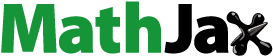
ABSTRACT
Visual routines theory posits that vision is critical for guiding sequential actions in the world. Most studies on the link between vision and sequential action have considered individual agents, while substantial human behaviour is characterized by multi-party interaction. Here, the actions of each person may affect what the other can subsequently do. We investigated task execution and gaze allocation of 19 dyads completing a Duplo-model copying task together, while wearing the Pupil Invisible eye tracker. We varied whether all blocks were visible to both participants, and whether verbal communication was allowed. For models in which not all blocks were visible, participants seemed to coordinate their gaze: The distance between the participants' gaze positions was smaller and dyads looked longer at the model concurrently than for models in which all blocks were visible. This was most pronounced when verbal communication was allowed. We conclude that the way the collaborative task was executed depended both on whether visual information was available to both persons, and how communication took place. Modelling task structure and gaze allocation for human-human and human-robot collaboration thus requires more than the observable behaviour of either individual. We discuss whether an interactive visual routines theory ought to be pursued.
Introduction
How do humans act in the world? Describing, explaining, and predicting the varied nature of human behaviour or action is a daunting task, and has to be approached from various perspectives. To name but a few examples, researchers have investigated the settings in which certain patterns of behaviour occur (Barker, Citation1968), how sequential patterns of action may be controlled (Botvinick & Plaut, Citation2004, Citation2006; Cooper & Shallice, Citation2000, Citation2006; Norman & Shallice, Citation1986), or how humans interact with others, including interpersonal coordination (Marsh et al., Citation2006, Citation2009; Paxton & Dale, Citation2013; Sebanz et al., Citation2006), non-verbal exchange (Patterson, Citation1982), interpersonal intimacy (Argyle & Dean, Citation1965; Patterson, Citation1976), and the modes and flow of face-to-face interaction (Hadley et al., Citation2022; Hessels, Citation2020; Ho et al., Citation2015; Maran et al., Citation2021, Citation2022; Wohltjen & Wheatley, Citation2021). The observant reader of this literature may notice an apparent dichotomy between individual or object-oriented action and multi-agent (social) action. For example, the studies on sequential action by Land et al. (Citation1999), Hayhoe (Citation2000), Botvinick and Plaut (Citation2004), Cooper and Shallice (Citation2006) are concerned with making tea or sandwiches, which involve objects (cups, knives, faucets, kettles) that do not act back. In contrast, any action an agent carries out in interaction with another, may evoke a response which changes the course of sequential behaviour of both individuals. In this paper, we are concerned with the role of visual behaviour at this interface of sequential action and social interaction.
Humans are visual animals, and the link between vision and (sequential) action has been widely studied. A well-established theoretical framework in this context is that of visual routines (Hayhoe, Citation2017; Ullman, Citation1996), which postulates that “vision is critical for gathering knowledge about the world to choose rewarding actions as well as for guiding the execution of those actions” (Hayhoe, Citation2017, p. 391). As Hayhoe points out, this framework puts vision for action, memory and prediction at its core unlike e.g., Marr (Citation1982), who emphasizes object recognition and localization, or ecological approaches (e.g., Warren, Citation2006; Zhao & Warren, Citation2015) that emphasize informational coupling and online control (i.e., without memory and prediction). In the visual routines framework, knowledge about the world may be gathered by making eye movements, i.e., directing one's gaze to locations in the world to acquire the relevant informationFootnote1 for ongoing behaviour (Hayhoe & Ballard, Citation2005, Citation2014). The pioneering studies on the relation between gaze behaviour and task execution were conducted by Land et al. (Citation1999), Hayhoe (Citation2000), Pelz and Canosa (Citation2001), who used wearable eye-tracking technology to show that the fixation location in the world was tightly linked to the task, e.g., looking at the faucet prior to pouring water in the kettle while making tea. According to Land et al. (Citation1999), less than 5% of fixations were on task-irrelevant locations, while Pelz and Canosa (Citation2001) concluded that most fixations were related to the immediate action, and some fixations were of a look-ahead nature for an upcoming action (see also Sullivan et al., Citation2021, for more recent work on look-ahead fixations). In follow-up work, task-related gaze behaviour was investigated in the context of e.g., collision avoidance (Jovancevic et al., Citation2006; Jovancevic-Misic & Hayhoe, Citation2009; Tong et al., Citation2017), crowd navigation (Hessels, van Doorn et al., Citation2020), foot control in rough terrain (Matthis et al., Citation2018), and stair climbing (Ghiani et al., Citation2023). The core idea of the visual routines theory is that cognitive goals or tasks (e.g., “walk across street”) can be subdivided into subtasks (“monitor context,” “avoid obstacles,” “approach goal”) (see Figure in Hayhoe, Citation2017). For each subtask, a different area of the world can be fixated to provide the relevant visual information to successfully achieve that subtask, i.e., gaze behaviour serves an “information-gathering” functioning (cf. Võ et al., Citation2012).
Figure 1. Schematic top view of the setup for the collaborative Duplo model copying experiment. Dyads copied a model in the build area, using the blocks provided in a separate area. Fiducial markers were black and white in the real setup, but lowered in contrast here for visualization purposes.
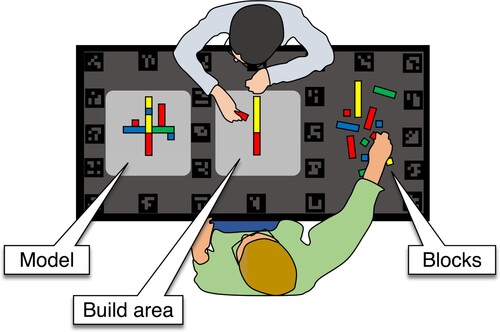
Yet, one's gaze direction has also been posited to serve an “information-signalling” function. Argyle and Cook (Citation1976) write that “whenever organisms use vision, the eyes become signals as well as channels” (p. xi), which Risko et al. (Citation2016) explicate as “the eyes both gather information […] and communicate information to others” (p. 71). This relevance of gaze direction for social interaction has long been known, as Kendon (Citation1967) points out, and it is well established that one's gaze direction may, for example, regulate the maintenance or exchange of speaking turns in conversation (Hessels et al., Citation2019; Ho et al., Citation2015; Maran et al., Citation2021; Wohltjen & Wheatley, Citation2021) and may express intimacy or exercise social control (Argyle & Dean, Citation1965; Kendon, Citation1967; Kleinke, Citation1986). Moreover, one's gaze direction may be used as a (gaze) cue to disambiguate expressions in spontaneous dialogue (Hanna & Brennan, Citation2007) or when following instructions (Macdonald & Tatler, Citation2013). This combination of “information-gathering” and “information-signalling” functions is colloquially described as the dual function of gaze, and has seen a resurgence in the scientific literature in recent years. In particular, this resurgence seems to follow the realization that humans do not always look at other people (or their faces and eyes) in many social contexts, in contrasts to what one might have expected given the bias for looking at others reported in eye-tracking research with pictures and videos of humans (for reviews on this topic from different perspectives, see Holleman, Hooge et al., Citation2020; Kingstone, Citation2009; Risko et al., Citation2012, Citation2016). In particular, researchers have investigated to what degree gaze behaviour to another person, particularly their face, depends on whether the other person can (or is believed to be able to) interact with them or not (Gobel et al., Citation2015; Gregory & Antolin, Citation2019; Holleman, Hessels et al., Citation2020; Macdonald & Tatler, Citation2018). Such studies attest to the relevance of the putative dual function of gaze for understanding gaze behaviour in various contexts, although they do not yet reveal how humans may balance perceptual ( “information-gathering ”) and communicative ( “information-signalling”) functions in face-to-face interactions.
Clearly, a substantial part of human behaviour may be characterized both as sequential action and as interaction with others, such as collaborative work on oil drilling rigs or in aircraft cockpits.Footnote2 In such situations, each person may complete their own tasks, monitor the progress of others, communicate with others, and adjust their own plans accordingly. How humans achieve this has received substantially less attention than the sequential action of an individual agent, at least in the individual cognitivist tradition (cf. Marsh et al., Citation2006, Citation2009). As Hayhoe and Ballard (Citation2014) write in their review of the task-control of gaze: “The focus […] has been on endogenous attentional control of tasks, but a complete story has, in addition, to account for exogenous stimuli that can change the agent's agenda” (p. R628). In other words, the focus by Hayhoe and Ballard (Citation2014) is on the spatiotemporal relation between gaze location in the world and the sequential actions carried out by an individual person while completing one or more clearly defined tasks, for example making tea or sandwiches. Less attention has been paid to how something external to these tasks can change the behaviour of the individual. One category of such “exogenous stimuli” that may change the individual's agenda is another individual's actions or attempts at communication. Thus, in collaborative work, the actions of each person may affect what the other can or must subsequently do, and how gaze is allocated as a result.
In the present study, we investigate the relation between task performance, communication and gaze behaviour to faces and objects in a face to face dyadic collaborative task, i.e., two people copying a Duplo model together (based on Ballard et al., Citation1995). When an individual completes this model-copying task, (s)he does so in a serial fashion and makes saccades to the relevant areas (model to copy, individual blocks to place, and the workspace) just before a manual action is carried out there (Ballard et al., Citation1995). In serial tasks like the model-copying task, most fixations are assumed to be related to immediate manual actions required for completing the task (e.g., Land et al., Citation1999; Pelz & Canosa, Citation2001). Thus, in order to predict an individual's gaze behaviour during task execution, one needs a good model of how the task ought to be executed. According to Hayhoe and Ballard (Citation2005), in the Duplo model-copying experiment, “the task structure is evident” (p. 189). Would one expect the task structure to be different in a dyadic version of the same task, i.e., two people copying the Duplo model together? Intuitively perhaps not, unless dyads coordinate the way in which they complete the task. However, previous research on collaboration and communication (e.g., Clark & Brennan, Citation1991) suggests that dyads do coordinate, and the manner in which they do so depends on e.g., the shared visual information (Gergle et al., Citation2004, Citation2013) and modes of communication (Wang et al., Citation2017). Moreover, such coordination may take time (see e.g., Brennan et al., Citation2008). The question here is when and whether such coordination occurs and how it affects task execution and gaze allocation during the dyadic collaboration. In essence, we ask to what degree the visual routines theory (specifically the task-control of eye movements) can usefully be applied to dyadic task execution. This touches upon the theoretical divide between individual cognitivist and embedded/embodied perspectives (cf. Marsh et al., Citation2006, Citation2009). Practically, moreover, knowledge on the relation between gaze behaviour, sequential action and communication in collaborative interactions may be particularly useful for the development of e.g., anthropomorphic virtual avatars and social robots (see e.g., Huang & Mutlu, Citation2012; Ruhland et al., Citation2015).
To answer our research question, dyads were asked to copy a Duplo model together as accurately and fast as possible. We varied (1) whether all Duplo blocks in a model were visible to both participants in a dyad or not and (2) whether verbal communication was allowed or not. What may be expected of the participants' behaviour under these different conditions? When all Duplo blocks are visible to both participants in a dyad, there may be no need for any (verbal) communication to correctly copy the model. Both participants can see which blocks have already been placed, which blocks still need to be placed, and which block the other person might currently be placing. When verbal communication is allowed, the two individuals may either act in a solitary fashion, or they may verbally coordinate and agree on a joint strategy. Crucially, when not all Duplo blocks are visible to both participants, some form of coordination is required, which may take time (see e.g., Brennan et al., Citation2008). Each individual in the dyad is dependent on the other for placing at least a number of the Duplo blocks. Here, we might expect that performance is impaired (longer completion times and/or more errors) when verbal communication is not allowed. Crucially, should we observe differences in gaze behaviour across the four conditions, we may conclude that the manner in which the Duplo model-copying task is executed depends on whether visual information is shared (i.e., all blocks are visible to both participants) and what modes of communication are allowed. This conclusion is based on the assumption that gaze behaviour is tightly coupled to task execution (as Hayhoe & Ballard, Citation2005, Citation2014, strongly suggest), and thus the task execution must differ. If so, modelling task execution requires more than a description of the dyadic task, but also knowledge of whether visual information is shared and what communication opportunities exist. We discuss whether this is feasible in an extended visual routines theory, or whether the visual routines theory (specifically the task-control of eye movements) may be less applicable to dyadic tasks than the sequentially-executed tasks of an individual.
Methods
Participants
Participants were recruited at the Faculty of Social and Behavioural Sciences of Utrecht University and through the network of the first two authors. 46 participants (22 female, 23 male, 1 unspecified) completed the experiments, arranged in 23 dyads (12 female-male, 5 female-female, 5 male-male dyads, 1 male-unspecified). Dyads were arranged such that participants did not know each other well and spoke the same language (English or Dutch). Mean age was 23.1 years ( years, range [18, 31] years). The mean age difference between the participants in each dyad was 2.9 years (
years, range [0, 7] years). Four dyads (3 female-male, 1 female-female) had to be excluded (see below). After exclusion, mean age was 23.3 years (
years, range [19, 31] years). The mean age difference between the participants in each dyad was 2.8 years (
years, range [0, 7] years).
Most participants (n = 36) reported normal or corrected-to-normal vision with contact lenses. However, the wearable eye trackers could not be worn simultaneously with regular glasses, which therefore had to be removed. Another 9 participants reported sometimes wearing glasses or actually needing glasses, but with minor correction only (between +0.75 and −1.5 diopter). One participant normally wore glasses at −3.5 diopter. When assessing his vision for the experiments (asking him to identify the colour and number of a few blocks), no hindrance was reported by the participant nor observed by the experimenters.
All participants gave written informed consent prior to the start of the experiment, which was again confirmed upon completion of the experiments. Participants were compensated for their time with either course credit or money (€2 per 15 min). The experiment took approximately 30 minutes. The study was approved by the Ethics Committee of the Faculty of Social and Behavioural Sciences of Utrecht University (protocol number 22-0206).
Setup & apparatus
Each participant was seated in an office chair on one side of a cm table. As Figure depicts, the table was divided into three areas. One area was reserved for the model to be copied by the dyad (Model), one area contained the individual Duplo building blocks (Blocks), and the centre of the table contained a plate on which the model was to be built (Build area).
On the table, 26 fiducial markers (ArUco markers, dictionary size , Garrido-Jurado et al., Citation2014) were placed to allow mapping of the gaze position in the scene camera's coordinate system to the coordinate system of the table. Each marker on the table measured
cm. Each fiducial marker used was unique, i.e., those on the table and those used to signal trial start and stop (see section Procedure).
Each participant was fitted with a Pupil Invisible eye tracker (Pupil Labs, Tonsen et al., Citation2020) connected to a OnePlus 8 running Android (Oxygen OS 11.0.7.7.IN21BA) and the Pupil Invisible companion application (version 1.4.23-prod). The Pupil Invisible is a calibration-free eye tracker that can record gaze at 200+ Hz (empirically determined measurement frequencies are given below). The scene camera of the eye tracker recorded video at 30 Hz ( pixels, 82
by 82
field of view). The exposure was set to a value of 100 to minimize motion blur, which is problematic for detection of the fiducial markers.
To ensure a recording in which both participants and the puzzle area were visible, a Logitech BRIO 4K Stream Edition webcam (version 1.0.40) was mounted approximately 2 m above the table. We refer to this camera as the top view camera. It recorded a full HD video (1920 by 1080 pixels) at 30 Hz. The webcam was controlled by the Logitech Capture software (version 2.06.34) running on a MacBook Pro (macOS 10.14.6). Autofocus was set to a value of 0, white balance to a value of 3008, and field of view to 65. The first two recordings were recorded at 60 Hz. However, due to visually noticeable jitter during the second recording (see also section Data quality & exclusion), we switched to 30 Hz. The top view camera and the two eye tracker scene cameras included audio recordings.
Task
Participants were instructed that they would collaboratively copy a Duplo model in front of them. This was to be achieved as quickly and accurately as possible. The Duplo model could not be rotated or disassembled. Participants were allowed to move their bodies and heads to get a closer look, as long as they did not get up from their chair or move around the table. Once participants felt they copied the model accurately, they were to notify the experiment leader verbally.
The experiment began with a practice trial to familiarize participants with the procedure. During the practice trial, participants copied a Duplo model as they would during the experimental trials. However, the practice model consisted of only 11 blocks and was easier to complete then all subsequent Duplo models. Hereafter, 8 experimental trials were conducted, during which a Duplo model had to be copied. Each Duplo model consisted of 26 individual Duplo blocks, varying in colour (yellow, red, blue, green) and shape (standard ,
and
Duplo blocks). Half of the models were constructed such that all blocks were visible from each participant's perspective. Most blocks were placed on the plate, with a few blocks (approximately 5) on top of other blocks, creating a two-tier model (see Figure A). The other half of the models were constructed such that some blocks were hidden from each participant's view (see Figure B). These models were 3-tiered.
Figure 2. Schematic example of (A) a model where are blocks are (partly) visible to both participants, and (B) a model where some blocks are fully hidden from view for each participant. All schematics of the Duplo models used can be found at https://osf.io/2mc8p/.
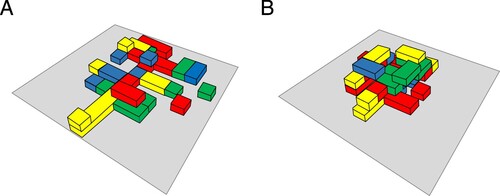
For brevity, we will henceforth refer to the models allowing shared visual information as “visible” models, as all blocks are at least partly visible to both participants. Note also that participants could move their head to get a better look at the partly occluded blocks. The models in which some blocks are fully hidden from each participant's view are referred to as “hidden” models. We expected the visible models to be easier to complete than the hidden models. The different versions of the hidden and visible models were constructed such that we expected them to be of equal difficulty.
For half of the trials, participants were instructed that they could not talk or otherwise communicate verbally with each other. They were allowed to point and/or attract each other's attention in other ways.
Thus, 4 of the 8 different models were “visible” (all blocks visible to both participants), 4 “hidden” (some blocks hidden from each participant's view). Of each of those 4, 2 allowed all communication, and 2 restricted verbal communication. Every other trial participants switched from verbal communication allowed to verbal communication not allowed. Every set of two trials consisted of visible or hidden models. Trial types were counterbalanced in such a way that a dyad began with one of the four possible combinations of model type and verbal communication.
The building materials available to participants consisted of the 26 blocks needed to recreate the model, and 8 additional blocks.
Procedure
When participants entered the lab, written informed consent and demographic information was acquired. Hereafter, instructions were given regarding the overall procedure. The experimenter fitted the participants with the Pupil Invisible eye tracker and checked whether gaze could be recorded.
While one may expect that a wearable eye tracker delivers gaze data that is synchronized to the scene camera video, this need not be the case and should be empirically verified (see e.g., Hooge et al., Citation2022; Matthis et al., Citation2018). In order to check and, if needed, correct the synchronization of the eye-tracking data and the eye tracker scene video, we asked participants to continuously fixate a 0.5 cm white dot on a 10 cm green disk placed in front of them, while nodding yes and no 5 times each (see Hooge et al., Citation2022, for an elaborate description of the procedure). When nodding one's head, the fast vestibulo-ocular reflex counterrotates the eyes in the head to maintain fixation in the world with virtually no latency. If there is a temporal offset between the gaze signal and the scene camera video, it should be evident during this procedure. Any offset is erroneous and is removed by shifting the gaze position signal in time.
Hereafter, an audiovisual transient (1000 Hz tone and visual transient from black to coloured screen) was presented to all cameras (eye tracker scene cameras and top view camera) by means of a digital clapperboard app.Footnote3 This allowed us to synchronize recordings of the three cameras post-recording.
Each trial in the experiment was marked by a paddle containing a unique fiducial marker on each side. This paddle was placed in front of participants on the table. Participants were instructed to always face the paddle when it was on the table such that the fiducial marker was visible in the eye tracker scene videos (in addition to being visible in the top view camera). As each marker could be automatically detected in the videos, we could find the start and end of a trial automatically by detecting the occurrence of the specific markers on the paddle. A trial start was marked by flipping the paddle from one side to the other exposing the two unique fiducial markers in quick succession to all three cameras. Trial stops were marked in a similar fashion, but now by flipping the paddle in the reverse order. Thus, the presence of fiducial marker 1 followed by the presence of marker 2 in all three cameras indicates trial start. The presence of marker 2 followed by the presence of marker 1 indicates trial stop.
After the experiment was completed, the synchronization procedure was repeated. That is, participants fixated the white dot while nodding yes and no 5 times, and the audiovisual transient was presented to all cameras.
Data processing & analysis
Figure depicts a flowchart of the data processing steps for the current study. First the two Pupil Invisible (step 1) eye trackers and scene cameras were synchronized (step 2), using the procedure described in Hooge et al. (Citation2022). In brief, we automatically detected the position of the green disk in the eye tracker scene camera video. For each synchronization episode, we plotted the position of the green disk in the scene camera video and the gaze position in the scene camera video. As the participant maintains fixation on this disk, the two should coincide, given a maximum delay of 10 ms due to the latency of the vestibulo-ocular reflex. We then manually shifted the gaze position signal until it overlapped approximately with the position of the target. The error of this synchronization procedure is expected to be around 10 ms (for more details, see Hooge et al., Citation2022). Gaze and target signals were manually aligned by author RH.
Figure 3. Signal processing flowchart. Eye tracker signals and scene camera images are combined with known locations of ArUco markers and a top camera video (left-hand side of flowchart) to produce Area-of-Interest based measures on the table or face, paired gazed states, a gaze-gaze distance, and a gaze-overlaid movie (right-hand side of flowchart). See main body for description of the individual processing steps.
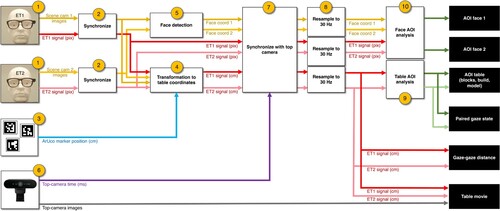
Using the array of ArUco markers on the table with known size and spatial configuration (step 3), gaze was mapped to the table (step 4). Specifically, it was performed using part of the code from the GlassesValidator tool for wearable eye trackers (Niehorster et al., Citation2023). For each frame of each eye tracker's scene camera video, this method determines where the scene camera is located and how it is oriented with respect to the table. To determine the gaze position on the table in the table's reference frame, this information together with the calibration of the scene camera provided by Pupil Labs is then used to turn the gaze position on the scene video into a 3D ray in the table's reference frame, and gaze position on the table is determined as this ray's intersection with the plane of the table.
To allow automatic mapping of gaze to faces, we ran face detection on the scene camera videos of both eye trackers (step 5). The bounding box around the face in each scene camera was located with the cvzone library (https://github.com/cvzone, git revision a6d0d6d), building on MediaPipe (https://mediapipe.dev/).
We take the start of the top view camera as the reference time point (step 6); all cameras are synchronized to that camera (step 7). For all three cameras (top view and two eye tracker scene cameras), author RH and MT determined the first frame in which the first visual transient (clapperboard) was visible. The offsets between the eye tracker scene camera timestamps and the top view camera are then determined. The second visual transient was later used to assess the reliability of the synchronization. Gaze coordinates and face coordinates where then resampled to the top view camera sampling frequency (30 Hz) using standard MATLAB routines (step 8).
We assigned gaze coordinates on the table to three Areas of Interest (AOIs): the block selection area, the build area, and the model (step 9). AOIs encompass the full width of the table (80 cm). The length of the table is split into the AOIs as follow: 0–52 cm for the block selection area, 52–104 cm for the build area, and 104–160 cm for the Duplo model. When gaze is not on the table, we determine when it is on the face of the other (step 10). Gaze was mapped to the face AOI when it does not exceed 150 pixel from the centre of the bounding box around a face in the eye tracker scene camera image. All gaze not on one of the table AOIs nor on the face was assigned to the “none” AOI.
Gaze measures
We report a number of different individual and pair-based gaze measures, using the terminology by Holmqvist et al. (Citation2011). First we describe the total dwell time in seconds on the various AOIs. The distribution of total dwell times over the AOIs may reveal which areas need to be looked at most in order to successfully complete the Duplo-copying task. We specifically use the total dwell time measure instead of e.g., number of fixations on each AOI, as the total dwell times for large AOIs are not very susceptible to imprecision of the gaze position signal or short bursts of data loss (Hessels et al., Citation2016), while the number of fixations and corresponding average fixation duration are (Hessels et al., Citation2017). As the transformation of the gaze position from the scene camera image to the table coordinate system was not possible when the head moved substantially (causing motion blur in the image), short bursts of data loss in this gaze position signal were common.
Total dwell time was calculated as the number of samples (i.e., frame of the top view camera) an individual looks at an AOI, after transformation of the gaze position in the scene camera image to the table coordinate system, multiplied by the inter-sample interval of the top view camera (33 ms). As the trial durations may differ substantially, we also express the total dwell time as a proportion of the trial duration. To clarify the nature of the difference between the absolute and relative total dwell time, consider e.g., a hidden model that takes twice as long to complete as a visible model. If on both trials participants look equally long in absolute terms at the building material for picking up blocks, this will be reflected in a much smaller relative total dwell time for the hidden model than for the visible model, as it is relative to a longer trial duration. In this case, the absolute total dwell times and the relative total dwell times give qualitatively different insights into the gaze behaviour.
To gain insights into the sequential nature of gaze behaviour during the Duplo copying-task, transitions between AOIs were considered for the three table AOIs (model, build area, and blocks). Transitions were operationalized as dwells of at least 0.1 s on one of the table AOIs followed by a dwell on another table AOI after maximally 0.3 s. The relatively long gap of 0.3 s was because head movements often temporally prevented gaze from being mapped to the table due to motion blur in the scene camera, thus yielding brief bursts of data loss.
Finally, we investigated the combination of the two participants gaze locations, which we refer to as paired gaze states. The time spent in each possible paired gaze state (e.g., both look at model, or one participant looks at model while the other looks at the build area), was computed as the number of samples in a state multiplied by the inter-sample interval of the top view camera (33 ms). Total dwell time for each paired gaze state was also expressed as a proportion of the trial duration.
Analysis of speech
In order to contextualize the differences in gaze behaviour between the hidden and visible models with respect to the overall amount of verbal communication, we annotated when participants spoke to each other. For this analysis we only considered the first trial containing a visible model and the first trial containing a hidden model. As the duration of each model-copying trial may vary between conditions (visible versus hidden models) but also from dyad to dyad we sought a measure that we could compare across all trials. We therefore only annotated the first minute of each trial (each of these trials took at least 60 s).
We annotated one of the scene camera audio streams (using ELAN 6.4, The Language Archive, Citation2022) for when each participant spoke and what they said. In some cases, participants spoke at the same time, or it was difficult to hear what they said over the sound of the Duplo blocks being placed. We therefore estimated the amount of verbal communication by the total time that either participant spoke. Annotations were done independently by authors RH and MT, using only the audio stream such that the annotator could not see which model was being copied. The results below are based on annotations of author RH, but the overall pattern and conclusions are identical when using the annotations of author MT.
Statistical analysis
For the statistical analyses, we take a two-pronged approach as in our previous studies (e.g., Hessels et al., Citation2022, Citation2021). For group averages, we report the Harrell-Davis estimated median and use nonparametric bootstrapping to compute 95% confidence intervals around the median. We do this with the decilespbci MATLAB function provided by Rousselet et al. (Citation2017), with the number of bootstrap samples set to the default value of 2000. We supplement these descriptives with Bayesian statistical analyses conducted in JASP 0.16 (JASP Team, Citation2021). We use the notations for Bayes Factors as implemented in JASP. Bayes Factors represent the evidence in favour of a statistical hypothesis given the data. The higher the value, the more evidence in favour. In the case of a Bayesian t-test, for example, values above 3 are considered moderate evidence for the alternative hypothesis (means are not equal), while values above 10 indicate strong evidence for the alternative hypothesis. For more detailed interpretation of the values we refer the reader to Table 1 in Schönbrodt and Wagenmakers (Citation2018).
More specifically, we conduct Bayesian analyses of variance (ANOVAs) on the Duplo model-copying performance, individual measures of gaze behaviour, and paired measures of gaze behaviour. We determine whether the model including the interaction of model type (visible/hidden) and mode of communication (talking allowed/no talking allowed) is best supported by the data. We then either conduct all relevant pairwise comparisons if they are essential to our research questions or use robust graphical means (Rousselet et al., Citation2017) to select specific pairwise comparisons to quantify statistically. We believe this approach is more conservative than putting all possible pairwise comparisons through additional statistical analyses, and follows recent advice made by Brenner (Citation2016).
Eye-tracking data quality
We follow the guidelines by Holmqvist et al. (Citation2023) that eye-tracking data quality should be reported in every eye-tracking study. To this end, we assessed (1) the effective frequency of the eye tracker and (2) the accuracy and precision of the gaze position data.
As the Pupil Invisible always reports a gaze coordinate (i.e., no data loss occurs), the effective frequency is a better measure for data loss than e.g., a proportion of lost samples (see Hooge et al., Citation2022, for an elaborate discussion of this measure). The effective frequency was determined as the average measurement frequency of the eye tracker throughout an entire recording.
The accuracy was computed as the angular distance between the centre of the green disk and the gaze position for a 1 s window during the first synchronization episode. The 1-second window was set manually by author RH such that the least amount of head movement occurred in the window. This decision was made on the basis of the gaze position signal in the scene camera video and marked using a custom MATLAB script. Using camera calibration parameters of the eye tracker scene camera, the position of the green disk and the gaze position in the scene camera image were transformed to directions and the median angular offset was then estimated.
Precision was operationalized as the root mean square (RMS) sample to sample distance of the gaze position on the table. More specifically, we report the median RMS deviation of a 300 ms moving window slid over the entire recording. As our analyses are about where people look on the table and participants may move closer and further from the table surface, we report the precision in millimetres on the table instead of the more common angular RMS deviation (see e.g., Holmqvist et al., Citation2023).
Results
Data quality & exclusion
Data from 4 out of the 23 dyads had to be excluded. Data from two dyads were excluded as the top view camera was set to 60 Hz, which led to noticeable jitter in the video and we therefore did not trust the analysis of the top view video. For another pair, the recording application of the eye trackers failed after commencing recording. There was no data saved for either participant. The last excluded pair had an eye tracking recording that failed halfway through the experiment. For 2 out of the remaining 19 dyads, one eye tracker failed during the last trial. Only data from the first 7 trials of these dyads are therefore considered. For all analyses reported below, data from these 19 dyads were considered.
We first describe the quality of the synchronization between the three cameras, and between the eye trackers (specifically the gaze position data) and their respective scene cameras. For the 17 dyads for which all trials were recorded with the top view camera and both eye trackers, we assessed the reliability of the camera synchronization by comparing the time shift between scene camera and top view camera between the first and second synchronization episode. The mean offset was 32.97 ms ( ms). Thus, the synchronization of the three cameras was reliable to approximately one frame of the top view camera (33 ms at 30 Hz). For the 17 complete dyads and the two participants from the remaining incomplete dyads (last trial missing for one participant) we likewise assessed the reliability of the synchronization of eye tracker to scene camera. For these 36 participants the mean time shift between eye tracker and scene camera was 14.47 ms (
ms, range [0, 30] ms) at the first synchronization episode. The mean offset between the first and second synchronization episode was 1.39 ms (
ms, range [−20, 20] ms).
The mean effective frequency of the eye trackers across all participants was 219.23 Hz (range across participants [217.26, 225.21] Hz), and 30.83 Hz for the scene cameras (range [30.72, 30.94] Hz). Thus, all eye trackers and eye tracker scene cameras recorded as specified by the manufacturer (or at higher frequencies). The mean spatial precision across all participant-trial combinations for gaze position on the table was 19.17 mm ( mm, range [9.79, 44.28] mm). An RMS deviation of 44 mm is well within the AOI sizes on the table (at least 500 mm). Finally, we found a mean inaccuracy of 4.48
(
, range [0.86, 10.39]
). A maximum inaccuracy of 10
might seem substantial: For a Full-HD 23
monitor at 57 cm distance, a 10
inaccuracy covers approximately 20% of the screen. However, in our experiment, the AOIs on the table were at least 50 cm in width. At the 50–60 cm distance that participants were seated from the centre of the AOIs, the AOIs encompassed approximately 45- 50
. Moreover, while completing the model-copying task, people often moved their head to around 30 cm distance from the table. At this distance, our 50 cm AOIs encompassed about 80
. Thus, for the analysis of where people looked on the table, these inaccuracies are not problematic. Only for the face AOI, the relatively large inaccuracy may be problematic. However, very little looking at faces occurred during the puzzle copying (see below), even for participants with very low inaccuracies. Given the above, no participants or pairs were excluded further.
Model-copying performance
Figure depicts the average completion time and number of errors for the four different conditions: (1) visible models when talking is not allowed, (2) visible models when talking is allowed, (3) hidden models when talking is not allowed, and (4) hidden models when talking is allowed. An error was defined as (1) using one block too few, (2) using one block too many or (3) having interchanged one block with another (e.g., a green one where a red one should be). The number of errors per condition was computed as the sum of these three occurrences, and then averaged across the two trials in a condition. The left panel depicts much longer completion times for the hidden models than for the visible models, which indicates that the hidden models were more difficult to copy. Contrary to our expectations, there does not seem to be a difference in completion time between the talking and no talking conditions, not even for the hidden models. This was confirmed by a Bayesian repeated-measures ANOVA. The statistical model best supported by the data included only the model type term, not the term for mode of communication nor the interaction term (). The number of errors was low overall and there does not seem to be a systematic difference between the conditions. A Bayesian repeated measures ANOVA revealed that the best statistical model only included the model type term (
). This suggests that number of errors might have been a bit higher for the hidden models. Thus, there is no evidence for any speed-accuracy trade-off.
Figure 4. Average completion time (left panel) and number of errors (right panel) for the four different conditions in the present experiment. Square green markers depict the Harrell-Davis estimated medians for the hidden models, triangular purple markers for the visible models. Dark purple or dark green reflect the conditions where talking is not allowed, light purple or light green reflect the conditions when talking is allowed. The error bars represent bootstrapped 95% confidence intervals of the median. Circular grey markers represent data from individual dyads.
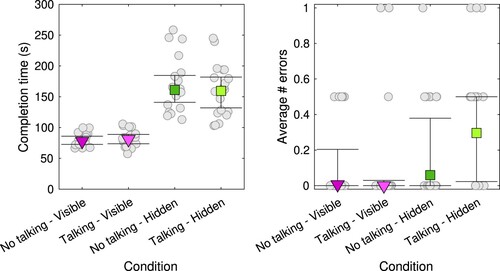
Where did individuals look?
Where did individuals look as they completed the model copying task? Panels A and B in Figure depict the (relative) total dwell time for the blocks, build area and model AOIs on the table, and the none AOI (not on the table, nor on the face). The face AOI is not depicted, as less than 0.5% of total time was spent looking at the face. As can be seen in panel A, participants spent more time looking at the build area, model, and none AOI for the hidden models compared to the visible models. The time spent looking at the blocks seems equal across all conditions. What does this mean? We interpret the roughly equal time spent looking at the blocks (in absolute terms) across conditions as evidence that looking at the blocks in the selection area is only required for finding the right piece to place on the model: The number of pieces to place is identical across visible and hidden models. The longer time spent looking at the build area and model indicate that participants took longer to determine what block to place and whether the model was copied correctly. The none AOI captures all gaze not on the table nor on the face of the other person. This happens e.g., when participants gaze at task-irrelevant areas in the room, but also when participants make a large head movement and the ArUco markers cannot be detected to map the gaze to the table. As such, it can be seen as a measure of task-irrelevant gaze and data loss, which is expected to scale with the length of the recording. Indeed, the proportion of total dwell time on the none AOI is roughly equal across all conditions (panel B).
Figure 5. Individual measures of gaze behaviour. Total dwell time (panel A) and proportion of total dwell time (panel B) on the blocks, build area, model, and none Area of Interest. Number of transitions (panel C) and proportion of transitions (panel D) between the blocks, build area, and model Area of Interest. Bars represent Harrell-Davis estimated medians for the four different conditions. Green represents the hidden models, purple the visible models. Dark purple or dark green reflect the conditions where talking is not allowed, light purple or light green reflect the conditions when talking is allowed. The error bars represent bootstrapped 95% confidence intervals of the median.
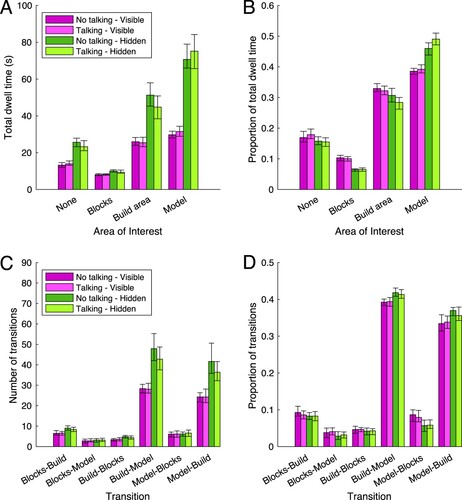
Statistical analyses back up our interpretation of these results. Bayesian repeated measures ANOVAs with AOI (none, blocks, build, model, face), model type (visible, hidden) and talking (allowed, not allowed) were conducted on the total dwell time and proportion of total dwell time. For total dwell time, the best statistical model included the AOI term, model type term, and AOI x model type interaction term (). For the proportion of total dwell time, the same model was best (
). Thus, (relative) total dwell time differ as a function of AOI, model type, and the interaction of AOI and model type.
Why did participants spend more time looking at the build and model area? It can be that individual steps (checking the model, placing the block at the right location) take longer. It may also be that participants switch back and forth more between model and build area to determine what to place and where, or to check whether blocks have been placed correctly. The latter should be evident from transitions between the AOIs on the table. Panels C and D in Figure depicts the number of transitions and proportion of transitions between the AOIs on the table. As can be seen in panel C, more transitions between the build and model area occurred (in both directions). This also led to slightly higher proportions for these transitions (panel D). Bayesian repeated measures ANOVAs with transition (six possibilities), model type (visible, hidden) and talking (allowed, not allowed) were conducted on the number and proportion of transitions. For both measures, the best statistical model was one which included the transition term, model type term, and the transition x model type interaction term: for the number of transitions;
for the proportion of transitions.
We conclude that participants spend longer looking at the build and model area for hidden models, and make more transitions between these areas in order to determine what block to place or to check whether blocks have been placed correctly. Selecting the block from the selection area does not seem to differ as a function of model type or mode of communication. There does not seem to be any difference in participants’ gaze behaviour as a function of the mode of communication.
Did dyads coordinate?
Although no differences in individual measures of gaze behaviour were observed as a function of mode of communication, it may be evident only for pair-based measures of gaze behaviour. For example, if participants coordinated their behaviour verbally, this might be evident in a smaller distance between their gaze position on the table when they could talk compared to when they could not talk. Therefore, we computed the median distance in mm between the gaze positions for each frame of the top view camera for which both participants had a gaze position on the table.
The left panel in Figure depicts the median distance between the gaze positions of the two participants as a function of condition. This distance seems smaller for the hidden models, and particularly so for the hidden models when talking is allowed. We therefore computed the per-pair difference in the median gaze-gaze distance between the talking and no talking conditions for the hidden model, which is depicted in the right panel in Figure . As can be seen, the median distance is smaller when talking is allowed (median −49.01 mm, 95%CI [−75.63, −7.26 mm]).
Figure 6. Median distance between the gaze position on the table of the two participants as a function of condition (left panel). Square green markers depict the Harrell-Davis estimated medians for the hidden models, triangular purple markers for the visible models. Dark purple or dark green reflect the conditions where talking is not allowed, light purple or light green reflect the conditions when talking is allowed. The error bars represent bootstrapped 95% confidence intervals of the median. Circular grey markers represent data from individual dyads. The right panel depicts the per pair difference (grey markers) and the group median difference with bootstrapped confidence intervals (black square marker).
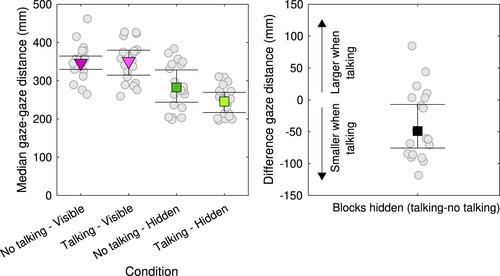
A Bayesian repeated measures ANOVA on the distance between the gaze positions revealed that the best statistical model included the model type (visible, hidden) term, talking (allowed, not allowed) and the interaction term (). Subsequent Bayesian paired-samples t-tests indicated evidence for differences in the median gaze distance only for the hidden model (
), but not for the visible model (
). We interpret this as evidence for more coordination between participants when talking is allowed, but only for the hidden model not for the visible model.
Another way of looking at coordination between participants, is to look at the total time spent in each area on the table by the two participants at the same time. Figure depicts the total time (left panel) and relative total time (right panel) that dyads spent in these paired gaze states. When looking at the proportion of total dwell time (right panel), it seems that the time that both participants look at the model at the same time is higher for the hidden models than for the visible models, and particularly so when talking is allowed. We therefore visualized this in more detail in Figure . As can be seen in the left panel, indeed the proportion of time that both participants spent simultaneously looking at the model is higher for the hidden models. The right panel shows that this is more so when talking is allowed. The median difference in the proportion of time both participants look at the model between talking and no talking for the hidden model was 0.034 (95%CI [0.015, 0.055]).
Figure 7. Total dwell time (left panel) and proportion of total dwell time (right panel) for the paired gaze states on the table (AOI combination of the two participants). Bars represent Harrell-Davis estimated medians for the four different conditions. Green represents the hidden models, purple the visible models. Dark purple or dark green reflect the conditions where talking is not allowed, light purple or light green reflect the conditions when talking is allowed. The error bars represent bootstrapped 95% confidence intervals of the median.
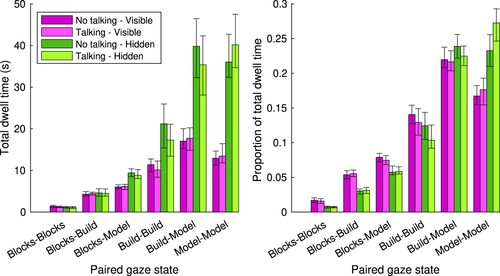
Figure 8. Proportion of total time during which both participants look at the Duplo model as a function of condition (left panel). Square green markers depict the Harrell-Davis estimated medians for the hidden models, triangular purple markers for the visible models. Dark purple or dark green reflect the conditions where talking is not allowed, light purple or light green reflect the conditions when talking is allowed. The error bars represent bootstrapped 95% confidence intervals of the median. Circular grey markers represent data from individual dyads. The right panel depicts the per pair difference (grey markers) and the group median difference with bootstrapped confidence intervals (black square marker).
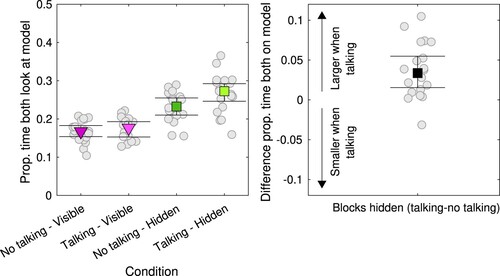
A Bayesian repeated measures ANOVA on the proportion both participants spent looking at the model revealed that the best statistical model again included the model type (visible, hidden) term, talking (allowed, not allowed) and the interaction term (). Subsequent Bayesian paired-samples t-tests indicated evidence for differences in the proportion of time both participants look at the model for the hidden model (
), but not for the visible model (
). Thus, coordination of behaviour between participants seems to occur when participants can talk, and is evident in the time that they both look at the Duplo model to be copied.
Coordination through verbal communication?
The differences in the pair-based measures of gaze behaviour between the talking and no talking conditions for the hidden models suggest that coordination is (partly) achieved through verbal communication. If that is the case, the amount of speech or the content of the speech should differ between the hidden and visible model when talking was allowed.
Figure depicts the total time that either participant is speaking for the visible and hidden model. Indeed, dyads spoke less while completing the visible model (median 22.70 s, 95%CI [15.26, 27.32] s) than while completing the hidden model (median 29.54 s, 95%CI [23.83, 34.78] s). The per-dyad difference in time spent talking between hidden and visible model was 7.90 s (95%CI [3.49, 11.73] s). This was corroborated by a Bayesian paired-samples t-test, which indicated strong evidence for differences in the time spent talking between the visible and hidden model ().
Figure 9. Time spent talking during the first minute of each first visible and hidden model while talking was allowed (left panel). Talking is defined as any moment when either of the participants or both speak. The triangular purple and square green markers depict the Harrell-Davis estimated medians for the visible and hidden model, respectively. The error bars represent bootstrapped 95% confidence intervals of the median. Circular grey markers represent data from individual dyads. The right panel depicts the per pair difference (grey markers) and the group median difference with bootstrapped confidence intervals (black square marker).
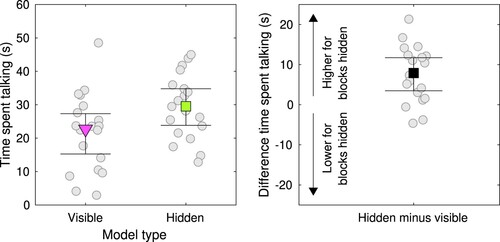
The episodes of speech were also transcribed and qualitatively assessed. Almost all intelligible utterances were about the task, e.g., which block to place, how much space there should be between two blocks, whether a particular block to place was a long or short block. Very few off-task utterances were observed. As dyads spoke more for the hidden models, the coordination of gaze behaviour observed for these models, may have been the result of the verbal communication or at least partly.
Discussion
We investigated the relation between task performance, communication and gaze behaviour in a dyadic Duplo model-copying task (based on Ballard et al., Citation1995). We varied (1) whether all blocks were visible to both participants or not (i.e., whether visual information is shared), and (2) whether verbal communication was allowed or not. Assuming a tight coupling between task execution and gaze behaviour (Hayhoe & Ballard, Citation2005, Citation2014), differences in gaze behaviour between these conditions are indicative of differences in task execution.
Summary of results
Performance was worse for the models in which some blocks were hidden from either participant's view (labeled the “hidden” models for brevity) than for the models in which all blocks were visible to both participants (labeled the “visible” models for brevity). The hidden models took roughly twice as long to complete and more copying errors were made. Performance in the Duplo copying task was not affected by restricting the mode of communication.
Participants looked at the model and build area most of the time, while the collaborators' faces were hardly ever looked at. The latter finding corroborates previous research on dyadic collaboration (Macdonald & Tatler, Citation2018) showing that the collaborators' faces do not receive gaze if not immediately task-relevant, even in dyadic interaction. It should also be noted that we did observe the dyads looking at each other's faces in between experimental trials. This suggests that participants did not actively avoid looking at faces during the experiment, for example because they were aware that their gaze location was recorded (cf. Risko & Kingstone, Citation2011).
Individual aggregate measures of gaze behaviour (total dwell time and number of transitions) did not vary as a function of the mode of communication (i.e., whether talking was allowed or not), but did differ between the visible and hidden models. Specifically, the longer time for the hidden models was spent looking mainly at the build and model area and transitioning between them. Picking blocks from the selection area did not require more time for hidden models than for visible models.
Pair-based measures of gaze behaviour did differ as a function of whether verbal communication was allowed, specifically for the hidden models, and suggested between-participant coordination. The spatial distance between the gaze locations of the two participants was smaller for hidden models than for visible models, and particularly so when talking was allowed. Similarly, the proportion of time that both participants looked at the model simultaneously was larger for the hidden models, and particularly when talking was allowed. Thus, when talking was allowed, dyads solved the Duplo model copying task differently than when talking was not allowed. Crucially, this was only the case when some blocks could not be seen by either participant. However, the altered behaviour did not lead to differences in performance. Coordination of gaze behaviour seemed to be partly related to the verbal communication, the vast majority of which was about the task execution. Note that we did not have the sample size, both in terms of dyads and number of trials per condition, to further investigate the potential correlations between coordination, communication and performance. We conclude that the manner in which the collaborative task was executed depended on both the visual information available to both persons, and if or how communication took place.
Implications
We employed a dyadic version of the Duplo model-copying experiment introduced by Ballard et al. (Citation1995). The reason that we employed this particular task, is that according to Hayhoe and Ballard (Citation2005), “the task structure is evident” (p.189), and very few fixations on task-irrelevant locations are expected (cf. Land et al., Citation1999; Pelz & Canosa, Citation2001). We show that in a dyadic context, the task execution depends on the availability of shared visual information and the mode of communication, which one might expect based on previous research on coordination in communication (e.g., Clark & Brennan, Citation1991; Gergle et al., Citation2004, Citation2013; Wang et al., Citation2017). This raises a number of specific questions.
What are the implications of our findings for modelling dyadic task execution? We consider this question from the perspective of visual routines theory and the task-control of eye movements (henceforth “visual routines theory”; Hayhoe & Ballard, Citation2005, Citation2014). In brief, visual routines theory holds that “fixations are tightly linked in time to the evolution of the task. Very few irrelevant areas are fixated” and “Highly task-specific information is extracted in different fixations” (Hayhoe & Ballard, Citation2005, p.189). In our experiment, individual and paired measures of gaze behaviour did not differ as a function of whether verbal communication was allowed when all visual information was available to both participants (the “visible” condition). Thus, when both participants can work relatively independently of each other, perhaps the task execution need not differ much from the individual situation. Participants only need to consider that another person places some of the blocks and those need not be placed by oneself anymore. It should be noted that verbal communication still occurred when allowed, even when all visual information was shared. Such verbal communication may have been used to agree upon strategies. However, better performance or differences in gaze behaviour were not observed for these visible models when verbal communication was allowed. When visual information was not shared, the mode of communication affected paired measures of gaze behaviour. This suggests that the manner in which the task was executed differed between the situation where verbal communication was allowed from when it was not allowed. Thus, modelling dyadic task execution requires more than a description of the dyadic task (the sequential actions required to copy a Duplo-model), but also knowledge of whether visual information is shared and what communication opportunities exist. This leads us to another question, that is, how does one go from a task description (“copy a Duplo model”) to a model of task execution?
In visual routines theory, the simplifying assumption is that “complex behaviour can be broken down into simpler sub-tasks, or modules, that operate independently of each other, and thus must be attended to separately” (Hayhoe & Ballard, Citation2014, p. R622). For example, the task of copying a Duplo model together with a partner may be considered as a sequence of sub-tasks, including the checking of what block to place, finding the right colour block, placing a block, verifying whether a block is correctly placed, etc., which each may have a corresponding manual or communicative action and gaze location in the world. But how is this task structure, i.e., the sub-tasks and order, arrived at? Hayhoe and Ballard (Citation2014) write about the case of sandwich making (Hayhoe, Citation2000), that “it's possible to infer the underlying task structure very accurately by incorporating the observable data, such as the gaze location, hand position, hand orientation, and image features as well as the prior sequence of states of the task” (p. R626). If an algorithm can automatically identify the sub-task being executed from information about the manual action, the gaze location in the world, and the state of the scene, Hayhoe and Ballard (Citation2014) state that this means we have “a valid model of the task execution” (p. R626). Thus, the task structure is derived from the observable behaviour by annotation. In the case of our dyadic model copying task, deriving a task structure for one participant may require the observable behaviour of both participants. Even in our case, where the task structure for an individual ought to be evident, we saw that the availability of shared visual information and communication opportunities may affect the task execution. One therefore wonders how feasible deriving a task structure is for perhaps less straightforward real-world tasks such as work on an oil platform or in construction, or when the task is less constrained (see e.g., Hessels, Benjamins et al., Citation2020, for a discussion). Yet, such task structures may be necessary components for developing robots that collaborate effectively with humans in e.g., industrial settings (Villani et al., Citation2018; Zhao et al., Citation2020). Our findings highlight that for collaborative tasks, besides the directly observable behaviour, it may be relevant to consider the communicative opportunities and whether information sources are shared between collaborators.
A third question that arises is whether modelling the behaviour of a dyad completing a collaborative model-copying task is best achieved from the perspective of two individual agents or from the perspective of the dyad. This is best addressed in the context of our findings on coordination. We find that for the hidden models verbal communication may be used to coordinate the task execution, as evident from e.g., a smaller distance between the gaze locations of the two individuals. While this may be understood as coordination from the perspective of the dyad, one wonders how it may be understood from the perspective of the individual in the context of visual routines theory. Hayhoe and Ballard (Citation2014) already pointed out that a complete account has, “in addition, to account for exogenous stimuli that can change the agent's agenda” (p. R628). When not all visual information is shared between collaborators, one participant may ask another to elucidate which blocks to place in a location that (s)he cannot see. This can be considered as an exogenous stimulus that changes the priority for what action to complete next. Thus, what is coordination at one level of abstraction (the dyad) may be considered as multiple instances of exogenous attraction of attention and subsequent changes of the current agenda at another level of abstraction (the individual information-processing system).
How does the discussion of individual versus interactive perspectives relate to visual routines theory proper? Visual routines theory made a point about vision being critical for choosing and executing actions, with gaze allocated at each step to pick up highly specific information. The interactive perspective here would be that what information to pickup and when may be decided in the interaction. In other words, an interactive visual routines theory may hold that the task structure is co-constructed by two or more individuals as they interact, constraining what information each may pickup from the environment at each moment in time. Research in other domains of interaction suggests that such an interactive or system perspective may be fruitful. For example, research on dialogue has switched from a purely individualistic to an interactive view, theorizing that dialogue is constructed in interaction through alignment (Pickering & Garrod, Citation2004) or synergy (Fusaroli et al., Citation2014; Fusaroli & Tylén, Citation2016). Likewise, cognition and memory may be considered from the perspective of an entire system of collaborating individuals and the technical system they operate, as opposed to only the individual perspective (see e.g., Hutchins, Citation1995; Hutchins & Klausen, Citation1996, in the context of airline cockpits). More generally speaking, the question is what the right unit of analysis is for describing the behaviour of interest (cf. Hutchins, Citation2010). As Dingemanse et al. (Citation2023) argue, “A fundamental fact about human minds is that they are never truly alone: all minds are steeped in situated interaction […] we benefit from looking beyond single minds toward cognition as a process involving interacting minds” (p. 1). Thus, it seems sensible to pursue an interactive visual routines theory. It should prove useful for understanding task execution and corresponding gaze behaviour in interaction, and may be particularly helpful in describing (spontaneous) leader/follower roles in collaboration (see e.g., Luft et al., Citation2022; Macdonald & Tatler, Citation2018), or how interactants adjust their behaviour to each other over time as they collaborate.
Clearly, substantial empirical and theoretical work remains for the development of an interactive visual routines theory. However, studies on attentional coordination (Pagnotta et al., Citation2020; Richardson & Dale, Citation2005), synchronization during collaborative task execution (Coco et al., Citation2017), and dyadic visual search (Brennan et al., Citation2008; Coco et al., Citation2018; Niehorster et al., Citation2019) may be particularly insightful in what ought to be modelled. We foresee that it may be difficult to develop a theory that predicts behaviour at the level of individual dwells to an AOI. Higher-level measures of gaze patterns as derived from e.g., recurrence quantification analysis (Anderson et al., Citation2013; Coco et al., Citation2021; Pérez et al., Citation2018), or those based on entropy (Allsop & Gray, Citation2014; Hessels et al., Citation2019; Niehorster et al., Citation2019) may be useful in this regard. In addition, investigating the dynamic relation between gaze behaviour and verbal or gestural communication may be necessary to understand how interactants decide which actions to carry out (see e.g., Coco & Keller, Citation2012; Kendrick et al., Citation2023, for studies on the relation between these modes of communication and gaze behaviour), or how communication and coordination may subsequently affect collaborative performance (e.g., Coco et al., Citation2018).
An additional interesting future line of research is the relation between task-related gaze behaviour and psychopathology, personality characteristics, and culture. For example, it has been shown that gaze behaviour in face-to-face interactions may relate to traits of social anxiety and autism (Chen et al., Citation2023; Chen & Westenberg, Citation2021; Hessels et al., Citation2018; Vabalas & Freeth, Citation2016), charisma (Maran et al., Citation2019), and cultural background (Haensel et al., Citation2020, Citation2022). Moreover, in relatively unconstrained situations, stable individual differences in gaze behaviour to other people have been observed (e.g., Hessels, Benjamins et al., Citation2020; Holleman et al., Citation2021; Peterson et al., Citation2016). It would be worthwhile to understand whether and how these may relate to task-related gaze behaviour. Does culture, for example, modulate which visual routines emerge at which point in time during collaborative interaction?
To conclude, we show that task execution in a dyadic model-copying task depends on both the availability of shared visual information and the mode of communication. Our findings are relevant to the modelling of task structure and gaze allocation for dyadic human-human and human-robot collaboration, and attest to the usefulness of integrating research on task-related gaze control with research on communication and grounding and interactive perspectives on dialogue, cognition, and memory. An interactive visual routines theory ought to be pursued.
Acknowledgments
The authors would like to thank Hinaho Ishikawa and Ellen Verbunt for valuable help with earlier pilots.
Disclosure statement
No potential conflict of interest was reported by the author(s).
Additional information
Funding
Notes
1 “Relevant information” is to be understood here in terms of reinforcement learning models on the task-control of eye movements (e.g., Sprague & Ballard, Citation2004; Sprague et al., Citation2007), see Koenderink (Citation2010) for a relevant discussion on the term “information.” It is not immediately clear how “information” in the term “information-signalling” discussed later is to be understood.
2 One finds ample video material illustrating such collaborative work online, just one example being https://www.youtube.com/watch?v=KZxUiFFVEAQ (the real action starts around 1 minute).
References
- Allsop, J., & Gray, R. (2014). Flying under pressure: Effects of anxiety on attention and gaze behavior in aviation. Journal of Applied Research in Memory and Cognition, 3(2), 63–71. https://doi.org/10.1016/j.jarmac.2014.04.010
- Anderson, N. C., Bischof, W. F., Laidlaw, K. E. W., Risko, E. F., & Kingstone, A. (2013). Recurrence quantification analysis of eye movements. Behavior Research Methods, 45(3), 842–856. https://doi.org/10.3758/s13428-012-0299-5
- Argyle, M., & Cook, M. (1976). Gaze and mutual gaze. Cambridge University Press.
- Argyle, M., & Dean, J. (1965). Eye-contact, distance and affiliation. Sociometry, 28(3), 289–304. https://doi.org/10.2307/2786027
- Ballard, D. H., Hayhoe, M. M., & Pelz, J. B. (1995). Memory representations in natural tasks. Journal of Cognitive Neuroscience, 7(1), 66–80. https://doi.org/10.1162/jocn.1995.7.1.66
- Barker, R. G. (1968). Ecological psychology: Concepts and methods for studying the environment of human behavior. Stanford University Press.
- Botvinick, M., & Plaut, D. C. (2004). Doing without schema hierarchies: A recurrent connectionist approach to normal and impaired routine sequential action. Psychological Review, 111(2), 395–429. https://doi.org/10.1037/0033-295X.111.2.395
- Botvinick, M. M., & Plaut, D. C. (2006). Such stuff as habits are made on: A reply to Cooper and Shallice (2006). Psychological Review, 113(4), 917–927. https://doi.org/10.1037/0033-295X.113.4.917
- Brennan, S. E., Chen, X., Dickinson, C. A., Neider, M. B., & Zelinsky, G. J. (2008). Coordinating cognition: The costs and benefits of shared gaze during collaborative search. Cognition, 106(3), 1465–1477. https://doi.org/10.1016/j.cognition.2007.05.012
- Brenner, E. (2016). Why we need to do fewer statistical tests. Perception, 45(5), 489–491. https://doi.org/10.1177/0301006616637434
- Chen, J., van den Bos, E., Karch, J. D., & Westenberg, P. M. (2023). Social anxiety is related to reduced face gaze during a naturalistic social interaction. Anxiety, Stress, & Coping, 36(4), 460–474.
- Chen, J., van den Bos, E., Velthuizen, S. L. M., & Westenberg, P. M. (2021). Visual avoidance of faces in socially anxious individuals: The moderating effect of type of social situation. Journal of Experimental Psychopathology, 12(1), 1–12. https://doi.org/10.1177/2043808721989628.
- Clark, H. H., & Brennan, S. E. (1991). Grounding in communication. In Perspectives on socially shared cognition (pp. 127–149).
- Coco, M. I., Badino, L., Cipresso, P., Chirico, A., Ferrari, E., Riva, G., Gaggioli, A., & D'Ausilio, A. (2017). Multilevel behavioral synchronization in a joint tower-building task. IEEE Transactions on Cognitive and Developmental Systems, 9(3), 223–233. https://doi.org/10.1109/TCDS.2016.2545739
- Coco, M. I., Dale, R., & Keller, F. (2018). Performance in a collaborative search task: The role of feedback and alignment. Topics in Cognitive Science, 10(1), 55–79. https://doi.org/10.1111/tops.2018.10.issue-1
- Coco, M. I., & Keller, F. (2012). Scan patterns predict sentence production in the cross-modal processing of visual scenes. Cognitive Science, 36(7), 1204–1223. https://doi.org/10.1111/cogs.2012.36.issue-7
- Coco, M. I., Mønster, D., Leonardi, G., Dale, R., & Wallot, S. (2021). Unidimensional and multidimensional methods for recurrence quantification analysis with CRQA. The R Journal, 13(1), 145–161. https://doi.org/10.32614/RJ-2021-062
- Cooper, R., & Shallice, T. (2000). Contention scheduling and the control of routine activities. Cognitive Neuropsychology, 17(4), 297–338. https://doi.org/10.1080/026432900380427
- Cooper, R. P., & Shallice, T. (2006). Hierarchical schemas and goals in the control of sequential behavior. Psychological Review, 113(4), 887–916. https://doi.org/10.1037/0033-295X.113.4.887
- Dingemanse, M., Liesenfeld, A., Rasenberg, M., Albert, S., Ameka, F. K., Birhane, A., Bolis, D., Cassell, J., Clift, R., Cuffari, E., De Jaegher, H., C. D. Novaes, Enfield, N. J., Fusaroli, R., Gregoromichelaki, E., Hutchins, E., Konvalinka, I., Milton, D., Ŗczaszek-Leonardi, J., … Wiltschko, M. (2023). Beyond single-mindedness: A figure-ground reversal for the cognitive sciences. Cognitive Science, 47(1), E13230. https://doi.org/10.1111/cogs.v47.1
- Fusaroli, R., Ra̧czaszek-Leonardi, J., & Tylén, K. (2014). Dialog as interpersonal synergy. New Ideas in Psychology, 32, 147–157. https://doi.org/10.1016/j.newideapsych.2013.03.005
- Fusaroli, R., & Tylén, K. (2016). Investigating conversational dynamics: Interactive alignment, interpersonal synergy, and collective task performance. Cognitive Science, 40(1), 145–171. https://doi.org/10.1111/cogs.2016.40.issue-1
- Garrido-Jurado, S., Muñoz-Salinas, R., Madrid-Cuevas, F. J., & Marín-Jiménez, M. J. (2014). Automatic generation and detection of highly reliable fiducial markers under occlusion. Pattern Recognition, 47(6), 2280–2292. https://doi.org/10.1016/j.patcog.2014.01.005
- Gergle, D., Kraut, R. E., & Fussell, S. R. (2004). Action as language in a shared visual space. In Proceedings of the 2004 ACM Conference on Computer Supported Cooperative Work -- CSCW '04, Chicago, Illinois, USA (pp. 487–496). ACM Press.
- Gergle, D., Kraut, R. E., & Fussell, S. R. (2013). Using visual information for grounding and awareness in collaborative tasks. Human – Computer Interaction, 28(1), 1–39.
- Ghiani, A., Van Hout, L. R., Driessen, J. G., & Brenner, E. (2023). Where do people look when walking up and down familiar staircases? Journal of Vision, 23(1), 7. https://doi.org/10.1167/jov.23.1.7
- Gobel, M. S., Kim, H. S., & Richardson, D. C. (2015). The dual function of social gaze. Cognition, 136, 359–364. https://doi.org/10.1016/j.cognition.2014.11.040
- Gregory, N., & Antolin, J. (2019). Does social presence or the potential for interaction reduce social gaze in online social scenarios? Introducing the “live lab” paradigm. The Quarterly Journal of Experimental Psychology, 72(4), 779–791. https://doi.org/10.1177/1747021818772812
- Hadley, L. V., Naylor, G., & Hamilton, A. F. d. C. (2022). A review of theories and methods in the science of face-to-face social interaction. Nature Reviews Psychology, 1(1), 42–54. https://doi.org/10.1038/s44159-021-00008-w
- Haensel, J. X., Danvers, M., Ishikawa, M., Itakura, S., Tucciarelli, R., Smith, T. J., & Senju, A. (2020). Culture modulates face scanning during dyadic social interactions. Scientific Reports, 10(1), 1958. https://doi.org/10.1038/s41598-020-58802-0
- Haensel, J. X., Smith, T. J., & Senju, A. (2022). Cultural differences in mutual gaze during face-to-face interactions: A dual head-mounted eye-tracking study. Visual Cognition, 30(1–2), 100–115. https://doi.org/10.1080/13506285.2021.1928354
- Hanna, J. E., & Brennan, S. E. (2007). Speakers' eye gaze disambiguates referring expressions early during face-to-face conversation. Journal of Memory and Language, 57(4), 596–615. https://doi.org/10.1016/j.jml.2007.01.008
- Hayhoe, M. (2000). Vision using routines: A functional account of vision. Visual Cognition, 7(1–3), 43–64. https://doi.org/10.1080/135062800394676
- Hayhoe, M., & Ballard, D. (2005). Eye movements in natural behavior. Trends in Cognitive Sciences, 9(4), 188–194. https://doi.org/10.1016/j.tics.2005.02.009
- Hayhoe, M., & Ballard, D. (2014). Modeling task control of eye movements. Current Biology, 24(13), R622–R628. https://doi.org/10.1016/j.cub.2014.05.020
- Hayhoe, M. M. (2017). Vision and action. Annual Review of Vision Science, 3(1), 389–413. https://doi.org/10.1146/vision.2017.3.issue-1
- Hessels, R. S. (2020). How does gaze to faces support face-to-face interaction? A review and perspective. Psychonomic Bulletin & Review, 27(5), 856–881. https://doi.org/10.3758/s13423-020-01715-w
- Hessels, R. S., Benjamins, J. S., Niehorster, D. C., van Doorn, A. J., Koenderink, J. J., Holleman, G. A., de Kloe, Y. J. R., Valtakari, N. V., van Hal, S., & Hooge, I. T. C. (2022). Eye contact avoidance in crowds: A large wearable eye-tracking study. Attention, Perception, & Psychophysics, 84(8), 2623–2640. https://doi.org/10.3758/s13414-022-02541-z
- Hessels, R. S., Benjamins, J. S., van Doorn, A. J., Koenderink, J. J., G. A. Holleman, & Hooge, I. T. C. (2020). Looking behavior and potential human interactions during locomotion. Journal of Vision, 20(10), 1–25. https://doi.org/10.1167/jov.20.10.5
- Hessels, R. S., Benjamins, J. S., van Doorn, A. J., Koenderink, J. J., & Hooge, I. T. C. (2021). Perception of the potential for interaction in social scenes. i-Perception, 12(5), 1–26. https://doi.org/10.1177/20416695211040237
- Hessels, R. S., Holleman, G. A., Cornelissen, T. H. W., Hooge, I. T. C., & Kemner, C. (2018). Eye contact takes two–autistic and social anxiety traits predict gaze behavior in dyadic interaction. Journal of Experimental Psychopathology, 9(2), 1–17. https://doi.org/10.5127/jep.062917
- Hessels, R. S., Holleman, G. A., Kingstone, A., Hooge, I. T. C., & Kemner, C. (2019). Gaze allocation in face-to-face communication is affected primarily by task structure and social context, not stimulus-driven factors. Cognition, 184, 28–43. https://doi.org/10.1016/j.cognition.2018.12.005
- Hessels, R. S., Kemner, C., van den Boomen, C., & Hooge, I. T. C. (2016). The area-of-interest problem in eyetracking research: A noise-robust solution for face and sparse stimuli. Behavior Research Methods, 48(4), 1694–1712. https://doi.org/10.3758/s13428-015-0676-y
- Hessels, R. S., Niehorster, D. C., Kemner, C., & Hooge, I. T. C. (2017). Noise-robust fixation detection in eye movement data: Identification by two-means clustering (I2MC). Behavior Research Methods, 49(5), 1802–1823. https://doi.org/10.3758/s13428-016-0822-1
- Hessels, R. S., van Doorn, A. J., Benjamins, J. S., Holleman, G. A., & Hooge, I. T. C. (2020). Task-related gaze control in human crowd navigation. Attention, Perception & Psychophysics, 82(5), 2482–2501. https://doi.org/10.3758/s13414-019-01952-9
- Ho, S., Foulsham, T., & Kingstone, A. (2015). Speaking and listening with the eyes: Gaze signaling during dyadic interactions. PLoS ONE, 10(8), e0136905. https://doi.org/10.1371/journal.pone.0136905
- Holleman, G. A., Hessels, R. S., Kemner, C., & Hooge, I. T. C. (2020). Implying social interaction and its influence on gaze behavior to the eyes. PLoS ONE, 15(2), e0229203. https://doi.org/10.1371/journal.pone.0229203
- Holleman, G. A., Hooge, I. T. C., Huijding, J., Deković, M., Kemner, C., & Hessels, R. S. (2021). Gaze and speech behavior in parent–child interactions: The role of conflict and cooperation. Current Psychology, 42(3), 12129–12150.
- Holleman, G. A., Hooge, I. T. C., Kemner, C., & Hessels, R. S. (2020). The “real-world approach” and its problems: A critique of the term ecological validity. Frontiers in Psychology, 11, 721. https://doi.org/10.3389/fpsyg.2020.00721
- Holmqvist, K., Nyström, M., Andersson, R., Dewhurst, R., Jarodzka, H., & van de Weijer, J. (2011). Eye tracking: A comprehensive guide to methods and measures. Oxford University Press.
- Holmqvist, K., Örbom, S. L., Hooge, I. T. C., Niehorster, D. C., Alexander, R. G., Andersson, R., Benjamins, J. S., Blignaut, P., Brouwer, A.-M., Chuang, L. L., Dalrymple, K. A., Drieghe, D., Dunn, M. J., Ettinger, U., Fiedler, S., Foulsham, T., van der Geest, J. N., Hansen, D. W., Hutton, S., …Hessels, R. S. (2023). Eye tracking: Empirical foundations for a minimal reporting guideline. Behavior Research Methods, 55, 364–416. https://doi.org/10.3758/s13428-021-01762-8
- Hooge, I. T. C., Niehorster, D. C., Hessels, R. S., Benjamins, J. S., & Nyström, M. (2022). How robust are wearable eye trackers to slow and fast head and body movements? Behavior Research Methods.
- Huang, C.-M., & Mutlu, B. (2012). Robot behavior toolkit: Generating effective social behaviors for robots. In Proceedings of the Seventh Annual ACM/IEEE International Conference on Human-Robot Interaction -- HRI '12, Boston, Massachusetts, USA (pp. 25–32). ACM Press.
- Hutchins, E. (1995). How a cockpit remembers its speeds. Cognitive Science, 19(3), 265–288. https://doi.org/10.1207/s15516709cog1903_1
- Hutchins, E. (2010). Cognitive ecology. Topics in Cognitive Science, 2(4), 705–715. https://doi.org/10.1111/tops.2010.2.issue-4
- Hutchins, E., & Klausen, T. (1996). Distributed cognition in an airline cockpit. Cognition and communication at work (pp. 15–34).
- JASP Team (2021). JASP (Version 0.16) [Computer software]. Technical report.
- Jovancevic, J., Sullivan, B., & Hayhoe, M. (2006). Control of attention and gaze in complex environments. Journal of Vision, 6(12), 1431–1450. https://doi.org/10.1167/6.12.9
- Jovancevic-Misic, J., & Hayhoe, M. (2009). Adaptive gaze control in natural environments. The Journal of Neuroscience, 29(19), 6234–6238. https://doi.org/10.1523/JNEUROSCI.5570-08.2009
- Kendon, A. (1967). Some functions of gaze-direction in social interaction. Acta Psychologica, 26, 22–63. https://doi.org/10.1016/0001-6918(67)90005-4
- Kendrick, K. H., Holler, J., & Levinson, S. C. (2023). Turn-taking in human face-to-face interaction is multimodal: Gaze direction and manual gestures aid the coordination of turn transitions. Philosophical Transactions of the Royal Society B: Biological Sciences, 378(1875), 20210473. https://doi.org/10.1098/rstb.2021.0473
- Kingstone, A. (2009). Taking a real look at social attention. Current Opinion in Neurobiology, 19(1), 52–56. https://doi.org/10.1016/j.conb.2009.05.004
- Kleinke, C. L. (1986). Gaze and eye contact: A research review. Psychological Bulletin, 100(1), 78–100. https://doi.org/10.1037/0033-2909.100.1.78
- Koenderink, J. J. (2010). Vision and Information. In L. Albertazzi, G. J. Van Tonder, & D. Vishwanath (Eds.), Perception beyond inference: The information content of visual processes (pp. 27–57). MIT Press.
- Land, M., Mennie, N., & Rusted, J. (1999). The roles of vision and eye movements in the control of activities of daily living. Perception, 28(11), 1311–1328. https://doi.org/10.1068/p2935
- Luft, C. D. B., Zioga, I., Giannopoulos, A., Di Bona, G., Binetti, N., Civilini, A., Latora, V., & Mareschal, I. (2022). Social synchronization of brain activity increases during eye-contact. Communications Biology, 5(1), 412. https://doi.org/10.1038/s42003-022-03352-6
- Macdonald, R. G., & Tatler, B. W. (2013). Do as eye say: Gaze cueing and language in a real-world social interaction. Journal of Vision, 13(4), 1–12. https://doi.org/10.1167/13.4.6
- Macdonald, R. G., & Tatler, B. W. (2018). Gaze in a real-world social interaction: A dual eye-tracking study. Quarterly Journal of Experimental Psychology, 71(10), 2162–2173. https://doi.org/10.1177/1747021817739221
- Maran, T., Furtner, M., Liegl, S., Kraus, S., & Sachse, P. (2019). In the eye of a leader: Eye-directed gazing shapes perceptions of leaders' charisma. The Leadership Quarterly, 30(6), 101337. https://doi.org/10.1016/j.leaqua.2019.101337
- Maran, T., Furtner, M., Liegl, S., Ravet-Brown, T., Haraped, L., & Sachse, P. (2021). Visual attention in real-world conversation: Gaze patterns are modulated by communication and group size. Applied Psychology, 70(4), 1602–1627. https://doi.org/10.1111/apps.v70.4
- Maran, T., Hoffmann, A., & Sachse, P. (2022). Early lifetime experience of urban living predicts social attention in real world crowds. Cognition, 225, 105099. https://doi.org/10.1016/j.cognition.2022.105099
- Marr, D. (1982). Vision: A computational investigation into the human representation and processing of visual information. W. H. Freeman and Company.
- Marsh, K. L., Richardson, M. J., Baron, R. M., & Schmidt, R. (2006). Contrasting approaches to perceiving and acting with others. Ecological Psychology, 18(1), 1–38. https://doi.org/10.1207/s15326969eco1801_1
- Marsh, K. L., Richardson, M. J., & Schmidt, R. C. (2009). Social connection through joint action and interpersonal coordination. Topics in Cognitive Science, 1(2), 320–339. https://doi.org/10.1111/tops.2009.1.issue-2
- Matthis, J. S., Yates, J. L., & Hayhoe, M. M. (2018). Gaze and the control of foot placement when walking in natural terrain. Current Biology, 28(8), 1224–1233. https://doi.org/10.1016/j.cub.2018.03.008
- Niehorster, D. C., Cornelissen, T., Holmqvist, K., & Hooge, I. (2019). Searching with and against each other: Spatiotemporal coordination of visual search behavior in collaborative and competitive settings. Attention, Perception, & Psychophysics, 81(3), 666–683. https://doi.org/10.3758/s13414-018-01640-0
- Niehorster, D. C., Hessels, R. S., Benjamins, J. S., Nyström, M., & Hooge, I. T. C. (2023). GlassesValidator: A data quality tool for eye tracking glasses. Behavior Research Methods.
- Norman, D. A., & Shallice, T. (1986). Attention to action: Willed and automatic control of behavior. In R. J. Davidson, G. E. Schwartz, & D. Shapiro (Eds.), Consciousness and self-regulation (pp. 1–18). Springer US.
- Pagnotta, M., Laland, K. N., & Coco, M. I. (2020). Attentional coordination in demonstrator-observer dyads facilitates learning and predicts performance in a novel manual task. Cognition, 201, 104314. https://doi.org/10.1016/j.cognition.2020.104314
- Patterson, M. L. (1976). An arousal model of interpersonal intimacy. Psychological Review, 83(3), 235–245. https://doi.org/10.1037/0033-295X.83.3.235
- Patterson, M. L. (1982). A sequential functional model of nonverbal exchange. Psychological Review, 89(3), 231–249. https://doi.org/10.1037/0033-295X.89.3.231
- Paxton, A., & Dale, R. (2013). Multimodal networks of interpersonal interaction and conversational contexts. Proceedings of the Annual Meeting of the Cognitive Science Society, 35, 1121–1126.
- Pelz, J. B., & Canosa, R. (2001). Oculomotor behavior and perceptual strategies in complex tasks. Vision Research, 41(25–26), 3587–3596. https://doi.org/10.1016/S0042-6989(01)00245-0
- Pérez, D. L., Radkowska, A., Raczaszek-Leonardi, J., & Tomalski, P., & The TALBY Study Team (2018). Beyond fixation durations: Recurrence quantification analysis reveals spatiotemporal dynamics of infant visual scanning. Journal of Vision, 18(13), 1–17. https://doi.org/10.1167/18.13.1
- Peterson, M. F., Lin, J., Zaun, I., & Kanwisher, N. (2016). Individual differences in face-looking behavior generalize from the lab to the world. Journal of Vision, 16(7), 12–18. https://doi.org/10.1167/16.7.12
- Pickering, M. J., & Garrod, S. (2004). Toward a mechanistic psychology of dialogue. Behavioral and Brain Sciences, 27(2), 169–226.
- Richardson, D. C., & Dale, R. (2005). Looking to understand: The coupling between speakers' and listeners' eye movements and its relationship to discourse comprehension. Cognitive Science, 29(6), 1045–1060. https://doi.org/10.1207/s15516709cog0000_29
- Risko, E. F., & Kingstone, A. (2011). Eyes wide shut: Implied social presence, eye tracking and attention. Attention, Perception & Psychophysics, 73(2), 291–296. https://doi.org/10.3758/s13414-010-0042-1
- Risko, E. F., Laidlaw, K. E. W., Freeth, M., Foulsham, T., & Kingstone, A. (2012). Social attention with real versus reel stimuli: Toward an empirical approach to concerns about ecological validity. Frontiers in Human Neuroscience, 6(1), 143.
- Risko, E. F., Richardson, D. C., & Kingstone, A. (2016). Breaking the fourth wall of cognitive science: Real-world social attention and the dual function of gaze. Current Directions in Psychological Science, 25(1), 70–74. https://doi.org/10.1177/0963721415617806
- Rousselet, G. A., Pernet, C. R., & Wilcox, R. R. (2017). Beyond differences in means: Robust graphical methods to compare two groups in neuroscience. European Journal of Neuroscience, 46(2), 1738–1748. https://doi.org/10.1111/ejn.2017.46.issue-2
- Ruhland, K., Peters, C. E., Andrist, S., Badler, J. B., Badler, N. I., Gleicher, M., Mutlu, B., & McDonnell, R. (2015). A review of eye gaze in virtual agents, social robotics and HCI: Behaviour generation, user interaction and perception. Computer Graphics Forum, 34(6), 299–326. https://doi.org/10.1111/cgf.12603
- Schönbrodt, F. D., & Wagenmakers, E.-J. (2018). Bayes factor design analysis: Planning for compelling evidence. Psychonomic Bulletin & Review, 25(1), 128–142. https://doi.org/10.3758/s13423-017-1230-y
- Sebanz, N., Bekkering, H., & Knoblich, G. (2006). Joint action: Bodies and minds moving together. Trends in Cognitive Sciences, 10(2), 70–76. https://doi.org/10.1016/j.tics.2005.12.009
- Sprague, N., & Ballard, D. (2004). Eye movements for reward maximization. Advances in neural information processing systems (pp. 1467–1474).
- Sprague, N., Ballard, D., & Robinson, A. (2007). Modeling embodied visual behaviors. ACM Transactions on Applied Perception, 4(2), 1–23. https://doi.org/10.1145/1265957.1265960
- Sullivan, B., Ludwig, C. J. H., Damen, D., Mayol-Cuevas, W., & I. D. Gilchrist (2021). Look-ahead fixations during visuomotor behavior: Evidence from assembling a camping tent. Journal of Vision, 21(3), 13. https://doi.org/10.1167/jov.21.3.13
- The Language Archive (2022). ELAN (Version 6.4) [Computer software]. Technical report, Max Planck Institute for Psycholinguistics, Nijmegen.
- Tong, M. H., Zohar, O., & Hayhoe, M. M. (2017). Control of gaze while walking: Task structure, reward, and uncertainty. Journal of Vision, 17(1), 28–19. https://doi.org/10.1167/17.1.28
- Tonsen, M., Baumann, C. K., & Dierkes, K. (2020). A high-level description and performance evaluation of pupil invisible. CoRR, abs/2009.00508.
- Ullman, S. (1996). High-level vision: Object recognition and visual cognition. MIT Press.
- Vabalas, A., & Freeth, M. (2016). Brief report: Patterns of eye movements in face to face conversation are associated with autistic traits: Evidence from a student sample. Journal of Autism and Developmental Disorders, 46(1), 305–314. https://doi.org/10.1007/s10803-015-2546-y
- Villani, V., Pini, F., Leali, F., & Secchi, C. (2018). Survey on human–robot collaboration in industrial settings: Safety, intuitive interfaces and applications. Mechatronics, 55, 248–266. https://doi.org/10.1016/j.mechatronics.2018.02.009
- Võ, M. L. H., Smith, T. J., Mital, P. K., & Henderson, J. M. (2012). Do the eyes really have it? Dynamic allocation of attention when viewing moving faces. Journal of Vision, 12(13), 1–14. https://doi.org/10.1167/12.13.1
- Wang, I., Narayana, P., Patil, D., Mulay, G., Bangar, R., Draper, B., Beveridge, R., & Ruiz, J. (2017). Exploring the use of gesture in collaborative tasks. In Proceedings of the 2017 CHI Conference Extended Abstracts on Human Factors in Computing Systems (pp. 2990–2997). ACM.
- Warren, W. H. (2006). The dynamics of perception and action. Psychological Review, 113(2), 358–389. https://doi.org/10.1037/0033-295X.113.2.358
- Wohltjen, S., & Wheatley, T. (2021). Eye contact marks the rise and fall of shared attention in conversation. Proceedings of the National Academy of Sciences, 118(37), e2106645118. https://doi.org/10.1073/pnas.2106645118
- Zhao, F., Henrichs, C., & Mutlu, B. (2020). Task interdependence in human-robot teaming. In 2020 29th IEEE International Conference on Robot and Human Interactive Communication (RO-MAN) (pp. 1143–1149). IEEE.
- Zhao, H., & Warren, W. H. (2015). On-line and model-based approaches to the visual control of action. Vision Research, 110, 190–202. https://doi.org/10.1016/j.visres.2014.10.008