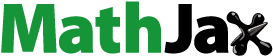
Abstract
The European Smart Specialisation Strategy (S3) with its underlying aim to support regional growth, cohesion, and innovation has by now been widely applied to European Sparsely Populated Areas (SPAs). Previous works have identified that intra-regional stakeholder networks are important for a successful S3 implementation, and that their establishment is related to the level of proximity between stakeholders; however, the nature of such networks in the context of developing S3 in SPAs deserves further investigation. This paper explores how different dimensions of proximity are associated with intra-regional networks aimed at implementing S3 in a Northern European SPA (Lapland, Finland). The results suggest that geographical and cognitive proximity are positively associated with both formal and informal networking, while institutional proximity is negatively related with informal networking, suggesting that effective policy interventions require multiple relationships to be activated through supporting greater diversity between SPAs stakeholders.
1. Introduction
Regions characterised by intensive cooperation of public and private stakeholder organizations are expected to excel in innovation and regional development (Santoalha Citation2019): however, this is by no means a simple task (Cooke and Morgan Citation1993). A case in point are sparsely populated areas (SPAs), which typically lack the critical mass of relevant stakeholders for establishing these cooperation networks (Virkkala Citation2007) and often share several social and cultural features as part of a distinct geographical category (Le Tourneau Citation2020). SPAs mainly correspond to the northern parts of the Nordic countries, Scotland and central Spain (Teräs et al. Citation2015), and they are characterized by distinct environmental, socio-demographic, and economic contexts. Policy support is needed for facilitating collaborative relations between SPAs stakeholders in such contexts, i.e. local government and public authorities, universities, research institutions, private civil society organizations, local development companies, enterprises, and enterprise associations. The conceptual developments related to networking have led to the coining of the European Union's Smart Specialisation Strategy (S3) as a regional development tool that underlines the importance of cooperation in driving economic growth and innovation across EU regions (Foray Citation2014, Citation2018; Nauwelaers, Kleibrink, and Stancova Citation2014; Moodysson, Trippl, and Zukauskaite Citation2017; Morgan Citation2017; Uyarra, Marzocchi, and Sörvik Citation2018; Martin, Martin, and Zukauskaite Citation2019). S3 is a perfect example of ‘policy running ahead of theory’ (Foray, David, and Hall Citation2011, 1); yet it still contains caveats in its implementation (Sörvik et al. Citation2019). Moreover, the adoption of S3-driven cooperation strategies in SPAs are influenced by mobilizing stakeholders and multilevel governance processes (Sörvik et al. Citation2019). Thus, understanding the preconditions of cooperation in S3 implementation in SPAs offers valuable insights for improved policy design at the regional and EU-level.
As illustrated by Sörvik et al. (Citation2019), SPAs have taken advantage of the opportunities offered by the S3 approach, and several have been able to establish innovative environments. Their specific characteristics include the presence of ‘strong regional cross sectorial networks and low hierarchies with quick access to regional decision makers’ (Sörvik et al. Citation2019, 1073). Therefore, the implementation of S3 in SPAs needs intra-regional connectivity for supporting local development and employment in SPAs (Dubois, Kristensen, and Teräs Citation2017). However, although the importance of promoting network relationships between local stakeholders is acknowledged in the S3 literature (Morgan Citation2017), limited evidence exists on the exact nature of these relationships in the context of SPAs (Kaivo-oja et al. Citation2017; Virkkala, Mäenpää, and Mariussen Citation2017; Aranguren et al. Citation2019; Sörvik et al. Citation2019; Gianelle, Guzzo, and Mieszkowski Citation2020; Pagliacci et al. Citation2020). Previous work on regional innovation policy suggests that strengthening local stakeholders’ relationships is one of the key dimensions of S3 (McCann and Ortega-Argilés Citation2013), based on the premise that the more stakeholders are embedded in relational networks, the higher the probability that the region achieves its S3 targets. Caloffi et al. (Citation2017) point out that the network-based approach to S3 allows to introduce multiple relational structures in terms of public, private, and public-private partnerships. In particular, it is possible to distinguish between two main types of relationships when considering organizational networks shaping territorial governance: informal and formal (del Castillo, Paton, and Barroeta Citation2014). The establishment of informal relationships is commonly used to exchange advice and knowledge without specific constraints, and it is often based on the presence of trust between the representatives of the organization involved in the exchanging process (Fitjar and Rodríguez-Pose Citation2011). High levels of (regional) trust have a positive effect on informal knowledge sharing, whereas dishonouring informal deals will lead to social sanctions towards the ‘trust breaking’ organization including reputation loss (‘bad name’) and lost chances for future collaboration. As such, informal collaboration, while not legally-binding, is, nonetheless, governed by informal rules (Dei Ottati Citation1994). Contrarily, formal relationships are tangible legally binding elements (such as agreements for R&D project collaboration) that also characterize territorial governance in the implementation of S3 strategies (Foray Citation2018). Aranguren et al. (Citation2019) discuss the effectiveness of formal versus informal governance mechanisms also tied to the size of regions, to suggest that formal regional coordination mechanisms are usually built as consequence of hierarchical coordination, while informal mechanisms are often a feature of smaller regions.
Yet, in spite of evidence that stimulating cooperation as part of S3 can be relevant for supporting regional growth (Mariussen, Rakhmatullin, and Stanionyte Citation2016; Uyarra, Sörvik, and Midtkandal Citation2014) and innovation (Nauwelaers, Kleibrink, and Stancova Citation2014; Santoalha Citation2019), and in spite of a call for a greater focus on micro-level issues and relations (Benner Citation2015; Aranguren et al. Citation2019), we continue to observe a lack of studies on how stakeholders in SPAs establish relationships aimed at supporting S3.
Recent evidence helps to understand the elements associated with networking, as it suggests that local stakeholders can overcome coordination weaknesses by establishing multiple relationships in the implementation of S3 (Ghinoi et al. Citation2020; Sörvik et al. Citation2019). This discussion has included the concept of proximity – which is a relevant driver of inter-organizational networking (Knoben and Oerlemans Citation2006) – as an essential precondition in SPAs for successful collaboration (Uyarra, Sörvik, and Midtkandal Citation2014; Dubois, Kristensen, and Teräs Citation2017). That is, stakeholders need to be close enough to be able to establish relationships. An exhaustive literature has identified multiple dimensions of proximity in addition to the classical concept of geographical proximity based on physical distance (Boschma Citation2005). Their importance has received support in recent studies on S3 (e.g. Dubois, Kristensen, and Teräs Citation2017; Virkkala, Mäenpää, and Mariussen Citation2017; Mäenpää and Virkkala Citation2019; Sörvik et al. Citation2019). Nevertheless, what remains striking is the lack of studies that have quantitatively assessed the influence of different proximity dimensions on S3 implementation in SPAs (Pugh Citation2018).
The objective of this paper is to explore the extent to which three proximity dimensions (geographical, institutional, and cognitive) are associated with multiple (informal and formal) networks among stakeholders in the implementation of S3 in a SPA. Furthermore, our paper follows calls for more work on the nature and role of relational networks as the cornerstones of bottom-up approaches in S3 (Moodysson, Trippl, and Zukauskaite Citation2017). Thereby, the paper aims to improve our understanding of stakeholder networks aimed at addressing S3 related governance issues, and it contributes to the contextualization of S3 as it has been judged still a ‘work in progress’ (Sörvik et al. Citation2019, 1070). Our empirical analysis focuses on Lapland (Finland) as a representative region of the Northern Sparsely Populated Areas Network (NSPA Citation2018), using Social Network Analysis (SNA) to assess to what extent spatial (geographical) and non-spatial (institutional and cognitive) proximity dimensions are related to networking activities aimed to implement S3.
Our contribution is thus threefold. First, we enrich the sparse S3 stakeholder-focused literature (e.g. Virkkala, Mäenpää, and Mariussen Citation2017; Santoalha Citation2019) by applying SNA, allowing us to further integrate the network perspective into the S3 framework. Second, we complement and extend previous studies which have also assessed proximity as part of analyzing SPAs (e.g. Sörvik et al. Citation2019). In particular, we complement a previous qualitative S3 study focusing on the Finnish North Karelia region, which suggests that proximity can play an important role in facilitating exchange of tacit knowledge through face-to face interactions (Nauwelaers, Kleibrink, and Stancova Citation2014). Third, we contribute by assessing interactions of proximity metrics in the context of S3 in SPAs.
The remainder of the paper is structured as follows: section 2 provides a discussion of the relevant literature leading to our hypotheses; section 3 introduces the method of analysis, while section 4 presents our findings, followed by the discussion of results, policy implications and limitations (section 5).
2. Literature
2.1. Smart Specialisation Strategy (S3) in Sparsely Populated Areas (SPAs)
S3 is recognized as a key tool for achieving the Europe 2020 goals of competitiveness and sustainable economy (Foray Citation2014), and its institutional role has been consolidated by the European Commission (EC), which has imposed its completion as a precondition for accessing EU funding. The idea behind the concept of S3 is that regions should decide to specialize in sectors where they already have strengths and competences in order to pursue an ‘intelligent entrepreneurial discovery process’ (Pugh Citation2018, 531), instead of focusing on industry sectors which are trending in other contexts. S3 is aimed to take advantage of the territorial diversity of EU regions and developing their potential for future development (Sörvik et al. Citation2019); this approach is not purely bottom-up nor top-down, since the government defines the general policy framework for entrepreneurial discovery, while local stakeholders – typically representing research institutes, public authorities and businesses – can intervene in the design of policy priorities, leading to relatedness that supports a bottom-up policy approach to S3 (Aranguren et al. Citation2019; Balland et al. Citation2019).
Local and regional governments and authorities are, naturally, in a crucial role in orchestrating the S3 process. Their role is to advance the implementation of S3 by, for example, providing adequate incentives for the development of effective collaborations among stakeholders (Rodríguez-Pose, Di Cataldo, and Rainoldi Citation2014). Local stakeholders beyond regional governments and authorities can also play multiple roles in S3. Universities and research institutes have the potential to contribute to the assessment of the region's strengths and competences; moreover, they play an important role in bringing regional actors together and connecting them with extra-local partners, and they provide expertise and teaching programmes that contribute to regional knowledge creation (Kempton et al. Citation2013; Kangas and Aarrevaara Citation2020); Enterprises and enterprise associations are at the heart of entrepreneurial discovery processes by bringing in their knowledge on the actual needs of the local market (Kroll Citation2019). Civil society actors can be actively involved in both policy dialogue when drafting the S3 strategy and in the implementation of the S3 policy (Carayannis and Rakhmatullin Citation2014). Due to their complementary roles, the networking process established by the above stakeholders is particularly relevant for a successful S3 implementation (Uyarra, Marzocchi, and Sörvik Citation2018).
The focus of S3 on sustainable and inclusive growth has produced an increased interest on innovative activities in peripheral regions and SPAs (Smart Specialisation Platform Citation2012; Nauwelaers, Kleibrink, and Stancova Citation2014). European SPAs are mainly located in Northern Scotland, in the Nordic countries, and in Central Spain. These areas are characterized by a number of environmental, socio-demographic (e.g. harsh climatic conditions, geographical distance from the main European and global markets, combination of low population density and dispersed settlement patterns), and economic challenges (e.g. focus on resource-intense industries, low levels of science-based industries and R&D activities, lack in human resources and population in general [Martinus Citation2018]). Because of these challenges, SPAs benefit from the European Structural Investment Funds, with the aim to maximize their regional knowledge-based potential in order to promote growth and employment (Asheim Citation2019). As illustrated by Foray (Citation2014) and Sörvik et al. (Citation2019), SPAs are nevertheless in an advantageous position with regard to the exploitation of natural resources and the green economy narrative (Teräs et al. Citation2015) that is pursued by the European Union. Since S3 is related to the idea of place-based development and cohesion (Barca Citation2009; Pagliacci et al. Citation2020), it does not imply a standardized process for implementing innovation policy in all European regions (Gianelle, Guzzo, and Mieszkowski Citation2020). Rather, the opposite view holds: regions have very different characteristics, and their competitiveness depends upon their institutional configurations, knowledge resources, natural resources, and innovation measures (Dubois and Roto Citation2012; Nauwelaers, Kleibrink, and Stancova Citation2014; Grillitsch Citation2016). Hence, SPAs have invested in the innovative exploitation of their natural capital and the efficient use of natural resources to become more competitive (Teräs et al. Citation2015).
According to Dubois and Roto (Citation2012) and Balland et al. (Citation2019), to make sparse territories more competitive, it is necessary to adopt strategies based on the improvement of soft and hard connectivity, promote a new entrepreneurial culture, design cluster policies that take advantage of the local economic context, and avoid replicating practices implemented in urban regions. An important role is played by the relational capital found in these areas: the presence of networks of relations between local stakeholders is related to innovativeness, business growth, and efficiency in the adoption of local development policies (Fitjar and Rodríguez-Pose Citation2011; Habersetzer et al. Citation2019). Therefore, relatedness and knowledge complexity are important for building local related capabilities in addressing regions’ diversification dilemma (Balland et al. Citation2019). S3 benefits from the existence of these inter-organizational networks because the prioritizing of innovation policies aimed to business development requires a coherent common perspective by all local stakeholders, in order to avoid fragmentation and wastage of resources, which can have a detrimental effect on SPAs (McCann and Ortega-Argilés Citation2013). These findings corroborate with the European Commission's view, which advocates an ‘inclusive process of stakeholders’ involvement’ in the S3 process, since it ‘builds on the assets and resources available to regions […] in order to identify unique opportunities for development and growth’ (EU Citation2018).
2.2. Proximity and networking in SPAs
Despite a fast-growing literature on the application of S3 to SPAs (e.g. Asheim, Grillitsch, and Trippl Citation2017; Dubois, Kristensen, and Teräs Citation2017; Kaivo-oja et al. Citation2017) and the importance of S3 stakeholder networks to mediate conflicting interests and support the development of a shared vision (Grillitsch Citation2016; Sotarauta Citation2018), the role of proximity in SPAs networks has received limited attention in the literature. The following sections aim to develop our key hypotheses regarding proximity dimensions and their association with (informal and formal) S3 networking in SPAs, focusing on previous evidence from the literature on regional economics and innovation. The first set of hypotheses (H1a, H1b, H1c) focuses on single proximity dimensions. The second set of hypotheses (H2a, H2b) focuses on the combination of institutional and cognitive proximity with geographical proximity.
2.2.1. Geographical proximity
The seminal studies of Marshall (Citation1925), Becattini (Citation1979), and Jaffe, Trajtenberg, and Henderson (Citation1993) point to the importance of geographical proximity between regional stakeholders, and its effect on economic measures of performance, such as productivity and innovativeness. Broadly speaking, the more stakeholders have the possibility to interact face-to-face due to short geographic distance, the more probable is the exchange of knowledge and information aimed to enforce their organizational skills and competences. Some authors argue that geographical proximity per se is not a pre-determined fixed concept, but it should be disentangled to take into account different perspectives. Strikingly, Presutti et al. (Citation2019) provide evidence on the negative impact of geographical proximity for innovation. Torre and Rallet (Citation2005) suggest that meetings and other activities, like trade shows and the temporary sharing of employees, allow temporal geographical proximity for the establishment of a collaboration even between long distance partners. Recent evidence also suggests that the relation between geographic proximity and the establishment of collaboration is positive, both regarding informal and formal collaborations (Capone and Lazzaretti Citation2018), since localization of knowledge is crucial for its diffusion and thus regional growth (Huggins and Thompson Citation2017). The overall evidence seems thus to support the notion that geographical proximity, as a broad concept, is positively associated with knowledge spillovers, organizations’ collaborations, and organizational performance (Huggins and Johnston Citation2010; Boschma, Balland, and de Vaan Citation2014). This is consistent in the context of SPAs, since the large distance between stakeholders (due to geographical conditions) influences stakeholders’ interactions, which therefore need to be compensated through special events (Zamyatina and Pilyasov Citation2017). Moreover, Virkkala, Mäenpää, and Mariussen (Citation2017) find that geographical proximity is a relevant driver for collaboration aimed to developing S3 in Finnish regions. In light of the above evidence for geographical proximity, and the limited evidence on the role of multiple relationships in SPAs, we put forward the following hypothesis:
H1a: The development of networks (both informal and formal) related to S3 is positively associated with geographical proximity between stakeholders.
2.2.2. Institutional proximity
Institutional proximity is related to norms, rules, and values, and the notion of embeddedness of interrelations between organizations (Kirat and Lung Citation1999): when organizations share similar rules and values, economic coordination and interactive learning are activated, providing stability. However, Capone and Lazzaretti (Citation2018) argue that institutional proximity can be detrimental for the exchange of informal knowledge. In the context of S3, Grillitsch (Citation2016) suggests that the more a region is characterized by institutional diversity, the more local enterprises can benefit from an enhanced entrepreneurial discovery process. This finding is confirmed also by a study on SPAs from Dubois, Kristensen, and Teräs (Citation2017), which points to the importance of the institutional context for innovation and regional development. Moreover, Grillitsch (Citation2016) suggests that institutional diversity, rather than institutional proximity, is the key for entrepreneurial discovery in S3, in particular for developing formal relationships between stakeholders (e.g. establishing university-industry connections). Therefore, a range of institutions with different tasks operating in the same region could be conjectured to foster growth in SPAs. In light of the above evidence, we propose the following hypothesis:
H1b: The development of networks (both informal and formal) related to S3 is negatively associated with institutional proximity between stakeholders.
2.2.3. Cognitive proximity
Cognitive proximity refers to the existence of a common knowledge base between actors, which facilitates understandability and thereby increases the efficiency of collaboration (Lundquist and Trippl Citation2013). Nooteboom (Citation2000) suggests a distinction between cognitive distance for the sake of novelty and learning, and cognitive proximity for the sake of efficiency of knowledge exchange. In on the context of Finnish peripheral areas, Virkkala (Citation2007) and Mäenpää and Virkkala (Citation2019) found that cognitive proximity is positively associated with firms’ knowledge exchange towards collaboration for innovation. Nooteboom et al. (Citation2007) and Laursen, Reichstein, and Salter (Citation2011) argue that cognitive proximity is necessary for setting up formal relationships characterized by a stable collaboration between stakeholders, such as in the case of industry-university R&D project partnerships. Considering the above evidence, we propose the following hypothesis:
H1c: The development of networks (both informal and formal) related to S3 is positively associated with cognitive proximity between stakeholders.
2.2.4. Interaction between proximity dimensions
Lagendijk and Lorentzen (Citation2007) put forward that cognitive and institutional proximities can both facilitate knowledge exchange between stakeholders when combined with geographical proximity. This idea relates to Boschma's work (Citation2005, 62), where it is pointed out that ‘geographical proximity per se is neither a necessary nor a sufficient condition for learning to take place: at most, it facilitates interactive learning, most likely by strengthening the other dimensions of proximity’.
However, to the best of our knowledge, not only have few studies empirically tested the combined effect of geographical proximity and other dimensions of proximity on networking, but there is also an absence of studies in the context of SPAs. With regard to the combination of geographical and institutional proximity, Cao, Derudder, and Peng (Citation2019) suggest that the negative effect of a long spatial distance between actors is mitigated by the presence of institutional proximity; hence, these dimensions of proximity are considered substitutes in supporting collaboration. Moreover, Ponds, van Oort, and Frenken (Citation2007, 442) highlight that geographical proximity ‘seems to matter for collaboration in the case of institutional differences, thereby facilitating successful collaboration’, confirming the substitutionary effect between geographical and institutional proximity. With regard to the combination of geographical and cognitive proximity, Malmberg and Maskell (Citation2006, 9) argue that spatial proximity strengthens cognitive proximity by developing a common institutional, social, and cultural setting; being physically close reinforces the learning processes underlying the acquisition of cognitive skills, which supports a ‘knowledge alignment’ between actors, that in turn enables innovative activities. In this vein, the work of Cao, Derudder, and Peng (Citation2019) shows that geographical and cognitive proximity have a complementary relation, i.e. collaboration between organizations with a similar knowledge background is reinforced by geographical proximity. In light of the lack of studies distinguishing between informal and formal network collaborations, inconsistent evidence (Hansen Citation2015), and the absence of evidence from S3 implementation in SPAs, we put forward the following hypotheses:
H2a: The positive association between geographical proximity and networking (both informal and formal) is substituted by institutional proximity.
H2b: The positive association between geographical proximity and networking (both informal and formal) is reinforced with cognitive proximity.
3. Data and methods
3.1. The empirical setting: Lapland
Lapland is an example of how S3 implementation has been utilized to steer regional collaboration for advancing common regional development targets. It has been regarded as a model SPA in terms of its exceptional capability in adapting the S3 concept into its regional development plans and implementation programmes (Teräs et al. Citation2018). Lapland is one of the 14 regions across the Scandinavian Peninsula (including Norway, Sweden and Finland) which make up the Northern Sparsely Populated Areas (NSPAs). Despite their geographical marginality and the difficult climatic conditions, these regions show above OECD average levels of well-being (OECD Citation2017). However, they suffer from challenges related to an ageing population, rising unemployment, and the migration of the younger population to other, primarily urban, areas (Carson et al. Citation2011). A recent OECD study finds that possible solutions to address these challenges include being innovative and fostering collaborations between local stakeholders, as well as supporting internationalization and connection with external markets (OECD Citation2017).
To respond to the above SPA challenges, the Regional Council of Lapland launched its S3 in 2013 to boost competitiveness and innovation. In Lapland, the concept of ‘Arctic Smartness’ (sustainable utilization and commercialization of arctic natural resources) is the leading initiative for implementing S3 based on regional cooperation networks and shared goals to develop the region. A recent impact assessment report by Auri et al. (Citation2019) highlights the relation between the S3 implementation process and local cooperation and networking in Lapland. This process has led to market benefits for directing regional development. First, under S3, different stakeholders work as partners, not as competitors, to develop the region. Second, under S3 the cooperation is directed towards the long-term development of larger thematic focus areas, instead of the conventional short-term project-based focus characterising former development efforts in the region. Third, the cooperation has removed redundant and overlapping activities between different stakeholders, helping them to focus their resources more effectively.
Indeed, while the design process of Lapland's S3 strategy was launched and supervised by the Regional Council of Lapland, the opinions of a multitude of other stakeholdersFootnote1 (e.g. universities, municipalities, research institutes, local business organizations, local development companies, etc.) were also taken into account in its formulation. Further, local universities and development companiesFootnote2, rather than the Regional Council, are responsible for implementing the Arctic Smartness concept via orchestrating networking events and other cluster activities underlining the perceived importance of complementarity in stakeholder networking. Lapland's S3 focuses on the development of a structured knowledge transfer system based on regional clusters, whose domains and aims are the followingFootnote3: development infrastructures; design; industry and circular economy; smart rural networks; safety and security. As in other SPAs (Sörvik et al. Citation2019), Lapland's main economic domain is based on natural resource exploitation. Hence, sustainability in the use of natural resources is the keyword in Lapland's S3 report presented to the European Commission.
3.2. Data collection
In order to identify the relevant local stakeholders involved in the S3 process, we adopted a two-steps approach. The stakeholders included in the steering group of Lapland's Arctic Specialisation Programme and those in charge of the Arctic clusters constitute our primary roster for the data collection. Further, we assessed the list of attendees and participants of S3 workshops and fairs in Lapland; we also analyzed the members of Lapland's Cooperation Group (which is responsible for coordinating regional development measures in Lapland) to supplement our roster with other active stakeholders. As a result, we identified a population of 49 stakeholders (i.e. organizations) associated with 74 possible informants (i.e. individuals representing those organizations) that took part in S3 activities in Lapland. We contacted these informants during October 2018, sending them an e-mail with a link to an online questionnaire. Those who had not responded after two additional reminders were contacted to complete the questionnaire via phone interview. We selected more than one informant per stakeholder for two practical reasons: (a) there are often more individuals responsible for S3-related issues within an organization; (b) we wanted to increase the probability of receiving a positive answer to our request and reducing the risk of non-responsiveness.
The questionnaire included questions for collecting data on informal and formal relationships activated during the 2015–2018 period. In particular, we distinguished these relationships by specifying that:
informal relationships are considered those involving exchange of advice and/or information between two stakeholders (e.g. informal knowledge exchange, advice etc. during conferences or training);
formal relationships are considered those concluded through contracts between two stakeholders and aimed at the production of a specific outcome (e.g. the creation of a start-up).
Although Lapland's S3 final report was already presented in 2013, we focused on the 2015–2018 period because new funding opportunities related to innovation and entrepreneurship have been introduced in the EU during the programming period 2014–2020. Therefore, we conjectured that new networking strategies have been activated since 2013 among local stakeholders. By using a roster method (Scott and Carrington Citation2011), we asked the respondents to indicate with which of the 49 organizations their own organization established one or more relationships. A copy of the questionnaire for collecting relational data (anonymized version) is presented in Appendix 1. Following the above procedure, we received responses from 28 informants, affiliated with 19 stakeholder organizations (response rate of 38%); this response rate is comparable with previous related works on networks in SPAs (e.g. Virkkala, Mäenpää, and Mariussen Citation2017). When we obtained more than one completed questionnaire from a single stakeholder, we followed the conventional strategy adopted in the network literature (Tsai Citation2001) which assumes that a relationship declared by any informant from organization i is considered valid if any respondent from organization j confirmed its existence.
3.3. Social Network Analysis (SNA)
In this study we use SNA to investigate the network relationships between S3 stakeholders in Lapland. SNA has been applied extensively in the regional economics and innovation literature (e.g. ter Wal and Boschma Citation2009; Balland, Boschma, and Frenken Citation2014; Capone and Lazzaretti Citation2018; Maghssudipour, Lazzaretti, and Capone Citation2020); moreover, it has also been used in S3-related research for analyzing patterns of collaboration between regional stakeholders involved in S3 (e.g. Huggins and Prokop Citation2017; Virkkala, Mäenpää, and Mariussen Citation2017). We use Exponential Random Graph Models (ERGMs) (Snijders et al. Citation2006) to estimate the association between proximity dimensions and the development of informal and formal relationships between stakeholders. By using cross-sectional network data, ERGMs are ‘built from a more realistic construal of the structural foundations of social behaviour’ (Robins et al. Citation2007, 173), and they allow to model underlying social elements driving network development (Lusher, Koskinen, and Robins Citation2013). The general form of an ERGM is the following (Robins et al. Citation2007):
The probability that the observed network y is identical to the randomly generated network Y is given by an exponential model assuming that ƞA is the parameter corresponding to network configuration A, and ZA(y) is the network statistic corresponding to configuration A; k is a constant term. Network configurations are small subgraphs that can be detected within the network, representing identifiable structures such as dyads (basic network configurations made by two actors connected together by a tie), triads (subgraphs made by three interconnected actors), or other structures. Moreover, actor attributes and dyadic covariates can be added to the model to investigate how relationships develop. Assuming homogeneity amongst relational ties (i.e. all counted network formation instances are equiprobable), Markov dependence allows to identify network configurations, and associated parameters, for directed and undirected networks.
Since we focus on two different types of relationships (informal and formal), we use different ERGMs. As dependent variables, we use two square network matrices 19 × 19 identifying the informal and formal networks. These matrices are binary and symmetric, since the relationships between stakeholders do not have a direction (if i has an informal or formal collaboration with j, j has an informal or formal collaboration with i). As explanatory variables, we include five dyadic covariates that are represented by five symmetric network matrices 19 × 19. One matrix (‘Geog_prox’) identifies geographical proximity, where each cell contains the negative value (in kilometres) of the physical distance between the centroids of stakeholders’ municipalities (since we are measuring proximity, instead of distance, positive values are turned into negative). With regard to the other dimensions of proximity (institutional and cognitive), we rely on previous works on proximity and inter-organizational networks (Balland Citation2012; Balland, Belso-Martínez, and Morrison Citation2016; Molina-Morales et al. Citation2015) to operationalize the variables identifying the proximity dimensions. ‘Instit_prox’ is a symmetric matrix where the cells take value 1 if two stakeholders present the same legal category and 0 otherwise. As suggested by Balland (Citation2012), we adopt the triple helix categorization (science, policy, and industry) to identify similar stakeholders: research institutions, government and institutions/local authorities, and development companies. The third dyadic covariate (‘Cogn_prox’) captures cognitive proximity according to the stakeholders’ similarity in carrying out their activities (e.g. both providing business and financial services, both supporting rural development activities, both focusing on research activities, both offering training services). Cells in this matrix take the value 1 when stakeholders share the same knowledge expertise and 0 otherwise. The decision of considering stakeholders’ similarity in carrying out activities as a proxy for cognitive proximity follows previous studies on inter-organizational networks in regional clusters, which have used NACE codes (e.g. Balland, Belso-Martínez, and Morrison Citation2016; Molina-Morales et al. Citation2015). The fourth and fifth matrices (‘GeogXInstit’ and ‘GeogXCogn’, respectively) present the interaction between geographical proximity and the other dimensions of proximity. These matrices have been created by using the matrix product between ‘Geog_prox’ and the other proximity matrices.
The ERGMs also include an ‘Edge’ term, which is a network structural effect representing the tendency for developing relationships (ties) (Lusher, Koskinen, and Robins Citation2013), an organizational ‘Size’ term, a continuous variable expressed in terms of number of employees (data on the number of employees for each stakeholder/organization have been collected through web pages and reports), and a ‘Balance’ term, which is a network structural effect that accounts for the number of balanced triads in networks (Morris, Handcock, and Hunter Citation2008). ‘Size’ is used to control for possible spillover effects, assuming a greater absorptive capacity of knowledge from bigger organizations (Cao, Derudder, and Peng Citation2019). ‘Balance’ allows to consider the presence of balanced sub-structures, a relevant influencing factor in networking (Davis and Leinhardt Citation1972). Descriptive statistics of the variables used in the ERGMs (except for the network structural effects ‘Edge’ and ‘Balance’) are presented in .
Table 1. Descriptive statistics.
The analysis has been realized using the R software statnet package, which supports statistical network analysis based on ERGMs (Handcock et al. Citation2003). The results for the goodness of fit tests are presented in Appendix 2.
4. Results
Stakeholders who participated in the survey are found to be similar compared to those who did not participate, in terms of key characteristics. Both groups have a large prevalence of government and institutions/local authorities (over 50%), while development companies are around 25% among both respondents and non-respondents. and show the two networks representing informal and formal relationships, respectively. The informal network is characterized by an extensive number of relations, while the formal network is less dense. In network science, density is defined as the proportion between all the potential ties of a network and those which are actually observed: the informal network has a density of 0.60, while the formal network has a density of 0.26. The average degree in the informal network (the average number of stakeholder ties) is 10.84, while it is 4.63 in the formal network; the latter presents 39 transitive triads (Davis and Leinhardt Citation1972), while the former has 249 transitive triads. Moreover, the two networks are positively correlated, showing a QAP correlation of 0.31.
Figure 1. Informal relationships. Legend: Circle = Research institute; Square = Development company; Up triangle = Government and institution/local authority.
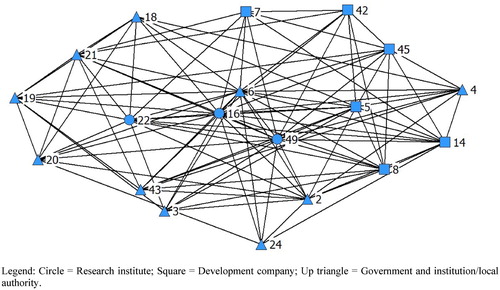
Figure 2. Formal relationships. Legend: Circle = Research institute; Square = Development company; Up triangle = Government and institution/local authority.
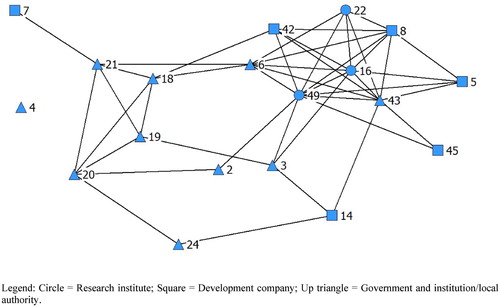
Regarding the formal network (), it is possible to observe that stakeholders are grouping around focal actors. This cohesive subgroup of focal actors, which includes universities, research centres, and the Regional Council of Lapland, is the ‘core’ of the network. The composition of this subgroup suggests that large and formalized structures are more prone to establish formal relations among each other.
The results of the ERGMs are illustrated in and . The estimated parameters of the models reflect the change in the (log-odds) likelihood of a tie for a unit change in the predictors. Therefore, the correspondent probabilities must be estimated using the exponential function. A positive coefficient means that the covariate is positively associated with the propensity to establish a relationship, while a negative coefficient indicates that the covariate is negatively associated with networking. The information criteria AIC and BIC are reported for each model. Except for the basic ERGMs (Version 1 for the informal and formal network, including the controls ‘Edge’, ‘Size’, and ‘Balance’), the coefficients are relatively stable, suggesting robustness of the results.
Table 2. ERGM: informal network.
Table 3. ERGM: formal network.
By considering the coefficients for the controls, ‘Edge’ assumes positive values in the informal network and negative values in the formal network. A possible explanation is that stakeholders in Lapland have an incentive to expand their informal connections as much as possible, because it is not as costly (in terms of human and financial resources) as it is for establishing formal relationships in the S3 context. ‘Size’ is significant only in the model 1 of the informal network, but the magnitude of the coefficients is almost null. Therefore, we can assume that the organizational dimension is not relevant for networking; indeed, because of the heterogeneity of the stakeholders operating in Lapland (from micro enterprises to large research centres), both informal and formal relationships are likely driven by other elements rather than the number of employees. Finally, ‘Balance’ is positive and statistically significant only in the formal network. Hence, balanced triads are positively associated with networking because of the type of relationship: in formal networks, stakeholders develop these configurations because S3-related activities require symmetrical relationships when activating formal contracts.
Geographical proximity (‘Geog_prox’) is positive and statistically significant in all models. In particular, the magnitude of the coefficients is higher for the formal network ERGMs. In relation with H1a, this result suggests that both informal and formal collaborations related to S3 are positively associated with the geographical proximity between stakeholders, a finding that is supported by other studies on knowledge networks and innovation systems (e.g. Breschi and Lissoni Citation2001; Broekel and Boschma Citation2012).
The association between institutional proximity (‘Instit_prox’) and the presence of informal relations between stakeholders is negative in all the different ERGMs presented in . Therefore, we assume that similar stakeholders, in terms of institutional form, are not likely to exchange S3-related informal knowledge or advice, confirming H1b. However, the coefficients for ‘Instit_prox’ are not statistically significant when considering the formal network (), hence the importance of this dimension of proximity depends on the type of relationships activated by local stakeholders.
With regard to cognitive proximity, we find that ‘Cogn_prox’ is positive and statistically significant in all different models, confirming H1c. In particular, ‘Cogn_prox’ shows a strong effect in the informal network, which indicates that a common knowledge base between stakeholders is important when establishing informal relationships.
Finally, versions 3–5 of the ERGMs in and introduce the interaction terms created for investigating how the combinations of geographical proximity and the other dimensions of proximity are associated with networking (H2a, H2b). Our findings corroborate the idea that it is important to consider different types of relationships between stakeholders to assess the effect of the interaction between multiple dimensions of proximity. As illustrated by Cao, Derudder, and Peng (Citation2019), when the coefficients are positive and statistically significant it means that there is likely a substitution effect between the proximity dimensions, while when they are negative and statistically significant it means that there is a complementarity effect. The combination of geographical and institutional proximity (‘GeogXInstit’) is positive and significant only in relation to informal networking, i.e. we accept H2a but only for the informal network. The presence of institutional proximity between stakeholders substitutes geographical proximity, increasing the propensity to collaborate between stakeholders when they are geographically distant; nevertheless, this effect is not observed in the formal network, where ‘GeogXInstit’ is not statistically significant. Moreover, ‘GeogXCogn’ is negative and statistically significant in the informal network (ERGM version 5). This result implies that there is a complementary relation between geographical and cognitive proximity in relation to the development of informal networks: stakeholders who are cognitively proximate can be more prone to establish relationships if they are geographically close. However, this finding does not apply to formal networks, where ‘GeogXCogn’ is not statistically significant (). Therefore, we confirm H2b only for the informal network.
5. Discussion and conclusions
Recent works on regional relationships and diversification in the context of S3 have suggested that a greater focus on micro-level issues and relations is needed (e.g. Benner Citation2015; Moodysson, Trippl, and Zukauskaite Citation2017), based on the idea that regions should leverage their existing capabilities (Balland et al. Citation2019). Through analyzing informal and formal relational networks, this paper identifies how different dimensions of proximity are related to the establishment of regional networks aimed to support S3 in SPAs, complementing and extending previous evidence that highlighted the importance of networking for successful S3 implementation in SPAs (Asheim, Grillitsch, and Trippl Citation2017; Sörvik et al. Citation2019). This paper also complements and extends previous works that have highlighted the role of relationships as part of regional innovation policy (McCann and Ortega-Argilés Citation2013) and focused on governance mechanisms in the context of S3 (Gianelle, Guzzo, and Mieszkowski Citation2020).
In spite of earlier works suggesting that the way stakeholders engage in networks varies by the type of the relationship (informal or formal) and by geographical and other non-spatial dimensions of proximity (Boschma, Balland, and de Vaan Citation2014; Aranguren et al. Citation2019), there appears to be a striking absence of studies that have empirically investigated these aspects in the context of SPAs and networking inherent in S3.
The results of this paper underline the importance of geographical proximity for networking in SPAs, thereby corroborating with Boschma and Gianelle (Citation2013) and Sörvik et al. (Citation2019). Cognitive proximity is found to be positively associated with informal relationships, suggesting an important role of trust, which is fundamental for reducing transaction costs in the knowledge exchange process (Fitjar and Rodríguez-Pose Citation2011); indeed, these transaction costs could be expected to increase when stakeholders are not able to overcome difficulties due to communication problems or lack of knowledge of the partner's expertise in carrying out certain activities. Further, we find that institutional proximity between stakeholders has a negative relation with knowledge exchange in informal networks. Therefore, stakeholders with similar institutional forms are less likely to exchange knowledge through informal relationships, suggesting that organizational diversity matters in SPAs. This finding corroborates with earlier evidence on smart specialisation strategies in the context of science parks (also in Finland), as the evidence there suggested that embedding a wide range of regional stakeholders is a key success factor of smart specialisation (Nauwelaers, Kleibrink, and Stancova Citation2014). However, the results of this paper also suggest that, when considering informal relationships, cognitive proximity reinforces the positive effect of geographical proximity, while institutional proximity substitutes it.
These results lead to several policy implications. First, S3 policy efforts and governance mechanisms should be targeted differently for various stakeholder types, to increase their effectiveness in implementing S3 in SPAs. In particular, it may be beneficial to support stakeholder diversity in SPAs informal networks, for example by encouraging different stakeholders in supporting local infrastructures such as incubator hubs (Etzkowitz Citation2002; Phan, Siegel, and Wright Citation2005; Nauwelaers, Kleibrink, and Stancova Citation2014). Moreover, this support and need for stakeholder diversity might call for the involvement of stakeholders typically not considered, namely civic society stakeholders. As Dubois, Kristensen, and Teräs (Citation2017) highlights, new governance approaches in SPAs may require the broadening of the notion of entrepreneur, taking essentially the form of Quadruple Helix initiatives, by promoting the inclusion of civil society actors at different phases of the regional development process. The creation and strengthening of both physical and virtual infrastructures may also be a more natural route for promoting cooperation in SPAs, since low numbers of similar organizations might hinder initial efforts to establish dense networks in the S3 implementation phase. Second, policy efforts in the form of support infrastructures likely also contribute towards establishing informal and formal collaborative linkages. This is particularly important in the context of SPAs, where the likelihood of serendipitous contact is less likely to occur, due to extensive geographical distances and low numbers of actors. Taking a step-wise policy-approach, policymakers may be advised to first encourage informal collaboration between stakeholders with a similar knowledge background, since cognitive proximity eases informal network relationships, and only later support the connection among stakeholders with formal agreements. Once dense informal networks are in place, it becomes easier to connect these sub-networks of different types of organizations via formal linkages, as previous evidence from technology-based small firms also suggests (Martin-Rios and Erhardt Citation2017).
Furthermore, to overcome significant geographical distances between SPAs stakeholders, policy efforts and investments may be needed to actively develop and support different types of interactions: e.g. providing R&D subsidies that have proved to be successful for stimulating collaboration initiatives (Ahn, Lee, and Mortara Citation2020), or organizing virtual and physical regional networking events (this will also facilitate serendipitous contact) to allow for temporal geographical proximity between distant partners (Bathelt and Spigel Citation2012).
Our study faces several limitations. First, we do not have information on the relationships developed for non-S3 activities; hence, we have not been able to assess potential overlap with other non-S3 activities. Moreover, we have not assessed the role of external collaboration networks in SPAs. While the previous work of Andersson and Karlsson (Citation2007) on regional economic growth in a similar context (Sweden) has suggested that extra-regional knowledge accessibility is insignificant for growth, this knowledge can be important in the S3-context for reducing issues related to lock-in effects of local stakeholders. Further studies could thus investigate the boundaries of inter-regional networks to take into account the potential influence of external knowledge and inter-regional networks. Second, the cross-sectional nature of our study means that we lack information on the dynamics of S3 implementation in the SPA under investigation. In addition, we have no information about possible causal effects between informal and formal relationships, or vice versa. In the context of regional clusters, Molina-Morales et al. (Citation2015) show that proximity dimensions can negatively affect the creation of linkages in advanced stages of the cluster life cycle. Hence future SPA studies could accompany the S3 implementation process, to address how the interaction of different proximity dimensions may change in their role for informal and formal relationships as part of investigations into possible non-linear relationships between proximity dimensions and the propensity for the formation of relationships. Finally, although Lapland can be considered as a representative European SPA, SPAs can still be characterized by rather different social and cultural features (Le Tourneau Citation2020), thereby potentially limiting the transferability of our results. Therefore, further comparative analyses with other SPAs are needed for a more comprehensive assessment of the role of proximity dimensions for networking activities aimed to support the implementation of S3.
Supplemental Material
Download MS Word (159.1 KB)Supplemental data
Supplemental data for this article can be accessed https://doi.org/10.1080/13511610.2021.1879631
Notes
1 For example, the steering group of Lapland's Arctic Specialisation Programme had participants from the Regional Council of Lapland; Lapland Centre for Economic Development, Transport and the Environment; Lapland Chamber of Commerce; Municipality of Sodankylä; City of Rovaniemi; University of Lapland; The Sámi Education Institute; City of Kemi; Municipality of Pelkosenniemi; Lapland University of Applied Sciences; Finnish Forest Research Institute.
2 Kemi Technology Park; University of Lapland; Lapland University of Applied Sciences; ProAgria; Geological Survey of Finland; Natural Resources Institute Finland.
References
- Ahn, J. M., W. Lee, and L. Mortara. 2020. “Do Government R&D Subsidies Stimulate Collaboration Initiatives in Private Firms?” Technological Forecasting and Social Change 151: 119840.
- Andersson, M., and C. Karlsson. 2007. “Knowledge in Regional Economic Growth – The Role of Knowledge Accessibility.” Industry and Innovation 14 (2): 129–149.
- Aranguren, M. J., E. Magro, M. Navarro, and J. R. Wilson. 2019. “Governance of the Territorial Entrepreneurial Discovery Process: Looking Under the Bonnet of RIS3.” Regional Studies 53 (4): 451–461.
- Asheim, B. T. 2019. “Smart Specialisation, Innovation Policy and Regional Innovation Systems: What About New Path Development in Less Innovative Regions?” Innovation: The European Journal of Social Science Research 32 (1): 8–25.
- Asheim, B., M. Grillitsch, and M. Trippl. 2017. “Smart Specialization as an Innovation-Driven Strategy for Economic Diversification: Examples from Scandinavian Regions.” In Advances in the Theory and Practice of Smart Specialization, edited by S. Radosevic, A. Curaj, R. Gheorghiu, L. Andreescu, and I. Wade, 73–98. London: Academic Press.
- Auri, E., J. Harkko, B. Heikkinen, T. Hirvonen, A. Jolkkonen, P. Kahila, A. Kurvinen, et al. 2019. “Vaikutusten jäljillä. Kestävää kasvua ja työtä rakennerahasto-ohjelman vaikuttavuuden arviointi. Rakennerahasto-ohjelman arvioinnin väliraportti 3.” https://www.rakennerahastot.fi/documents/10179/1700053/Vaikutusten+j%C3%A4ljill%C3%A4%20%E2%80%93%20vaikuttavuusarviointi+7.1.2019/b7ad32cc-2cd6-4e66-8f1f-53e791890e1a.
- Balland, P.-A. 2012. “Proximity and the Evolution of Collaboration Networks: Evidence from Research and Development Projects within the Global Navigation Satellite System (GNSS) Industry.” Regional Studies 46 (6): 741–756.
- Balland, P.-A., J. A. Belso-Martínez, and A. Morrison. 2016. “The Dynamics of Technical and Business Knowledge Networks in Industrial Clusters: Embeddedness, Status, or Proximity?” Economic Geography 92 (1): 35–60.
- Balland, P.-A., R. Boschma, J. Crespo, and D. L. Rigby. 2019. “Smart Specialization Policy in the European Union: Relatedness, Knowledge Complexity and Regional Diversification.” Regional Studies 53 (9): 1252–1268.
- Balland, P.-A., R. Boschma, and K. Frenken. 2014. “Proximity and Innovation: From Statics to Dynamics.” Regional Studies 49 (6): 907–920.
- Barca, F. 2009. “An Agenda for Reformed Cohesion Policy: A Place-Based Approach to Meeting European Union Challenges and Expectations.” Independent Report prepared at the request of Danuta Hubner, Commissioner for Regional Policy, European Commission, Brussels.
- Bathelt, H., and B. Spigel. 2012. “The Spatial Economy of North American Trade Fairs.” Canadian Geographer 56 (1): 18–38.
- Becattini, G. 1979. “Dal settore industriale al distretto industriale. Alcune considerazioni sull’unitá di indagine dell’economia industriale.” Rivista di Economia e Politica Industriale 5 (1): 7–21.
- Benner, M. 2015. “From Smart Specialisation to Smart Experimentation.” Zeitschrift für Wirtschaftsgeographie 58 (1): 33–49.
- Boschma, R. A. 2005. “Proximity and Innovation: A Critical Assessment.” Regional Studies 39 (1): 61–74.
- Boschma, R., P.-A. Balland, and M. de Vaan. 2014. “The Formation of Economic Networks: A Proximity Approach.” In Regional Development and Proximity Relations, edited by A. Torre and F. Wallet, 243–266. Cheltenham: Edward Elgar Publishing.
- Boschma, R., and C. Gianelle. 2013. “Regional Branching and Smart Specialization Policy.” JRC technical reports, No. 06/2104.
- Breschi, S., and F. Lissoni. 2001. “Knowledge Spillovers and Local Innovation Systems: A Critical Survey.” Industrial and Corporate Change 10 (4): 975–1005.
- Broekel, T., and R. Boschma. 2012. “Knowledge Networks in the Dutch Aviation Industry: The Proximity Paradox.” Journal of Economic Geography 12 (2): 409–433.
- Caloffi, A., S. Pryke, S. R. Sedita, and M. Siemiatycki. 2017. “Public–Private Partnerships and Beyond: Potential for Innovation and Sustainable Development.” Environment and Planning C: Politics and Space 35 (5): 739–745.
- Cao, Z., B. Derudder, and Z. Peng. 2019. “Interaction Between Different Forms of Proximity in Inter-Organizational Scientific Collaboration: The Case of Medical Sciences Research Network in the Yangtze River Delta Region.” Papers in Regional Science 98 (5): 1903–1924.
- Capone, F., and L. Lazzaretti. 2018. “The Different Roles of Proximity in Multiple Informal Network Relationships: Evidence from the Cluster of High Technology Applied to Cultural Goods in Tuscany.” Industry and Innovation 25 (9): 897–917.
- Carayannis, E. G., and R. Rakhmatullin. 2014. “The Quadruple/Quintuple Innovation Helixes and Smart Specialisation Strategies for Sustainable and Inclusive Growth in Europe and Beyond.” Journal of the Knowledge Economy 5 (2): 212–239.
- Carson, D., R. O. Rasmussen, P. Ensign, and L. Huskey. 2011. Demography at the Edge: Remote Human Populations in Developed Nations. Abingdon: Routledge.
- Cooke, P., and K. Morgan. 1993. “The Network Paradigm: New Departures in Corporate and Regional Development.” Environment & Planning D: Society & Space 11 (5): 543–564.
- Davis, J. A., and S. Leinhardt. 1972. “The Structure of Positive Interpersonal Relations in Small Groups.” In Sociological Theories in Progress, Volume 2, edited by J. Berger, 218–251. Boston: Houghton Mifflin.
- Dei Ottati, G. 1994. “Trust, Interlinking Transactions and Credit in the Industrial District.” Cambridge Journal of Economics 18 (6): 529–546.
- del Castillo, J., J. Paton, and B. Barroeta. 2014. “Territorial Governance in the Context of RIS3 Smart Specialisation Strategy.” Paper presented at the 54th Congress of the European Regional Science Association, St. Petersburg, 26–29 August.
- Dubois, A., I. Kristensen, and J. Teräs. 2017. “Outsmarting Geography: Implementing Territorial Innovation Strategies in Sparsely Populated Regions.” European Planning Studies 25 (8): 1316–1333.
- Dubois, A., and J. Roto. 2012. “Making the Best of Europe’s Sparsely Populated Areas. On Making Geographic Specificity a Driver for Territorial Development in Europe.” NORDREGIO Working Paper 2012: 15.
- Etzkowitz, H. 2002. “Incubation of Incubators: Innovation as a Triple Helix of University-Industry-Government Networks.” Science and Public Policy 29 (2): 115–128.
- EU. 2018. “S3 Platform.” http://s3platform.jrc.ec.europa.eu/what-is-smart-specialisation.
- Fitjar, R. D., and A. Rodríguez-Pose. 2011. “Innovating in the Periphery: Firms, Values and Innovation in Southwest Norway.” European Planning Studies 19 (4): 555–574.
- Foray, D. 2014. Smart Specialisation: Opportunities and Challenges for Regional Innovation Policy. London: Routledge.
- Foray, D. 2018. “Smart Specialisation Strategies and Industrial Modernisation in European Regions—Theory and Practice.” Cambridge Journal of Economics 42 (6): 1505–1520.
- Foray, D., P. A. David, and B. H. Hall. 2011. “Smart Specialization. From Academic Idea to Political Instrument, the Surprising Career of a Concept and the Difficulties Involved in Its Implementation.” MTEI Working Paper, November. Lausanne: MTEI.
- Ghinoi, S., B. Steiner, T. Makkonen, and R. Hassink. 2020. “Smart Specialization Strategies in the Periphery: A Data Triangulation Approach to Governance Issues and Practices.” Regional Studies (in press). doi:10.1080/00343404.2020.1791321.
- Gianelle, C., F. Guzzo, and K. Mieszkowski. 2020. “Smart Specialisation: What Gets Lost in Translation from Concept to Practice?” Regional Studies 54 (10): 1377–1388.
- Grillitsch, M. 2016. “Institutions, Smart Specialisation Dynamics and Policy.” Environment and Planning C: Government and Policy 34: 22–37.
- Habersetzer, A., S. Grèzes-Bürcher, R. Boschma, and H. Mayer. 2019. “Enterprise-related Social Capital as a Driver of Firm Growth in the Periphery?” Journal of Rural Studies 65: 143–151.
- Handcock, M. S., D. R. Hunter, C. T. Butts, S. M. Goodreau, and M. Morris. 2003. statnet: Software Tools for the Statistical Modeling of Network Data. http://statnetproject.org.
- Hansen, T. 2015. “Substitution or Overlap? The Relations Between Geographical and Non-spatial Proximity Dimensions in Collaborative Innovation Projects.” Regional Studies 49 (10): 1672–1684.
- Huggins, R., and A. Johnston. 2010. “Knowledge Flow and Inter-Firm Networks: The Influence of Network Resources, Spatial Proximity and Firm Size.” Entrepreneurship and Regional Development 22 (5): 457–484.
- Huggins, R., and D. Prokop. 2017. “Regional Innovation and the Network Structure of University-Industry Links.” In The Empirical and Institutional Dimensions of Smart Specialisation, edited by P. McCann, F. van Oort, and J. Goddard, 57–80. Abingdon: Routledge.
- Huggins, R., and P. Thompson. 2017. “Networks and Regional Economic Growth: A Spatial Analysis of Knowledge Ties.” Environment and Planning A 49 (6): 1247–1265.
- Jaffe, A. B., M. Trajtenberg, and R. Henderson. 1993. “Geographic Localization of Knowledge Spillovers as Evidenced by Patent Citations.” Quarterly Journal of Economics 108 (3): 577–598.
- Kaivo-oja, J., S. Vähäsantanen, A. Karppinen, and T. Haukioja. 2017. “Smart Specialization Strategy and its Operationalization in the Regional Policy: Case Finland.” Business, Management and Education 15 (1): 28–41.
- Kangas, R., and T. Aarrevaara. 2020. “Higher Education Institutions as Knowledge Brokers in Smart Specialisation.” Sustainability 12 (7): 3044.
- Kempton, L., J. Goddard, J. Edwards, F. B. Hegyi, and S. Elena-Pérez. 2013. Universities and Smart Specialisation. S3 Policy Brief Series, No. 03/2013.
- Kirat, T., and Y. Lung. 1999. “Innovation and Proximity: Territories as Loci of Collective Learning Processes.” European Urban and Regional Studies 6 (1): 27–38.
- Knoben, J., and L. A. G. Oerlemans. 2006. “Proximity and Inter-Organizational Collaboration: A Literature Review.” International Journal of Management Reviews 8 (2): 71–89.
- Kroll, H. 2019. “Smart specialisation in economically and institutionally less favoured regions.” In Strategic approaches to regional development: Smart experimentation in less-favoured regions, edited by I. Kristensen, A. Dubois, and J. Teräs, 36–51. Abingdon, UK: Routledge.
- Lagendijk, A., and A. Lorentzen. 2007. “Proximity, Knowledge and Innovation in Peripheral Regions. On the Intersection Between Geographical and Organizational Proximity.” European Planning Studies 15 (4): 457–466.
- Laursen, K., T. Reichstein, and A. Salter. 2011. “Exploring the Effect of Geographical Proximity and University Quality on University–Industry Collaboration in the United Kingdom.” Regional Studies 45 (4): 507–523.
- Le Tourneau, F.-M. 2020. “Sparsely Populated Regions as a Specific Geographical Environment.” Journal of Rural Studies 75: 70–79.
- Lundquist, K.-J., and M. Trippl. 2013. “Distance, Proximity and Types of Cross-Border Innovation Systems: A Conceptual Analysis.” Regional Studies 47 (3): 450–460.
- Lusher, D., J. Koskinen, and G. Robins. 2013. Exponential Random Graph Models for Social Networks. Theory, Methods, and Applications. New York: Cambridge University Press.
- Mäenpää, A., and S. Virkkala. 2019. “The Role of Proximity in Peripheral Regions: Smart Experimentation Between Triple Helix Actors.” In Strategic Approaches to Regional Development: Smart Experimentation in Less-Favoured Regions, edited by I. Kristensen, A. Dubois, and J. Teräs, 183–203. Abingdon: Routledge.
- Maghssudipour, A., L. Lazzaretti, and F. Capone. 2020. “The Role of Multiple Ties in Knowledge Networks: Complementarity in the Montefalco Wine Cluster.” Industrial Marketing Management 90: 667–678.
- Malmberg, A., and P. Maskell. 2006. “Localized Learning Revisited.” Growth and Change 37 (1): 1–18.
- Mariussen, Å., R. Rakhmatullin, and L. Stanionyte. 2016. Smart Specialisation: Creating Growth through Transnational Cooperation and Value Chains. JRC Science for Policy Report, EUR, 28049.
- Marshall, A. 1925. Principles of Economics. 8th ed. London: Macmillan.
- Martin-Rios, C., and N. Erhardt. 2017. “Small Business Activity and Knowledge Exchange in Informal Interfirm Networks.” International Small Business Journal 35 (3): 285–305.
- Martin, H., R. Martin, and E. Zukauskaite. 2019. “The Multiple Roles of Demand in New Regional Industrial Path Development: A Conceptual Analysis.” Environment and Planning A 51 (8): 1741–1757.
- Martinus, K. 2018. “Labor Networks Connecting Peripheral Economies to the National Innovation System.” Annals of the American Association of Geographers 108 (3): 845–863.
- McCann, P., and R. Ortega-Argilés. 2013. “Modern Regional Innovation Policy.” Cambridge Journal of Regions, Economy and Society 6 (2): 187–216.
- Molina-Morales, F. X., J. A. Belso-Martínez, F. Más-Verdú, and L. Martínez-Cháfer. 2015. “Formation and Dissolution of Inter-Firm Linkages in Lengthy and Stable Networks in Clusters.” Journal of Business Research 68 (7): 1557–1562.
- Moodysson, J., M. Trippl, and E. Zukauskaite. 2017. “Policy Learning and Smart Specialization: Balancing Policy Change and Continuity for New Regional Industrial Paths.” Science and Public Policy 44 (3): 382–391.
- Morgan, K. 2017. “Nurturing Novelty: Regional Innovation Policy in the Age of Smart Specialisation.” Environment and Planning C: Politics and Space 35 (4): 569–583.
- Morris, M., M. S. Handcock, and D. R. Hunter. 2008. “Specification of Exponential-Family Random Graph Models: Terms and Computational Aspects.” Journal of Statistical Software 24 (4): 1548–7660.
- Nauwelaers, C., A. Kleibrink, and K. Stancova. 2014. The Role of Science Parks in Smart Specialisation Strategies. S3 Policy Brief Series, No. 08/2014.
- Nooteboom, B. 2000. Learning and Innovation in Organizations and Economies. Oxford: Oxford University Press.
- Nooteboom, B., W. Van Haverbeke, G. Duysters, V. Gilsing, and A. van den Oord. 2007. “Optimal Cognitive Distance and Absorptive Capacity.” Research Policy 36: 1016–1034.
- NSPA (Northern Sparsely Populated Areas). 2018. The Northern Sparsely Populated Network. http://www.nspanetwork.eu/.
- OECD. 2017. OECD Territorial Reviews: Northern Sparsely Populated Areas. Paris: OECD Publishing.
- Pagliacci, F., P. Pavone, M. Russo, and A. Giorgi. 2020. “Regional Structural Heterogeneity: Evidence and Policy Implications for RIS3 in Macro-Regional Strategies.” Regional Studies 54 (6): 765–775.
- Phan, P. H., D. S. Siegel, and M. Wright. 2005. “Science Parks and Incubators: Observations, Synthesis and Future Research.” Journal of Business Venturing 20 (2): 165–182.
- Ponds, R., F. van Oort, and K. Frenken. 2007. “The Geographical and Institutional Proximity of Research Collaboration.” Papers in Regional Science 86 (3): 423–443.
- Presutti, M., C. Boari, A. Majocchi, and X. Molina-Morales. 2019. “Distance to Customers, Absorptive Capacity, and Innovation in High-Tech Firms: The Dark Face of Geographical Proximity.” Journal of Small Business Management 57 (2): 343–361.
- Pugh, R. 2018. “Questioning the Implementation of Smart Specialisation: Regional Innovation Policy and Semi-Autonomous Regions.” Environment and Planning C: Politics and Space 36 (3): 530–547.
- Robins, G., P. Pattinson, Y. Kalish, and D. Lusher. 2007. “An Introduction to Exponential Random Graph (p*) Models for Social Networks.” Social Networks 29: 173–191.
- Rodríguez-Pose, A., M. Di Cataldo, and A. Rainoldi. 2014. “The Role of Government Institutions for Smart Specialisation and Regional Development.” RIS3 Policy Brief Series, No. 04/2014.
- Santoalha, A. 2019. “Technological Diversification and Smart Specialisation: The Role of Cooperation.” Regional Studies 53 (9): 1269–1283.
- Scott, J., and P. C. Carrington. 2011. The SAGE Handbook of Social Network Analysis. London: Sage.
- Smart Specialisation Platform. 2012. Guide to Research and Innovation Strategies for Smart Specialisation (RIS 3). Luxembourg: Publications Office of the European Union.
- Snijders, T. A. B., P. E. Pattison, G. L. Robins, and M. S. Handcock. 2006. “New Specifications for Exponential Random Graph Models.” Sociological Methodology 36 (1): 99–153.
- Sörvik, J., J. Teräs, A. Dubois, and M. Pertoldi. 2019. “Smart Specialisation in Sparsely Populated Areas: Challenges, Opportunities and New Openings.” Regional Studies 53 (7): 1070–1080.
- Sotarauta, M. 2018. “Smart Specialization and Place Leadership: Dreaming About Shared Visions, Falling into Policy Traps?” Regional Studies, Regional Science 5 (1): 190–203.
- Teräs, J., A. Dubois, J. Sörvik, and M. Pertoldi. 2015. “Implementing Smart Specialisation in Sparsely Populated Areas.” S3 Working Paper Series n° 10/2015.
- Teräs, J., K. Jokelainen, T. Ejdemo, and D. Örtqvist. 2018. “Smart Specialisation at the Edge of Europe.” In Strategic Approaches to Regional Development: Smart Experimentation in Less-Favoured Regions, edited by I. Kristensen, A. Dubois, and J. Teräs, 71–85. New York: Routledge.
- ter Wal, A. L. J., and R. A. Boschma. 2009. “Applying Social Network Analysis in Economic Geography: Framing Some Key Analytic Issues.” Annals of Regional Science 43: 739–756.
- Torre, A., and A. Rallet. 2005. “Proximity and Localization.” Regional Studies 39 (1): 47–59.
- Tsai, W. 2001. “Knowledge Transfer in Intraorganizational Networks: Effects of Network Position and Absorptive Capacity on Business Unit Innovation and Performance.” Academy of Management Journal 44: 996–1004.
- Uyarra, E., C. Marzocchi, and J. Sörvik. 2018. “How Outward Looking Is Smart Specialisation? Rationales, Drivers and Barriers.” European Planning Studies 26 (12): 2344–2363.
- Uyarra, E., J. Sörvik, and I. Midtkandal. 2014. “Inter-Regional Collaboration in Research and Innovation Strategies for Smart Specialisation (RIS3).” Institute for Prospective Technological Studies and Joint Research Centre (JRC) technical reports, S3 Working Paper Series No. 06/2014, Seville.
- Virkkala, S. 2007. “Innovation and Networking in Peripheral Areas: A Case Study of Emergence and Change in Rural Manufacturing.” European Planning Studies 15 (4): 511–529.
- Virkkala, S., A. Mäenpää, and Å Mariussen. 2017. “A Connectivity Model as a Potential Tool for Smart Specialization Strategies.” European Planning Studies 25 (4): 661–679.
- Zamyatina, N. Y., and A. N. Pilyasov. 2017. “Concept of Proximity: Foreign Experience and Prospects of Application in Russia.” Regional Research of Russia 7 (3): 197–207.