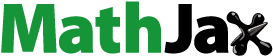
Abstract
In this paper, we argue that China's P2P lending is influenced by the behavioural factors of P2P platforms. This is because severe information asymmetry results in high uncertainty surrounding China's P2P lending industry. Specifically, we examine three behavioural aspects of P2P lending: lending sentiments, herding, and speculation. Using a sample of 918 P2P platforms from October 2015-September 2019, we document that positive P2P news release in the media (sentiments) encourages P2P lending; P2P platforms herd on their peers in making lending decisions; and P2P lending contains speculative elements and is driven by real estate bubbles. Moreover, we find that these behavioural effects are less profound if P2P platforms adopt a fund custody mechanism in which commercial banks provide custodian services for investor funds used for P2P lending. This result suggests that behavioural factors are more important in explaining P2P lending when information asymmetry is more severe. We obtain these results by controlling for other usual factors that can explain P2P lending, including characteristics of P2P platforms, macroeconomic variables, and other variables reflecting features of P2P operating environment. Our results suggest that regulators should monitor risk management of P2P platforms and reduce asymmetric information faced by participants in China's P2P lending market.
1. Introduction
The Chinese P2P lending industry has been experiencing a crisis recently. Fraudulent P2P lending cases have been exposed by the media since 2015. In 2016 one-third of China's P2P lending platforms were ‘problematic’ (Leng Citation2016).Footnote1 The Chinese Banking Regulatory Commission (CBRC) statistics also shows that about 40% of P2P lending platforms in China were in fact Ponzi schemes in 2016. In response to the crisis, the Chinese government has taken a series of measures to clean up the P2P market since later 2015, which aims to curtail small and medium-sized lending platforms.Footnote2
Unlike conventional means of financing, P2P platforms directly connect investors and borrowers through the internet. In mature market economies (hereafter MMEs), P2P platforms mainly function as financial services providers who offer information on borrowers and potential investment projects. They are not financial intermediaries and their financial accounts are separated from that of their clients. However, a striking difference between Chinese P2P lending platforms and their counterparts in MMEs is that many Chinese P2P platforms themselves directly initiate loans to borrowers and raise funds from investors, i.e. they do not focus on providing financial services, instead, they are directly engaged in financing and lending activities (Huang Citation2018). In this context, the conventional principal-agent problem (Stiglitz Citation1974) between investors and borrowers becomes the principal-multiple agents problem. On the one hand, investors are the principal of borrowers. Investors have little information about the creditworthiness of borrowers and they mainly rely on P2P platforms to obtain such information. On the other hand, investors are also the principal of P2P platforms that directly raise and manage funds on behalf of investors. Therefore, the relationship between investors and P2P platforms in China is more complicated than that in MMEs. Due to information asymmetry, investors are not able to judge the quality of P2P platforms, hence moral hazard and adverse selection behaviour of P2P managers will hurt investors’ interest. In addition to the relation between investors and P2P platforms, information asymmetry also exists between P2P firms and borrowers. In this relationship, P2P platforms are not able to distinguish between good and bad-quality borrowers. Hence one would also observe moral hazard and adverse selection behaviour of borrowers. This suggests that P2P firms face risks of not being able to get loans back. Obviously, there should be strong mechanisms to guarantee that P2P platforms do not deviate from the interest of their investors, and that borrowers are capable to repay loans. In MMEs, these mechanisms are in place in the form of laws and regulations on P2P lending, government guarantee schemes, independent third-party credit evaluation institutions, and sophisticated credit information systems. However, formal regulations on P2P online lending were absent in China before 2015. In addition, unlike MMEs where P2P fund suppliers are often institutional investors, P2P capital providers in China are mostly individual investors who seek short-term capital gains. Consequently, both P2P platforms and their investors have strong intention to use the P2P online lending market as a speculative investment tool (Yoon, Li, and Feng Citation2019). On the demand side, P2P borrowers are very risky in China. They are often privately-owned small and medium-sized enterprises (SMEs) and individual consumers who are not able to obtain funds from sources of formal financing. In addition, Chinese national credit information system is still underdeveloped, when carrying out due diligence, P2P lending platforms in China have to rely on their own small-scale individual credit assessment and risk control mechanisms. Moreover, risks embedded in Chinese P2P borrowers are reinforced by higher interest rates charged by P2P lenders, especially privately-owned P2P platforms (Lo et al. Citation2020). In sum, ‘P2P platforms comprise one of the riskiest and least regulated slices of the shadow banking system in China’ (Bloomberg, 2 January 2019).
Due to severe information asymmetry and the lack of regulations, both P2P investors and P2P platforms in China face high uncertainty when making investment and lending decisions. P2P platforms face risks of borrowers’ default, and investors cannot effectively monitor P2P platforms. High uncertainty aggravates moral hazard and adverse selection problems of P2P platforms in relation to their investors. Under such circumstances, one would expect that P2P lending decisions would be heavily influenced by behavioural factors of P2P firms’ managers who make lending decisions. If this is the case, then we would observe that China's P2P lending is responsive to behavioural factors, such as lending sentiments, herding, and speculation.
Against this background, in this paper we examine behavioural factors explaining P2P lending in China based on a sample of 918 P2P platforms during October 2015-September 2019. Our research is timely for the ongoing reshuffling of China's P2P lending industry. Given that the debate about China's P2P lending has been surrounding risks it has brought about to the Chinese financial system, the overarching objective of reshuffling China's P2P industry is to reduce risks embedded in P2P lending. Considering that these risks are likely to be resulted from behavioural factors in making P2P lending decisions, we ask the following research question: to what extent can China's P2P lending be explained by behavioural factors of P2P platforms?
We contribute to the literature in the following aspects: Firstly, we use the most updated data on China's P2P lending platforms during October 2015-Septmber 2019. China's P2P platforms operating in this period are subject to formal regulations, hence information released by P2P platforms in this period should be more reliable, which makes our sample P2P platforms more representative of China's P2P industry. Secondly, we consider different aspects of P2P lenders’ behaviour in one single study, including lending sentiments, herding, and speculation. This allows us to have a comprehensive understanding about different types of behavioural factors affecting P2P lending in China. We are not aware of any existing studies that examine more than one behavioural factors regarding China's P2P lending in a single study.
The paper is structured as follows: in Section 2 we review related literatures. Section 3 contains data, empirical models and measurement of variables. Section 4 discusses empirical results. Section 5 concludes.
2. Literature review
Existing studies on P2P online lending based on MMEs mostly focus on the conflict between investors and borrowers, which is underpinned by the asymmetry information theory (Akerlof Citation1970; Stiglitz and Weiss Citation1981). The central argument concerns that the degree of information asymmetry is higher for P2P lending as compared to traditional financial intermediaries, such as banks. This is because financial intermediaries can provide research, evaluative, and monitoring services more efficiently than individual investors in the market (King and Levine Citation1993). P2P lenders are less able than formal financial intermediaries to invest in systematic mechanisms in collecting information and monitoring borrowers. Therefore, information asymmetry becomes more severe for P2P lending. This explains why many existing researches on P2P lending based on P2P platforms in MMEs focus on how to reduce borrowers’ risks faced by both investors and P2P platforms. More specifically, there are three sub-streams of research on borrower's creditability in the standard literature. Firstly, some studies examine how demographic characteristics of borrowers affect P2P lending (e.g. Herzenstein et al. Citation2008). Secondly, some scholars emphasise the role of borrowers’ social connections in P2P lending (e.g. Lin, Prabhala, and Viswanathan Citation2013; Lu et al. Citation2020). Thirdly, some studies specifically focus on credit scores of borrowers (e.g. Duarte, Siegel, and Young Citation2012; Emekter et al. Citation2015). In sum, the standard literature on P2P lending in MMEs has paid dominating attention to the creditworthiness of P2P borrowers.
Existing literature on China's P2P lending is very thin. Overall, the focus of very few researches is different from that based on P2P lending platforms in MMEs. The central research question regarding China's P2P lending is the extent to which P2P platforms are trustworthy to their investors. Huang (Citation2018) points out that the business model of Chinese P2P lending platforms is different from that in MMEs in which the ‘client segregated account model’ is adopted. In that model, P2P platforms are services providers, they facilitate P2P transactions, but they are not a part of the transaction. However, in the Chinese P2P lending industry, the most popular model before 2016 is the ‘platform lender model’ (Huang Citation2018). In this model, P2P platforms are directly involved in transactions. They originate loans to borrowers and manage a pool of funds on behalf of investors through a collective investment scheme.
The above-mentioned business model of China's P2P lending industry suggests that moral hazard and adverse selection problems are very serious in China's P2P lending industry. This explains why many P2P platforms in China are in fact Ponzi schemes (see the introduction). Therefore, among very few studies on Chinese P2P lending, scholars have paid great attention to P2P platforms themselves rather than borrowers. More specifically, studies mainly focus on P2P platforms’ characteristics that may make P2P platforms more trustworthy. For example, Lan, Hua, and Liu (Citation2018) document the importance of social capital owned by P2P platforms for their survival. They measure P2P platforms’ social capital by online posts on its website and online communication activities of the P2P platform. Yang et al. (Citation2017) use data from a survey of 358 Chinese P2P platforms in 2016 and find that investors’ trust on the P2P platform enhances their intention to invest in the P2P platform repeatedly. In addition, Li and Yang (Citation2019) examine whether ownership background of P2P platforms affect P2P transaction volume. They find that P2P firms owned by venture capitalists, state-owned enterprises (SOEs), listed companies, and banks would lend more than privately-owned P2P firms because private ones are riskier as perceived by investors and borrowers. Jiang et al. (Citation2019) also document that SOE-affiliated P2P platforms in China survive better during market downturns and they have higher trading volumes, attract more investors, and offer lower interest rates as compared to non-SOE-affiliated P2P platforms.
Given that moral hazard and adverse selection problems are more severe in China's P2P industry, one can conjecture that P2P platforms (lenders) are more likely to take risks and speculate in making lending decisions. Yoon, Li, and Feng (Citation2019) provide indirect evidence that China's P2P lending market is speculative in the sense that more revenue from the gambling industry is positively associated with higher default risk of P2P platforms. More recently, Allen et al. (Citation2019) study another element of China's shadow banking system: entrusted loans. They document that about half of nonaffiliated entrusted loans flew into the Chinese real estate industry and these loans are more likely to have problematic performance ex post. Because China's real estate industry has accumulated a lot of bubbles over the years and there were regulations that restrict financing of the formal banking sector from lending to the real estate industry, the result provided by Allen et al. (Citation2019) can be seen as evidence that lenders of nonaffiliated entrusted loans have been chasing higher returns in the Chinese real estate industry. If this is the case, then we would expect that China's P2P lenders behave similarly to lenders of nonaffiliated entrusted loans. In fact, it is very likely that P2P lenders overlap lenders of nonaffiliated entrusted loans in China.
When making decisions facing severe information asymmetry and high uncertainty, economy agents intend to herd on other people's decisions, hence the localised conformity of behaviour can be observed (Bikhchandani, Hirshleifer, and Welch Citation1992). There are a few studies documenting herding behaviour in P2P lending based on P2P platforms in MMEs. For example, Herzenstein, Dholakia, and Andrews (Citation2011) document herding behaviour among Prosper's lenders. They find that lenders are likely to bid in the presence of more existing bids, which results in that a loan application that has already received lending offers is more likely to attract more offers. Zhang and Liu (Citation2012) provide evidence of rational herding among Prosper's lenders and document that lenders do not only mimic peers’ lending decisions, but also make use of publicly observable borrowers’ characteristics in making their own decisions, hence it is ‘rational herding’. Lee and Lee (Citation2012) examine a large sample of P2P daily transactions and provide strong evidence of P2P lenders’ herding behaviour in Korea. Recently, studies on herding bahaviour based on Chinese P2P platforms have emerged. For example, Caglayan, Talavera, and Zhang (Citation2019) investigate investors’ bidding bahaviour based on transactions data from RenRenDai during October 2010-October 2018. They document that investors herd in bidding in the sense that they prefer projects that had already attracted strong interests in previous periods. Moreover, the authors find that investors who are active in the platform only for a short duration of the bidding process and more experienced investors are more likely to herd. In another study, Jiang et al. (Citation2018) argue that automatic bidding can reduce investors’ herding behaviour as compared to manual bidding. Using transactions data from PaiPaiDai in 2015, they document that automatic bidding weakens investors’ herding behaviour. Moreover, automatic bidding produces a rational herding because the outcomes of automatic bidding are very similar to the observed pattern of loan amount and loan period.
Apart from herding behaviour, lending decisions are also often influenced by P2P lenders’ perception of the industry. A recently growing literature has shown that measures from various aspects of newspaper articles and other textual sources are documented to have power in explaining stock market returns. According to Shiller (Citation2000) investors follow the printed word even though much of it is pure hype, suggesting that market sentiment is driven by news content. Along this line of argument, Calomiris and Mamaysky (Citation2018) use the aggregate news at a monthly horizon from the Thomson Reuters Machine Readable News Archive to derive measures of word flow as news measures. They find that these news measures have predictive powers in explaining stock market risks and returns. Moreover, Garcia (Citation2013) constructs investor sentiment from media contents by counting the number of positive and negative words from two financial columns published in the New York Times. The author finds that positive words embodied in the news help predict stock returns. Moreover, this news effect is more profound during economic recessions. In sum, the above-mentioned studies justify that it is meaningful to derive a measure of lending sentiments from news contents. In the empirical analysis of this paper, we use positive P2P news release to derive a measure of lending sentiments and examine whether it affects P2P online lending in China.
3. Empirical design
3.1. Data
The data on P2P platforms used in the empirical analysis of this paper is taken from China P2P lending dataset compiled by the Home of online lending (Wangdaizhijia). Wangdaizhijia was established in October 2011 as China's first authoritative P2P online lending portal. It contains original data of all P2P platforms in China. The data, reports, and opinions published by Wangdaizhijia are often regarded as most influential in the Chinese online lending industry. Apart from information on P2P platforms, macro-and industrial-level data are taken from the WIND database, which is produced by China's leading financial data service provider WIND. WIND database is internationally recognised, and it has been widely used in academic research on China. We select the sample period from October 2015-September 2019 because this is the period during which the Chinese P2P lending industry is officially regulated. Before late 2015, there were no explicit regulations on P2P platforms in China (Huang Citation2018). Therefore, P2P lending platforms in this period are closer to their counterparts in MMEs and they are mostly in line with future development of China's P2P lending industry. We started from 1041 P2P platforms during the sample period. We excluded 123 P2P platforms that either have zero turnovers, or that have extreme values in other variables. We ended up with 918 P2P platforms for which we have monthly information during October 2015-September 2019, which forms an unbalanced pane dataset.
3.2 Empirical models, measurement of variables, and descriptive statistics
We use the following fixed effect panel data modelFootnote3 to explain total lending volume of P2P platforms during the sample period.
(1)
(1)
In model (1) the dependent variable is P2P lending (volume) which is measured by the natural logarithm of total monthly lending volume of the P2P firm. P2P lending is related to profitability of the P2P platform since the firm's revenue is proportional to its lending. Our key independent variable in model (1) is ‘behaviour’. It stands for three behavioural aspects of P2P lending, i.e. lending sentiments, herding, and speculation, respectively. We explain how to construct the proxy for these behavioural aspects (‘behaviour’) in the next paragraph. In model (1), represents regression coefficients, i represents P2P platforms, t represents time (months), and
is the residual.
We are interested in three aspects of P2P lenders’ behaviour. Firstly, lending sentiments (news1). It captures P2P lenders’ overall perception of the P2P industry in response to positive P2P news release in the media. We manually collect news titles published in the news column of the website of Home of Online Lending (Wangdaizhijia). We define positive news as that either the news title explicitly contains positive words about P2P or the title's tone is positive about P2P, we then count the number of pieces of positive news within a month. We denote this measure as ‘news1’. This measure of positive news can be justified by the literature. For example, Garcia (Citation2013) constructs investor sentiment from media contents by counting the number of positive and negative words from two financial columns published in the New York Times. The author finds that positive words embodied in the news help predict stock returns. We stress that the news column of Home of Online Lending (Wangdaizhijia) is specific on the P2P industry and they mostly report on recent events in this industry both locally and nationwide. Given server information asymmetry and high uncertainty in China's P2P lending industry, we believe that P2P lenders would be very sensitive to the related news.
The second behavioural aspect we examine in the paper concerns herding behaviour of P2P platforms (lenders). Although herding behaviour has been documented for P2P lenders outside China (e.g. Herzenstein, Dholakia, and Andrews Citation2011), we are not aware of any evidence of herding in Chinese P2P online lending. In this paper, herding refers to the situation in which P2P platforms mimic lending decisions of their peers. We define peers of a P2P platform as other P2P platforms in the same province in which the P2P platform operates. If a P2P platform herds, then we should observe that this P2P firm's lending will be driven by lending decisions of its peers. If this is the case, then the lending volume of this P2P platform can be explained by the average lending volume of its peers. Therefore, we use the natural logarithm of the monthly average lending volume of other P2P firms in the same province (excluding the P2P firm of concern) as a proxy for herding (herd1).
The third behavioural aspect we examine in this paper is the speculative element of China's P2P lending. More specifically, we use the price inflation of the provincial commercial property market as a proxy for speculation (speculation1). Given that China's real estate market is perhaps the most representative industry that reflects bubbles in the Chinese economy, if P2P lenders are speculative, then we would expect to see that P2P lending should be associated with the price inflation of commercial properties in the province in which the P2P platform operates. Therefore, from the WIND database we collect monthly information on total sales and total sold area of commercial properties in the province. We then compute the ex post monthly unit selling price, based on which we calculate the monthly growth rate of selling price of commercial properties in the province (speculation1).
Apart from the above-mentioned key independent variables, we control for usual factors documented in the existing literature that can explain P2P lending. We classify control variables in model (1) into two groups: characteristics of P2P platforms and macro-level variables. P2P platform-level variables include: (1) P2P size (size), which is measured by the natural logarithm of the registration capital of the P2P platform. (2) P2P age (age), which is measured by the natural logarithm of the number of months since the P2P firm started operation in the online lending industry. The longer a P2P platform has been operating in the business, the more likely the firm has established a larger client network. But if P2P firms are speculative, then they do not have strong incentives to invest in risk management mechanisms, they may only survive for a short-term due to fierce competition. (3) Number of investors (investor), which is measured by the natural logarithm of total number of investors who have made at least one investment via this online lending platform. Total number of investors is relevant because it indicates the supply of funds available for P2P lending. (4) Number of borrowers (borrower), which is measured by the natural logarithm of total number of borrowers who have borrowed at least once from the P2P platform. Total number of borrowers is an indicator of the demand for P2P lending. (5) Loan duration (duration). We use average loan duration to proxy for the length of outstanding loans. The platform reports its daily average loan duration across loans. We calculate the average of daily average loan duration over a month. (6) P2P interest rate (interest). It is the average of the P2P lending platform's ’s daily interest rate over a month. The P2P platform reports its daily interest rate which is the weighted average interest rate across loans. P2P interest rate carries two pieces of information. It is the rate of return for P2P lending, meanwhile it is the cost of borrowing for borrowers.
We also consider the following macro-level factors as control variables: (1) Economic growth (growth), which is measured by GDP growth rate. Macroeconomic performance should be a fundamental driver for the growth of the P2P lending industry. Because there is no monthly data on GDP growth, we use the quarterly growth rate of GDP for the corresponding month, the data is taken from WIND. (2) Total new credit (credit), which is measured by the ratio of total new credit to GDP in the same period. Total new credit refers to total amount of new loans issued by banks and financial institutions in a month. Total new credit matters for the scale of P2P lending. This is because liquidity in the financial system affects both the demand for and supply of P2P financing through either the availability of liquidity or the changes in the interest rate in the financial system. Information on total new credit is taken from WIND. (3) Internet penetration (internet), which is measured by the ratio of population who has access to the internet to total population in the same period. We collect the number of internet users in each month, and then scale it by total population in the corresponding month. The data is taken from WIND. The internet is the infrastructure for P2P transactions. (4) Stock market volatility (volatility). We use domestic stock market volatility as a proxy for uncertainty embedded in the Chinese financial system. Participants in the P2P lending market should be responsive to financial market volatilities. More specifically, we first compute monthly variance of the Shanghai composite index and the Shenzhen composite index, respectively, and then we take the average of monthly variances of the two stock markets. The data is taken from WIND.
Table summarises descriptive statistics of the variables used in the empirical analysis. Table shows correlation coefficients. An average P2P platform in our sample has more investors than borrowers. The mean value of the logarithm of total number of investors is 7.718 (median 7.583), whereas the mean value of the log of total number of borrowers is 5.372 (median 4.878). The mean of the logarithm of loan duration is 1.329, which indicates that P2P online lending platforms mainly lend money for a short period. On average, over the sample period, the median number of positive news on P2P in a month is 5 (news1) and positive P2P news appeared in over half of months (53.3%) covered by the sample period.
Table 1. Descriptive statistics.
Table 2. Correlation analysis.
4. Empirical results
4.1. Main test on the whole sample
Table presents the results of estimating the empirical model (1) for the whole sample. Columns (1)–(3) in Table refer to the estimation in which a specific measure of behavioural variable is used separately, and column (4) reports the results when all measures of behavioural variables are included in the estimation.
Table 3. Impact of behavioural factors on P2P lending: main test on the whole sample.
We first discuss the results regarding the behavioural variables. Overall, the estimated coefficients for indicators of behaviroural variables are all positively significant in Table , which suggests that China's P2P lending is indeed influenced by behavioural factors related to P2P lending platforms. More specifically, we observe that (a) P2P lending responds positively to positive news about the P2P industry (news1). We interpret this result as that P2P lenders show a stronger lending sentiment in response to more positive news on P2P. It is important to mention that this sentiment effect may also be reflected by other participants in the P2P lending market, including investors and borrowers. However, after controlling for both the supply side (investor) and the demand side (borrower) effects (see the empirical model (1)), we believe that the estimated coefficient regarding the variable ‘news1’ should carry lending sentiments of P2P platforms. (b) Lending volume of a specific P2P platform is positively explained by lending volume of its peers. Column (2) of Table shows that the estimated coefficient for the average lending volume of other P2P platforms in the same province (excluding the P2P firm of concern) (herd1) is highly and positively significant. This result suggests that herding behaviour is an important feature of China's P2P lending. This result is consistent with the literature on herding behaviour in P2P lenders in MMEs (e.g. Herzenstein, Dholakia, and Andrews Citation2011). (c) P2P lending is positively explained by the price inflation of commercial properties in the province (speculation1). This suggests that China's P2P lending is likely to be driven by bubbles in the real estate market. This result is consistent with Allen et al. (Citation2019) who document that more than half of China's nonaffiliated entrusted loans, another element of the Chinese shadow banking system, flew into China's real estate industry. According to Allen et al. (Citation2019), this is because Chinese formal financing channels are restricted from investing in the real estate market, hence some investment in the real estate industry had to be financed by shadow banking activities. In the empirical analysis of this paper, we consider this financing effect by controlling for total new credit (credit) in formal financing channels in the estimation. After controlling for liquidity from formal financing channels, we believe that the estimated coefficient for the price inflation of commercial properties (speculation1) can be seen as the evidence of speculation of P2P lending. China's real estate market is the most representative industry that reflects bubbles in the Chinese economy. The qualitative nature of the above-mentioned results remains in column (4) when three different behavioural factors are considered in the same estimation.
Regarding control variables in Table , we observe that (a) the estimated coefficient for P2P firm size (size) is insignificant. (b) the estimated coefficient for P2P firm age (age) is negatively significant in explaining P2P lending. This suggests that the longer the P2P firm has been operating in the industry, the less lending the firm makes. This result may be explained by the notion that many P2P platforms that entered into China's P2P lending market at earlier stages were less sustainable, and they have gradually disappeared from the market after late 2015 as the result of the government's cleaning up of this market. (c) the estimated coefficient for the number of investors (investor) is highly and positively significant. More investors imply stronger supply of funds, which supports P2P lending. (d) the estimated coefficient for the number of borrowers (borrower) is highly and positively significant, confirming the demand effect. (e) the estimated coefficient for average loan duration (duration) is not significant. (f) the estimated coefficient for P2P interest rate (interest rate) is positively significant, which suggests that high lending interest rates do not deter demand for P2P lending.
Regarding macro-level factors, the results show that (a) GDP growth (growth) positively explains the scale of P2P lending, which is logical. Strong overall economic performance increases the demand for financing from P2P lending on the one hand, and on the other it also increases the supply of capital available for P2P lending. (b) The estimated coefficient for total new credit (credit) is positively significant in columns (2)–(4), which suggests that when total new credit is higher in formal financing channels, P2P lending is also higher. This result can be explained by a few possibilities. First, P2P borrowers in China do not have access to formal financing channels to the extent they wish. If they do have sufficient access to formal financing, then when there is more liquidity available in formal financing channels, the demand for P2P financing should be lower. We find the opposite. Second, the overall interest rate may become lower when liquidity in formal financing channels is higher. Lower overall interest rate in the financial system provides downward pressure on interest rates charged by P2P lending, which might attract more borrowers to the P2P market. Third, there may be a liquidity leakage from formal financing channels to P2P lending. This refers to that when liquidity in formal financing channels is high, some funds will be leaked to informal financing channels, such as P2P lending. For example, some P2P platforms are set up as subordinates of SOEs. These SOEs can obtain cheaper loans from formal financing channels due to their connections with the government, they then use these borrowings to invest in P2P lending to seek for higher capital gains.Footnote4 Yao, Gu, and Cao (Citation2019) claim that there exists a ‘leakage effect’ between SOEs and private equity (PE) investment in China. They argue that when the government controls the interest rate and/or the credit allocation, SOEs obtain funds from banks by the officially controlled interest rate. They then ‘relend’ their credits to PEs in the ‘black’ market. (c) The estimated coefficient for the internet accessibility (internet) is also highly and positively significant. The internet is the infrastructure for participants to carry out P2P transactions. (d) the estimated coefficient for stock market volatility (volatility) is negatively significant. This suggests that uncertainties embedded in the Chinese financial system not only discourage the supply of funds from investors but also reduce demand for P2P lending from borrowers due to weak real economic activities.
4.2. Robustness tests on the whole sample
We conduct a set of robustness tests based on the whole sample by constructing alternative measures for behavioural aspects of P2P lenders. Firstly, we replace the variable news1 by news2. The variable news2 is a dummy variable, which takes the value of one if there appears to be positive news on P2P in this month, otherwise it is zero. Similar to the construction of the variable news1, we define positive news as that either the news title explicitly contains positive words about P2P or the title's tone is positive about P2P. Therefore, the difference between news1 and news2 is that news1 is a count variable, whereas news2 is a dummy variable. Secondly, we construct an alternative measure of herding. In the main test shown in Table , when constructing the proxy for herding (herd1), we define peers of a P2P platform as other P2P platforms in the same province in which the P2P platform operates. Here in the robustness test, we define the peers of a P2P platform as other P2P platforms in the P2P industry (nationwide). Considering that we do not have information on the entire population of P2P platforms in the whole industry, we use the available P2P platforms in our sample. More specifically, we use the nature logarithm of the monthly average lending volume of other P2P platforms in our sample (excluding the P2P platform of concern) as the proxy for herding (herd2). Thirdly, regarding the proxy for speculation, in the main test shown in Table , we use the monthly growth rate of selling price of commercial properties in the province (speculation1). Here in the robustness test we use the monthly growth rate of total investment in the real estate industry (speculation2) as an alternative measure.
Table displays the results of estimating empirical model (1) by using alternative measures of behavioural variables. As we can see from Table , the qualitative conclusion regarding these behavioural variables clearly confirms what we obtained in Table . In addition, the estimated results regarding control variables are also consistent with the results for these variables shown in Table .
Table 4. Impact of behavioural factors on P2P lending: robustness test on the whole sample.
In summary, both Tables and provide evidence that P2P lending in China is influenced by different behavioural aspects of P2P platforms, including lending sentiments, herding, and speculation. The results on these behavioural aspects are robust across different ways of constructing proxies for behavioural factors. These results are obtained by controlling for other usual factors documented in the literature that can explain P2P lending.
4.3. Further tests based on split samples
We argue that information asymmetry is severe in China between P2P platforms and borrowers on the one hand, and between P2P platforms and investors on the other. Both types of asymmetric information problems would enhance the likelihood that P2P platforms’ lending decisions are influenced by behavioural factors, such as lending sentiments, herding, and speculation. These behavioural aspects should be more important in explaining P2P lending when the degree of information asymmetry is larger between P2P platforms and investors. In this case, the adverse selection and moral hazard problems of P2P platforms are more severe, which leads to stronger influence of behavioural factors. In this section, we conduct another set of robustness test by using subsamples. More specifically, we split the sample by whether the P2P platform has adopted a fund custody mechanism. Since 2016 Chinese P2P regulations have been encouraging P2P platforms to adopt a fund custody mechanism. In response, some P2P platforms have signed agreements with commercial banks that are willing to provide custodian services for investor funds used for P2P lending transactions. Obviously, the adoption of a fund custody mechanism can reduce adverse selection and moral hazard problems between investors and P2P platforms. This is because in a fund custody mechanism commercial banks function as a monitor of P2P platforms on behalf of investors, which reduces the degree of asymmetric information between investors and P2P platforms. Therefore, it disincentivizes P2P platforms to take irresponsible or risky projects. This suggests that lending decisions made by P2P platforms that have a fund custody mechanism in place should be influenced by behavioural factors to a less extent as compared to P2P platform that have not applied a fund custody mechanism. Therefore, we split the whole sample into P2P platforms that have adopted a fund custody mechanism versus P2P platforms that have not. We estimate the empirical model (1) for the two subsamples. The results are reported in Table for P2P platforms with a fund custody mechanism and Table for P2P platforms without a fund custody mechanism, respectively. For expositional purposes, we report the estimation results of using two alternative measures of behavioural variables in one table for each subsample.
Table 5. Impact of behavioural factors on P2P lending: P2P platforms with a fund custody mechanism.
Table 6. Impact of behavioural factors on P2P lending: P2P platforms without a fund custody mechanism.
Comparing Table with Table , we observe that the results concerning control variables are very consistent with each other and they are in line with the results on these variables shown in both Tables and . However, differences do exist in the results regarding behaviroual variables between Tables and . More specifically, we observe that (a) the estimated coefficients for both news1 and news2 are not significant for P2P firms that have adopted a fund custody mechanism in Table , whereas they are positively significant for P2P firms that have not in Table . (b) Although the estimated coefficient for herd2 is positively significant for both subsamples, the estimated coefficient for herd1 is not significant for P2P firms that have adopted a fund custody mechanism in Table , whereas it is positively significant for P2P firms that have not in Table . (c) Although the estimated coefficient for speculation2 is positively significant for both subsamples, the estimated coefficient for speculation1 is not significant for P2P firms that have adopted a fund custody mechanism (Table ), whereas it is positively significant for P2P firms that have not adopted a fund custody mechanism (Table ). The above-mentioned differences regarding results concerning behavioural factors suggest that P2P lending undertaken by P2P platforms that have not adopted a fund custody mechanism are more likely to be influenced by behaviroual factors than their counterparts. This may be explained by that in P2P platforms that have not adopted a fund custody mechanism, investors suffer more from adverse selection and moral hazard problems, consequently P2P platforms are more likely to be irresponsible and risk-taking.
In summary, the subsample results not only support the results we obtained based on the whole sample regarding how behaviroural factors affect P2P lending, but also suggest that the influence of these behaviroural factors is more profound when information asymmetry between investors and P2P platforms is more severe.
5. Discussions and conclusions
An important feature of Chinese P2P lending industry concerns information asymmetry and lack of regulations before 2015. Under information asymmetry and high uncertainty, adverse selection and moral hazard problems would become more severe, which results in more risk-taking behaviour of P2P lending platforms. Consequently, their lending decisions would be more likely to be influenced by behavioural factors, such as lending sentiments, herding, and speculation.
We provide evidence that China's P2P lending market is significantly influenced by behavioural factors of P2P platforms. Moreover, the behavioural effect becomes more profound if the asymmetric information problem is more serious between investors and P2P platforms. Our study suggests that policymakers should focus on how to reduce asymmetric information faced by participants in China's P2P lending market. This could be done by developing a reliable credit evaluation system in China on the borrowers’ side, and on the side of P2P platforms by setting up more restrictive regulations on risk management mechanisms, such as the compulsory application of a fund custodian mechanism. In addition, some measures should be taken to strengthen corporate governance of P2P platforms.
Acknowledgement
Anonymous referees are acknowledged for comments on earlier versions of this paper.
Disclosure statement
No potential conflict of interest was reported by the author(s).
Notes
1 According to the Home of Online Lending, an organisation that compiles P2P data in China, ‘problematic’ or ‘troubled’ P2P platforms are the ones that have difficulty paying off investors, have been investigated by national economic crime investigation department, or whose owners have run away with investors’ money.
2 For example, in 2015, the central bank issued guidance on promoting healthy development of internet finance. In August 2016, the Chinese Banking Regulatory Commission (CBRC) issued ‘Interim Measures for the Administration of the Business Activities of Online Lending Information Intermediary Institutions’. In June 2017, China issued regulations on internet financial information disclosure, and a series of notices on special rectification, capital management rectification, compliance supervision, and self-regulatory inspection.
3 We include a few macro-level variables in the empirical model. The monthly observation of these variables is the same for all cross-section units (P2P platforms). This makes the GMM estimation procedure difficult to implement. Therefore, we use a panel data fixed effect model. We use the lagged-one observations of explanatory variables to consider the endogeneity issue.
4 Although this is a plausible explanation, it appears to be very difficult to formally test it due to data restrictions.
References
- Akerlof, G. 1970. “The Market for "Lemons": Quality Uncertainty and the Market Mechanism.” The Quarterly Journal of Economics 84: 488–500. doi:https://doi.org/10.2307/1879431
- Allen, F., Y. Qian, G. Tu, and F. Yu. 2019. “Entrusted Loans: A Close Look at China's Shadow Banking System.” Journal of Financial Economics 133: 18–41. doi:https://doi.org/10.1016/j.jfineco.2019.01.006
- Bikhchandani, S., D. Hirshleifer, and I. Welch. 1992. “A Theory of Fads, Fashion, Custom, and Cultural Change as Informational Cascades.” Journal of Political Economy 100: 992–1026. doi:https://doi.org/10.1086/261849
- Caglayan, M., O. Talavera, and W. Zhang. 2019. Herding Behaviour in P2P Lending Markets, BOFIT Discussion Papers 22/2019, Bank of Finland, Institute for Economies in Transition.
- Calomiris, Charles W., and M. Mamaysky. 2018. Harry, How News and Its Context Drive Risk and Returns around the World, Columbia Business School Research Paper No.17-40.
- Duarte, J., S. Siegel, and L. Young. 2012. “Trust and Credit: The Role of Appearance in Peer-to-Peer Lending.” Review of Financial Studies 25: 2455–2484. doi:https://doi.org/10.1093/rfs/hhs071
- Emekter, R., Y. Tu, B. Jirasakuldech, and M. Lu. 2015. “ Evaluating Credit Risk and Loan Performance in Online Peer-to-Peer (P2P) Lending.” Applied Economics 47 (1): 54–70. doi:https://doi.org/10.1080/00036846.2014.962222
- Garcia, D. 2013. “Sentiment During Recessions.” The Journal of Finance 68 (3): 1267–1300. doi:https://doi.org/10.1111/jofi.12027
- Herzenstein, M., R. L. Andrews, U. M. Dholakia, and E. Lyandres. 2008. “ The Democratization of Personal Consumer Loans? Determinants of Success in Online Peer-to-Peer Lending Communities.” Bulletin of the University of Delaware 5 (3): 274–277.
- Herzenstein, M., U. M. Dholakia, and R. L. Andrews. 2011. “ Strategic Herding Behavior in Peer-to-Peer Loan Auctions.” Journal of Interactive Marketing 25 (1): 27–36. doi:https://doi.org/10.1016/j.intmar.2010.07.001
- Huang, R. H. 2018. “ Online P2P Lending and Regulatory Responses in China: Opportunities and Challenges.” European Business Organization Law Review 19 (1): 63–92. doi:https://doi.org/10.1007/s40804-018-0100-z
- Jiang, J., L. Liao, Z. Wang, and X. Zhang. 2019. Government Affiliation and Peer-to-Peer Lending Platforms in China. Available at SSRN: https://ssrn.com/abstract=3116516.
- Jiang, C., Q. Xu, W. Zhang, M. Li, and S. Yang. 2018. “ Does Automatic Bidding Mechanism Affect Herding Bahaviour? Evidence from Online P2P Lending in China.” Journal of Behavioral and Experimental Finance 20: 39–44. doi:https://doi.org/10.1016/j.jbef.2018.07.001
- King, R., and R. Levine. 1993. “ Finance and Growth: Schumpeter Might Be Right.” The Quarterly Journal of Economics 108: 717–737. doi:https://doi.org/10.2307/2118406
- Lan, M., X. Hua, and X. Liu. 2018. Financial Capital or Social Capital: Evidence from the Survival Analysis of Online P2P Lending Platforms, CONF-IRM 2018 Proceedings 3.
- Lee, E., and B. Lee. 2012. “ Herding Behavior in Online P2P Lending: An Empirical Investigation.” Electronic Commerce Research and Applications 11 (5): 495–503. doi:https://doi.org/10.1016/j.elerap.2012.02.001
- Leng, S. 2016. “One-Third of China’s 3,000 Peer-to-Peer Lending Platforms Are ‘Problematic’: New Report.” South China Morning Post https://www.scmp.com/news/hong.
- Li, L., and J. Yang. 2019. “ Study on the Effect Path of Different Equity Backgrounds on the Volume of Transaction of P2P.” Advances in Economics, Business and Management Research 76: 2019.
- Lin, M., N. R. Prabhala, and S. Viswanathan. 2013. “Judging Borrowers by the Company They Keep: Friendship Networks and Information Asymmetry in Online Peer-to-Peer Lending.” Management Science 59 (1): 17–35. doi:https://doi.org/10.1287/mnsc.1120.1560
- Lo, Kai Lisa, Jackson Jinhong Mi, Minhua Yang, and Shuyu Zhang. 2020. “ Do Financial Regulations Have Impacts on Ownership Structure of P2P Firms?” Applied Economics Letters 27 (14): 1156–1159. doi:https://doi.org/10.1080/13504851.2019.1675859
- Lu, H., B. Wang, H. Wang, and T. Zhao. 2020. “ Does Social Capital Matter for Peer-Peer-Lending? Empirical Evidence.” Pacific-Basin Finance Journal 61 (June 2020): 101338. doi:https://doi.org/10.1016/j.pacfin.2020.101338
- Shiller, Robert J. 2000. Irrational Exuberance. Princeton, NJ: Princeton University Press.
- Stiglitz, J. 1974. “Incentives and Risk Sharing in Sharecropping.” The Review of Economic Studies 41 (2): 219–255. doi:https://doi.org/10.2307/2296714
- Stiglitz, J., and A. Weiss. 1981. “ Credit Rationing in Markets with Imperfect Information.” American Economic Review 71: 393–419.
- Yang, M., H. Li, Z. Shao, and W. Shang. 2017. Influencing Lenders’ Repeat Investment Intention in P2P Lending Platforms in China through Signaling, PACIS 2017 Proceedings, 72. http://aisel.aisnet.org/pacis2017/.
- Yao, Z., D. Gu, and W. Cao. 2019. “ SOEs as Intermediation: Leakage Effect Under Financial Repression.” Pacific-Basin Finance Journal 53: 349–361. doi:https://doi.org/10.1016/j.pacfin.2018.12.001
- Yoon, Y., Y. Li, and Y. Feng. 2019. “ Factors Affecting Platform Default Risk in Online Peer-to-Peer (P2P) Lending Business: An Empirical Study Using Chinese Online P2P Platform Data.” Electronic Commerce Research 19 (1): 131–158. doi:https://doi.org/10.1007/s10660-018-9291-1
- Zhang, Juanjuan, and Peng Liu. 2012. “Rational Herding in Microloan Markets.” Management Science 58 (5): 892–912. doi:https://doi.org/10.1287/mnsc.1110.1459