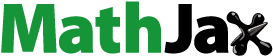
ABSTRACT
The monthly issuance of the World Agriculture Supply and Demand Estimates (WASDE) by the United States Department of Agriculture (USDA) plays a pivotal role in shaping market dynamics through its comprehensive projections and assessments of agricultural commodities. This paper examines the influence of WASDE reports on the intraday volatility of grain futures. We analyse the calendar effect on volatility, focusing on the diurnal patterns on WASDE release days compared to other trading days. This study leverages high-frequency intraday data to explore the immediate and sustained market reactions to the reports, aiming to identify distinct volatility patterns and their implications for traders and market analysts. Our findings confirm the presence of unique diurnal volatility patterns on WASDE release days, significantly diverging from non-release days. This research contributes to the literature by providing refined insights into how public information releases affect market volatility, offering valuable perspectives for understanding and anticipating market movements in response to agricultural reports.
I. Introduction
The United States Department of Agriculture (USDA) publishes the World Agriculture Supply and Demand Estimates (WASDE) report monthly, which is essential for understanding economic and agricultural dynamics. It provides projections on key U.S. crop production, and comprehensive assessments of domestic and international commodity production, trade, consumption, end-of-year stocks, and farm-level pricing. The WASDE report is closely monitored by market analysts, highlighting its importance as a crucial resource for interpreting commodity supply and demand fundamentals (Good and Irwin Citation2003; McKenzie Citation2008; Summer and Mueller Citation1989).
The literature on commodity futures price volatility is comprehensively reviewed and categorized by specific issues in Massa et al. (Citation2023). Initially, research primarily relied on daily price data to analyse daily fluctuations in price volatility. A seminal study by Adjemian (Citation2012) identified volatility patterns surrounding the WASDE release using data from before 2013. More recent studies have utilized high-frequency intraday data to examine realized volatility, identifying variations on the report’s release day and the subsequent market reactions (Adjemian and Irwin Citation2018; Bunek and Janzen Citation2024; Lehecka, Wang, and Garcia Citation2014). These investigations aimed to detect volatility patterns on WASDE release days compared to non-release days by analysing average realized volatility to reveal intraday trends. Furthermore, a recent focus in the literature is the shift in the WASDE release schedule to 11 AM CST in 2013 (Adjemian and Irwin Citation2018; Kauffman Citation2013; Lehecka, Wang, and Garcia Citation2014). These studies have shown increased volatility and different durations of volatility following the schedule change.
This study focuses on investigating the calendar effect, which refers to diurnal patterns of intraday volatility. Specifically, it examines whether volatility patterns on WASDE release days differ from those on other days. Differing from previous research, we employ a novel statistical method (Andersen et al. Citation2023) to evaluate the calendar effect on intraday grain futures price volatility. This method allows us to rigorously analyse diurnal volatility patterns and test their consistency between WASDE release days and non-release days. Moreover, it helps us understand how volatility shifts persist in the futures market post-WASDE release. This temporal approach provides valuable insights into intraday volatility patterns following significant changes in the report release schedule. By utilizing this advanced method, our study not only confirms the existence of diurnal volatility patterns but also contributes to the literature by offering a more nuanced understanding of volatility dynamics in the context of major informational releases.
This research makes two key contributions. First, it pioneers estimating the calendar effect on grain futures price volatility, providing insights beyond traditional analyses of average realized volatility. Second, it highlights intraday volatility patterns, particularly the differences on WASDE release days compared to other days, enhancing our understanding of market dynamics in response to agricultural reports.
II. Data
Our study examined corn and soybean futures price data from January 2013 through April 2023. This specific timeframe was chosen due to notable adjustments in trading hours and WASDE report release timings in late 2012 and early 2013, which significantly influenced market responses to the reports, as highlighted by Adjemian and Irwin (Citation2018). This temporal focus is essential for distinguishing our findings from prior research and emphasizing the nuanced effects of the WASDE report on futures price volatility.
We sourced the WASDE report release dates from the USDA’s official website, where they are systematically listed in Excel format. Our analysis covers 122 distinct release days, except for October 2013 and January 2019, when the reports were not published.
To dissect the nuances of price volatility associated with WASDE report releases, we segmented our dataset into three categories: WASDE release days, the day before releases, and the day after releases. This segmentation facilitated a comprehensive analysis extending from 2013 to April 2023. Post-2013, WASDE reports have been uniformly released at 12:00 PM Eastern Time. Our investigation focused exclusively on price movements during the standard trading hours from 8:30 AM to 2:00 PM, Monday to Friday. The analysed dataset included 122 WASDE release days, each corresponding to a 330-minute trading session, providing a robust dataset of 40,260 observations.
III. Methods
We employ a novel statistical method suggested by Andersen et al. (Citation2023), which assesses the calendar impact on intraday volatilities and tests two distinct patterns in volatility of the two different periods. The method defines an estimator , for the calendar effect on volatility, under the presumption that the logarithmic price process
is observed at discrete intervals, uniformly distributed across the span
. Within each interval
, where
, there exist
observational points
, and the relationship
for
. Notably,
, and for
and
, we define
.
To estimate spot volatilities, considering data points within a specific time interval . We analyse segments
, where k is an integer, and
. The high-frequency logarithmic returns are denoted as
for
,
, and
. We employ the following formulations to approximate the spot volatility
and the average of the integrated volatility:
Initially, we set for
. Based on these values, a general estimator of the calendar effect
on volatility can be approximated as:
We also test whether the estimated volatility profiles within a day remain consistent or exhibit variations across distinct calendar intervals. Considering two separate time spans. We denote their true intraday calendar effects as and
for the nonintersecting intervals
and
. To test the equality of these effects, we set up the following hypotheses. We assert that there is no difference between the volatility patterns under the null hypothesis and a noticeable difference under the alternative hypothesis in the two periods, formulated as:
Following Andersen et al. (Citation2023), we construct the empirical null distribution through simulation (see Andersen et al. Citation2023 for details). We divide the range
into 100 equal subintervals
, where
. Each subinterval follows a chi-square distribution with
degrees of freedom. We then generate 1,000,000 chi-square samples for each grid point
. The summation of the chi-square samples sets the empirical null distribution
. We then sort the empirical distribution
to obtain the p-value.
IV. Results
shows intraday volatility curves for corn and soybeans on the day of the WASDE report release, while shows these curves for the day before and after the release. Both figures reveal a U-shaped volatility pattern common in financial time series.
Figure 1. Intraday volatility calendar effect for corn and soybeans futures prices on WASDE release day.
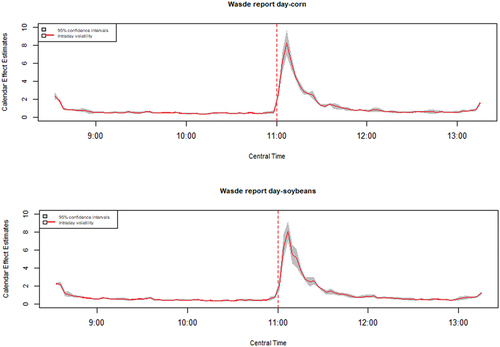
Figure 2. Intraday volatility calendar effect for corn and soybeans futures prices on one day before and after WASDE release.
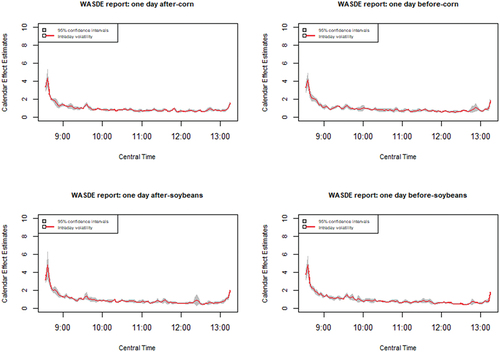
Specifically, illustrates realized volatility and its calendar effect for corn and soybean futures on the WASDE report release day. There is a notable increase in volatility after the 11:00 AM CST release time, which then decreases by 12:00 PM. In contrast, displays realized volatility and its calendar effect for the days surrounding the WASDE release, showing a U-shaped pattern without the sharp volatility increase seen on the release day. The grey shaded areas behind the red lines represent 95% pointwise confidence intervals, following the framework outlined by (Andersen et al. Citation2023), providing statistical support for our analysis. Importantly, increased volatility variability is evident in both corn and soybean futures after the 11:00 AM CST WASDE release, as depicted in , indicating heightened volatility post-release during these periods.
Comparing volatility on WASDE release days to non-release days reveals significant differences. We conducted a formal equality test for intraday calendar effects across three periods: the WASDE release day, the day before, and the day after. The p-values in from our tests confirm that intraday volatility patterns significantly differ on WASDE release days compared to adjacent days. The null hypothesis is rejected for comparisons involving the release day but not for comparing the day before with the day after, suggesting comparable volatility patterns for these days.
Table 1. Test results for equality of intraday volatility pattern by the day of WASDE release.
V. Discussion
Our findings, depicted in , confirm the substantial impact of WASDE report releases on market volatility, particularly evident after April 2013. The spikes in intraday volatility align with patterns identified in Adjemian and Irwin (Citation2018) and are consistent with earlier research analysing volatility trends pre-2013 (Adjemian Citation2012; Bunek and Janzen Citation2024; Fernandez-Perez et al. Citation2019; Lehecka, Wang, and Garcia Citation2014). Through nonparametric testing, we confirmed that volatility patterns vary across three specific days – before, on, and after the WASDE release – extending these observations to periods before the 2013 adjustment in report release timing. The increased variability in post-release volatility suggests monthly fluctuations in the WASDE reports’ market impact, as shown by the wide confidence intervals indicating the range of these effects. The graphs also indicate that the volatility shift lasts less than 60 minutes for both commodities, contrasting with Lehecka et al. (Citation2014) who stated it lasted 10 minutes before the regime change, thus confirming that Kauffman’s (Citation2013) results also apply to the WASDE release regime changes post-2013.
illustrates distinct volatility levels immediately after the market opens across three days. By examining data around the 11 AM WASDE release, we observed a trend where the beginning-of-day realized volatility on WASDE release days is lower than on other days. This suggests that while financial series typically experience an initial surge in volatility, the market’s response on WASDE days is more subdued, likely due to traders anticipating the report. The WASDE report serves as a crucial benchmark for production and inventory forecasts, influencing trader behaviour on release days.
presents the test results for the equality of daily volatility patterns, indicating significant differences between the release day and the adjacent days but no notable difference between the day before and after the release. These results support our hypothesis regarding unique market behaviours on WASDE release days.
Table 2. Test results for equality of intraday volatility pattern by the day of WASDE release with sample before 11:00 AM.
VI. Conclusion
Our study identifies unique diurnal volatility patterns on days when the WASDE report is released. These patterns underscore the significant influence of WASDE reports on market behaviour, particularly in corn and soybeans futures markets
Our approach to estimating the calendar effect opens avenues for further investigation into the market’s response to WASDE reports, particularly considering the 2013 changes in report release and trading start times. Future studies could explore the consistency of these calendar effects over varied periods to better understand the impacts of these regime changes.
Disclosure statement
No potential conflict of interest was reported by the author(s).
References
- Adjemian, M. K. 2012. “Quantifying the WASDE Announcement Effect.” American Journal of Agricultural Economics 94 (1): 238–256. https://doi.org/10.1093/ajae/aar131.
- Adjemian, M. K., and S. H. Irwin. 2018. “USDA Announcement Effects in Real-Time.” American Journal of Agricultural Economics 100 (4): 1151–1171. https://doi.org/10.1093/ajae/aay018.
- Andersen, T. G., T. Su, V. Todorov, and Z. Zhang. 2023. “Intraday Periodic Volatility Curves.” Journal of the American Statistical Association 119 (546): 1181–1191. https://doi.org/10.1080/01621459.2023.2177546.
- Bunek, G. D., and J. P. Janzen. 2024. “Does Public Information Facilitate Price Consensus? Characterizing USDA Announcement Effects Using Realized Volatility.” Journal of Commodity Markets 33:100382. https://doi.org/10.1016/j.jcomm.2024.100382.
- Fernandez-Perez, A., B. Frijns, I. Indriawan, and A. Tourani-Rad. 2019. “Surprise and Dispersion: Informational Impact of USDA Announcements.” Agricultural Economics 50 (1): 113–126. https://doi.org/10.1111/agec.12470.
- Good, D. L., and S. H. Irwin. 2003. Understanding USDA Corn and Soybean Production Forecasts: An Overview of Methods, Performance and Market Impacts. AgMAS Project Research Reports. Urbana-Champaign, IL, USA: Dept of Agr and Con Econ. University of Illinois Urbana-Champaign. https://doi.org/10.22004/ag.econ.37496.
- Kauffman, N. 2013. “Have Extended Trading Hours Made Agricultural Commodity Markets Riskier?” Economic Review-Federal Reserve Bank of Kansas City Q III 67–94.
- Lehecka, G. V., X. Wang, and P. Garcia. 2014. “Gone in Ten Minutes: Intraday Evidence of Announcement Effects in the Electronic Corn Futures Market.” Applied Economic Perspectives and Policy 36 (3): 504–526. https://doi.org/10.1093/aepp/ppu010.
- Massa, O. I., B. Karali, and S. H. Irwin. 2023. “What Do We Know About the Value and Market Impact of the US Department of Agriculture Reports?” Applied Economic Perspectives and Policy 46 (2): 698–736. https://doi.org/10.1002/aepp.13409.
- McKenzie, A. M. 2008. “Pre‐Harvest Price Expectations for Corn: The Information Content of USDA Reports and New Crop Futures.” American Journal of Agricultural Economics 90 (2): 351–366. https://doi.org/10.1111/j.1467-8276.2007.01117.x.
- Summer, D. A., and R. A. Mueller. 1989. “Are Harvest Forecasts News? USDA Announcements and Futures Market Reactions.” American Journal of Agricultural Economics 71 (1): 1–8. https://doi.org/10.2307/1241769.