influences like the economic situation or the course of the pandemic. Sectoral level effects cancel out due to the application of orthogonal deviations (Arellano and Bover Citation1995). A dynamic panel model with a lagged endogenous variable is estimated by GMM. Two further lags of are used as instruments to avoid the Nickell bias. The share of firms subject to material bottlenecks which was calculated from the Ifo surveys is included as an explanatory variable, lagged if the explanatory power is improved. In addition, we control for the sector-specific production (obtained from destatis), thereby taking into account the fact that both the estimation of shortages and the labour market results may depend on the business activity.
The panel model is shown in EquationEquation (1)(1)
(1) :
where to
represent the coefficients,
is the relevant labour market variable,
the shortage indicator,
the industrial production,
the time fixed effects and
the error terms. The sector index is denoted by
, the time index by
and a potential lag by
.
The estimation period is April 2021 until January 2022. We chose the start because in April the second corona lockdown in Germany ended. Therefore, no drastic containment restrictions were in place within our sample. In addition, although the regulations for short-time work were made more generousFootnote3 when the pandemic began in March 2020, they did not change during our sample period. This ensures that these institutional changes do not interfere with our estimation of material bottleneck effects that apply given the institutional framework. Furthermore, in view of our research question, we focus on the most recent phase of extreme material bottlenecks. This phase bears no comparison to the previous course over time (see ), so that including earlier observations would not be suitable for our purposes. In our sample, there are a total of 240 observations available across the time and sector dimensions.
We focus on manufacturing due to data availability and because supply bottlenecks are most relevant in this sector. Moreover, our focus has a further advantage regarding identification: Contact-intensive service sectors have been affected by the corona containment measures and witnessed a recovery afterwards. In contrast, manufacturing firms were not subject to closure or other severe restrictions of their activity. Thus, within our sample, containment measures did not lead to diverging trends that may bias the results.
IV. Results
shows the estimated effects of the material shortage indicator on the three labour market dimensions.
Table 2. Effects of the material shortage indicator on labour market dimensions (in %).
There are statistically significant effects shown on all of the labour market variables observed. One point more in the shortage indicator increases the entries into unemployment by 0.88% and reduces the exits from unemployment by 0.73%. The effect on short-time work notifications is greater, at +3.01%. Regarding exits from unemployment and short-time work notifications, the significant effects are seen in the same month, while for entries into unemployment there is a lag of one month. Short-time work can be imposed very quickly, whilst it generally takes longer to end employment relationships in the German institutional context. Various robustness checks are described in Appendix A2.
The overall effects over the estimation period can be identified in a counterfactual scenario. To do this, we calculate a hypothetical development in which the material bottlenecks would not have worsened since April 2021. The differences in the bottleneck indicator can be applied to the estimated percentage labour market effects from (per point in the indicator). As a result, for all of the industries observed, if the bottlenecks had not worsened until January 2022, the percentage of entries into unemployment would have been 21% (or 47,000) lower, the exits from unemployment 17% (or 29,000) higher and the short-time work notifications 71% (or 446,000) lower.
Adverse supply shocks like those caused by material shortages are typically accompanied by higher inflation and unemployment and thus an outward shift of the Phillips curve. While inflation is indeed rising, it seems to move along the curve in . While Del Negro et al. (Citation2020) found that changes in the Phillips curve before the COVID-19 pandemic were due to a muted reaction of inflation to cost pressures, inflation is now rising strongly (compare Attinasi et al. Citation2021).
Figure 3. The Phillips curve in Germany since January 2008.
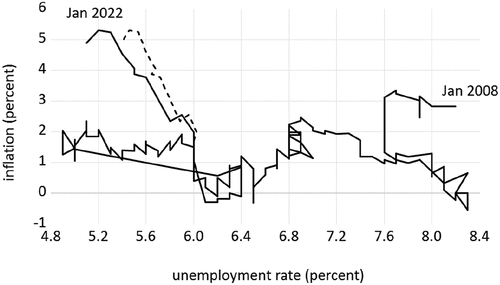
However, increases in unemployment do not seem to happen in the face of adverse supply shocks. Due to the use of short-time work, unemployment does not mirror the whole slack in the labour market. Therefore, we created an alternative unemployment measure by adding the short-time work effect from our counterfactual scenario calculation (446,000) to the number of unemployed (weighted by 0.3 as the average share of working hours lost due to short-time work in manufacturing). The effect is distributed linearly from April 2021 to January 2022. The dashed line in shows the alternative Phillips curve development. The steepness is now much higher than before and after the Great Recession. This mirrors the expected outward shift due to adverse supply shocks.
V. Conclusion
For an empirical analysis of the effects of supply bottlenecks, we combine survey data and administrative labour market data for sectors in Germany. We quantify effects on the labour market in a panel setting.
The effects on unemployment are noticeable but still relatively limited. This is consistent with the finding that the development of employment in Germany has become less dependent on economic fluctuations (Klinger and Weber Citation2020). The effect on the short-time work notifications, on the other hand, is considerable. It is evidently short-time work that is predominantly used in order to adjust to the material bottlenecks. This follows the general pattern that has emerged from the COVID-19 crisis in both Germany (Gehrke and Weber Citation2020) and Europe (e.g. Giupponi, Landais, and Lapeyre Citation2022). Whilst short-time work is traditionally adopted when there are slumps in demand, our results show that it is also used in the case of supply shocks. With regard to hampering structural change this is usually viewed critically (see Giupponi, Landais, and Lapeyre Citation2022), but in the case of exogenous and temporary shocks, short-time work is an effective means of stabilizing employment until the business activity can recommence or be reframed.
Acknowledgement
We thank Brigitte Hochmuth for helpful suggestions. A previous non-reviewed version of this paper was published in German in Wirtschaftsdienst.
Disclosure statement
No potential conflict of interest was reported by the author(s).
Notes
1 We thank the Ifo Institute for providing the data. Details on the data are described in Appendix A1.
2 Before short-time work can begin, a notification is required.
3 In case of reduced working hours in the form of short-time work, the employees receive pro-rata wage replacement benefits from the unemployment insurance system. The more generous regulations during the pandemic include: The replacement rate rises by 10% points if the reduced hours exceed 50% for more than 3 months, and by a further 10% points after 6 months. The social security contributions are fully paid by the Federal Employment Agency. Short-time work compensation is paid if already 10% of the workers are affected. Temporary agency workers are eligible, too, and secondary employment does not affect short-time work benefits.
References
- Arellano, M., and O. Bover. 1995. “Another Look at the Instrumental Variable Estimation of Error-Components Models.” Journal of Econometrics 68 (1): 29–51. doi:10.1016/0304-4076(94)01642-D.
- Attinasi, M. G., R. De Stefani, E. Frohm, V. Gunnella, G. Koester, M. Tóth, and A. Melemenidis. 2021. “The Semiconductor Shortage and Its Implication for Euro Area Trade, Production and Prices.” Economic Bulletin Boxes, European Central Bank 4. https://www.ecb.europa.eu//pub/economic-bulletin/focus/2021/html/ecb.ebbox202104_06~780de2a8fb.en.html
- Chow, Gregory, and Lin, An-loh 1971 “Best Linear Unbiased Interpolation, Distribution, and Extrapolation of Time Series by Related Series.” The Review of Economics and Statistics 53 (4): 372–375.
- Del Negro, M., M. Lenza, G. E. Primiceri, and A. Tambalotti 2020: “What’s Up with the Phillips Curve?.” Brookings Papers on Economic Activity, 301–357.
- Gehrke, B., and E. Weber 2020: “Short-Time Work, Layoffs, and New Hires in Germany: How the COVID Crisis Differs from the Financial Crisis of 2009.” IAB-Forum, 16.12.
- Giupponi, G., C. Landais, and A. Lapeyre. 2022. “Should We Insure Workers or Jobs During Recessions?.” Journal of Economic Perspectives 36 (2): 29–54. doi:10.1257/jep.36.2.29.
- Guerrieri, Veronica, Lorenzoni, Guido, Straub, Ludwig, and Werning, Iván 2022. “Macroeconomic Implications of Covid-19: Can Negative Supply Shocks Cause Demand Shortages?.” American Economic Review 112 (5): 1437–1474.
- Klinger, S., and E. Weber. 2020. “GDP-Employment Decoupling in Germany.” Structural Change and Economic Dynamics 52: 82–98. doi:10.1016/j.strueco.2019.10.003.
- Krolikowski, P. M., and K. N. Naggert. 2021. “Semiconductor Shortages and Vehicle Production and Prices.“Economic Commentary 2021 (17): 1–7.
- Wohlrabe, K. 2021. “Material Shortages in Industry: Who Is Affected and How Are Companies Reacting?.“ ifo Schnelldienst 74 (9): 60–65.
Appendix
A1: Details on data
The data includes 22 groupings of the manufacturing industry (sector C in the Industrial Classification 2008). Furthermore, we also draw on data from the Ifo Institute’s monthly survey of the main construction industry (divided into structural and civil engineering), which enquires about current hindrances to construction due to material shortages or insufficient technical equipment. To obtain monthly time series, the quarterly material bottleneck variable is interpolated with the aid of the monthly variable for the estimation of inventory levels (likewise Ifo data) in accordance with the Chow-Lin method (Citation1971).
A2: Robustness checks
We carry out various robustness checks in the panel models. In the models for the short-time work notifications and the exits from unemployment, the material bottlenecks exert effects within the contemporaneous month. As a robustness check, we instrument these with their first lag. Here, the effect remains significant even if the coefficients become slightly smaller.
Furthermore, we dispense with production as a control variable. This does not lead to strong changes in the results of the estimation. In a further robustness check, we consider input-output linkages between the sectors. While each sector is directly hit by its own bottlenecks, those in other sectors could lead to reduced demand due to dampened economic activity (Guerrieri et al. Citation2022). To account for this, we premultiply the vector of bottlenecks by an input-output matrix (obtained from the destatis National Accounts) and repeat the regression with the new variable. This only results in small changes.