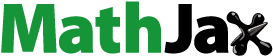
ABSTRACT
In everyday group conversations, we must decide whom to pay attention to and when. This process of dynamic social attention is important for goals both perceptual and social. The present study investigated gaze during a conversation in a realistic group and in a controlled laboratory study where third-party observers watched videos of the same group. In both contexts, we explore how gaze allocation is related to turn-taking in speech. Experimental video clips were edited to either remove the sound, freeze the video, or transition to a blank screen, allowing us to determine how shifts in attention between speakers depend on visual or auditory cues. Gaze behaviour in the real, interactive situation was similar to the fixations made by observers watching a video. Eyetracked participants often fixated the person speaking and shifted gaze in response to changes in speaker, even when sound was removed or the video freeze-framed. These findings suggest we sometimes fixate the location of speakers even when no additional visual information can be gained. Our novel approach offers both a comparison of interactive and third-party viewing and the opportunity for controlled experimental manipulations. This delivers a rich understanding of gaze behaviour and multimodal attention during a conversation following.
Introduction
In a world that is full of complex social scenes, the ability for us to selectively attend to targets of the most important is a rapid, fluid, and sophisticated process. A considerable amount of research has helped us determine the systems involved in selectively attending to social elements in static images, but less is understood about the processes involved in observing dynamic situations, in particular within complex social settings. A relevant observation when thinking about our own everyday interactions, and one that is easily replicated in the lab, is that we should attend to someone who is speaking. We look to a speaker not only to aid language comprehension but also as a signal to show we are listening. From childhood, we are often told, “look at me when I am speaking to you” and having our eyes on the teacher indicates that we are listening.
To converse efficiently, an exquisitely attuned, adaptive, and coordinated system is required to process the dynamic information present (Penn, Citation2000). In the current study, we investigate such dynamic processing during participation in live group conversations and compare this with third-party observations of those conversations. In particular, we examine gaze behaviour as a way to investigate the perception of social cues which allow people to follow a conversation. First, we introduce what is known about social gaze in the lab and in the real world.
Social gaze to conversation in video
A number of recent studies have investigated gaze using pre-recorded clips of turn-taking in conversation (Foulsham et al., Citation2010; Foulsham & Sanderson, Citation2013; Tice & Henetz, Citation2011). These studies have begun to explore how the behaviour of the actors within the clips affects the gaze of third-party observers. For example, Hirvenkari et al. (Citation2013) explored how the natural signalling displayed during turn-taking transitions affects conversation following when clips of such conversation are watched at a later stage. Their study involved asking participants to observe a pre-recorded conversation between a dyad pair, with no further instructions. The clips shown to participants included the manipulation of audio (silent) and visual (freeze-framed) clip conditions to explore which modalities evoked gaze shifting.
The results demonstrated that overall participants looked at the speaker on average 74% of the time. The authors argue that this percentage closely resembles that in a real-world dyad conversation, highlighting an observation by Argyle and Ingham (Citation1972), where it was reported that a live listener looks at a speaker similarly 75% of the time. Equally, Hirvenkari et al. (Citation2013) found that changes in the speaker directed the gaze behaviour of the third-party observers. These results indicate that the organization of turn-taking conversation has a strong influence on third-party gaze behaviour, rather than, for example, observers looking at speakers and listeners equally. This is not too surprising given the evidence that we typically attend to the location of a sound source in various contexts. In a social situation, there is also evidence to suggest that being able to view a speaker’s face helps with perceptual ambiguity and conversation following. For example, Zion-Golumbic et al. (Citation2013) explored the “Cocktail Party” problem whereby participants viewed conversational videos simultaneously with multiple sources of sound. Their results indicated that being able to view the speaker’s face enhanced the capacity for the auditory cortex to track the temporal speech envelope of that speaker.
However, it is not clear at which point observers move their gaze to a speaker during group interactions. Whether observers move their gaze in advance of a change in speaker or whether this gaze shift is reactive can be investigated by exploring the cues in conversation which lead people to shift their gaze. In the present study, we investigate the precise timing pattern and the presence of these cues, such as signals in speech or gestures and other physical behaviours. In order to understand these cues and their impact on gaze, we can manipulate the audio and visual content of the conversation.
For example, Hirvenkari et al. (Citation2013) found when looking at the temporal characteristics of gaze shifts, at the crucial turn-taking transition, gaze predicted rather than followed speakership. Upon a turn-taking transfer, the attentional shift to the speaker was slightly before the beginning of the utterance, and, although alone both modalities evoked a shift, the anticipatory shift was most apparent when both audio and visual modalities were present.
A further example is Latif et al.’s (Citation2018) study which investigated the role of auditory and visual cues on predicting turn-taking behaviour. Their study involved presenting participants with clips of a dyad pair engaged in a natural conversation. Participants were asked to watch the clips and respond with a button press when they felt the speaker was about to finish their turn of talking. The authors manipulated the stimuli by preparing trials where the audio or visual information was removed, giving three modality conditions: Visual-Only, Auditory-Only, and Control. Decisions of turn-taking behaviour were assessed with the strongest performance when both audio and visual information was present in the Control condition. Participants responded significantly earlier in the Visual-Only condition in comparison to both auditory inclusive conditions. The authors deduce that visual information functions as an early signal indicating an upcoming exchange; whilst the auditory counterpart is used as information for individuals to precisely time a response to turn ends. This suggests that visual information might be critical for guiding gaze in advance of the next speaker, although anticipation was observed regardless of the modality presented.
These studies, which demonstrate the ability to predict speakership, involve dyad pairs. There is also evidence for anticipation in larger groups (e.g., Holler & Kendrick, Citation2015), with gaze moving before a change in speaker. However, the evidence, in this case, is more mixed. For example, a similar result to the aforementioned studies, demonstrating that gaze in third-party participants can predict speakership in a group setting, was reported by Foulsham et al. (Citation2010). When analysing the temporal offset in the relationship between speaking and fixation, they found that participants tended to look to the speaker slightly (roughly 150 ms) before the utterance beginning.
The temporal characteristics of following conversation were further investigated by Foulsham and Sanderson (Citation2013). In this case, their study used a similar video-watching task and did not find that gaze moved in advance of the change in speaker. Instead, the authors reported that speaking preceded gaze by roughly 800 ms, with the authors suggesting this may be due to the complexity of the video interactions. The authors included the manipulation of removing the sound in order to investigate whether auditory information would affect when speakers were fixated, how fixations between different observers were synchronized and the number of fixations on the eyes and mouth. Differences between regions of the face were investigated in the expectation that removing the sound might increase looks to the mouth if participants were trying to decode speech from lip movements alone. The results demonstrated that removing sound led to decreased attention towards the current speaker, and instead, more time was spent looking at the other non-speaking targets. There were increases in looks to the mouth upon removing the sound; however, the eyes continued to attract most of the fixations. Despite these changes in gaze behaviour, the participants still appeared to follow the conversation without audio. Hence, as participant’s gaze patterns continued to follow the turn-taking conversation without any auditory information, it appears the participants were using a visual cue to guide their gaze to the speakers, and that this strategy may have helped follow the depicted interaction. If this is indeed the case, then a condition where the sound continues but visual information is removed should produce a very different pattern of gaze.
In the present study, we investigate the effect of visual and auditory modulations on the precise timing patterns of gaze to a speaker. While the role of visual information in the intelligibility of speech has often been studied, it is less clear how visual versus auditory cues are involved in the precise timing of gaze patterns. We thus include these manipulations to help understand the factors underlying how attention is deployed during complex social interactions.
In sum, it appears that the gaze of participants watching clips of conversation at a later stage is influenced by the behaviour of the targets during their conversation. The precise time and which cue guides this shift is debated, and we here explore this further. In the next section, we discuss gaze timing in real, face-to-face interaction.
Social gaze during conversation in live settings
Gaze timing during conversation has also been investigated in real interactions. Ho et al. (Citation2015), explored the precise timing of gaze during a live face-to-face conversation. This study provides evidence that an anticipatory effect occurs in a live conversation setting, with a lag between changes in gaze and changes in speaker (roughly 400 ms) similar to at least one study which used pre-recorded video (Foulsham et al., Citation2010). Ho et al. (Citation2015) monitored dyad pairs engaging in two turn-taking games while both participants’ eye movements were tracked. The authors assessed the temporal characteristics in terms of both gaze and participant-generated speech. This analysis enabled a detailed measurement of how speakers and listeners avert and direct their gaze. Interestingly, because this study looked at real people in a face-to-face situation rather than someone watching a video clip, the results may reflect the dual function of social gaze (Risko et al., Citation2016). In that, in a live environment, the eyes not only take in information, but they also signal to others. In other words, the gaze movements involved in live studies such as Ho et al. (Citation2015) were not merely picking up on the information from the speaker but also sending a signal about listener engagement and turn-taking. This signalling can take place in real face to face interactions, for example, when it is your time to speak; but not when looking at pictures of faces which are often used in classic social attention studies (Risko et al., Citation2012). Considering the discrepancies about timing in the video studies described above, it is interesting to compare this behaviour between real and video conditions. If there are large differences between comparable “lab” and real interactions, then it would suggest that gaze to conversations is strongly affected by the ability to interact in the real situation. Arguably, these settings might show the same anticipatory effect for a different reason, in that the signalling cues that were exhibited in the live situation (which allowed for a dual function of gaze), may guide attention in the pre-recorded videos, hence allowing participants to pre-empt the speaker. In the next section, we discuss how social attention manifests differently in studies which compare the “lab” versus real interactive settings.
Third-party versus live interaction
The present study uses a combination of pre-recorded conversations shown to participants and a live situation to help us to understand how visual attention is distributed during social interactions. We include this comparison as the extent to which findings from studies with pictures and video can be generalised to real life is debated (see Risko et al., Citation2012, for a comprehensive review). Studies that have explored to what degree a lab scenario reflects a “real” situation has uncovered distinct differences in social behaviour. For example, Hayward et al. (Citation2017) demonstrate differences in social attention engagement between a real-world task and a more typical lab-based social cueing task. This is further echoed in work by Foulsham and Kingstone (Citation2017), who demonstrated a fairly poor relationship between real-world gaze behaviour and fixations on static images of the same environment. Risko et al. (Citation2012) explain how we should exercise caution when drawing conclusions solely from findings using static stimuli in a controlled experiment. Risko et al. (Citation2016), advocate “Breaking the Fourth Wall” within social attention research, to enable a method which is more representative of a real interaction. Their paper argues that social attention research has often failed to recognize that in a real-world scenario, the person or agent within the scene can interact with the participant, while a pre-recorded video or image cannot. This interactive element will clearly have dramatic effects on participant behaviour.
An instructive example is given by Laidlaw et al. (Citation2011), who explored to what extent participants look at a person if they are in the room or are presented on a video camera. Participants were more inclined to look at a person on a video than in real life, demonstrating the effect of real social presence on visual attention. Foulsham et al. (Citation2011) found that when comparing the eye movements of people walking through a campus with participants watching those clips at a later stage, although there were similarities, the live presence of other people did affect gaze. For example, pedestrians who were close to the observer were looked at more by observers watching the event on video than by people in the real world. Social norms may play a role in these discrepancies, but the differences can also be explained in terms of real versus implied social presence and the previously discussed dual purpose of the eyes. Any differences, which could be explained by the signalling of the eyes in a live situation, will be investigated here by comparing between a live interaction and responses to pre-recorded video.
Research questions
The present study investigates the signalling cues utilized in visual attentional shifts during turn-taking conversation, whilst offering a unique method to compare a live scenario with people watching a recording. To our knowledge, this study is unique in allowing the comparison of live and third-party group gaze behaviours. We have three main research questions.
Does third-party viewing reflect live gaze behaviour?
First, we explore how visual attention differs in the lab and in a natural conversation using methods that will allow for a comparison that has not previously been available. We do this by recording video stimuli during a naturalistic interaction. In line with previous research (such as by Freeth et al., Citation2013 and Laidlaw et al., Citation2011) we may find less looks to speakers in the live situation than in the video observations due to social avoidance. Alternatively, if the reason we look to a speaker is to signal to others that we are listening, it could be argued that looks to speakers may increase in a live situation compared to a video; something which would be redundant in a third-party setting. If we see similar results in both situations, this would indicate that we do not look at a speaker just to signal, instead, perhaps this is a habit or aids comprehension in some way. Comparing the interlocutors’ visual attention with that of third-party observers watching the same conversation on video will also help establish the ecological validity of understanding social attention via pre-recorded videos, which will add confidence that our additional research questions are relevant for real interactions. To assess this, we will examine the degree of looking to current speakers, other targets and elsewhere in both a live interaction and when watching a video, and we will additionally evaluate the “agreement” between looking behaviour in each setting over time.
How do audiovisual cues affect conversation following?
Second, we test the signalling cues which attract our visual attention to a speaker during videos of group interactions. Previous studies have examined the impact of removing the sound (Foulsham & Sanderson, Citation2013). We will additionally manipulate the visual information available to the participant by freeze-framing the image or transitioning to a blank screen while the audio continues. These conditions will be compared to a control clip where both audio and visual information is available. The research by Hirvenkari et al. (Citation2013) suggests that participants will continue to look at the image of the person speaking, even in the freeze-frame condition where no information from their movements is available. This might occur because observers have built an association between an individual voice and that person’s face, although whether this has benefits for comprehension remains to be established. Participants might even be linking the voice to a spatial location on the screen. Therefore, this study will also explore the association between audio and spatial location with a blank screen condition inspired by past work on “looking at nothing” (Richardson et al., Citation2009). This line of research explores how participants have a tendency to look to the blank space where stimuli were previously presented when later hearing information relating to those stimuli. Altmann (Citation2004) proposes that this is due to a “spatial index” which is part of the memory representation of the object.
As people look to social elements of a scene, we hypothesize that the bias to fixate the person speaking may be reduced but still present in the freeze-frame condition. Participants may not show a pattern of following the speaker in either of the visual manipulation conditions, as no additional visual information can be gained. However, if participants adopt a “looking at nothing” approach a pattern of conversation following may remain. This will help us to uncover how the auditory component of conversation allows for conversation following and whether observation of the targets (moving, frozen, or not at all) is crucial for this. We will investigate the effect of the sound being removed, the image freezing or the image being completely removed on looks to people, their features (eyes and mouth), and in particular the time spent looking at the current speaker.
When are speakers looked at?
Third, this study aims to investigate the precise timing of looks to a speaker. There are some inconsistencies in previously discussed findings regarding this time course, with most studies showing that participants’ gaze anticipates changes in the conversation turn but others observing that there is a lag between the utterance beginning and fixation on the speaker. Often, the research which demonstrates an anticipatory effect involves stimuli depicting just two individuals. This might facilitate conversation following in that participants can easily distinguish who will be the next speaker. The evidence for third-party anticipation in larger groups is limited and less consistent. However, we expect that participants will continue to shift their gaze to the speaker prior to the utterance beginning. If the anticipatory effect is equal in all conditions, this would suggest that participants use visual and auditory elements equally to guide their attention to speakers. However, if participants rely on auditory cues, we would expect the anticipatory effect to be diminished in the silent condition. Equally, if participants rely on visual elements (e.g., head and eye movements of the depicted people) to induce an early gaze shift, we would expect there to be no anticipatory effect in the freeze-frame and blank condition. To explore this, we will analyse at which point participants make a fixation to current speakers upon that utterance beginning.
In addition to our three research questions, we aim to test these aspects of social attention in a more complex environment than the one in which they have previously been studied. The research conducted to date is often scripted with dyad pairs, and fewer studies have considered larger group interactions, even though these are common in everyday life. The present study uses naturally formed groups of six individuals, all of whom were members of sports teams at the University of Essex, seated around a table to enable a fluid discussion. Adding additional people to the group and the use of free-flowing conversation comes with increased complications for analysis, but also increased visual attention decisions which need to be made by the observer, providing us with rich multimodal data. With multiple targets and multiple turn-taking transitions, we might expect more variation in observer gaze and perhaps less attuned timing patterns of conversation following. In a dyad pair paradigm, often used in third-party eye tracking studies which include audiovisual modulations, the decision for the interlocutors involved is only whether to direct or avert one’s gaze. In a larger group, one must decide who to look at and when (for example distributing attention between the speaker and people who are listening). Although we still expect a speaker will dominate fixations in both the live and third-party participants, with a larger group gaze may be more distributed than previously reported. In addition, using a larger group setting will add supporting or contradictory evidence of predicting utterance starts within a complex social environment.
Materials and methods
The aims and analysis of this study were pre-registered (see https://osf.io/m2dp5/).
Participants
We here analyse data from small groups interacting in real life, as well as from participants later watching video recordings of these groups. The individuals recorded in the real interaction are hereafter referred to as the “targets” and the third-party participants referred to as “participants.”
The targets were drawn from four groups of six individuals comprised of various sports teams at the University of Essex. There were two groups of males and two groups of females. An initial request to take part was sent to the Presidents of the sports clubs. A full description of this interaction and the target recordings is provided in the next section. For analysis of behaviour from the group interaction, and for creating stimuli, we relied on data from one half of the table. There were, therefore, 12 targets in these clips. This sample size was predetermined based on the required stimuli for the second part of the study.
The third-party, eyetracked participants were 40 volunteers (7 male and 33 female), with a mean (standard deviation) age of 20.9 (2.9) years old. This sample size was pre-registered and with this within-subjects design gives excellent power for effects such as the one of sound on gaze in Foulsham and Sanderson (Citation2013; dz = 2.4). All participants were undergraduate students from the University of Essex, recruited for course credit. All participants had a normal or corrected-to-normal vision and gave their informed consent before taking part.
Target clip preparation
The video clips shown to third-party participants depicted six individuals having a discussion while sitting around a table, with only three individuals (one side of the table) in view in each clip. This is shown schematically in .
Figure 1 . Schematic view of target individuals (T1–T6) and video camera set up during stimuli creation.
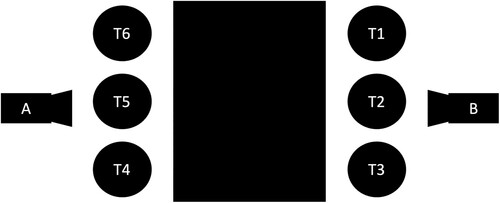
The clips were derived from a 1 hour recording with two static video cameras (with microphone) placed discretely, which are a permanent feature of the Observation Laboratory at the University of Essex. Each camera was adjusted so that only the view of one side of the table was present within the recording. The view from camera A was used to create the clips for the eye tracking study. The view from both cameras A and B were used to code behaviour of the targets in the live interaction. The discussion took place in a well-lit room. The targets were given several questions, in a randomized order, which they were to discuss as a group. The questions given to the targets were questions or topics designed to enable natural conversing from all team members. Examples include: “find out who has moved house the most”, “what are you most grateful for?” and “what is your most embarrassing moment?”. Two experimental clips were selected from each continuous recording and featured moments where all visible targets spoke at least once. These clips were selected to ensure that Targets 1, 2 and 3 were the predominant speakers, with minimal involvement from the targets on the other side of the table. Additionally, a “familiarity clip” was prepared for each target group. This clip also featured the visible targets, all speaking at least once. These clips were included to ensure that the third-party participant was familiar with each of the targets’ voices. The familiarity clips were not used in further analyses. Hence participants were shown a total of 3 clips per target group (12 clips total). Clips varied in length from 36–54 seconds.
The audiovisual information in the experimental clips was manipulated to produce a Control condition and three alternative conditions: Silent, Freeze Frame and Blank. For these conditions, a critical time when the manipulation began was chosen for each clip. This was roughly mid-way through the clip, but at a point when all three target faces were clearly visible (i.e., not covered by hands), and given the range of durations, the time of the manipulation was unpredictable for the participants. For the same reason, we did not count an exact number of turn exchanges prior to the manipulation and instead chose a point when there was fluid conversation. In the Silent condition, the audio was removed at this point while the video continued. In the Freeze Frame condition, the visual image was frozen, and in the Blank condition, all visual stimuli were removed, and a plain white screen was presented. In both the Freeze Frame and Blank conditions, the audio continued (see for a visualisation). The videos were re-encoded to a frame resolution of 1024 × 768 pixels and displayed at a rate of 25 frames per second.
Apparatus
An EyeLink 1000 eye tracker and ExperimentBuilder software were used to present the stimuli and record eye movements. Monocular eye position was recorded by the Eyelink 1000 system by tracking the pupil and the corneal reflection at 1000 Hz. A nine-point calibration and validation procedure ensured mean gaze-position errors of less than 0.5°. Saccades and fixations were defined according to Eyelink’s acceleration and velocity thresholds.
The video clips were presented on a 19 inch colour monitor. During all conditions, the sound was played through headphones which the participants were required to wear throughout the study. The participants’ head movements were restricted using a chinrest which kept the viewing distance constant at 60 cm. At this distance, the video frame subtended approximately 38° × 22° of visual angle.
Participant procedure
The participants read and completed consent forms and were asked to confirm that they had normal or corrected to normal vision before beginning the experiment. Participants then took part in a 9-point calibration. After the participant’s right eye had been successfully calibrated and validated, the experiment began.
There were four blocks of trials, with each block consisting of clips from one of the target groups in one of the four conditions. Participants watched three clips per block, with the single familiarity clip always preceding the (2) experimental clips. Participants only saw each clip once, but clips were counterbalanced across the conditions, such that over the whole experiment, each clip appeared in each condition equally often. This ensures that any peculiarities of the particular clip or the following question could not explain differences between conditions. Condition blocks were presented in a randomized order.
Participants were simply instructed to watch the scene and not given any further instructions about how to view the scene. Participants were informed that they would be asked a question after each clip based on what they had seen. After watching each clip, participants were given a simple comprehension question based on the conversation the targets were engaging in. The questions were in the style of “Which person said X”, with participants responding to the questions by pressing “1”, “2”, or “3” on the keypad to indicate one of the three targets. Questions were based on each particular clip, but again these were randomly ordered and counterbalanced across conditions as described above. The questions were piloted for difficulty before beginning the study and only used as an attentional check to ensure participants were paying attention to the clips. After each clip, there was a drift check which ensured accurate tracking throughout. The overall testing session (of eye-movement collection) lasted approximately nine minutes.
Results
This study is unique in that it offers a comparison between the third-party viewing of a conversation (which we would expect from a typical eye tracking study) with gaze at the time of recording the stimuli in a live situation. We begin by making this comparison as a manipulation check, to first assess how visual attention in the live interaction compares with third-party viewing in the lab. Then we progress to the effects of audio and visual modalities on conversation following. Data and scripts for our analysis can be found at https://osf.io/m2dp5/.
Does third-party viewing reflect live gaze behaviour?
Behaviour in the live interaction
During the live interaction, all six targets in each of the groups were filmed during the interactions. Third-party viewers, when watching the manipulated clips, only saw one side of the table (Targets 1, 2, and 3 as seen in ). However, the fact that the other side of the table was also filmed during data collection enables us to analyse the live viewing behaviour of the three targets not present in the stimuli (Targets 4, 5, and 6). This gives us live visual attention data for 3 observer targets per group (a total of 12). When collecting the video prior to beginning the conversation, targets were asked to systematically look at each person in the room. This gave us a “calibration” to which the researcher could refer when making decisions regarding coding where the target was looking.
Clips of the live behaviour from the other side of the table were trimmed to the exact time of the eight experimental clips used in our main experiment. By choosing these exact moments of conversation, we can make a comparison between the gaze of people sitting in the room with the targets and the gaze of our eyetracked participants who later watched the videos. Of course, there are differences between these two sources of data because we only have three people sitting opposite each target in real life, and we rely on coding their gaze from video, compared to a much larger group of eyetracked participants. In this section, we, therefore, focus on describing similarities and differences rather than null hypothesis significance testing.
We logged the time at which each utterance began and ended alongside where each target was looking (for Targets 4, 5, and 6) at each point in time. To accurately log when the utterances began and ended, we used the auditory signal with the visual signal to assist in identifying the speaking target. We found we could reliably determine when the targets (T4, T5, and T6) were looking at T1, T2, or T3 (located opposite). Gaze to these locations was clearly visible and accompanied by head movements. To code the looking behaviour, we used VideoCoder (1.2), a custom software tool designed for accurately time-stamping events in video. Gaze locations were then manually categorized according to which target was being fixated and whether that target was currently speaking. This log was prepared by the author and one other naive researcher, with high interrater reliability of the coding (98% agreement of sample compared).
Time series for gaze and speaking were analysed in MATLAB. We removed the small percentage of time in which the monitored targets were speaking themselves. Overall, averaged across the 12 individuals and 8 clips which we coded, targets spent 59.04% of the time looking at a target on the other side of the table who was currently speaking, 34.88% of the time on a non-speaking target, and 6.09% of the time looking elsewhere (such as at the table or their own hands).
Comparing live interaction with third-party viewing
We began by comparing gaze in the live situation with that from the Control condition, in which third-party observers watched videos of the same targets without any audiovisual manipulation. Here we are comparing manual coding of looking behaviours (live interaction), with eyetracked data, collected in the lab, from the Control condition (third-party viewing). compares the proportion of time that the interacting targets spent looking at the speaker with the same percentages from third-party video watching.
Table 1. Average percentage time spent in each of the gaze locations for the live behaviour and the third-party viewing of the Control condition.
In general, the percentages are quite similar, with a majority of time spent looking at the person speaking in both the live and lab situation. We make this comparison cautiously, given the differences in sample size and data collection, and refrain from a statistical comparison. It may be that the bias towards the speaker is reduced in third-party participants watching the interaction on video, who look at the non-speaking targets more than in the live situation. A potential explanation for this is that in a live situation, we tend to look to a person speaking. We do this to signal that we are listening (e.g., Freeth et al., Citation2013). When watching a recording, this signalling is not possible, which perhaps meant that participants felt more able to visually explore the other targets.
Comparing timing of looks
For a more in-depth assessment of the similarities in looking behaviour between the live interaction and participants watching the videos, we compared the time series of who was being looked at in each case. illustrates one example in which we compare the eight participants (P1-8) who saw the first clip in the Control condition with the three targets (T4, T5, and T6) who were present in the room. Gaze time series are displayed for each observer (right panels), while we also plot the proportion of observers looking at each target at each point in time (left panels). It is clear that observers are highly consistent, both within a group and between the two environments. For example, most observers shift gaze from Target 3 to Target 1 about a third of the way through the clip. Target 2 receives less attention, with looks to this person peaking at the end of the clip.
Figure 3. Time series representing the gaze location of each eyetracked participant (P1–P8, third-party participants) and each interacting target (T4–T6, live interaction) as they looked at the targets of interest (T1–T3). Line charts on the left show the proportion of observers gazing at each location (data smoothed over time). Coloured bars on the right show the target being looked at by each observer. In each case, time is on the x-axis (clip duration = 39,000 ms). Within this example there is an average of 88% agreement between live and third party (average κ = .76 with all pairings p < .001).
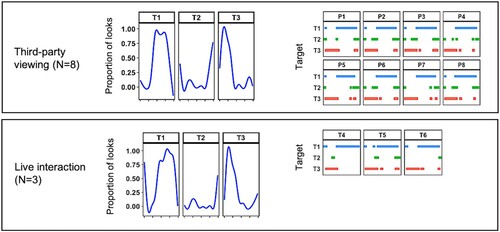
Measuring agreement
To test the strength of gaze “agreement” (the extent to which those in the live and third-party condition were looking at the same target at the same time), we calculated the amount of time when the gazed-at location was the same in each pair of observers, comparing each live observer to each video-watcher in the lab. We excluded times when observers were looking elsewhere (this included blinks or data loss in the eyetracked data and times when the real-life observers were looking down or at people on their own side of the table).
When analysing the time series, across all combinations of pairs in each clip, an average of 60.10% of looks were to the same target (T1–T3) at the same time, which is greater than chance (33.3% of all visual attention if this was shared equally between targets T1, T2, and T3). Additionally, a Cohens kappa analysis was run to determine the strength of agreement in gaze. The kappa statistic provides a proportion of agreement over and above chance when comparing nominal data. In general, there was substantial to a strong agreement, with all combinations providing a kappa coefficient which was significantly different from chance. Across the eight experimental clips, there was a mean (SD) kappa of .79 (0.1), with all combinations reaching at least a kappa of .59.
Together, these analyses demonstrate that people view the conversation in a similar way whether they are in a real situation taking part in the conversation themselves or different participants watching the conversation on video at a later stage. This is reassuring as it suggests that gaze behaviour to the videos will be a good proxy for complex social attention in a face-to-face situation.
Eye tracking experiment
We have established that there are similarities between visual attention in conversation following when watching videos (Control condition) and in the wild (real interaction between targets). Observers tend to look at the speaker, and show consistent patterns over time related to the conversation. We now test how these patterns are affected by manipulations of audio and visual cues, as well as examining the timing of gaze in the detail afforded by a controlled eye tracking experiment. Due to the nature of the live interaction, we cannot compare how audiovisual cues affect visual attention during live setting. The remainder of the analysis is therefore for third-party participants only. As described in “Target Clip Preparation”, we had 4 audiovisual conditions: Control, Silent, Freeze Frame and Blank. If the tendency to look at the speaker at a particular time is dependent on auditory information and/or visual cues such as gestures, then we should expect this to be reduced in the Silent and Freeze Frame conditions, respectively.
Outliers and exclusions
All participants scored over 50% on the comprehension questions, with a minimum accuracy of 58% and an overall mean of 88% correct, so no participants were excluded on this basis. However, three participants were excluded due to a failure to calibrate and validate the eyetracker within satisfactory parameters, resulting in frequent missing data. For this reason, 37 participants were included in the main analysis.
How do audiovisual cues affect conversation following?
The presented analysis considers gaze behaviour only after the critical time point in each clip (i.e., from the point at which the clip was silenced, frozen, or blanked) and the equivalent time in the Control condition. This critical time point varied slightly across the eight experimental clips but was identical for all conditions within a particular clip. Due to the differences in the critical time period, we report durations as a percentage of the total length of this period.
Fixations on targets
To explore how the condition affected how much participants looked at the three targets, a region of interest (ROI) was defined around each target individual that was present. The ROIs subtended approximately 10° × 11.5° of visual angle, see ; however, this varied according to the physical size of the target within the scene. For comparison, in the Blank condition, the same ROIs were used even though the image had at this point disappeared.
The number of fixations on these ROIs was then analysed. Pooling these ROIs together, we found that, regardless of condition, participants spent the majority of time looking at the targets rather than elsewhere on screen (see ).
Table 2. Overall percentage of fixations on targets, post clip manipulation (average taken from 37 participants).
A repeated-measures ANOVA established that there was a significant difference between the percentage of fixations on targets in the different conditions, F(3,108) = 43.43, p < .001, .
Pairwise comparisons with a Bonferroni correction (SPSS adjusted p values), revealed that the percentage of fixations on target ROIs in the Blank condition was significantly lower than the other conditions (all t(36) > 6.67, p < .001, dz > 1.10) . This is perhaps not surprising given that the targets were no longer visible in the Blank condition. There was also a significant difference between the Control and the Freeze Frame conditions (t(36) = 3.03, p = .027 dz = 0.63), with slightly fewer fixations on the targets when the video was paused. Although the Freeze Frame and Blank conditions elicited fewer fixations on targets, participants still looked at these regions on 94% and 72% of their fixations, respectively. Hence, despite there being no new visual information available at this point (since the video had paused or disappeared), participants continued to fixate the location where the targets had been for the majority of the time. It could be argued that the targets take up a large proportion of the screen, although the targets do not consume an area of the screen approaching these high percentages (roughly 42%).
Fixations on targets’ eyes and mouth
Previous studies have found differences in fixations on targets’ eye and mouth regions when sound information is removed, but these have been small or inconsistent (Foulsham & Sanderson, Citation2013; Võ et al., Citation2012). For this reason, in our next analysis, moving ROIs were created for the eight experimental clips using Data Viewer (SR research). An ROI was drawn around each of the targets’ eye and mouth area, and its position throughout the recording was adjusted by slowly playing the clip back with “mouse record” (an inbuilt function in Data Viewer). For comparison, the location of these interest areas remained at the same location in the Freeze Frame and Blank conditions (i.e., at the location of the eyes and mouth when the video paused or was removed). Fixations were then analysed to determine whether they were inside this area.
shows the percentage of all fixations on the targets' eyes, mouth, and elsewhere averaged across participants. It is important to note that “elsewhere” includes the rest of the target and background areas.
Figure 5. The overall percentage of fixations on the targets’ eyes, mouth and “elsewhere” (sum per condition = 100%), averaged across the participants. Error bars show standard error.
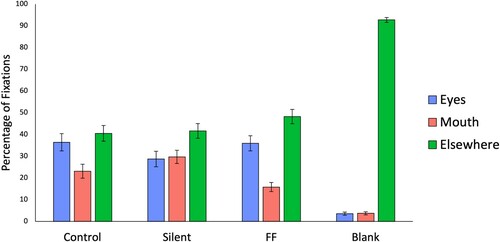
A repeated-measures ANOVA established that there was a significant difference between conditions when analysing looks to the eyes, F(3,108) = 40.94, p < .001, .
Post hoc analysis with the Bonferroni correction (SPSS adjusted p values) revealed there were no significant differences between the Control, Silent and Freeze Frame conditions (all t(36) < 2.50, p > .10, dz < 1.33). Hence, the looks to eyes did not significantly decrease with the sound muted or with the image stilled. The only condition with a different pattern was the Blank condition which was significantly different from all other conditions (all t(36) > 7.26 p < .001, dz >1.24).
When analysing looks to the mouth, a repeated-measures ANOVA established that there was a significant difference between conditions, F(3,108) = 30.88, p < .001, . With a Bonferroni correction (SPSS adjusted p values), there were significant differences between the Blank condition and all other conditions (all t(36) > 5.97, p < .001, dz >1.11). There were no significant differences when comparing the Control condition with the Silent and Freeze Frame conditions (both t(36) < 2.61 p > .79, dz < 1.18). Looking at the average percentages of looks to the mouth, we can see that removing the sound did slightly increase looks to the mouth (and reduce those to the eyes), which is similar to the pattern reported by Foulsham and Sanderson (Citation2013). However, as in that study, the difference was not significant.
Fixations on speaking targets
Next, we used the previously described record of who was speaking to examine how the condition affected the tendency to look at the speaker. shows the total proportion of fixations that landed on a target who was currently speaking, grouped by condition.
Figure 6. Percentage of fixations on target speakers for each condition (note “other targets” refers to the two other non-speaking targets grouped together). Error bars show standard error.
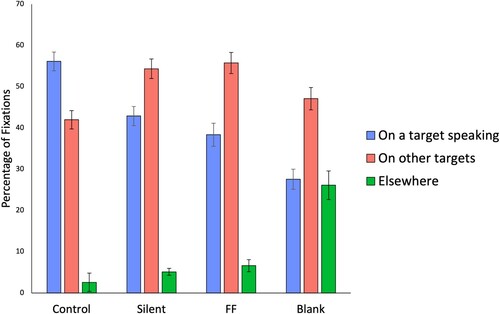
A 3 × 4 fully within subjects ANOVA was carried out for percentage of fixations on the (3) regions of interest and in each of the (4) conditions. There was no significant main effect of condition; however, there was a main effect of fixation location F(2,72) = 338.18, p < .00,1 . More importantly, there was an interaction between condition and fixation location F(6,216) = 18.31, p < .001,
. This emerged since the distribution of fixations to locations in the Control condition was different from the other conditions. In this condition, most of the fixations were on the person currently speaking, with fewer on one of the (non-speaking) targets (even though this comprised two different targets). The Control condition is most similar to the live viewing behaviour, which we discussed earlier, and has a similar ratio of looks to speakers and non-speakers. In contrast, fixations on the speaker were reduced in the Silent and Freeze Frame conditions and reduced further in the Blank condition.
When are speakers looked at?
Timing of fixations on speaking targets
We then analysed the point in time at which participants made a fixation on a speaker, in order to understand whether conversation following varied with the cues provided in the four conditions. The start times of each utterance from each target in each clip were used to create 10 ms bins ranging from 1000 ms before speech beginning to 1000 ms after speech beginning. We then compared these bins to the fixation data and coded bins as to whether they contained a fixation on the target speaking, a fixation elsewhere, or no fixation. The result was an estimate of the probability of looking at a speaker, time-locked to the beginning of their speech. plots this estimate averaged across all clips and utterances and split by condition.
Figure 7. Probability of fixation being on the speaker, relative to when they started speaking. Lines show the smoothed, average proportion of fixations at this time on the speaker, in 10 ms bins (with 95% CI). A time of 0 indicates the time at which a speaker began speaking. FF: freeze frame condition.
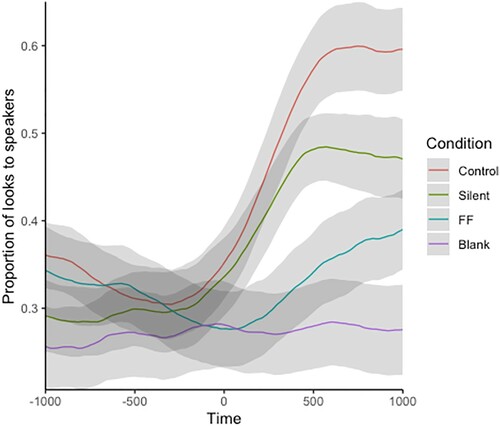
As previously discussed, from , we can see there are fewer looks to speakers in the modified conditions in comparison to the Control condition. There are, however, clear increases at the time of speech onset in both the Silent and the Freeze Frame condition, which indicates that participants are still shifting their visual attention to that target. The slope of this increase is similar in the Silent and Control conditions. Although the slope of the Freeze Frame condition is more gradual, it still rises to a peak, indicating increased attraction to a speaking target.
To quantify the time at which the probability of fixation diverges between conditions, we ran a cluster-based permutation analysis between each pair of conditions. This is a non-parametric method for comparing timecourses while controlling for multiple comparisons (Dink & Ferguson, Citation2015). The results indicated that most of the conditions diverged significantly close to the moment the utterances began (between −50 ms and 60 ms relative to when the speaker began talking). Interestingly, as can be seen in , the visually dynamic conditions (Control and Silent) diverge from each other much later (with a significant difference after 450 ms). The visually static conditions (FF and Blank) also diverge later (after 570 ms). This implies significant increases in the proportion of looks to speakers at the time of utterance when dynamic visual information is present and a slower effect of the divergence between the combinations of the visual and auditory information.
Discussion
The present study provided an innovative way to evaluate viewing behaviours during a conversation in a live situation and when observers watched a recording. In line with previous research, this study found that most visual attention was directed towards the targets, with little to no attention to background areas. There was also a tendency to look to the person currently speaking. This was apparent for both real interactants and third-party observers. There were a number of interesting findings relating to manipulation of signalling cues and the timing pattern of looks to targets, which we here discuss with reference to our three research questions.
Does third-party viewing reflect live gaze behaviour?
First, we investigated visual attention during a real group conversation and how it relates to third-party viewing. There is existing observational research investigating gaze in face-to-face conversation (see Hessels, Citation2020 for an extensive review), and previous studies have used eye tracking while people watch pre-recorded conversations (e.g., Foulsham et al., Citation2010; Foulsham & Sanderson, Citation2013; Tice & Henetz, Citation2011). However, to our knowledge, this is the first time that gaze in a real multi-party conversation has been explicitly compared to fixations from people watching videos of the same interaction. Our findings demonstrated that in a live situation, people sitting opposite their interlocutors show a similar pattern of gaze behaviour to participants who were watching the conversation at a later stage. For instance, the tendency to look at the person who is currently speaking was similar, comprising 59% and 51% of the time, for live and third-party viewing, respectively. The similarities in gaze distribution in the two settings indicate that the sole purpose of looking to a speaker isn’t to signal that we are listening, as we see this effect in the third-party video setting, which does not require any social signalling. Therefore, looking to the speaker must benefit conversation following for another reason or may be a habitual behaviour. It is not surprising that these percentages are somewhat less than previous studies (such as Argyle and Ingham (Citation1972)), as the present study included a complex group interaction, rather than a dyad, with more targets for participants to distribute their attention between.
The temporal pattern of looks to different potential targets was also strikingly similar between the real interaction and the video watching condition. Different individuals tended to look at the same target at the same time, and this was also true when we compared eyetracked observers with the people who were actually in the room with these targets. There was a high level of agreement in all cases. It, therefore, seems that visual attention to the conversation in live interlocutors and third-party observers shows a similar pattern. Future studies could also explore to what extent there is a lag when comparing the timecourse of looks to speakers in real interactions and when watching video.
Although there are good reasons to think that social gaze operates differently in face-to-face situations (Risko et al., Citation2012; Risko et al., Citation2016), it may be that the pattern of conversation following observed here is rather unaffected by actual social presence. This would indicate that investigations, where third-party participants observe conversations on video, provide a good test-bed for realistic social attention. On the other hand, we did observe some differences between settings that could be pursued in future research. On average, third-party observers spent more time looking at targets who were not speaking than those in the face-to-face situation. This could be explained by social norms, which are only present with social presence when interacting face to face. For example, in a live group conversation, it might be considered odd to look at someone who is not speaking when they're currently is another member of the group speaking and when collectively, the group attention is on the speaker. However, if you are watching a video recording of the conversation, this social rule is not present, and participants may have felt freer to explore the reactions of other targets to the speaker. In larger groups, this might be particularly prevalent so that listeners can check group agreement or monitor other’s reactions to the conversation. This relates to previous early work, such as by Ellsworth et al. (Citation1972), who demonstrated the discomfort which arises when being stared at. Additionally, this dovetails with research by Laidlaw et al. (Citation2011), where it was demonstrated how the presence of a confederate (versus the same confederate on videotape) increased visual avoidance. Critically, in the video condition in both Laidlaw et al. (Citation2011) and our study, participants could not see or interact with the targets, and so any “signalling” function of gaze was absent (Risko et al., Citation2016).
Interestingly, we saw striking similarities in eye movement behaviour in the two settings even though in the live situation, the targets were acquaintances and in the third-party situation, the targets were strangers. In future studies, it would be interesting to explore the effect of familiarity on both the live eye movements and in the third-party participants (for example, using observers from the same sports team).
Overall, we analysed visual attention within a larger group setting, with multiple targets and multiple turn-taking transitions. Despite some differences in attention to non-speaking targets, those who were taking part in the conversation and those who watched the same conversation at a later stage still show a bias to the speaker. This suggests that the distribution of gaze is similar in both settings and furthermore suggests social gaze in a complex environment is similar to that of studies which use dyad pairs. This provides further evidence for the ecological validity of understanding social attention via pre-recorded videos.
How do audiovisual cues affect conversation following?
An advantage of using video recorded stimuli, of course, is that they can be controlled and manipulated in a way that real interactions cannot. In the present study, we manipulated conversation video clips in order to test the role of audio information (by removing the sound) and dynamic and static visual information (by freezing the image or completely blanking the display while the sound continued). After these manipulations occurred, participants spent 98%, 97%, and 94% of the time looking at the targets in the Control, Silent, and Freeze Frame conditions, respectively. Percentages this high are not particularly surprising considering that the targets were the main focus of the scene and that previous evidence indicates that observers are biased to look at people. In the Control and Silent condition, the targets were also the only moving objects within the scene. However, the result in the Freeze Frame condition is particularly interesting as no new visual information could be gained from continuing to fixate the targets. In short, the tendency to look at the targets in these clips was not due to either the audio information or the dynamic visual information, which would allow integration of speech and vision.
In the Blank condition, the percentage of fixations on where the targets once were dropped to 72%. Although the targets were the main focus of the scene, the ROIs around the targets took up less than half of the screen area. Hence it could be argued that even in the Blank condition, participants did continue to fixate the location of the targets once the image had been removed. This could relate to previous research on “looking at nothing”. For example, Richardson and Spivey (Citation2000) demonstrated that when participants are shown a blank screen and asked to recall information, there is a tendency for them to look to the space in which the recalled item was once located. The authors argue that this is linked to the memory of spatial location and the cognitive-perceptual system’s ability to attach a spatial tag to a semantic location. A second study that supports this notion of visually “following” or attending to an object which is not visually present, is that by Spivey et al. (Citation2000). That study found that when participants are passively listening to audio of a story which includes directionality (such as a train moving from right to left), saccades follow the same pattern, in that the eye movements cluster along the same axis, even when the eyes are closed. The current study provides some evidence for spatial indexing in that participants may have associated voices and the mental model of the conversation with locations on the screen. As a result, blank regions where targets had previously been located remained salient to look at. In the Freeze-Frame condition, participants may also have created an association between the voice of the target and their spatial position on screen. Future research could investigate whether this association and related eye movements might facilitate the participants’ understanding of the scene.
We then explored whether our clip manipulations affected looks to the current speaker. Foulsham and Sanderson’s (Citation2013) study, which used a similar methodology, found that participants looked most to a person currently speaking (both in their Control and Sound Off condition). Arguably, the results in their Sound Off condition could be due to the participant attempting to lip read, or to the fact that movement attracts our attention. We questioned whether we too would find this effect in our Silent condition and whether manipulating the visual information would affect looks to speakers. We found that participants fixated on speaking targets for 56% of fixations in the Control condition and 43% in the Silent condition, and comparably to Foulsham and Sanderson (Citation2013), removing the sound did slightly increase looks to the mouth. In comparison, Võ et al. (Citation2012) report that removing the audio decreased looks to the mouth region. This may be explained in terms of the task. In the present study, there were functional benefits of looking at the mouth (to provide a correct answer on the attention check question), whereas Võ et al. (Citation2012) asked the observer to rate likeability, which may not have required conversation understanding. Interestingly, in the current study, when the video was paused, participants continued to look at the eyes with a frequency similar to the normal Control condition, while their looks to the mouth decreased.
Although there was no additional information gained, participants fixated speaking targets (or the space where they once resided) for 38% and 28% of fixations for the Freeze Frame and Blank condition, respectively. The low percentages are not surprising in the Blank condition. However, the Silent and Freeze Frame condition do show some evidence to suggest that people continue to track a speaker without dynamic or audio information. The differences between the Control and Freeze Frame condition could be explained in that without any dynamic visual information (i.e., observing the targets’ mouth moving), it is difficult to determine the current speaker from audio alone. However, even in the Freeze Frame condition, there were more fixations on the current speaker than we would expect if attention was allocated equally to all the targets. This suggests that people may attempt to look at a speaker upon hearing their voice to gain more information, as in spatial indexing during the “looking at nothing” phenomena. Perhaps attaching the voice to a static image of the speaker provides richer understanding of the conversation and helps us to explain why we see a gaze shift towards a speaker.
Further work should attempt to understand to what extent and why do we continue to associate the audio of the target’s voices with their spatial location and what benefit, if any, there is to this behaviour.
When are speakers looked at?
Foulsham et al. (Citation2010) reported that gaze tends to precede or predict a change in speaker, such that observers look at conversants slightly before they start to speak. Similarly, Tice and Henetz (Citation2011) found results that demonstrate anticipatory looks to a speaker (taking into account the 200 ms to plan and execute an eye movement). Gaze also tends to precede speech in real face-to-face conversations, at least in dyads, where speakers look at a listener at the end of their “turn” in order to signal a change in speaker. However, it is less clear cut as to whether this happens in group conversations and whether these anticipations rely on particular audiovisual cues. For example, Holler and Kendrick (Citation2015), report that when interacting in a group of three, interlocutors are able to anticipate speakership, yet Foulsham and Sanderson (Citation2013), who use a similar methodology to the present study, report no anticipatory effect. That study investigated the role of speech sound on gaze to speakers, by showing clips of group conversations to participants. The participants fixated the speaker quickly after they began speaking, but there was no evidence of a preceding shift. We expanded upon this to investigate whether an anticipatory effect would be present and whether this was affected by the presence of auditory cues (such as the content of the conversation) or visual signals (such as gestures or expressions before a new speech turn). In the present study, consistent with Foulsham and Sanderson (Citation2013), no anticipatory effect was found in the Control condition, with fixations on speaking targets occurring on average 450–500 ms after the start of an utterance.
There could be a number of reasons for this finding when using “natural” conversation as stimuli. First, the type of clip used in the current study was quite different from Tice and Henetz (Citation2011) despite both including a group conversation. In Tice and Henetz (Citation2011) the stimuli used are from a Hollywood movie, “Mean Girls,” which comprises a split-screen dialogue. Such clips are designed in a way to guide our visual attention to the most critical areas of the scene through the use of camera angles and cinematic effects. This is likely to make it very clear who is speaking and when. For example, when a new character speaks, the screen splits further, directing your attention to the new element within the scene. For this reason, the conversation following (and in this case, anticipation of speaker) is facilitated by the editing (for an in-depth computational model of gaze trajectory in staged conversation see Boccignone et al., Citation2020). In the present study, the conversations were unscripted, unedited, and more complex, reflecting a real-life chat amongst friends. Targets often interrupted or spoke over each other, and hence, perhaps in a real-world situation with multiple sources of sound, it is more difficult to predict the next speaker.
Despite there being no evidence of an anticipatory shift, participants do move quickly to fixate a target who begins speaking (see ). There is evidence for this in the Control condition, and to a lesser extent in the Silent and Freeze Frame conditions. Compared to the Control condition, there is a similar rise in the probability of looks over the time course in the Silent and Freeze Frame conditions. This adds further evidence that participants do follow the conversation without the use of the full set of audiovisual cues. We, therefore, suggest, in audiovisual recordings, when one modality is redundant, participants rely on the signalling cues available within the other modality to follow conversation.
Conclusions
The present study offered a chance to investigate audiovisual cues and make a comparison between live and third-party viewing behaviours, during a large group conversation. We demonstrate that live viewing behaviour during interactive conversation is similar for those taking part in the conversation and separate observers at a later stage, highlighting the propensity to follow speakers during a conversation in both situations. We further emphasized the ability for participants to exploit cues in both the spoken conversation and the movements of targets to follow turn-taking in conversation, even when one modality is removed. Removing the audio replicated the results of Foulsham and Sanderson (Citation2013), with participants able to follow only visual cues to guide their attention. In addition, there is evidence for a tendency to look at the speaker, even when no additional visual information is gained (when the scene is frozen). The results provide insight into using audio cues to direct our attention, as well as how and why we observe dynamic and complex group engagement scenes in a setting of more naturalistic composition. Overall, this study provides us with rich information about how visual attention is directed within multifaceted large group conversations with both manipulated videos and interactions during a live conversation.
Disclosure statement
No potential conflict of interest was reported by the author(s).
Additional information
Funding
References
- Altmann, G. T. M. (2004). Language-mediated eye movements in the absence of a visual world: The ‘blank screen paradigm’. Cognition, 93(2), 79–87. https://doi.org/https://doi.org/10.1016/j.cognition.2004.02.005
- Argyle, M., & Ingham, R. (1972). Gaze, mutual gaze, and proximity. Semiotica, 6(1), 32–49. https://doi.org/https://doi.org/10.1515/semi.1972.6.1.32
- Boccignone, G., Cuculo, V., D’Amelio, A., Grossi, G., & Lanzarotti, R. (2020). On gaze deployment to audio-visual cues of social interactions. IEEE Access, 8, 161630–161654. https://doi.org/https://doi.org/10.1109/ACCESS.2020.3021211
- Dink, J. W., & Ferguson, B. (2015). eyetrackingR: An R library for eye-tracking data analysis. http://www.eyetrackingr.com
- Ellsworth, P. C., Carlsmith, J. M., & Henson, A. (1972). The stare as a stimulus to flight in human subjects: A series of field experiments. Journal of Personality and Social Psychology, 21(3), 302–311. https://doi.org/https://doi.org/10.1037/h0032323
- Foulsham, T., Cheng, J. T., Tracy, J. L., Henrich, J., & Kingstone, A. (2010). Gaze allocation in a dynamic situation: Effects of social status and speaking. Cognition, 117(3), 319–331. https://doi.org/https://doi.org/10.1016/j.cognition.2010.09.003
- Foulsham, T., & Kingstone, A. (2017). Are fixations in static natural scenes a useful predictor of attention in the real world? Canadian Journal of Experimental Psychology/Revue Canadienne de Psychologie Expérimentale, 71(2), 172–181. https://doi.org/https://doi.org/10.1037/cep0000125
- Foulsham, T., & Sanderson, L. A. (2013). Look who’s talking? Sound changes gaze behaviour in a dynamic social scene. Visual Cognition, 21(7), 922–944. https://doi.org/https://doi.org/10.1080/13506285.2013.849785
- Foulsham, T., Walker, E., & Kingstone, A. (2011). The where, what and when of gaze allocation in the lab and the natural environment. Vision Research, 51(17), 1920–1931. https://doi.org/https://doi.org/10.1016/j.visres.2011.07.002
- Freeth, M., Foulsham, T., Kingstone, A., & Paterson, K. (2013). What affects social attention? Social presence, eye contact and autistic traits. PLoS One, 8(1), e53286. https://doi.org/https://doi.org/10.1371/journal.pone.0053286
- Hayward, D. A., Voorhies, W., Morris, J. L., Capozzi, F., & Ristic, J. (2017). Staring reality in the face: A comparison of social attention across laboratory and real world measures suggests little common ground. Canadian Journal of Experimental Psychology/Revue Canadienne de Psychologie Expérimentale, 71(3), 212–225. https://doi.org/https://doi.org/10.1037/cep0000117
- Hessels, R. S. (2020). How does gaze to faces support face-to-face interaction? A review and perspective. Psychonomic Bulletin & Review, 27(5), 856–881. https://doi.org/https://doi.org/10.3758/s13423-020-01715-w
- Hirvenkari, L., Ruusuvuori, J., Saarinen, V.-M., Kivioja, M., Peräkylä, A., Hari, R., & Lappe, M. (2013). Influence of turn-taking in a two-person conversation on the gaze of a viewer. PLoS ONE, 8(8), e71569. https://doi.org/https://doi.org/10.1371/journal.pone.0071569
- Ho, S., Foulsham, T., & Kingstone, A. (2015). Speaking and listening with the eyes: Gaze signaling during dyadic interactions. PLoS ONE, 10(8), 1–18. https://doi.org/https://doi.org/10.1371/journal.pone.0136905
- Holler, J., & Kendrick, K. H. (2015). Unaddressed participants’ gaze in multi-person interaction: Optimizing recipiency. Frontiers in Psychology, 6. https://doi.org/https://doi.org/10.3389/fpsyg.2015.00098
- Laidlaw, K. E. W., Foulsham, T., Kuhn, G., & Kingstone, A. (2011). Potential social interactions are important to social attention. Proceedings of the National Academy of Sciences of the United States of America, 108(14), 5548–5553. https://doi.org/https://doi.org/10.1073/pnas.1017022108
- Latif, N., Alsius, A., & Munhall, K. G. (2018). Knowing when to respond: The role of visual information in conversational turn exchanges. Attention, Perception, and Psychophysics, 80(1), 27–41. https://doi.org/https://doi.org/10.3758/s13414-017-1428-0
- Penn, C. (2000). Paying attention to conversation. Brain and Language, 71(1), 185–189. https://doi.org/https://doi.org/10.1006/brln.1999.2247
- Richardson, D. C., Altmann, G. T. M., Spivey, M. J., & Hoover, M. A. (2009). Much ado about eye movements to nothing: A response to Ferreira et al.: Taking a new look at looking at nothing. Trends in Cognitive Sciences, 13(6), 235–236. https://doi.org/https://doi.org/10.1016/j.tics.2009.02.006
- Richardson, D. C., & Spivey, M. J. (2000). Representation, space and Hollywood squares: Looking at things that aren’t there anymore. Cognition, 76(3), 269–295. https://doi.org/https://doi.org/10.1016/S0010-0277(00)00084-6
- Risko, E. F., Laidlaw, K. E. W., Freeth, M., Foulsham, T., & Kingstone, A. (2012). Social attention with real versus reel stimuli: Toward an empirical approach to concerns about ecological validity. Frontiers in Human Neuroscience, 6(143), 1–11. https://doi.org/https://doi.org/10.3389/fnhum.2012.00143
- Risko, E. F., Richardson, D. C., & Kingstone, A. (2016). Breaking the fourth wall of cognitive science: Real-world social attention and the dual function of gaze. Current Directions in Psychological Science, 25(1), 70–74. https://doi.org/https://doi.org/10.1177/0963721415617806
- Spivey, M., Tyler, M., Richardson, D., & Young, E. (2000). Eye movements during comprehension of spoken scene descriptions. In L. R. Gleitman & A. K. Joshi (Eds.), Proceedings of the twenty-second annual conference of the cognitive science society (pp. 487–492).
- Tice, M., & Henetz, T. (2011). The eye gaze of 3rd party observers reflects turn-end boundary projection. Proceedings of the 15th workshop on the semantics and pragmatics of dialogue (pp. 204–205).
- Võ, M. L.-H., Smith, T. J., Mital, P. K., & Henderson, J. M. (2012). Do the eyes really have it? Dynamic allocation of attention when viewing moving faces. Journal of Vision, 12(13), 3. 1–14. https://doi.org/https://doi.org/10.1167/12.13.3
- Zion-Golumbic, E., Cogan, G., Schroeder, C., & Poeppel, D. (2013). Visual input enhances selective speech envelope tracking in auditory cortex at a “cocktail party”. The Journal of Neuroscience: The Official Journal of the Society for Neuroscience, 33(4), 1417–1426. https://doi.org/https://doi.org/10.1523/JNEUROSCI.3675-12.2013