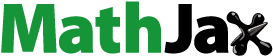
ABSTRACT
We use administrative data () on Finnish individuals who started university studies between 1991 and 2015 to explore whether mathematics and Finnish scores in upper secondary school matriculation examinations are related to university performance. We find that mathematical and verbal skills are complementary in predicting university course completions and grades.
JEL CLASSIFICATION:
I. Introduction
A relatively large literature shows that improving one’s mathematical skills pays off in the labour market (see e.g. Levine and Zimmerman (Citation1995); Joensen and Nielsen (Citation2009); Altonji, Blom, and Meghir (Citation2012)). In some countries policymakers have tended to prioritize the further development of mathematical skills in schools (see e.g. so called ‘algebra for all’-movement Footnote1). Aucejo and James (Citation2021) challenge this view by showing that verbal skills actually have a greater impact on college attendance and graduation probability than mathematical skills, which then translates into better job market outcomes through higher degree achievement. Delaney and Devereux (Citation2020) respond to findings of Aucejo and James (Citation2021) by stating that ‘maths matters’. They show that mathematical skills have a greater impact on degree quality and university completion than verbal skills among students who are selected into Irish universities.
An important aspect in Aucejo and James (Citation2021) is that their estimated production function of mathematical and verbal skills indicates that there is a positive feedback loop from verbal skills to mathematical skills during primary and secondary education. This effect gets stronger as students get older. This finding is in line with educational psychology literature showing that reading skills predict students’ success in solving mathematical word problems (see e.g. Vilenius-Tuohimaa, Aunola, and Nurmi Citation2008; Boonen et al. Citation2016) and that the nature of mathematics problems shifts towards word problems as students progress in their studies. While there is clear evidence of the interplay between mathematical and verbal skills in early education, as Neri, Wagner, and Retelsdorf (Citation2021) pointed out, little is known about the interplay later in life.
In this paper we explore the interplay between mathematical and verbal skills after high school. We document the complementarity of mathematical and verbal skills in predicting the persistence and performance in university studies. We use individual-level administrative data on Finnish students who started university studies between 1991 and 2015. The data allow us to track individuals’ performance through upper secondary school and university by using the results of matriculation examinations and university completion and grades. Our data are unique in the sense that, annually, each secondary school graduate takes the same matriculation exams in mathematics and Finnish and the grading is, in terms of its construction, based on normal distribution.
Our results show statistically significant complementarity of mathematical and verbal skills. Complementarity is most prevalent at high levels of mathematical and verbal skills and prevails both in STEM and non-STEM subjects .Footnote2
II. Data
Our data come from Statistics Finland and universities’ own student records on course completion and grades. Footnote3 We consider only those persons who completed a general program in upper secondary school, took advanced mathematics in matriculation examinations, went directly to university at the tertiary level, and graduated within 10 years. We focus on advanced mathematics students (roughly two-thirds of students) because in Finnish high schools, there are separate curricula and matriculation examinations for students who chose the short-form maths and there is no proper way to project the test results of two different tests into a single measure. After these restrictions our dataset consists of 61,553 individuals.
We use student records to calculate accumulated study credits [European Credit Transfer System (ECTS)] and Grade Point Average (GPA) three calendar years since their first appearance in these records. Footnote4 We construct measures for verbal and mathematical skills using nation-wide upper secondary school matriculation examination scores in Finnish and advanced mathematics. Specifically, we rank the scores into equal – sized 5% groups within each examination round. Footnote5 Our final dataset consists of field of study (6-digit), father’s highest obtained degree (1-digit), starting year of university studies, university ID, gender, ethnic background, and father’s highest obtained degree. summarizes the descriptive statistics.
Table 1. Descriptive statistics.
III. Results
In , we plot the nonparametric LOESS regression results of the relationship between cumulative study credits (GPA) at the end of 3rd study year and the two skills in our data separated into STEM and non-STEM subjects. Footnote6 We find visible evidence of skill complementarity at the boundaries of the plots. When the mathematics score is low, increasing the Finnish score has weaker effect both on credits and GPA compared with when the mathematics score is high. A similar result is observed for verbal skills. In addition, there is a spike at highest level of both skills, indicating that returns to skills might be nonlinear.
Figure 1. Plots of LOESS-regression results. On the horizontal axes, we present the percentiles of matriculation examination results in mathematics and Finnish, and on the vertical axis the projected credits compared to the university – study-year – study-field mean. The smoothing parameter is set to 0.1.
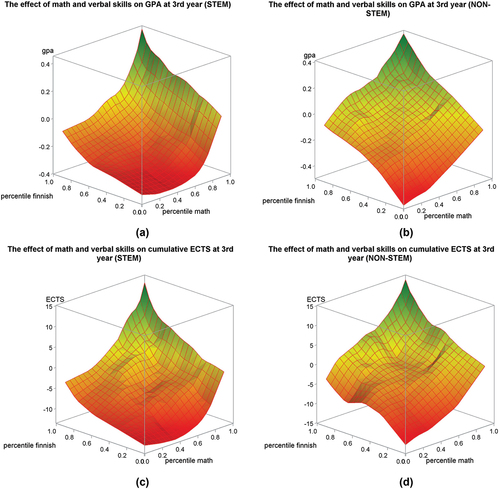
To demonstrate more specific evidence of complementarity, we use the following regressions:
where is either study credits or GPA,
and
are Finnish and mathematics scores,
their cross term,
and
are the higher order terms,
refers to the vector of control variables (gender, ethnic background, father’s highest obtained degree, squared university starting age, and the cross term of the field of study, starting year, and university ID) ,Footnote7 and
are the error terms. We run the regressions separately for STEM and non-STEM subjects.
presents our results. Regressions 1 and 2 show the separate effects of mathematical and verbal skills on GPA and credits. In line with Delaney and Devereux (Citation2020), we find that the mathematics score is a stronger predictor of university performance than the Finnish score. However, it should be noted that results in Aucejo and James (Citation2021) might differ from those in Delaney and Devereux (Citation2020) and ours since the former use a dynamic factor model to measure mathematical and verbal skills whereas we and the latter use a reduced form approach where test scores are treated as direct measures of underlying skills. The incremental effect of mathematical skills is higher in STEM subjects than non-STEM subjects. Increasing the mathematics score by 1%ile increases, on average, the GPA by 0.0059 (0.011) and accumulated study credits by 0.23 (0.37) in non-STEM (STEM) subjects. For verbal skills, we find that, for an increase in the Finnish language score by 1%ile, on average, the GPA increases by 0.0047 (0.0056) grades and accumulated study credits by 0.14 (0.16) in non-STEM (STEM) subjects.
Table 2. Regression results.
In regressions 3 and 4, we include complementarity into the regressions by adding the cross term of the mathematical and verbal skills. We can see that direct effects lose their statistical power, except for mathematics in STEM subjects and the mildly statistically significant effect of verbal skills in non-STEM subjects. The results display clear evidence of complementarity between mathematical and verbal skills. In line with Aucejo and James (Citation2021) we find that verbal skills complement the mathematical skills especially in the STEM fields. Specifically, a certain level of verbal skills is necessary to fully utilize one’s mathematical skills.
For robustness, we explore whether the effect of mathematical skills is nonlinear by adding second and third order terms for mathematics and Finnish scores into the regressions. These results are presented in regressions 5 and 6. We find that the complementarity remains, even when we add non linear terms into the regressions. However, the magnitude of complementarity decreases when nonlinearity is controlled.
IV. Conclusions
Recent research has presented mathematical and verbal scores as distinct skills in educational performance. In this paper, we treat them as complementary and show that this complementarity is an especially important feature in exploring university performance, both for STEM and non-STEM subjects. The complementarity finding indicates that verbal skills might continue to accelerate the accumulation of mathematical skills also later in life.
Lastly, we want to emphasize that further research is needed to properly establish the complementarity of mathematical and verbal skills in university performance. Due to limitation of our sample, we are unable to address (1) whether the standardized test scores are measuring mathematical and verbal skills, (2) the possible bias arising if university selection is driven by mathematical or verbal skills, and (3) the unobservable factors such as school-orientedness, that might explain the complementarity finding.
Disclosure statement
No potential conflict of interest was reported by the authors.
Notes
1 An analysis of it’s implementation in Towering Pines of California school district is done e.g. by Domina et al. (Citation2014).
Preprint submitted to ElsevierMarch 1, 2023
2 STEM (Science, Technology, Engineering and Mathematics) comprises of groups 5. Natural sciences, mathematics and statistics, 6. Information and communication technologies and 7. Engineering, manufacturing and construction in ISCED-2013 classification.
3 We have data from all universities in Finland except for University of Tampere, University of Lappeenranta, and Åbo Akademi University. The missing universities account for roughly 23% of full-time equivalent master’s – level students in Finland.
4 We use GPA and accumulated credits 3 years after first appearance in student records as measures for the student’s success in studies, instead of measuring e.g. the GPA at graduation, because during our study period (from 1991–2005), Finnish university students had permanent study-right which makes the identification of actual graduation time difficult. The students who started their studies after 2005 have a study-right for 7 years, but in practice they can apply for extension which is usually granted. If we measure the length of studies as the monthly difference between observed starting and graduation time, the mean length of studies in our sample is 6.44 years and standard deviation is 1.38 years.
5 The actual grading system uses a similar approach but percentile groups for grades are not of equal size.
6 The purpose of LOESS-regression in this paper is to illustrate the relationship between the two skills and our measures for study success as well as to motivate the model selection in the paper.
7 The controls are chosen to match roughly the set of controls in Delaney and Devereux (Citation2020).
References
- Altonji, J. G., E. Blom, and C. Meghir, 2012. Heterogeneity in Human Capital Investments: High School Curriculum, College Major, and Careers. Working Paper 17985. National Bureau of Economic Research. 10.3386/w17985.
- Aucejo, E., and J. James. 2021. “The Path to College Education: The Role of Math and Verbal Skills.” Journal of Political Economy 129: 2905–2946. doi:10.1086/715417.
- Boonen, A. J. H., B. B. de Koning, J. Jolles, and M. van der Schoot. 2016. “Word Problem Solving in Contemporary Math Education: A Plea for Reading Comprehension Skills Training.” Frontiers in Psychology 7: 191. doi:10.3389/fpsyg.2016.00191.
- Delaney, J. M., and P. J. Devereux. 2020. “Math Matters! The Importance of Mathematical and Verbal Skills for Degree Performance.” Economics Letters 186: 108850. doi:10.1016/j.econlet.2019.108850.
- Domina, T., A. Penner, E. Penner, and A. Conley. 2014. “Algebra for All: California’s Eighth-Grade Algebra Initiative as Constrained Curricula.” Teachers College Record 116 (1970): 1–32. doi:10.1177/016146811411600801.
- Joensen, J. S., and H. S. Nielsen. 2009. “Is There a Causal Effect of High School Math on Labor Market Outcomes?” The Journal of Human Resources 44: 171–198. doi:10.1353/jhr.2009.0004.
- Levine, P., and D. Zimmerman. 1995. “The Benefit of Additional High-School Math and Science Classes for Young Men and Women.” Journal of Business & Economic Statistics 13: 137–149. doi:10.1080/07350015.1995.10524588.
- Neri, N. C., J. Wagner, and J. Retelsdorf. 2021. “What Makes Mathematics Difficult for Adults? The Role of Reading Components in Solving Mathematics Items.” Educational Psychology 1–21. doi:10.1080/01443410.2021.1964438.
- Vilenius-Tuohimaa, P. M., K. Aunola, and J. Nurmi. 2008. “The Association Between Mathematical Word Problems and Reading Comprehension.” Educational Psychology 28: 409–426. doi:10.1080/01443410701708228.