Abstract
Blur detection is affected by retinal eccentricity, but is it also affected by attentional resources? Research showing effects of selective attention on acuity and contrast sensitivity suggests that allocating attention should increase blur detection. However, research showing that blur affects selection of saccade targets suggests that blur detection may be pre-attentive. To investigate this question, we carried out experiments in which viewers detected blur in real-world scenes under varying levels of cognitive load manipulated by the N-back task. We used adaptive threshold estimation to measure blur detection thresholds at 0°, 3°, 6°, and 9° eccentricity. Participants carried out blur detection as a single task, a single task with to-be-ignored letters, or an N-back task with four levels of cognitive load (0, 1, 2, or 3-back). In Experiment 1, blur was presented gaze-contingently for occasional single eye fixations while participants viewed scenes in preparation for an easy picture recognition memory task, and the N-back stimuli were presented auditorily. The results for three participants showed a large effect of retinal eccentricity on blur thresholds, significant effects of N-back level on N-back performance, scene recognition memory, and gaze dispersion, but no effect of N-back level on blur thresholds. In Experiment 2, we replicated Experiment 1 but presented the images tachistoscopically for 200 ms (half with, half without blur), to determine whether gaze-contingent blur presentation in Experiment 1 had produced attentional capture by blur onset during a fixation, thus eliminating any effect of cognitive load on blur detection. The results with three new participants replicated those of Experiment 1, indicating that the use of gaze-contingent blur presentation could not explain the lack of effect of cognitive load on blur detection. Thus, apparently blur detection in real-world scene images is unaffected by attentional resources, as manipulated by the cognitive load produced by the N-back task.
This research was supported by a grant from the Office of Naval Research to LL [#10846128]. We thank Jeremy Wolfe, Nelson Cowan, Jeffrey Rouder, Michael Dodd, and Irving Biederman for helpful comments, and Gabriel Hughes and Kevin E. Dean for technical assistance and programming.
Research in this article was previously presented at the 2013 Annual Meeting of the Vision Sciences Society, with the abstract published in the Journal of Vision.
INTRODUCTION
Blur detection and attention
Blur is a natural part of vision, and occurs due to changes in focal length, distance between objects in depth, motion, and vision problems such as refractive errors (e.g., myopia, hyperopia, or astigmatism). For people with normal vision, blur commonly occurs when an object they are looking at is brought closer, such as when picking up a picture to view it. This action causes a change in the focal length of the image on the retina, creating blur, which is detected by the visual system, and if it is sufficiently blurred, results in accommodating the lens to bring the retinal image into focus.
Perceptual blur can be defined in terms of a difference between the highest spatial frequencies that can be resolved in a stimulus relative to the potentially resolvable frequencies in that stimulus for a particular individual (Sawides, de Gracia, Dorronsoro, Webster, & Marcos, Citation2011). Importantly, not all loss of resolution is perceived as blur. For example, when imagery shown on a display is blurred progressively with increasing distance from the centre of vision, but the highest spatial frequencies at each retinal eccentricity are still higher than the visual system can resolve, viewers do not perceive the image as having been blurred (Loschky, McConkie, Yang, & Miller, Citation2005). Conversely, when the degree of image blurring exceeds a threshold, such that the highest spatial frequencies removed would have been potentially resolvable, then blur is detected (Loschky et al., Citation2005). The discussion raises an interesting question: how does blur detection relate to spatial attention? The current study investigates this question.
Researchers have shown that when blur exceeds the limits of resolution at a given retinal eccentricity by an amount greater than the depth of focus at that eccentricity, called “the effective accommodative error”, an accommodation response is made (Ciuffreda, Wang, & Vasudevan, Citation2007). Nevertheless, individuals show different slopes for their depth of focus by retinal eccentricity function (Ciuffreda, Wang, & Wong, Citation2005). The authors argued that this individual variability could be explained in terms of viewers’ breadth of attention. Specifically, some people might have a broader “attentional blur gain”, showing an accommodation response to peripheral blur, while others might have a local attentional blur gain, showing an accommodation response only to blur in their fovea (Ciuffreda et al., Citation2005, p. 2657).
Research questions and hypotheses
The above proposal of Ciuffreda and colleagues regarding the accommodation response and attention is consistent with research on the role of attention on visual resolution and contrast sensitivity. Research has shown that attention intensifies the sensory strength of a stimulus (e.g., Carrasco, Ling, & Read, Citation2004; Pestilli & Carrasco, Citation2005; Treue, Citation2004; Yeshurun, & Carrasco, Citation1998). Shifting attention to a region of the field of view, either endogenously or exogenously, can increase the resolvable contrast, spatial frequency, or salience of a stimulus at the attended location (Carrasco et al., Citation2004; Carrasco, Williams, & Yeshurun, Citation2002; Yeshurun & Carrasco, Citation1998, Citation1999). Furthermore, electrophysiological studies have shown that attention can alter neuronal responses to stimuli (Lee & Maunsell, Citation2010; McAdams & Maunsell, Citation1999; Moran & Desimone, Citation1985; Reynolds, Pasternak, & Desimone, Citation2000; Treue & Maunsell, Citation1996; Williford & Maunsell, Citation2006). Therefore, since attention modulates both neuronal and behavioural sensitivity, attending to a region may enable viewers to better detect threshold level blur there. If so, blur detection could serve as an index of a viewer's attentional breadth, as suggested by Ciuffreda et al. (Citation2005).
Alternatively, attention might play no causal role in either the accommodation response, or blur detection. Accommodation may occur without attention given that it is an autonomic process (Olmsted, Citation1944) with a short latency (200-350 ms) (Leukart, Citation1939; Lockhart & Shi, Citation2010). Like accommodation, the assumed function of making saccades is to decrease retinal sampling blur, in this case by moving the high-resolution fovea to points of interest in the visual periphery, in order to view them with greater resolution. Importantly, many theories of saccade targeting assume it is guided by pre-attentive processes. Pre-attentive processes are assumed to operate without attention, very quickly, in parallel across the field of view, on the basis of single perceptual features (e.g., colour, orientation, size, or direction of illumination) (Ramachandran, Citation1988; Treisman & Gormican, Citation1988; Wolfe & Horowitz, Citation2004), and perhaps blur is detected pre-attentively. Consistent with blur guiding saccade targeting, studies have consistently shown that when peripheral image blur is relatively intense, saccades are more likely to be targeted to less blurred regions (Enns & MacDonald, Citation2012; Loschky & McConkie, Citation2002; Nystrom & Holmqvist, Citation2007; Smith & Tadmor, Citation2012).Footnote1 Consistent with this idea, a similar effect has been shown on covert attentional selection, such that high-resolution target letter identification was worse when the letters were flashed to an eye viewing a video through a blurring lens compared to an eye viewing video through a clear lens (Shors, Wright, & Greene, Citation1992). Thus, it is possible that visual functions designed to decrease image blur, such as accommodation and saccade targeting, may be driven pre-attentively by blur detection. If so, then the degree of attention allocated to blur detection would have no effect on it.
In sum, on the one hand, research on accommodation and on the effects of attention on peripheral visual resolution suggest that blur detection sensitivity should be increased by attention. On the other hand, research on the effects of blur on overt and covert attention suggest that blur detection occurs pre-attentively. If so, then the degree of attention allocated to blur detection would have no effect on it.
In order to test these alternative hypotheses, we used blur detection thresholds as our dependent variable, while people looked at real-world scenes and we manipulated their attention by varying their cognitive load in a concurrent task. Importantly, this manipulation assumes that tasks requiring visual attention are disrupted when one taxes general executive attentional resources (either spatial or non-spatial, and either in the same or a different sensory modality), which has, indeed, been shown repeatedly (Crundall, Underwood, & Chapman, Citation2002; Matsukura, Brockmole, Boot, & Henderson, Citation2011; Mitchell, Macrae, & Gilchrist, Citation2002; Pomplun, Reingold, & Shen, Citation2001; Recarte & Nunes, Citation2003; Reimer, Mehler, Wang, & Coughlin, Citation2012). Equally importantly, blur detection in real world scene images varies considerably with retinal eccentricity due to the limits of visual resolution/contrast sensitivity across the field of view (Loschky et al., Citation2005). Thus, this must be taken account of in order to avoid confounding eccentricity-dependent contrast sensitivity effects and cognitive load effects on blur detection. Also importantly, we used a small-N design (three participants per experiment) in order to measure each participant's blur detection thresholds using an adaptive threshold estimation procedure. This controlled for individual differences in blur sensitivity, enabling us to probe the effects of cognitive load on blur thresholds with maximal sensitivity and reliability (having > 6000 observations per participant).
GENERAL METHOD
Apparatus
All experiments were conducted on a Origin Genesis PC running Microsoft Windows 7, with an Intel Core i7 970 processor (3.2 GHz), 24 GB DDR3 RAM, and a 2GB Radeon HD6950 video card. Stimuli were presented on a ViewSonic 19” CRT monitor (Model G90fb) at 85 Hz refresh rate, and at a screen resolution of 1024 x 768 pixels. A chin rest was used to stabilize head position at 60.33 cm away from the screen, providing a viewing angle of 33.67° x 25.50° for all images. The display was calibrated with a Spyder3Elite photometer with a maximum and minimum luminance of 91.3 cd/m2 and 0.33 cd/m2, respectively, and a gamma of 2.21.
Eye position was acquired using a video-based eye movement monitor (EyeLink 1000/2K, SR Research, Ontario). The EyeLink system recorded monocular eye position with a sampling resolution of 1000 Hz. Given the expected delay between an eye movement and the update of the stimulus on the screen, gaze-contingent display change latency was measured using an artificial eye (see Appendix A in Bernard, Scherlen & Castet, Citation2007 for details). We measured 100 recordings of the display change latency between the activation of the infrared LED and the corresponding change in resistance of the photosensitive diode, using a USB data acquisition device (USB-204, Measurement Computing), and calculating the latency in MATLAB. The measured latency in our apparatus ranged between 18.25 and 22.25 ms (Mean = 20 ms, 95% confidence interval of 19.75–20.25 ms), well under the 80 ms latency shown to first produce increased gaze-contingent image blur detection rates (Loschky & Wolverton, Citation2007). Consequently, the gaze-contingent display updates should not increase the detectability of blur within our participants.
Stimuli
We used 1296 images (1024 x 768 pixels) from the SUN image database (Xiao, Hays, Ehinger, Oliva, & Torralba, Citation2010). The images belonged to a large number of scene categories, including forests, mountains, street scenes, and building interiors. Images were excluded if they contained poor image defocus, predominantly low-frequency information, or watermarks.
To determine the degree to which participants could detect blur on specific fixations, we used the occasional gaze-contingent blur detection task (Loschky et al., Citation2005; Loschky & Wolverton, Citation2007). This task uses a variation on the “moving window” methodology originally pioneered by McConkie and Rayner to study the perceptual span in reading (McConkie & Rayner, Citation1975; Rayner, Citation1975), and later applied to study the visual span in scene perception (Loschky & McConkie, Citation2002; Loschky et al., Citation2005; Parkhurst, Culurciello, & Neibur, Citation2000; Reingold & Loschky, Citation2002; Sere, Marendaz, & Herault, Citation2000; Shioiri & Ikeda, Citation1989; van Diepen & d'Ydewalle, Citation2003). In the occasional gaze-contingent blur detection task, we use a gaze-contingent bi-resolution display, or moving window, in which images are presented with two levels of resolution, a circle of high resolution imagery surrounded by lower resolution imagery, with the centre of high resolution placed at the centre of gaze using eyetracking (Duchowski, Cournia, & Murphy, Citation2004; Loschky & McConkie, Citation2002; Reingold, Loschky, McConkie, & Stampe, Citation2003). We used a Gaussian low-pass filter (Gonzalez, Woods, & Eddins, Citation2009) when blurring an image, which has previously been shown to have linear detection properties (Murray & Bex, Citation2010). The Gaussian low-pass filters were defined by:
A video showing an example of such a gaze-contingent, occasional, bi-resolutional display can be seen in Video 1 (http://www.youtube.com/watch?v=-iSj14n9ufc&feature=youtu.be).
Procedure
Procedural overview
Within a trial, participants carried out three different tasks: (1) Memorization of the scene image, for a later (relatively easy) picture recognition task. This was done to encourage participants to actively explore the image with many eye movements. (2) Blur detection in the image, which only occurred occasionally for single fixations. Blur levels were varied across eccentricity, and thresholds were calculated using an adaptive threshold estimation algorithm that dynamically manipulated blur levels throughout the experiment. The trial continued until the participant made the requisite number of fixations, producing the requisite number of blur (and catch) presentations for every trial. (3) A cognitive load task (N-back), which varied in terms of the load from block to block. Below we describe each component of the experiment in greater detail.
Eyetracker calibration
A nine-point calibration routine was performed at the beginning of each block of trials, with a second nine-point calibration grid used to calculate the accuracy of the calibration. Calibration was repeated if the average spatial accuracy of all nine points was worse than 0.5°. Over all participants, the mean gaze error was 0.42° (SD = 0.14°), with a maximum error of 0.77° (SD = 0.21°) at the calibration positions closest to the corner of the screen. In addition, before each trial, a drift-correction procedure was used to revalidate the calibration, and to provide a common starting location for the task. The average offset correction over all participants was 0.22° (SD = 0.11°).
Scene memorization task
Because the blur detection task required that viewers make large numbers of eye movements (in order to produce sufficient numbers of blur presentations), participants were given a picture recognition task to encourage them to make many exploratory eye movements. In the “Learning Phase”, participants viewed a set of real-world scenes one time each in preparation for the later recognition test. In the later “Memory Phase” at the end of each block of trials, participants were given a new–old recognition memory test; 50% of the images were old, and 50% new, with each test image being presented for three seconds, followed by an old/new prompt to which they responded with a button press. Images were randomly assigned to Learning and Memory phases, and no images were ever repeated either within or across sessions (except showing each “old” image once again during its recognition memory test). The memory tests both motivated participants to thoroughly explore the scene images, and provided an additional converging measure of the effects of cognitive load on visual processing (Matsukura, Brockmole Boot, & Henderson, Citation2011).
Blur detection task
We used a simple detection task to test viewers’ ability to detect occasional gaze-contingent blur. For purposes of signal detection analyses, we presented an equal number of blur-present and blur-absent displays, which occurred on every 7th fixation. The order of blur-present/absent displays was randomized to prevent participants from detecting patterns in the displays. On blur-present displays, blur was presented for a single eye fixation, with the normal unaltered image shown on 13 out of 14 fixations. Participants pressed a mouse button with their right hand when they detected blur (Loschky et al., Citation2005; Loschky & Wolverton, Citation2007), and had up until the next blur-present/absent display (i.e., seven fixations) to make a detection response. During blur-absent displays, an identical copy of the original unaltered image was presented for the same duration.
As shown in , blur was presented at one of four retinal eccentricities (0°, 3°, 6° and 9°), outside of a gaze-contingent circular window, with blur values being controlled by the Single Interval Adjustment Matrix (SIAM) adaptive threshold estimation procedure, using a targeted accuracy rate of 50% (Kaernbach, Citation1990). Inside the window was unaltered imagery (thus, in the 0° window condition, the entire image was blurred). Each trial image ended when the participant had made 56 fixations (1 presentation every 7 fixations x 2 blur-present/absent displays x 4 eccentricities), with the final gaze-contingent blur (-present or -absent) presentation followed by 6 fixations within which to make a response. Order of eccentricities was randomized for each trial/image, and we estimated blur thresholds for each eccentricity on a per-block basis, with the estimations being continuously updated across all images within a block.
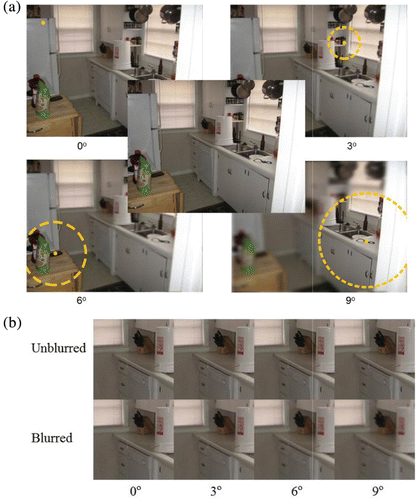
Cognitive load task
We used an N-back go-no-go running memory task using letter targets, which is known to reliably manipulate cognitive load (Cohen et al., Citation1997; Jaeggi, Buschkuehl, Perrig, & Meier, Citation2010; Kane, Conway, Miura, & Colflesh, Citation2007; Kirchner, Citation1958; Owen, McMillan, Laird, & Bullmore, Citation2005). The task requires a participant to hold in working memory a list of N-items in order to check if the most recently presented item is the same as the item presented N-items back in the list. If an item was valid, participants made a go-response on a game controller. Small N-back values (e.g., 0 or 1-back) produce a minimal cognitive load, while larger N-back intervals (e.g., 2 or 3-back) produce stronger cognitive load effects (Cohen et al., Citation1997; Jaeggi et al., Citation2010). Critically important for the current study, the time period between N-back item presentations (e.g., 2000–3000 ms ISI) (Chen, Mitra, & Schlaghecken, Citation2008; Jaeggi et al., Citation2010; Owen et al., Citation2005), while seemingly long in terms of visual processing times, is not long in terms of performing the N-back task, and is filled with numerous executive mental operations. These likely include matching the newest item with the one N-back in the list, deciding whether to make a response (including resolving interference from distractors), either making or inhibiting a response, then shifting the N-1 back item to the N-back list position, replacing the previous N-back item with the new one, and possibly also rehearsing the relevant section of the new list (Chen et al., Citation2008; Jaeggi et al., Citation2010).
In addition to 0, 1, 2, and 3-back tasks, we also included two control conditions: (1) N-back letters presented while participants were instructed to ignore them. (2) Single-task blur detection with no N-back letters. N-back performance feedback (% correct) was given after every six images to ensure that participants were sufficiently engaged in the cognitive load task. Importantly, no feedback was given on the blur detection task so that participants were encouraged to prioritize the N-back task. A video showing the complete task, including auditory N-back can be seen and heard in Video 2 (http://www.youtube.com/watch?v=PZEGOINT-Ok&feature=youtu.be).
Experimental design
The experiment consisted of six replications. Each replication contained all six cognitive load blocks, including four N-back levels, and two control conditions. To control for practice and fatigue effects, the order of cognitive load and control condition blocks was counter-balanced across six replications using a Latin-square, which was different for each participant. Each block consisted of 24 scene images. Because each image included one pair of blur-present/absent presentations for each eccentricity, a block of 24 images produced 48 blur threshold measures per eccentricity for a given cognitive load. Because there were six cognitive load blocks per replication, each replication took between 1.5 to 2 hours to complete. Participants completed all six replications of the experiment over a period of one week. Thus, participants viewed 864 unique images (24 images x 6 N-back blocks x 6 replications) resulting in 6912 blur detection observations per participant. However, only a fraction of these observations (reversals in each adaptive threshold staircase, excluding the first four) were used in the final analyses to estimate blur thresholds.
Practice trials
Prior to participating in the actual experiment, participants completed a practice session consisting of the blur detection task with all six levels of cognitive load (three images per level) so participants would become familiar with the tasks and their respective difficulty levels.
EXPERIMENT 1
The current experiment tested the effect of cognitive load on blur detection using an auditory N-back task. It was reasoned that the use of an auditory cognitive load would avoid introducing interfering visual stimuli into the display, which might unintentionally make the blur detection task more difficult.
Method
Participants
Three undergraduate lab members completed six sessions of the blur detection task. All participants had normal near acuity (≥ 20/30) using a Snellen acuity chart, and gave informed consent to participate in the study for course credit or as volunteers.
Procedure
The procedures were as described in the General Method, with the following qualifications. In the current experiment, the N-back was administered auditorily, with letter sounds presented for approximately 630 ms with inter-stimulus intervals (ISIs) of 2000 ms.
Results
For all statistical tests of significance of main effects and interactions, we used weighted mean threshold estimates in a Restricted Estimate of Maximum Likelihood (REML) analysis (Kenward & Roger, Citation1997).
N-back sensitivity
As shown in , the N-back results showed that participants were less sensitive in the N-back task as N increased from 0 to 3-back, F(3, 6) = 10.371, p = .008. This showed that the N-back task was capable of creating a cognitive load for our participants.
Memory performance as a function of cognitive load
As shown in , scene recognition memory accuracy showed a consistent, negative trend with increasing levels of N-back, F(5, 10.88) = 4.03, p = .027. The logically strongest test of this effect is to compare the simplest task (single-task, no sound control condition) to the most difficult task (dual-task 3-back), which shows a strong effect of cognitive load, F(1, 11.23) = 10.04, p = 0.009, using a least squared mean contrast. This suggests that the N-back task manipulated cognitive load to the extent that the visual processing involved in encoding simple recognition memory was affected (see also Matsukura et al., Citation2011).
Eye movement parameters as a function of cognitive load
We also examined the effects of N-back level on several eye-movement parameters known to reflect the effects of cognitive load: fixation durations, which should increase with cognitive load (Just & Carpenter, Citation1980; Nuthmann, Smith, Engbert, & Henderson, Citation2010; Rayner, Citation1998), saccadic amplitudes, which should decrease with cognitive load (Henderson & Hollingworth, Citation1998, Citation1999; Rayner, Citation1998), and fixation location dispersion, which should decrease with cognitive load (Miura, Citation1986; Recarte & Nunes, Citation2003; Reimer, Mehler, Wang, & Coughlin, Citation2012). For fixation durations and saccadic amplitudes, cognitive load produced mixed and uninterpretable results, which were not statistically significant; fixation durations, F(5, 10.01) = 0.335, p = .881; saccadic amplitudes, F(5, 10.06) = 1.214, p = 0.370. However, as shown in , the dispersion of fixation locations, quantified in terms of the bivariate contour ellipse (BCE, e.g., Crossland & Rubin, Citation2002), showed a clear and significant decrease with increasing cognitive load, F(5, 10) = 19.283, p < .0001.Footnote2 Again, the logically strongest test of this effect is the comparison of the single-task, no sound control condition to the dual-task 3-back condition, which shows a strong effect of cognitive load, F(1, 10) = 57.36, p < .0001. This is consistent with prior results showing that an increase in cognitive load produces a more restricted distribution of fixation locations, which has been interpreted as a reduction in viewers’ breadth of attention or useful field of view (UFOV) (Miura, Citation1986; Recarte & Nunes, Citation2003; Reimer et al., Citation2012).
Blur sensitivity
To estimate individual participants’ blur detection thresholds for each retinal eccentricity, cognitive load level, and session, we calculated the mean cpd values for those stimuli for which a participant's response triggered a reversal in stimulus magnitude in the adaptive threshold staircase procedure. As suggested by Kaernbach (Citation1990), prior to calculating the mean reversal values, we removed the first four reversals from each threshold estimation. These mean threshold estimates were used in a REML analysis to determine the fixed effects of retinal eccentricity and cognitive load for each participant (which was a random effect).
Blur sensitivity as a function of retinal eccentricity
As shown in , and as expected, blur detection spatial frequency cut-off thresholds (in cpd) decreased as retinal eccentricity increased, F (1, 1.661) = 3930.56, p = .0008. Thus, the highest spatial frequency blur thresholds were at 0° eccentricity (i.e., an infinitely small high-resolution window), which ranged from 29.7–18.7 cpd. Conversely, the lowest spatial frequency blur thresholds were at 9° eccentricity, which ranged from 11.1–3.9 cpd. This empirically-derived blur detection spatial frequency drop-off function is close to the blur detection threshold drop-off function given in Loschky et al. (Citation2005, p. 1082, Equation 3), fc = 43.1 * 1.55/(1.55 + E), where fc is the frequency cut-off, and E is eccentricity, and whose form was based on numerous previous studies of eccentricity-dependent contrast sensitivity.
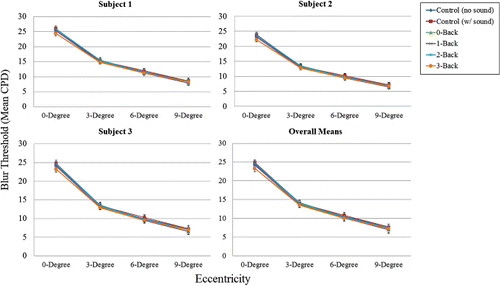
We can conclude that our use of the occasional, gaze-contingent, bi-resolutional blur detection task, together with the SIAM adaptive threshold estimation algorithm, was sensitive to changes in blur detection both across the visual field and between individual participants.
Blur sensitivity as a function of cognitive load
demonstrates more clearly the relationship between blur threshold and cognitive load (N-back and control conditions). As can be seen in , there was no main effect of cognitive load on blur detection thresholds, F(5, 8.181) = 2.248, p = 0.146, nor any interaction with eccentricity, F(5, 6.985) = 1.778, p = 0.236. In this case, even the logically strongest test of the effect of cognitive load, namely the comparison between the single-task, no sound control condition and the dual-task 3-back condition, showed no meaningful or significant difference in blur thresholds, F(1, 6.06) = 2.43, p = .170. This null effect of N-back cognitive load on blur detection thresholds is in stark contrast to (a) the strong effects of retinal eccentricity on blur detection thresholds, and (b) the clear effects of N-back cognitive load on both scene recognition memory performance and fixation location dispersion.
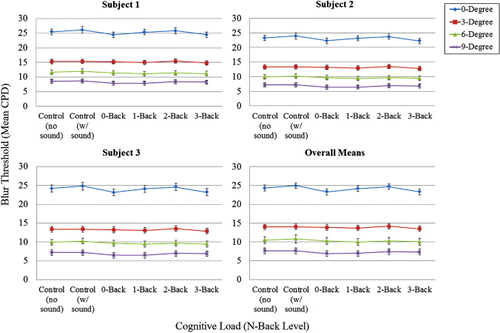
Discussion
Contrary to expectations that differences in attentional resources would affect blur detection across the visual field, we found that cognitive load had no effect on blur detection thresholds. Specifically, the results are inconsistent with the hypothesis that, when viewers have more attentional resources available, they will be more likely to detect blur that is at or near threshold, thus increasing their blur sensitivity. Instead, the current results are consistent with the hypothesis that blur is detected pre-attentively. In this hypothesis, changes in cognitive load would not be expected to affect blur detection, since blur detection would not require attention.
Importantly, we cannot explain the lack of effect of cognitive load on blur detection due to an insensitive measure of blur thresholds. This is because we have shown that our blur threshold estimates decrease with eccentricity as expected, and the thresholds differ between individuals as one might expect. Thus, we clearly had a sensitive measure of blur thresholds, but they were not influenced by N-back cognitive load.
Likewise, the lack of effect of cognitive load on blur thresholds cannot be explained by a lack of cognitive load. This is based on three findings in the experiment: (1) participants’ sensitivity in the N-back task significantly decreased with increasing N-back level, consistent with the idea that increasing N-back levels were increasingly difficult; (2) participants’ scene recognition memory significantly decreased with increasing N-back level, consistent with the idea that it caused a cognitive load, which hindered visual processing; and (3) participants’ fixation location dispersion significantly decreased with N-back level, consistent with the idea that it caused a cognitive load, which reduced viewers’ attentional breadth. Thus, the N-back clearly did cause a cognitive load, but it did not affect blur detection thresholds.
An alternative explanation for the results of Experiment 1 is that the cognitive load, namely the N-back task, was presented auditorily, whereas the blur detection task was visual. For example, according to Wickens’ multiple resource theory (Citation2002), tasks engaging the auditory modality are less likely to compete for attentional resources with tasks engaging the visual modality. Nevertheless, a study of the visual span (or useful field of view) in an attentionally demanding comparative visual search task (Pomplun, Reingold, & Shen, Citation2001) found that an auditory working memory load task was effective in reducing viewers’ visual span. Additionally, we have carried out a follow-up control experiment identical to Experiment 1, except that the N-back letters were presented visually (with a 3000 ms ISI to equate N-back difficulty to that in Experiment 1) and found essentially identical results. Thus, the modality of the cognitive load cannot explain our null effect of the N-back cognitive load on blur detection.
EXPERIMENT 2
Purpose
A possible explanation for the null effect of cognitive load on blur detection in Experiment 1 is that the blur onset captured attention because it was presented gaze-contingently. Specifically, because there is a non-zero delay between identifying the current gaze position on a critical fixation, and the display of the gaze-contingent bi-resolution display, the blur onset was always early in a fixation. Thus, the unblurred base image was already present on the retina during a fixation before the blur appeared, so the blur might have been perceived as a motion transient, thereby capturing attention. This is despite the results of our previous research showing that gaze-contingent multi-resolution display update delays as long as 60 ms do not increase blur detection (Loschky & Wolverton, Citation2007), whereas our own study had average delays of only 20 ms. Nevertheless, attentional capture by a gaze-contingent update delay-based artifact is a plausible explanation for the lack of effects of cognitive load on blur detection. To rule out such an explanation, in Experiment 2 we presented our blurred and un-blurred images non-gaze-contingently, or “tachistoscopically”. That is, participants fixated the centre of the screen (while their eyes were tracked) and each image was briefly flashed there for a duration too brief for participants to move their eye before it disappeared.
Of critical importance, using this method, any blur was an integral part of the image, such that the blur and the rest of the image onset together. Thus, the blur could not capture attention through a motion onset. Thus, if the gaze-contingent presentation of the blur in Experiment 1 explains the observed lack of effect of cognitive load on blur detection, then we should find an effect of cognitive load with the tachistoscopic presentation in Experiment 2.
Method
Participants
Three lab members, who were not in Experiment 1, two undergraduates and one graduate, completed six sessions of the blur detection task. All participants had normal near acuity (≥ 20/30) using a Snellen acuity chart, and gave informed consent to participate in the study for course credit or as volunteers.
Procedure
The procedures were as described in the General Method with the following qualifications. In contrast to Experiment 1, the blur detection task was presented tachistoscopically. Specifically, as illustrated in , at the start of each trial, participants fixated a central target and went through a drift correction procedure, then initiated the trial with a button press. Participants were then required to maintain gaze at the centre of the screen for the duration of the trial. A series of eight images was briefly flashed at the centre of the screen, each for a duration of 200 ms, unmasked, with a 3000 ms ISI between images (). Of the eight images, half were blurred at one of the four retinal eccentricities (0°, 3°, 6°, or 9°), and half were unaltered images, with blurred and unblurred images interleaved randomly. If a participant blinked or moved their eyes as an image was being drawn to the screen, the image presentation was repeated at the end of the trial. As in Experiment 1, the N-back task was presented auditorily, with letter sounds presented for approximately 630 ms with ISIs of 2000 ms. Additionally, the scene recognition memory task was eliminated because there was no need to encourage participants to make multiple fixations on the images – they could only see each image for the duration of a single (relatively short) eye fixation.
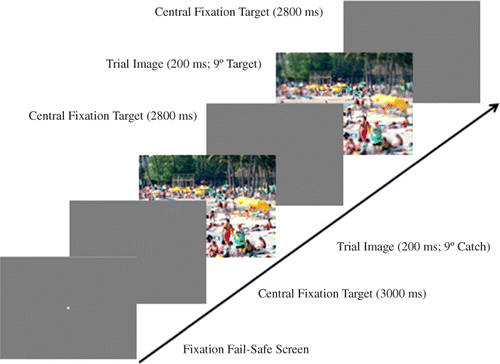
Results
N-back sensitivity
As shown in , the N-back results again showed that participants indeed were less sensitive in the N-back task as N increased from 0 to 3-back, F(3, 6.05) = 25.59, p < .001. This replicates the pattern found in Experiment 1, again showing that the N-back task created a cognitive load for our participants.
Blur sensitivity
We used the same analytical procedures as Experiment 1.
Blur sensitivity as a function of retinal eccentricity
As shown in , the blur detection threshold results showed the same sort of decrease in spatial frequency cut-off as a function of retinal eccentricity as found in Experiment 1, F(1, 1.99) = 131.85, p = .008. Likewise, we found minor individual differences in eccentricity-dependent blur detection threshold functions for each of our participants. Again, as in Experiment 1, our bi-resolutional blur detection task with the adaptive threshold estimation algorithm was quite sensitive to changes in blur detection across the visual field and between individual participants. Thus, whether blur was presented gaze-contingently or tachistoscopically, we were able to measure similar blur threshold functions.
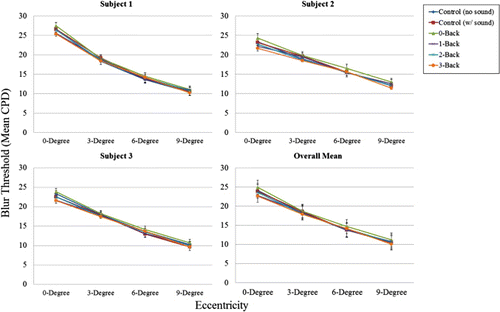
Blur sensitivity as a function of cognitive load
shows that, as in Experiment 1, there was a null effect of cognitive load on blur detection, F(5, 9.81) = 2.72, p = 0.085. While the main effect of cognitive load does seem to be approaching statistical significance, as before, the logically strongest test of the effect of cognitive load is the comparison of the single-task, no sound control condition with the dual-task 3-back condition, which, as before, showed no significant effect, F(1, 9.39) = 1.015, p = .339, as shown in . Likewise, the results replicated Experiment 1's lack of a significant interaction between retinal eccentricity and cognitive load on blur detection thresholds, F(5, 7.379) = .742, p = .615. Thus, the results are virtually identical to those found in Experiment 1, even though blur in the current experiment was presented simultaneously with the rest of the image, and therefore could not capture attention by virtue of having an onset separate from the rest of the image.
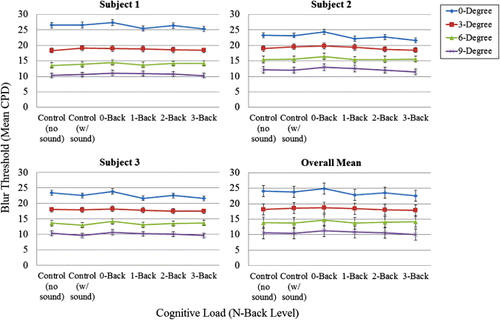
Discussion
Our results were consistent with those of Experiment 1. Cognitive load had no effect on blur detection thresholds, although our participants showed evidence of cognitive load effects as a function of N in the N-back task, with participants’ sensitivity in the N-back task decreasing as N increased. Again, we found that our measure of blur thresholds was sensitive and that the change from gaze-contingent to tachistoscopic presentation had no effect on those thresholds. As before, our blur threshold estimates decreased with eccentricity, and differed between individuals as one would expect.
Together, the results of Experiments 1 and 2 have ruled out plausible explanations for the lack of effect of cognitive load on blur detection, including having an insensitive measure of blur thresholds, and attentional capture by blur onset due to gaze-contingent presentation.
GENERAL DISCUSSION
The results of Experiments 1 and 2 showed that the cognitive load created by up to a 3-back (letter) task had no effect on blur detection thresholds. This suggests that either blur detection is unaffected by cognitive load, or that blur detection has a very high threshold for being affected by cognitive load, such that a 3-back task has no effect on it. This is in contrast to the fact that the same N-back levels caused decrements in recognition memory and fixation dispersion in Experiment 1. It also contrasts with the fact that while a growing number of studies have used the N-back task to induce cognitive load, as in the current study, none that we could find has used an N-back level greater than 3-back. For example, Gevins and Smith (Citation2003) used an automated cognitive load index based on a multivariate analysis of participants’ electroencephalogram theta and alpha bands. The authors found that the 3-back task was as cognitively demanding as the highest load level of the Multi-Attribute Task Battery (MATB; Comstock & Arnegard, Citation1992), which is used to simulate the cognitive load of air traffic control work, but was less cognitively demanding than taking a computerized version of the Graduate Management Admission Test (GMAT). Other research has shown that the 2-back task causes decreased performance in the anti-saccade task, which puts demands on the executive function of inhibiting pre-potent responses, in this case eye movements (Mitchell, Macrae, & Gilchrist, Citation2002). Another recent study showed that the 2-back task caused significant cognitive load in simulated driving, as measured by decreased gaze dispersion (Reimer et al., Citation2012). Thus, the fact that the 3-back task had no effect on blur detection relative to single-task blur detection is informative in-and-of-itself.
Nevertheless, to further investigate this issue, we calculated simple accuracy scores for the N-back data from both experiments, rather than our previously reported unbiased measure of sensitivity, d’. These calculations showed that although accuracy significantly decreased from 0-back to 3-back, even in the 3-back task, the mean accuracy across participants remained slightly above 90% in both experiments. This raised the possibility that the 3-back task may not have been difficult enough to affect our participants’ blur detection performance. We therefore carried out a follow-up experiment using an adaptive threshold estimation procedure to determine each individual's N-back level that would produce 75% accuracy (midway between chance, at 50%, and perfect, at 100%). However, our participants could not maintain consistent N-back performance at that level because it was too difficult. When we raised the N-back accuracy threshold to 82.5% accuracy (midway between 75% and 90%), the N-back threshold levels for three participants were 2-back, 3-back, and 4-back (i.e., on average 3-back). This is consistent with the fact that fMRI studies of N-back effects on brain activity have avoided N above 3-back, because “some authors have questioned the validity of results when the ability to successfully perform the task decreases” (Owen et al., Citation2005, p. 47). Thus, it seems that, consistent with prior research, our use of the 3-back as an upper limit for cognitive load was entirely reasonable.
Finally, one might argue that although the 3-back is a reasonable cognitive load, the specific type of cognitive load caused by the N-back task simply does not interfere with visual processing per se. However, the fixation dispersion and scene recognition memory results from Experiment 1 showed that the N-back task caused a significant impairment of visual attention and visual processing. The fixation dispersion data showed that the higher N-back levels caused a significant narrowing of viewers’ overt attentional breadth. Likewise, the picture recognition memory showed that at greater N-back levels, less visual information was encoded into long-term memory. Finally, in a separate study (Ringer et al., Citationin press), we have used a very similar design, but a visual task arguably more attentionally demanding than blur detection – Gabor orientation discrimination. In that experiment, participants had to discriminate whether the orientation of Gabor patches, presented gaze-contingently at either 5° or 10° eccentricity on pseudo-randomly selected fixations, differed from vertical. In a dual task condition, they also had to simultaneously carry out an auditory 2-back task. The results showed a significant decrease in performance in the dual-task condition relative to the single-task condition (Ringer et al., Citationin press). Thus, the auditory N-back task causes measurable decrements in both visual attentional breadth and visual task performance.
Given all of the above, we are therefore left to conclude that blur detection occurs without attention, namely pre-attentively. But how can we square this idea with the clear findings that attention increases peripheral resolution? A possible explanation depends on distinguishing between variability of resolution in the visual system versus within images. Carrasco and colleagues have shown that peripheral visual resolution is increased by attention. Those studies used low contrast stimuli that could be resolved better by increasing attention to them. The same is true with accommodation and blurred retinal images. After the visual system has detected retinal blur (the effective accommodative error), by changing the shape of the lens, the visual system can change blurred images into focused retinal images. That is not true, however, with blurred low-pass filtered images. If a viewer pre-attentively detects image blur due to low-pass filtering in their visual periphery, neither accommodation nor the allocation of covert attention will increase the clarity of the peripheral image, because the blur resides in the stimulus, not in the viewer's visual system. Interestingly, in such cases, the response of the visual system is often to target another location than the blurred stimulus (Enns & Di Lollo, 1997; Loschky & McConkie, Citation2002; Nystrom & Holmqvist, Citation2007; Smith & Tadmor, Citation2012), even though foveating a peripherally blurred stimulus would normally serve to improve visual resolution for that stimulus. Such excessively blurred stimuli may have reduced saliency. Thus, perhaps blur is not consciously registered unless it has been (1) pre-attentively detected, and (2) covertly attended to and/or accommodated to, and (3) neither attending nor accommodating has increased the resolution of the peripheral image. This lack of responsiveness of the blurred imagery to covert attention and/or accommodation would serve as an error signal triggering conscious awareness of the blur, leading to more determined efforts to clarify the image (e.g., rubbing ones’ eyes, wiping one's glasses, etc.).
The current study therefore contributes to our growing understanding of blur as a perceptual phenomenon, and its relationship to visual attention. Our study suggests that, together with other simple visual features such as colour, orientation, size, and the direction of illumination, visual blur may be detected pre-attentively. If so, this finding provides an important theoretical link between visual blur and attention in both normal and impaired visual functions.
Notes
1 Interestingly, if the task is to detect image regions differing in resolution from the rest of the image, blurred regions are fixated faster than clear regions (Enns & MacDonald, Citation2012).
2 The BCE, traditionally used in clinical ophthalmology to describe fixation stability, generates an ellipse that encompasses 68% (± 1 SD) of fixation locations.
REFERENCES
- Bernard, J.-B., Scherlen, A-C., & Castet, E. (2007). Page mode reading with simulated scotomas: A modest effect of interline spacing on reading speed. Vision Research, 47(28), 3447–3459. doi:10.1016/j.visres.2007.10.005
- Carrasco, M., Ling, S., & Read, S. (2004). Attention alters appearance. Nature Neuroscience, 7(3), 308–313. doi:10.1038/nn1194
- Carrasco, M., Williams, P. E., & Yeshurun, Y. (2002). Covert attention increases spatial resolution with or without masks: Support for signal enhancement. Journal of Vision, 2(6), 467–479. doi:10.1167/2.6.4
- Chen, Y.-N., Mitra, S., & Schlaghecken, F. (2008). Sub-processes of working memory in the N-back task: An investigation using ERPs. Clinical Neurophysiology, 119(7), 1546–1559. doi:10.1016/j.clinph.2008.03.003
- Ciuffreda, K. J., Wang, B., & Vasudevan, B. (2007). Depth-of-focus: Control system implications. Computers in Biology and Medicine, 37(7), 919–923. doi:10.1016/j.compbiomed.2006.06.012
- Ciuffreda, K. J., Wang, B., & Wong, D. (2005). Central and near peripheral retinal contributions to the depth-of-focus using naturalistic stimulation. Vision Research, 45(20), 2650–2658. doi:10.1016/j.visres.2005.02.023
- Cohen, J. D., Perlstein, W. M., Braver, T. S., Nystrom, L. E., Noll, D. C., Jonides, J., & Smith, E. E. (1997). Temporal dynamics of brain activation during a working memory task. Nature, 386(6625), 604–608. doi:10.1038/386604a0
- Comstock, J. R., & Arnegard, R. J. (1992). The multi-attribute task battery for human operator workload and strategic behavior research. 104174: NASA Technical Memorandum.
- Crossland, M. D., & Rubin, G. S. (2002). The use of an infrared eyetracker to measure fixation stability. Optometry & Vision Science, 79(11), 735–739. doi:10.1097/00006324-200211000-00011
- Crundall, D. E., Underwood, G., & Chapman, P. R. (2002). Attending to the peripheral world while driving. Applied Cognitive Psychology, 16(4), 459–475. doi:10.1002/acp.806
- Duchowski, A. T., Cournia, N., & Murphy, H. (2004). Gaze-contingent displays: A review. CyberPsychology & Behavior, 7(6), 621–634. doi:10.1089/cpb.2004.7.621
- Enns, J. T., & MacDonald, S. C. (2012). The role of clarity and blur in guiding visual attention in photographs. Journal of Experimental Psychology: Human Perception and Performance. Advance online publication Sept 17. doi:10.1037/a0029877
- Gevins, A., & Smith, M. E. (2003). Neurophysiological measures of cognitive workload during humaN-computer interaction. Theoretical Issues in Ergonomics Science, 4(1–2), 113–131. doi:10.1080/14639220210159717
- Gonzalez, R. C., Woods, R. E., & Eddins, S. L. (2009). Digital image processing using MATLAB (Vol. 2): Tennessee: Gatesmark Publishing
- Henderson, J. M., & Hollingworth, A. (1998). Eye movements during scene viewing: An overview. In G. Underwood (Ed.), Eye guidance in reading and scene perception (Vol. xi, pp. 269–293). Oxford: Elsevier Science Ltd.
- Henderson, J. M., & Hollingworth, A. (1999). High-level scene perception. Annual Review of Psychology, 50, 243–271. doi:10.1146/annurev.psych.50.1.243
- Jaeggi, S. M., Buschkuehl, M., Perrig, W. J., & Meier, B. (2010). The concurrent validity of the N-back task as a working memory measure. Memory, 18(4), 394–412. doi:10.1080/09658211003702171
- Just, M. A., & Carpenter, P. A. (1980). A theory of reading: From eye fixations to comprehension. Psychological Review, 87(4), 329–354. doi:10.1037/0033-295X.87.4.329
- Kaernbach, C. (1990). A single-interval adjustment-matrix (SIAM) procedure for unbiased adaptive testing. Journal of the Acoustical Society of America, 88(6), 2645–2655. doi:10.1121/1.399985
- Kane, M. J., Conway, A. R., Miura, T. K., & Colflesh, G. J. (2007). Working memory, attention control, and the N-back task: A question of construct validity. Journal of Experimental Psychology: Learning, Memory, and Cognition, 33(3), 615–622. doi:10.1037/0278-7393.33.3.615
- Kenward, M. G., & Roger, J. H. (1997). Small sample inference for fixed effects from restricted maximum likelihood. Biometrics, 983–997. doi:10.2307/2533558
- Kirchner, W. K. (1958). Age differences in short-term retention of rapidly changing information. Journal of Experimental Psychology, 55(4), 352–358. doi:10.1037/h0043688
- Lee, J., & Maunsell, J. H. R. (2010). Attentional modulation of MT neurons with single or multiple stimuli in their receptive fields. Journal of Meuroscience: The Official Journal of the Society for Neuroscience, 30, 3058–3066. doi:10.1523/JNEUROSCI.3766-09.2010
- Leukart, R. H. (1939). The speed of monocular accommodation. Journal of Experimental Psychology, 25(3), 257–270. doi:10.1037/h0055038
- Lockhart, T. E., & Shi, W. (2010). Effects of age on dynamic accommodation. Ergonomics, 53(7), 892–903. doi:10.1080/00140139.2010.489968
- Loschky, L. C., & McConkie, G. W. (2002). Investigating spatial vision and dynamic attentional selection using a gaze-contingent multi-resolutional display. Journal of Experimental Psychology: Applied, 8(2), 99–117. doi:10.1037/1076-898X.8.2.99
- Loschky, L. C., McConkie, G. W., Yang, J., & Miller, M. E. (2005). The limits of visual resolution in natural scene viewing. Visual Cognition, 12(6), 1057–1092. doi:10.1080/13506280444000652
- Loschky, L. C., & Wolverton, G. S. (2007). How late can you update Gaze-contingent Multiresolutioanl Displays without detection? Transactions on Multimedia Computing, Communications, and Applications, 3(4), 1–10 doi:10.1145/1314303.1314310
- Matsukura, M., Brockmole, J. R., Boot, W. R., & Henderson, J. M. (2011). Oculomotor capture during real-world scene viewing depends on cognitive load. Vision Research, 51(6), 546–552. doi:10.1016/j.visres.2011.01.014
- McAdams, C. J., & Maunsell, J. H. R. (1999). Effects of attention on orientatioN-tuning functions of single neurons in Macaque cortical area V4. Journal of Neuroscience, 19, 431–441.
- McConkie, G. W., & Rayner, K. (1975). The span of the effective stimulus during a fixation in reading. Perception & Psychophysics, 17(6), 578–586. doi:10.3758/BF03203972
- Mitchell, J. P., Macrae, C. N., & Gilchrist, I. D. (2002). Working memory and the suppression of reflexive saccades. Journal of Cognitive Neuroscience, 14(1), 95–103. doi:10.1162/089892902317205357
- Miura, T. (1986). Coping with situational demands: A study of eye movements and peripheral vision performance. In Vision in Vehicles (pp. 205–221). North-Holland: Elsevier Science Publishers B.V.
- Moran, J., & Desimone, R. (1985). Selective attention gates visual processing in the extrastriate cortex. Science (New York, N.Y.), 229, 782–784. doi:10.1126/science.4023713
- Murray, S., & Bex, P. J. (2010). Perceived blur in naturally contoured images depends on phase. Frontiers in Psychology, 1, 185. doi:10.3389/fpsyg.2010.00185
- Nuthmann, A., Smith, T. J., Engbert, R., & Henderson, J. M. (2010). CRISP: A computational model of fixation durations in scene viewing. Psychological Review, 117(2), 382–405. doi:10.1037/a0018924
- Nystrom, M., & Holmqvist, K. (2007). Variable resolution images and their effects on eye movements during free viewing. San Jose, CA: SPIE.
- Olmsted, J. (1944). The role of the autonomic nervous system in accommodation for far and near vision. Journal of Nervous and Mental Disease, 99(5), 794–798. doi:10.1097/00005053-194405000-00037
- Owen, A. M., McMillan, K. M., Laird, A. R., & Bullmore, E. (2005). N-back working memory paradigm: A meta-analysis of normative functional neuroimaging studies. Human Brain Mapping, 25(1), 46–59. doi:10.1002/hbm.20131
- Parkhurst, D., Culurciello, E., & Neibur, E. (2000). Evaluating variable resolution displays with visual search: Task performance and eye movements. In A. T. Duchowski (Ed.), Proceedings of the Eye Tracking Research & Applications Symposium 2000 (pp. 105–109). Palm Beach, FL: ACM.
- Pestilli, F., & Carrasco, M. (2005). Attention enhances contrast sensitivity at cued and impairs it at uncued locations. Vision Research, 45(14), 1867–1875. doi:10.1016/j.visres.2005.01.019
- Pomplun, M., Reingold, E. M., & Shen, J. (2001). Investigating the visual span in comparative search: The effects of task difficulty and divided attention. Cognition, 81(2), B57–B67. doi:10.1016/S0010-0277(01)00123-8
- Ramachandran, V. S. (1988). Perception of shape from shading. Nature (331), 163–166. doi:10.1038/331163a0
- Rayner, K. (1975). The perceptual span and peripheral cues in reading. Cognitive Psychology, 7(1), 65–81. doi:10.1016/0010-0285(75)90005-5
- Rayner, K. (1998). Eye movements in reading and information processing: 20 years of research. Psychological Bulletin, 124, 372–422. doi:10.1037/0033-2909.124.3.372
- Recarte, M. A., & Nunes, L. M. (2003). Mental workload while driving: Effects on visual search, discrimination, and decision making. Journal of Experimental Psychology-Applied, 9(2), 119–137. doi:10.1037/1076-898X.9.2.119
- Reimer, B., Mehler, B., Wang, Y., & Coughlin, J. F. (2012). A field study on the impact of variations in short-term memory demands on drivers’ visual attention and driving performance across three age groups. Human Factors: Journal of the Human Factors and Ergonomics Society, 54(3), 454–468. doi:10.1177/0018720812437274
- Reingold, E. M., & Loschky, L. C. (2002). Saliency of peripheral targets in gaze-contingent multiresolutional displays. Behavior Research Methods, Instruments and Computers, 34(4), 491–499. doi:10.3758/BF03195478
- Reingold, E. M., Loschky, L. C., McConkie, G. W., & Stampe, D. M. (2003). Gaze-contingent multi-resolutional displays: An integrative review. [review]. Human Factors, 45(2), 307–328. doi:10.1518/hfes.45.2.307.27235
- Reynolds, J. H., Pasternak, T., & Desimone, R. (2000). Attention increases sensitivity of V4 neurons. Neuron, 26, 703–714. doi:10.1016/S0896-6273(00)81206-4
- Ringer, R. V., Johnson, A. P., Gaspar, J., Neider, M., Crowell, J., Kramer, A. F., & Loschky, L. C. (in press). Creating a new dynamic measure of the useful field of view. In J. Mulligan (Ed.), Proceedings of the 2014 Symposium on Eye Tracking Research and Applications: ACM.
- Sawides, L., de Gracia, P., Dorronsoro, C., Webster, M. A., & Marcos, S. (2011). Vision is adapted to the natural level of blur present in the retinal image. PLoS ONE, 6(11), e27031. doi:10.1371/journal.pone.0027031
- Sere, B., Marendaz, C., & Herault, J. (2000). Nonhomogeneous resolution of images of natural scenes. Perception, 29(12), 1403–1412. doi:10.1068/p2991
- Shioiri, S., & Ikeda, M. (1989). Useful resolution for picture perception as a function of eccentricity. Perception, 18, 347–361. doi:10.1068/p180347
- Shors, T. J., Wright, K., & Greene, E. (1992). Control of interocular suppression as a function of differential image blur. Vision Research, 32(6), 1169–1175. doi:10.1016/0042-6989(92)90019-F
- Smith, W. S., & Tadmor, Y. (2012). Nonblurred regions show priority for gaze direction over spatial blur. The Quarterly Journal of Experimental Psychology( ahead-of-print), 1–19.
- Treisman, A., & Gormican, S. (1988). Feature analysis in early vision: Evidence from search asymmetries. Psychological Review, 95(1), 15–48. doi:10.1037/0033-295X.95.1.15
- Treue, S. (2004). Perceptual enhancement of contrast by attention. Trends in Cognitive Sciences, 8(10), 435–437. doi:10.1016/j.tics.2004.08.001
- Treue, S., & Maunsell, J. H. R. (1996). Attentional modulation of visual motion processing in cortical areas MT and MST. Nature, 382, 539–541. doi:10.1038/382539a0
- van Diepen, P. M., & d'Ydewalle, G. (2003). Early peripheral and foveal processing in fixations during scene perception. Visual Cognition, 10(1), 79–100. doi:10.1080/713756668
- Wickens, C. D. (2002). Multiple resources and performance prediction. Theoretical Issues in Ergonomics Science, 3(2), 159–177. doi:10.1080/14639220210123806
- Williford, T., & Maunsell, J. H. R. (2006). Effects of spatial attention on contrast response functions in macaque area V4. Journal of Neurophysiology, 96, 40–54. doi:10.1152/jn.01207.2005
- Wolfe, J. M., & Horowitz, T. S. (2004). What attributes guide the deployment of visual attention and how do they do it? Nature Reviews Neuroscience, 5(6), 495–501. doi:10.1038/nrn1411
- Xiao, J., Hays, J., Ehinger, K. A., Oliva, A., & Torralba, A. (2010). Sun database: Large-scale scene recognition from abbey to zoo. Paper presented at the Computer vision and pattern recognition (CVPR), 2010 IEEE conference on.
- Yeshurun, Y., & Carrasco, M. (1998). Attention improves or impairs visual performance by enhancing spatial resolution. Nature, 396(6706), 72–75. doi:10.1038/23936
- Yeshurun, Y., & Carrasco, M. (1999). Spatial attention improves performance in spatial resolution tasks. Vision Research, 39(2), 293–306. doi:10.1016/S0042-6989(98)00114-X