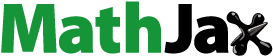
Abstract
The increasing frequency and intensity of catastrophic natural disasters have the potential to stress and imperil banks to the point of compromised viability or even bankruptcy. Using data of approximately 907 domestic/local banks and Spatial Hazard Events and Losses Database for the United States during the period 2010–2019, we explore how natural disasters impact bank stability. Our main findings support the aforementioned hypothesis that natural disasters decrease bank stability because total deposit and equity (capital) become more volatile and the bank is prone to increased lending margins, as well as a provision of loan loss. Thus, banks lose their competitiveness, ROA deteriorates, and Z-score becomes lower. Strong corporate governance and healthy financial strategy, nevertheless, assist bank recovery in the aftermath of these weather extreme events. Last but not least, we find a non-linear relationship between natural disasters and bank stability and posit the role of indemnity paid out from the Federal insurance programme (after natural hazards) in the high-damage group.
1. Introduction
Concurrently expanding with the awareness of adverse effects on smooth functioning of the various agents of the financial system, i.e. financial markets, and payments, settlement and clearing systems,Footnote1 the literature on how climate hazards destabilize financial institutions is emerging (Batten, Sowerbutts, and Tanaka Citation2016 and Breitenstein, Nguyen, and Walther Citation2021 for details).
The growing trend of frequency and intensity of climate-related disasters, such as extreme weather events (i.e. droughts, floods, and heat waves), has stressed banking operation and performance, including reducing the total amount of deposits (Brei, Mohan, and Strobl Citation2019), increasing the share of non-performing loans (Brahmana, Puah, and Chai Citation2016), or even loan portfolio restructuring (Collier, Katchova, and Skees Citation2011). Specifically, disasters may subject banks to insolvency owing to one of the following mechanisms: bank run, immediate withdrawals to replace loss, excessive write-off of loan losses, and collateral destruction or offices damages. Therefore, after disaster event, banks may be exposed to short-term pressure on capital adequacy, asset quality, managerial qualities, and liquidity risk as uncertainty and repayment increase (Cetorelli and Goldberg Citation2011; Collier and Skees Citation2012; Klomp Citation2014; Noth and Schüwer Citation2018).
However, the existing empirical evidence is mixed because the impact of climate risks on financial soundness of banks goes beyond these direct impacts. The ultimate consequence of climatic events has shown both perils and opportunities to operation of financial institutions, among which banking and insurance industry are crucial. For instance, the credit demand shows a rising trend in the aftermath of volcanic activities (Berg and Schrader Citation2012), and banks in unaffected areas find opportunities by providing credits to businesses in disaster-stricken regions (Koetter, Noth, and Rehbein Citation2020). Further, those realized disaster-related damages are affected by local economic structure and disaster management, such as public financial aid programmes supporting corporations and individuals in affected regions, and thus endogenous within local economic conditions. Additionally, banks are often a part of a larger financial conglomerate including an insurance branch, which may also incur substantial losses after a disaster. Hence, banks may choose their business regions and counties with more or less disaster risks for their business activities.
Different kinds of natural hazards may have different effects across different geographical regions (Skidmore and Toya Citation2002; Gassebner, Keck, and Teh Citation2010) since large countries tend to have a higher probability of experiencing a natural event. In particular, the United States (US) would offer an interesting case study of the effects of natural hazards on banking stability because it is one of the top countries which suffers from natural disastersFootnote2, and due to the fact that US banking financial system exhibits the strong influence over the global banking sector. In this study, we, thus, use the Spatial Hazard Events and Losses Database for the United States (SHELDUS)Footnote3 (Figure ) alongside the S&P COMPUSTAT to investigate the impact of natural disaster on banking system as well as providing underlying mechanisms driving the results. Those mechanisms can be both banking financial and governance strategies. As board plays a pivotal role in setting policies, level of corporate governance is usually linked with board characteristics (Iliev and Roth Citation2021). The study highlights the role of corporate governance on bank stability upon the occurrence of natural disasters.
Despite climate change opponents, greenhouse gas, among which CO2 plays the dominant role, emissions are believed to be assertively blamed for the severe changes in weather patterns, e.g. intensity and frequencies of weather extremes. While climate finance is burgeoning (Hong, Li, and Xu Citation2019; Bernstein, Gustafson, and Lewis Citation2019; Hong, Karolyi, and Scheinkman Citation2020; Baldauf, Garlappi, and Yannelis Citation2020; Murfin and Spiegel Citation2020), studies that explore the causality of CO2 emission and natural disasters and their detrimental impact on financial stability are scant. Moreover, United States is the second-largest emitter of greenhouse gas emissions per person in the world. Therefore, it is necessary to consider the effects of consumption-based carbon emission alongside the impact of weather extremes in the causal settings. We employ CO2 emission as an instrument in IV-GMM models.Footnote4
Our findings suggest that banks often recover from negative impacts caused by weather-related natural calamities on their stability and performance. Our approach, which uses two-stage least square, adds to the body of knowledge about how CO2 affects the financial stability in the long run. By looking at the process of how natural disasters affect bank risk, we discover that increasing the interest rate spread does not sufficiently compensate for the risk of natural disasters, but instead induces worse lending activity in the future. Good operating strategy, a sufficient loss buffer, and a strong loan portfolio are all important considerations in ensuring that banks remain resilient in the face of natural disasters. Sub-sample analyses illustrate that those banks unfamiliar with dealing with the aftermath of natural disasters are less equipped to climate risk, whereas those who are more likely to be exposed to disasters are better at effective forecast and preparation.Footnote5
We also perform additional tests utilizing interaction terms of governance traits and natural hazard dummy to gain a better understanding of how different board personalities contribute to the process of addressing climate change problems. Our results show that board characteristics do have influence on bank soundness. Particularly, gender diversification within board members helps banks stabilize better, in which 1% increase in female director helps explain 17% of bank stability improvement. Although board busyness shows a marginal net effect when banks are hit by natural disasters, board networks prove to increase bank stability, consistent with reputation hypothesis. Last but not least, the number of board members show a significant impact on bank stability, sharing the same results with Coles, Daniel, and Naveen (Citation2008) and Pathan and Faff (Citation2013). Therefore, we contribute to corporate governance literature by showing that board characteristics (e.g. gender diversity, board networks, and board size) reinforce banks stability against non-fundamental risk.
Additionally, we contribute to the literature of natural disaster by providing evidence of a U-shaped relationship between weather extreme damage and bank stability. Particularly, the Z-score initially reduces as natural hazards cause increasing damage up to a threshold; but when the total damage exceeds that threshold, the Z-score starts improving. This may be explained by the jointly effect of government aid programmes, such as the Federal Crop Insurance programmes administered by the Risk Management Agency (RMA). However, we also find that indemnity does not contribute to Z-score recovery in the low-damage group whereas the non-linear effect of indemnity on Z-score in the high-damage group is significant. We believe this result may refer to the situation when natural hazards severely damage a region to such a certain threshold that the indemnity is unable to recover the loss caused by hazards.
Our study though closely aligns with the other papers, Klomp (Citation2014), Noth and Schüwer (Citation2018) and Brei, Mohan, and Strobl (Citation2019) provide novel findings surrounding the association between ‘anthropogenic’ (human-induced) factors and weather extremes. Particularly, Klomp (Citation2014) is the first study that explores the relationship of large-scale natural hazards and banking Z-score on the global scale (1997–2010), using explanations such as country financial regulation strength, economic growth, credit to GDP and trade openness to gauge the recovery of commercial bank stability. While Noth and Schüwer (Citation2018) explores the impact of natural disasters on the US banking Z-score in 1994–2012 period, using derived proxies of natural disasters to assert the real effects, the authors neither concern about mediating mechanisms, such as corporate governance, nor emphasize about bank fundamental characteristics. Recently, Brei, Mohan, and Strobl’s (Citation2019) study explores the effect of hurricane on different components of bank balance sheets, through which they reveal the mechanism of how commercial banks from seven Eastern Caribbean countries experienced negative shocks over the period 2001–2012. However, their study does not cover other types of hazards, only concerns about hurricane even though the merit of this study is to build a hurricane index which considers the physical characteristics of hurricanes, and the ex-ante economic exposure to damage. Overall, those three studies cover the time period from mid-90s up to 2010/2012, coinciding with Kyoto Agreement (from first signed time-point (1997) to first commitment in 2012). While Kyoto Agreement calls for reducing greenhouse gas emission compared to pre-1990 levels, much attention has been shifted on multifaceted adverse impacts of climate change after Paris Agreement in 2016. Hence, our study not only adds to the literature by providing evidence for more recent period (i.e. 2010–2019) tallying with the worldwide attention on climate change, but also is different from their studies in several perspectives: (i) this study makes use of the concept of instrumental variable and a two-stage least square technique to explore the relationship of CO2 emission and natural disasters, (ii) we also explore how different bank’s fundamental channels and governance help mitigate the risk of natural disasters, and (iii) this study is one of the first pioneers investigating the non-linear effect of natural disasters on financial stability.
This paper proceeds as follows: Section 2 presents the literature review of our study. Section 3 describes the Data while Methodology and Identification strategy are presented in Section 4. Section 5 reports the empirical results. Section 6 shows further robust tests. Section 7 concludes, and Section 8 provides limitation and future research.
2. Literature review
2.1. A framework about natural disaster and financial stability
The number and cost of climatic disasters are elevating at global scale due to a combination of increasing various constituents, such as exposure (i.e. more assets at risk) and vulnerability (i.e. how much damage a hazard of given intensity – wind speed or flood depth, for example – causes at a location).Footnote6 Natural catastrophes are more likely to cause financial sectors to be in difficult situation if they misjudge risk ex-ante and hold insufficient capital (Batten, Sowerbutts, and Tanaka Citation2016). The diversity of natural hazards also have varying consequences across distinct geographical locations, yet large countries like the US have a larger possibility of encountering a natural catastrophe. Adverse shocks caused by natural disasters may affect the default risk of banks through various ways. On the one hand, catastrophic events can immediately affect banks’ deposits and lending operations, resulting in bank runs, urgent withdrawals to replace lost funds, excessive loan loss write-offs, an increase in non-performing loans, collateral destruction, or office damage inside disaster zones (Faiella and Natoli Citation2018; Barth, Sun, and Zhang Citation2019; Koetter, Noth, and Rehbein Citation2020).
For example, Brei, Mohan, and Strobl (Citation2019) find that the hurricane strike significantly deteriorates the total deposits of the Eastern Caribbean banks by 8%, of which 6% was the decrease in household deposits.Footnote7 Collier and Skees (Citation2012) indicates that there is a drop in the equity ratio immediately following the El Nino happened in Peru. In addition, Brahmana, Puah, and Chai (Citation2016) shows that the Nias Tsunami caused a huge negative impact to the non-performing loans (NPL) of Indonesian banks. In practice, the NPL of Indonesian banks increased dramatically by around 10% after the Nias Tsunami occurred in 2004.
Nevertheless, as per Cortés and Strahan (Citation2017) after the natural disaster happens, bank lending, especially in form of home mortgage, seems moderately increasing in the post natural hazards period. As a result, it is reasonable to expect banks to increase their lending spreads in order to hedge against future climate hazards, such that banks and lenders charge higher interest rates on mortgages and loans in areas prone to sea-level rise and natural disasters (see Correa et al. Citation2020 and Nguyen et al. Citation2020). Additionally, Huynh, Nguyen, and Truong (Citation2020) confirm the significant correlation between the higher cost of equity capital and drought risk. Thus we wonder whether this is sufficient or whether rising spreads would ultimately be detrimental or beneficial in a competitive business-like banking.
On the other hand, the ex-post effect of natural disaster materializes in increasing conflict of interest problem intervening the relationship between banks and customers, such as earnings management in post-disaster period (Cheng et al. Citation2019; Wu et al. Citation2021), spill-over effect onto lending activities in non-disaster areas (Rehbein and Ongena Citation2020; Koetter, Noth, and Rehbein Citation2020), alternation in bank operation (Chavaz Citation2016; Cortés and Strahan Citation2017; Bos et al. Citation2017; Ivanov, Macchiavelli, and Santos Citation2020; Ivanov et al. Citation2020), and biases in lending activities (Garbarino and Guin Citation2021; Kong et al. Citation2021). For instance, the credit demand after hazards is increasing whereas the access to credit is more likely to be limited as bank changes their operations according the record of risks (Berg and Schrader Citation2012; Gallagher and Hartley Citation2017). Consequently, Hosono et al. (Citation2016) find that an adverse natural disaster shock to bank lending capacity reduces client firms’ activity even in an economy with well-developed financial markets and institutions. In contrast, for those banks with multi-market branches, their response is to reallocate funds towards markets with high credit demand and away from other markets (‘connected markets’) in which they lend (Cortés and Strahan Citation2017). Nguyen and Wilson (Citation2020) emphasize the importance of bank existence at the affected regions in alleviating adverse lending effects. Simply said, natural catastrophes not only subject banks to short-term onerous strain on capital adequacy, asset quality, management qualities, and liquidity risk as uncertainty and payback increase, but also may lead to moral hazard and adverse selection in aftermath lending strategies.
The heterogeneous interaction between local economic structure and disaster management programme yields mixed findings, thus may pose difficulty in causally identifying the impact of natural disaster on bank risk. Klomp (Citation2014) suggests that large-scale weather-related disasters, on average, have no significant negative effect on the stability of the banking sector in developed countries, but rather only in emerging countries, indicating heterogeneous effects of extreme weather events in different countries. Noy’s (Citation2009) study also shows that countries with more developed credit markets appear to be more robust and better able to endure natural disasters, while Strömberg (Citation2007) points out that bank lending activities reduce rapidly after a climatic disaster in developing countries.
It is worth noting that the insurance programme plays a critical role in improving resilience to natural hazards or climate-related events by both promoting recovery and providing incentives for investments in hazard mitigation insurance programme (Kousky Citation2019). In particular, it helps households recover from natural disasters (Bertram-Huemmer and Kraehnert Citation2018) and thereby boosting the economy (Sawada and Takasaki Citation2017). Skidmore and Toya (Citation2002) state that damages caused by natural disasters are positively correlated with household saving rates, which suggest that households have attempted to self-insure against some catastrophic events as insurance markets have not provided a sufficient level of protection against possible losses arising from natural catastrophes.
Furthermore, banks are often part of a larger financial conglomerate including an insurance branch. While banks provide insurance segment in its operation, it might incur substantial pay-out after a disaster, which subject banks to short-term liquidity; changes in weather patterns might lead to higher flood risk in the coming decade, and the increasing demand for insurance offers a lucrative market for banks.Footnote8,Footnote9
2.2. Other determinants of bank risk-taking
Regarding banking characteristics, Boyd, Graham, and Hewitt (Citation1993) and De Nicolo (Citation2000) show that large banks’ returns on assets and return volatilities increase in size, suggesting that large banks choose higher risk than their optimal level, and that risk-taking behaviour fully offsets the size-related scale economies and/or diversification benefits. On the contrary, the size of the bank and its natural diversification through widespread franchise to some extent can mitigate adverse shocks like natural disasters. Therefore, this study aims to fill the void of relationship between bank size and impact of climate risks on financial stability.
In addition, prior literature has shown that other factors related to macro-economy, such as GDP, interest rate, or even house price, should be considered to conduct the relationship between natural hazard and financial stability. For instance, both GDP growth and GDP per capita have a positive effect on the financial stability since the banks’ investment opportunities may be correlated with business cycles, which in turn raises the banks’ asset quality (Laeven and Majnoni Citation2003; Uhde and Heimeshoff Citation2009; Bushman and Williams Citation2012; Liang, Moreira, and Lee Citation2020). However, Fouejieu (Citation2017) and IJtsma, Spierdijk, and Shaffer (Citation2017) find the reverse effect of GDP growth on financial stability, which can be explained by the fact that increased risk taking and financial imbalances are often pro-cyclical (Vural-Yavaş Citation2020).
Interest rate as a central tenet of the recent global financial crisis has been studied in the relationship with bank’s risk-taking behaviour. Recent empirical evidence lends support to the notion that low interest rates may help fuel increases in bank leverage and risk-taking (Maddaloni and Peydró Citation2011; Delis and Kouretas Citation2011; DellAriccia, Laeven, and Marquez Citation2014). This softening is amplified by securitization activity, weak supervision for bank capital, and low short-term interest rates for an extended period. In short, the negative relationship between interest rates and risk is more pronounced for banks with more liquid assets and highly capitalized banks (Ioannidou, Ongena, and Peydró Citation2015; Dell’Ariccia, Laeven, and Suarez Citation2017).
According to the so-called financial accelerator mechanism, real estate prices can have two contrasting effects on bank stability, which are the collateral value and the deviation hypotheses (Kiyotaki and Moore Citation1997; Niinimäki Citation2009). Almeida, Campello, and Liu (Citation2006) provide both empirical evidence on international housing markets and underlying mechanism – contractual features of housing finance. The former suggests a positive relation because rising nominal house prices increases the value of collateral pledged, net wealth of borrowers, boost bank capital, and thus reduces the bank’s probability of default (PD), enhancing the financial positions of bank (Niinimäki Citation2009; Killins Citation2020). The latter theory conjectures that persistent deviations from fundamentals may foster the adverse selection of increasingly excessive lending to risky real estate borrowers at unreasonably low rate seeking to expand their loan portfolios, which increases bank distress probabilities (Bernanke and Gertler Citation1995; Allen and Gale Citation2001).
Corporate governance has received an enormous attention in both academia and corporate. As the central theme of corporate governance is to align executive’s interest to those of stakeholders and thereby increase corporate accountability, corporate governance plays a key role in mitigating conflict of interest (Luo Citation2005). Hence, corporate governance is usually linked with bank performance (Denis and McConnell Citation2003; Barth, Sun, and Zhang Citation2003; Adams, Hermalin and Weisbach Citation2010). The existence of female director has been empirically linked to higher bank performance (Low, Roberts, and Whiting Citation2015; Garcia-Meca, Garcia-Sanchez, and Martinez-Ferrero Citation2015). Board size also receives an unsettling impact on bank performance. Barth, Sun, and Zhang (Citation2003) find a positive relationship between board size and bank performance, similar with Pathan and Faff (Citation2013), Coles, Daniel, and Naveen (Citation2008). In line with reputation hypothesis, Ferris, Jagannathan, and Pritchard (Citation2003), and Trinh et al. (Citation2020) document that a busy board will exhibit high bank performance and greater financial stability.Footnote10 Hence, we investigate whether corporate governance assist banks to better recover in the aftermath of natural disasters.
3. Data
3.1. Sample
We exploit the Spatial Hazard Events and Losses Database for the United States (SHELDUS),Footnote11 which is a rich database with several natural events at the county level over time. Recent empirical studies that use this database include Barrot and Sauvagnat (Citation2016), Bernile, Bhagwat, and Rau (Citation2017), Cortés and Strahan (Citation2017) for all data on natural disasters. In particular, SHELDUS compiles data from different sources.Footnote12 Advantages of the SHELDUS database include the annual availability of data, measurement at the county level, and a low threshold of inclusion that covers the impacts of comparably small events (Gall, Borden, and Cutter Citation2009). One limitation of SHELDUS is that assessments of flood damages are difficult to estimate (Gall, Borden, and Cutter Citation2009). We assume any variation in these estimates to be random over time and counties, which is plausible since the probability of extreme weather events occurrence is randomly drawn from a long-term distribution of weather pattern, i.e. climate.
By mainly combining SHELDUS data with financial banking information from S&P COMPUSTAT, we investigate how natural disaster affects the bank stability. Alongside that, we also combine with some other data sources such as DealScan and BoardEx to explore to what extent natural hazards may affect the banking activities. The full detail of the constructed sample can be obtained from Table A1.
3.2. Variables
3.2.1. Banking Z-score
Our main dependent variable of interest is Z-score. Representing the probability of insolvency within banking industry, Z-score receives a wide popularity with a role of measuring risk level in the banking and financial stability-related literature (Uhde and Heimeshoff Citation2009; Strobel Citation2011). The widespread use of z-score is due to its relative simplicity in computation using publicly available accounting data only. It thus can serve as either a complementary index to share market-based approaches or a main risk measure for investors where share prices are not available. In its general form, allowing for non-normal return distributions, it is generally attributed to Hannan and Hanweck (Citation1988) and Boyd, Graham, and Hewitt (Citation1993); Boyd and Graham (Citation1986) had previously introduced Z-scores in the special context of normal return distributions. The basic principle of the Z-score measure is to relate a bank’s capital level to variability of its returns. In doing so, one can identify the magnitude of the return variability absorbed by capital without the bank becoming insolvent. The main consequence of this measure is that a low-risk bank will have a high value of Z-score, which indicates that it requires a significant drop in standard deviations of a bank’s asset return to become insolvent, and vice versa; a lower value of Z-score indicates higher risk of the bank.
In general, Z-score is built using the return on asset ratio (ROA) augmented by the equity-to-asset ratio (EA), all divided by a measure of variability in returns, often the standard deviation of ROA. In a cross-sectional setting, the standard approach to estimate the Z-score for an individual bank is as follows:
(1)
(1) or
(2)
(2) where T = t − 3, .., t. µROAi,T, σROAi,T and µEAi,T are calculated using moving mean and standard deviation estimates with window width of 3 quarters and for each period t ∈ 1, … ,T.
Prior studies contend that Z-score is a noisy measure of financial stability as it can be skewed (Laeven and Levine Citation2009; Houston et al. Citation2010). Hence, motivated by these studies, we use the natural logarithm of Z-score to minimize the impact of skewness. We also employ other measure of bank stability, such as Loan loss provision, Change of equity, and Deposit volatility for robustness purpose. The data on the Z-score and other individual bank characteristics are obtained from the S&P COMPUSTAT Bank covering 2010–2020 period, available from WRDS database. For macroeconomic variables, we download and compile from two sources named as Bureau of Economic Analysis and US Census Bureau.
3.2.2. Banking fundamental characteristics
We look for a mechanism associated with (abnormal) profitability, risk, and growth. Our choice of signals within each category (profitability, prudence, and growth) is motivated by the guidance in the academic and practitioner literature (e.g. Calomiris and Nissim Citation2007; Koller et al. Citation2010).
3.2.2.1. Banking profitability
We emphasize the use of spread (ΔSpread) on a bank’s loan portfolio as the ratio of net interest income (interest income – interest expense) to total loans, higher spread not just only reflects higher risk on the loan portfolio but also implies about the profit margin of the bank.
Loan-to-deposit ratio (ΔLoans_Deposits): This metric assesses a bank’s capacity to effectively use its principal source of capital (deposits) to expand its primary generating asset (loans). If the ratio is very low, it signifies that the bank has a lot of idle cash, which indicates increasing inefficiency. It also indicates the risk of liquidity if a big number of depositors withdraw their funds at the same time.
Operating expense ratio (ΔExpense_Ratio): This determines how much of a bank’s revenue is spent on operational and administrative costs.
Non-interest income (ΔNoninterest_Income): This metric is especially valuable for bigger universal banks whose non-lending/deposit operations account for a major share of their revenue. These revenues are frequently derived from higher-margin value-added services (such as investment banking and brokerage) that are either very lucrative or have no direct expenses (such as service fees).
3.2.2.2. Banking prudence
Loan loss provision – LLP (ΔLLP): In terms of absolute quantity as well as influence on overall profitability and capital adequacy, this is the most critical accrual for banks.
Non-performing loan – NPL (ΔNPL): This is a credit risk statistic that measures the ratio of non-performing loans to total loans in the future. NPLs are noisy, yet they may be one of the most accurate predictors of future loan losses.
Allowance Adequacy (ΔAllowance_Adequacy): Banks having a higher loan loss allowance adequacy are typically better prepared to absorb the predicted credit losses without putting their capital at risk during difficult times.
3.2.2.3. Bank growth
Revenue Growth (SGR): We measure SGR as the percentage annual change in total revenue, not distinguishing whether revenue growth arises from traditional banking activities or other non-banking activities.
Loan Growth (LGR): This is the percentage change in gross loans on the balance sheet over a year. Regulators and market participants routinely assess a bank’s potential to extend its overall loan portfolio. On one side, increasing the loan base might result in more revenue. On the other hand, it might be a sign of a larger credit risk. During times of financial crisis, when banks are unwilling to issue loans due to concerns about the economy’s credit risk, these concerns are amplified.
3.3. Descriptive analysis
The indicator of bank stability (ln(Z-score)) has a mean value of 4.779 and a wide difference between minimum (−4.753) and maximum value (10.427) in Table . Return-on-assets ratio, whose average value stands at 0.2%, also indicates a strong heterogeneity amongst observations within sample data. Turning to independent variable of interest, natural disasters, a mean of 54.9% shows that more than 55 percentage data points in the sample suffer from extreme weather events. The records of injuries and fatalities and total damages indicate the differences in severity and location-wise of distinct catastrophic types.
Table 1. Descriptive statistics.
Both bank characteristic and governance variables again confirm the heterogeneity of observations. The mean value of bank age is 13 years.Footnote13 The similarity is observed with bank size, whose value stands 7.5 million dollars (natural logarithm value). Turning to female ratio, the approximately percentage of female directors sitting on board is 13.3. Lastly, a value of 11 in board size shows that on average, there are 11 members in bank’s board of directors, with the largest amount up to 33 members.
According to EM-DAT Citation2019, we reclassify 18 groups of natural disasters into 4 groups of natural hazards, including (i) hydrological disasters, (ii) meteorological disasters, (iii) geophysical disasters, and (iv) climatic disasters. In particular, hydrological disasters covers floods and wet mass movements; meteorological disasters usually relate to sudden and adverse changes in the weather or weather-forming processes, specifically storms and hurricanes; geophysical disasters entails earthquakes, tsunamis, and volcanic eruption, which rarely happen, but cause a massive losses upon occurrence; and climatic disasters are events caused by extreme temperatures, for example, heat wave, droughts, and wildfires. Table A8 shows the summary of 18 natural disasters in the US from 2000 to 2019. The bottom panel of Table A8 shows the distribution of four types of natural hazards, in which the meteorological disasters are the most common natural disasters (about 82%), while hydrological and climatic disasters take account of around 11% and 6%, respectively. Figures in show the total number of different hazards events in the US from 2000 to 2019 across states. On average, the East of the US experiences higher frequency of hydrological and meteorological disasters than the West of the US. Texas and Iowa are states with the greatest number of climatic disasters, whereas California and New York are states with the highest number of geophysical disasters.
To further identify how corporate governance intervenes the level of bank stability in the event of natural disasters, we obtain and combine with BoardEx sample with regard to board characteristics. This allows us to understand how director and board characteristics affect the bank performance (i.e. bank stability) in the context of natural disaster.
4. Methodology
4.1. Baseline model
We build our model to estimate the relationship between natural disasters and distance-to-default of banks based on the Z-score. We use a dynamic model based on a panel dataset including approximately 907 banks in 50 states between 2010 and 2019. The estimates is given as follows:
(3)
(3) where lnZi,t is the logarithm of distance-to-default of the ith bank at county c at time t based on the Z-score, disasterc,t is a dummy variable whether there is any disaster events happening in county c at quarter t where the ith bank is located. We also include lagged dependent variables to control auto-regressive tendencies and lagged variables of natural disaster because we presume that the bank performance will be affected by some events happened in the past period. Xi,t is a set of bank characteristics, Mc,t is a set of macroeconomic variables at the county level, and µt is a time fixed effect.
The alternative way of writing the previous equation is as follows:
(4)
(4) or
(5)
(5) In this specification, the dependent variable is the log-change of the Z-score. Further, to exploit the exogeneity of the timing and intensity of natural disasters and control for economic developments over time, we include time fixed effects in all regressions, which allows us to control for unobservable time-invariant variables, such as generic disaster risks, disaster management structures, and economic structures in the banks’ business regions.Footnote14
4.2. CO2 emission as instrumental variable in IV-GMM
We tackle the endogenous problem resulting from potential influence of unobserved heterogeneity and past realizations of climate risks as well as CO2 emission on the banking stability by employing IV-GMM technique. We test for the endogeneity of climate risk in the IV-GMM model. We argue that the IV-GMM model presented in this paper is appropriate for estimating the relationship between climate risks and banking stability due to the presence of both unobserved heterogeneity and the influence of past ‘natural’ and ‘anthropogenic’ (human-induced) factors.
Carbon dioxide (CO2) emissions due to fossil fuels account for more than half of greenhouse gas (GHG) emissions, where it is believed that greenhouse gas emissions or CO2 emissions are largely to blame for the several trends in weather extremes in the form of heatwaves, floods, storms, droughts, and rising sea levels. As such, the risk from natural disaster should not be analysed or treated in isolated, but instead should be integrated into the effects of climate change (Van Aalst Citation2006; and Phalkey and Louis Citation2016 for details). For example, the rise in temperature on land surfaces leads to the alteration of the hydrologic cycle (i.e. making it faster), which in turn intensifies cycles of droughts and floods. The rise in temperature at the higher humidity also increases the incidence of tropical storms (Phalkey and Louis Citation2016). Therefore, it is necessary to consider effects of consumption-based carbon emission together with the impact of natural disaster on financial stability in the causal settings.
Financial institutions’ decisions to fund activities with high CO2 emissions can, albeit indirectly, contribute to an increase in climate-related physical risks; on the other hand, their financing of technologies that help reduce CO2 emissions can contribute to a reduction in climate-related physical risks (Batten, Sowerbutts, and Tanaka Citation2016). Numerous efforts have been discussed to identify among which methods to properly apply for controlling GHG emissions. While carbon tax or trade-and-cap framework offers potential positive outcome, the uncertainty in pricing carbon has raised problems in implementation (Davis, Thurber, and Wolak Citation2020). The current literature has also documented connection between financial sectors and carbon emission (Omri et al. Citation2015 Cornille, Rycx, and Tojerow Citation2019). Hence, we use carbon emission as instrument for our model. Also, to strengthen our results by reducing selection bias, we re-analyse our data using propensity score matching technique, as reported in Appendix. This is to ensure that our control and treatment groups are as similar as possible before the event, and that our results are not driven by confounding variables.
5. Empirical results
5.1. Baseline results
In this section, we start by presenting how occurrence of natural disasters impacts banking Z-score. Results obtained by estimating Equation (3) are reported in Table . It is worth noting that we have estimated Equation (3) four times using different combinations of controls. Specifically, we simply run an univariate regression in model 1, presenting our naïve model, model 2 includes year fixed-effects. In models 3 and 4, we include controls for different individual bank characteristics and time fixed effects, subsequently while model 5 controls for cross-sectional macroeconomic condition. We find that negative coefficients of the first lagged variable of natural hazards are significant across the models. This means that the occurrence of natural disasters in the previous quarter has a negative impact on the bank Z-score. The coefficient of −0.072 in column (5) means that the occurrence of natural disasters in the previous quarter, on average, other things being unchanged, reduces the Z-score (i.e. bank stability) by approximately 7%. This result is in line with what we expect. The negative relationship between natural hazard and banking Z-scores persists when adding banking characteristics and macroeconomic condition controls as in columns (3)–(5). Hence, our findings are robust at the either 1% or 0.1% statistical significance level, irrespective of the model specification.
Table 2. How do natural disasters affect banking financial stability? (baseline result).
An additional point worth mentioning is the positively significant coefficients of lagged two quarters of variable of interest (L2.Natural hazard) in all columns (1)–(5). We believe that these coefficients capture the medium-term bank’s recovery ability. Put differently, the coefficients of previous two-quarter period signify the extent to how fast banks can recover after the occurrence of natural disasters. While L.Natural hazard captures the short-term effect of extreme weather events, the positive coefficient of previous two-quarter (L2.Natural hazard) implies that banks which are previously hit by natural disasters have recovered to adapt and stabilize in the aftermath, consistent with Klomp and De Haan (Citation2012). In other words, banks generally manage to overcome adverse effects on their stability and performance within few quarters after a weather-related natural disaster occurred, consistent with findings in previous studies (Brei, Mohan, and Strobl Citation2019; Chen and Chang Citation2021).
Table also indicates that the bank size positively associates with bank Z-score, implying that the bigger the local bank, the more solvent it is. One explanation can be that bigger bank might have more expertise in assessing risks upon loan approval and higher efficiency in terms of operation. Also, larger bank tends to have more branches so that it can reallocate its loss and profit faster than others (Collier, Katchova, and Skees Citation2011). Among macroeconomic controls, GDP growth and interest rate significantly affect banking Z-score as well. Our findings, nevertheless, depart from the traditional expectation of the existing literature supporting the positive effect of GDP growth on financial stability (Laeven and Majnoni Citation2003; Uhde and Heimeshoff Citation2009; Liang, Moreira, and Lee Citation2020). We conjecture that GDP growth might increase risk-taking behaviours of banks, thereby decreasing the finance stability, which is consistent with Fouejieu (Citation2017), IJtsma, Spierdijk, and Shaffer (Citation2017). Our results also suggest a negative relationship between interest rate and Z-score. This implies that the higher the interest rate, the lower the Z-score, which is contradictory with some previous studies, such as Maddaloni and Peydró (Citation2011), Delis and Kouretas (Citation2011), DellAriccia, Laeven, and Marquez (Citation2014). We argue that when interest rate suddenly increases, banks cannot immediately adjust their portfolio accordingly to the new rate, thus reducing the profit margin. As Z-score is estimated based on bank’s ROA, an unexpected thinner margin of profitability results in reduction of bank’s stability.
5.2. IV-GMM results
Human activities are largely to blame for the alarming increase in GHG emissions in the environment in recent decades (Kurz et al. Citation2018), causing dramatic changes in the Earth’s temperature (Ritchie and Roser Citation2020). These drastic changes in temperature divulge in shifts of weather patterns, such as floods, storms, droughts, and rising sea levels (Zhang, Wang, and Wang Citation2018). In particular, carbon dioxide (CO2) emission from fossil fuels accounting for more than half of GHG emissions has been the research subject for numerous studies nowadays.
To incorporate the effects of CO2 emission, we use consumption-based carbon emission reported by each state quarterly as an instrumental variable. As clarified in the literature review, we postulate that the greenhouse gas emission owns a relationship with natural hazard. Therefore, we redesign a model in which CO2 emission and retail natural gas consumption are instrumental variables for natural hazard and report the results in Table . We acknowledge that CO2 might not serve as the best instrument for climate change. One of the reasons is that banks are the major lender of ‘dirty’ industries, which, in turn, increase the level of CO2 emission, accelerating the materialization of climate change which takes the form of natural hazards. Additionally, we also include another instrument, which is the total damage (both crop and property damages, measured in US dollar) caused by natural disasters recorded by SHELDUS.
Table 3. How do natural disasters affect banking financial stability? (IV-GMM results).
As Batten, Sowerbutts, and Tanaka (Citation2016) show that though banks can also finance technology outbreaks increasing/reducing CO2 emission, banks do not necessarily or directly suffer the losses and gains from that in near future. The reporting results (Table )Footnote15 in the first stage show that CO2 emission is positively related with natural disasters. The endogeneity test statistic is significant at least at 1% level (p = 0.0157 (model 1), p = 0.01 (model 2), p = 0.0083 (model 3)) while Hansen test values are between the range 0.05 and 0.7 (models 2 and 3), which means natural hazard must be treated as endogenous and instrumental variables are partly acceptable, proving that our models are valid.
We find that controlling for endogeneity, the results in the second stage also support our baseline conclusions, which show that natural disasters may affect the banking stability (if that bank is not financially healthy) and this effect, in fact, raises banks’ awareness about new risks and develops adaptation plans to that shortly afterward. High capital intensity also means that bank may over-invest, thus lessen the buffer for loss provision and become riskier. Additionally, we conjecture that banks which have a large proportion of fixed assets will bear more damages caused by natural disasters relative to those with high intangible assets. Hence, our result confirms that capital intensity imposes negative relationship on bank stability.
5.3. Which channels climate risk affects banking stability
We argue that climate risks affect banking stability through its impact on several channels, i.e. profitability, prudence, and growth. To validate mechanisms, we examine the interactions between natural hazard and signals of bank financial health towards Z-score. For profitability, we employ loan spread,Footnote16 operating expense ratio, non-interest income, and loans to deposits. For signals related to prudence, we use loan loss provision, non-performing loans, and allowance adequacy. We include revenue growth and loan growth to measure the bank’s growth in its core traditional business and other activities. The choice and measures of mechanism channels follow the previous research (Calomiris and Nissim Citation2007; Koller et al. Citation2010; Mohanram, Saiy, and Vyas Citation2018).
Columns (1)–(4) in Table focus on how natural disasters affect the banking profitability. Starting with the spread on a bank’s loan portfolio, which is defined as the ratio of net interest income (difference between interest income and interest expense) to total loans, larger spread not only reflects the higher risk on loan portfolio, but also implies information regarding the bank’s profit margin. It is obvious that banks will increase the loan spread when being exposed to natural disasters (Huang et al. Citation2020; Correa et al. Citation2020).Footnote17 However, to which extent an increase in spread affects Z-score remains a question. Column (1) shows that the sign of interaction term is negative, and its absolute coefficient (0.076) is higher than that of natural hazard (0.049), implying that increasing the spread when bearing the climate risk is not the ultimate solution because Z-score still declines. The suggestive explanation is that since bank has to keep its spread comparable with its peers, it can only increase the spread up to a threshold but not indefinitely. The consequence is either bank may lose its customers and shrink its loan portfolio or the increasing spread cannot compensate all the natural disaster risks.
Table 4. How natural disasters affect banking Z-score through various channels?.
Supporting the above conjecture are results about interactions (of natural hazard) with each of operating expense ratio, loans to deposits, and non-interest income. The positive and significant coefficient in Column (4) indicates that if operating and administrative expenses are well-managed, it can help mitigate the climate risk. Meanwhile, there are no significant results for interactions (of natural hazard) with either loan to deposits or non-interest income. The insignificance of coefficients indicates that natural disasters do not have a material effect on these two variables. Put differently, non-interest income or lending efficiency cannot compensate for a bank’s stability upon extreme weather hazards.
Towards the prudence channel, we present the results of loan loss provision (LLP), non-performing loan ratio (NPL), and allowance adequacy (AA) in columns (5)–(7) subsequently. The positive interaction coefficients between natural hazard with either LLP or AA, which are both significant at 0.1% and 1%, respectively, strongly emphasize how appropriate the provision/allowance level helps mitigate climate risks without impairing capital during periods of distress. It also implies that if bank is well-diversified and has pertinent expertise to correctly provision for the loss of lending portfolio, Z-score will be enhanced better. On the other hand, banks holding more non-performing loans will be riskier when natural disasters strike. The results are confirmed with the sub-sample analyses in Columns (3) and (4) of Table . The coefficient of interaction term with NPL reported in column (6) is −1.010 and significantly at 5% level.
Table 5. Sub-sample analyses.
We use both revenue growth (SGR)Footnote18 and loan growth (LGR)Footnote19 to investigate the growth channel on banking stability. The interaction term between natural hazard and growth of bank loan portfolio (LGR) in column (9) is positive at 5% significance level, illustrating that bank can cope with extreme weather conditions by expanding and diversifying its loan portfolio. Nevertheless, negative result in column (8) representing the interaction term between natural hazard and total revenue growth still holds that revenue growth does not compensate bank’s stability in the unpredictable climate environment.
6. Additional tests
6.1. Sub-sample analyses
Table reports sub-sample results. First, we classify states into either less- or more-exposed group based on the frequency of natural hazard occurrence.Footnote20 We then categorize bank by its geographical locations in the above two groups and discover that the effect of extreme weather events is more pronounced in the less-exposed group. Consistent with the literature,Footnote21 banks that are not familiar with handling the aftermath of natural disasters are less prepared to cope with climate risk; while the more-exposed group is more likely to have good prediction and preparation. For instance, banks operating in wildfire-prone areas, such as California or Texas, will accumulate more experience in assessing the likelihood of destruction caused by potential fire, and require additional measure to mitigate before providing mortgage to households. Hence, these banks will have more expertise in preparing or handling the occurrence of natural disaster, compared to others.
Another way to split the sample is to base on its non-performing loan using the sample’s median as threshold (see columns (3)–(4)). We find that banks which hold higher portion of bad debts will suffer more severely, worsening natural disaster’s impacts. Intuitively, banks with high portion of bad debts in their portfolio are less sound and, consequently, subject to fragility when extreme climatic events occur (aforementioned in previous part).
Upon splitting sample by using ROE median value as threshold, banks with higher ROE need to take better care of climate risk comparing to those in a lower-ROE group. In this case, the higher magnitude (and stronger regarding significance level) of negative relationship between natural disaster and bank stability across two sub-samples (see columns (5)–(6), 0.099 > 0.031) illustrates our finding. Higher ROE may be attributable to many causes, among which are lower provision or riskier asset allocation leading thinner protection. Researchers and practitioners show that ROE actually encourages greater risk-taking by banks. These beliefs affect banks’ choices in terms of asset allocation and financial policy, distorting their strategic planning (Moussu and Petit-Romec Citation2014).
6.2. How board governance help mitigate climate risk?
To capture how the governance responses with the natural disaster, we also consider the below estimate:
(6)
(6) In addition, by combining with BoardEx dataset, we try to identify which kind of board characteristics better mitigate climate risks. Table illustrates the association of the board characteristics and bank stability in the context of natural disaster. We find that the coefficient of interaction term between natural disaster and female ratio is statistically significant in column (1). This means that given the natural disaster happens, a 1% increase in proportion of female directors on the board the Z-score increases by around 27%. This result is in line with previous studies (e.g. Abbott, Parker, and Presley Citation2012; Green and Homroy Citation2018) and once again confirms that one of primary female characteristics is risk-averse. Column (2) reports a positive coefficient (though not significant) of the interaction term regarding the variance in ages of the board, but a negative coefficient of itself.
Table 6. Which kind of board mitigate better climate risks?
Regarding the busyness of the board, we find the significance of its coefficient but no significance of the interaction term (shown in column (3)). This means that the bank stability is generally deteriorated with the busy board regardless of natural disasters (Core, Holthausen, and Larcker Citation1999; Kress Citation2018). According to reputation hypothesis, board networks also contribute to a better governance. In the last column, we account for average network size of the board and find the negative coefficient for itself and positive coefficient for the interaction term. However, these effects almost cancel out to leave the net effect of network size relatively marginal. Last but not least, the number of directors on the board is another factor contributing to banks’ corporate governance. The significantly positive coefficient in column (4) means that when there are more directors on boards, the banking financial health may be more stable in the event of natural disaster. This may be plausible when there is more bank management among the board members to get the bank over the natural disasters.
6.3. Testing on non-linear relationship
6.3.1. Does total damages effect follow a non-linear nexus?
This section provides additional test on non-linear relationship between natural disasters and banking stability, which have not been addressed well in previous studies. Rather using dummy variable to proxy for climate risk, we use total damage caused by natural disasters,Footnote22 and report the results of which are shown in Panel A of Table . The results suggest a U-shaped relationship between hazard damage and bank Z-score. The non-linearity relation implies that the Z-score initially reduces as natural hazards cause increasing damage, but starts improving when the total damage exceeds threshold. This sounds sensible because there is some indemnity has been paid out for the designated peril loss due to hazard events under the Federal crop insurance programmes administered by the Risk Management Agency (RMA).
Table 7. Non-linear effect of natural disaster on banking stability.
Alternatively, we divide the total damage into different bins to capture the non-linear effect of natural hazards on the banking stability. Panel B of Table shows how different bins of total damage affect the Z-score. We find the negative statistical significance of bin 3 and bin 4 relative to bin 1, but the absolute coefficient value of bin 3 greater than that of bin 4 implies a U-shaped relationship, which is consistent with the findings in Panel A.
6.3.2. Different levels of destruction: the role of indemnity
Furthermore, we also perform another test to see the effect of indemnity paid out due to natural hazards, which is illustrated in Table . First, we divide our sample into the high- and low-damaged ones using median value as threshold. Panel A of Table depicts the differences in Z-score between these two groups. Panel B of Table presents the regression results of the two groups. As we expect, indemnity does not help to recover Z-score in the low-damaged group, whereas we find significant result of indemnity on Z-score in the high-damaged group. The results suggest an inverse-U shaped relationship between indemnity and Z-score. This means that the Z-score improves as the paid-out indemnity keeps increasing. However, up to a certain level, the Z-score deteriorates as the indemnity paid-out keeps increasing. This refers to the situation when natural hazards hit us hard, and the indemnity cannot recover the loss caused by hazards. This result is similar to the findings of Linnerooth-Bayer and Mechler (Citation2006) which suggests that insurance would be an assisting adaptation to climate change in developing countries.
Table 8. Non-linear effect of indemnity on banking stability.
7. Conclusion
In this study, we provide results showing that the growing trend in the frequency and intensity of severe natural events due to climate change has potentiality to stress and threaten banks to the point of impaired viability or even insolvency. To date, the empirical evidence on the mentioned relationship is mixed due to the fact that realized disaster-related damages are affected by local economic structure and disaster management under local economic conditions. To do so, we use Spatial Hazard Events and Losses Database for the United States (SHELDUS) and banking financial data from S&P COMPUSTAT alongside several macroeconomic, market structure, and bank-related variables from Bureau of Economic Analysis (BEA) for the period 2010–2019.
Controlling for bank characteristics, macroeconomic factors, and time fixed effects, we show that natural disasters negatively influence the financial stability. Alternatively, we insist that the relationship between natural hazards and financial stability in fact should be considered under the association with CO2 (in the first stage of IV-GMM) to provide a revealing relationship. Addressing potential endogeneity issue with 2SLS, we show that our findings have significant economic interpretations. Specifically, the occurrence of natural disasters in the previous quarter, on average, ceteris paribus, reduces the Z-score (proxied for financial stability) by around 7%. This result holds true, especially for local medium-sized banks but not for big banks.
Through examining the mechanism by which natural disasters increase bank risk, we demonstrate that increasing the interest rate spread does not adequately compensate for the risk of natural disasters, but rather results in future declines in lending activity. Sub-sample analyses demonstrate that inexperienced banks in dealing with natural disasters are less able to cope with climate risk.
Further, we find suggestive evidence that strong corporate governance helps stabilize banks in the aftermath of disasters. Particularly, our results find that banks with more female directors and having large networks tend to recover better than their counterparts. Hence, board characteristics do play a role in determining the level of bank risk.
Last but not least, we find a non-linear relationship between weather extreme damage and bank stability, which may be resulted in the joint effect of government aid programmes. However, no matter how much indemnity is paid out, the loss of natural hazard barely recovers when natural hazards severely hit a region up to a certain limit.
Our results call for more attention from banks’ management team to increase efforts in preparing and adapting strategies, given the fact that global warming is occurring, potentially leading to growing numbers and intensity of extreme weather events. While natural hazards adversely affect the bank stability, banks can better adapt to increasing climate risk by adjusting operational activities to influence the level of loss provision or allowance adequacy. Additionally, strong corporate governance assists banks to recovery better than their cohort. Hence, our results also speak to policymakers to have prompt regulations, such as compulsory diversified board members, or capital adequacy in regions that are suffered from natural disasters at high frequency, with the ultimate aim of increasing bank’s health, ensuring normal function of financial institution when adverse climatic events strike.
8. Limitation and remark
Despite our efforts of trying to provide a comprehensive picture of how natural hazards affect bank stability, we derive our results by focusing on only bank’s headquarters, neglecting the effect of natural hazards on branches of the concerned banks in different regions. Recent findings by Correa et al. (Citation2020) prove a spillover effect of natural disasters on banking sectors. Hence, a closer investigation on network of bank establishments (i.e. branches, partnerships, or even competitors) or spatial effect (of natural hazard) might reveal a stronger outcome.
Second, the materialization of climate change might reveal beyond natural hazards and probably have second-order effect on banking sectors. According to Graff Zivin and Neidell (Citation2014), excessive temperatures reduce employee working hours, thus affecting firm’s – banks’ customers – bottom line. Third, banks might adopt certain mechanisms to better adapt to the increasing risk from climate change. Such adaptive strategies might provide certain banks competitive advantage relative to others, highlighting heterogeneity effect of future hazards events. Next, regulatory risk might become tightened in the future, which expose either banks or their customers, i.e. oil and gas companies, to higher costs of doing business. Lastly, insurance programme may be designed better to cope with severe risks like natural catastrophes. All together might lead to increasing bank stability.
Acknowledgments
We thank Dr. Panayiotis Papakyriakou and Dr. Laima Spokeviciute for discussing our paper. We thank Prof. Arman Eshraghi, Prof. Konstantinos Stathopoulos, and Dr. Svetlana Mira for constructive feedback at Cardiff Corporate Governance Research Group – The 2nd International Conference. We also appreciate the comments about the non-linear effect of natural hazards from anonymous scholars that we have included in this version. Last but not least, we owe many thanks to Professor Dimitrios Gounopoulos from the University of Bath who provided feedback for writing.
Disclosure statement
No potential conflict of interest was reported by the author(s).
Notes
2 See Statista, Countries with the most natural disasters in 2020, https://www.statista.com/statistics/269652/countries-with-the-most-natural-disasters/
3 The SHELDUS database is provided by Center for Emergency Management and Homeland Security at the Arizona State University Carolina https://cemhs.asu.edu/sheldus). Figure summarizes various types of natural disasters in the US from 2000 to 2019.
4 Together with the 2SLS analysis, the propensity score match constitutes one of the sharpest tests to isolate the effect of natural disasters on bank risk as it compares banks that are very similar to each other taking into account their propensity to be exposed with natural disasters. In Appendix, we provide the results of propensity score matching using a variety of factors such as bank characteristics, macroeconomic circumstances, CO2 emissions, and governance.
5 Extra findings (in Appendix) suggest that the negative influence of climate risks on financial stability is strongest for local medium-sized banks. On the other hand, we also provide solid evidence showing that local economic condition does invert the impact of natural hazard on financial stability, which also holds true in case of big banks due to their widespread franchise and better diversification.
7 As a result, two quarters after a hurricane, bank lending has been contracted. Similar findings have been found in studies on the impact of natural disasters, indicating that researchers should consider one- and/or two-period lag terms. As a result, when a natural hazard occurs, the effects on the financial system should include both the current and time-period lag terms Cortés (Citation2014) and Chen and Chang (Citation2021).
9 We do not address this problem of private sector insurance in this study as only federal insurance data is available.
10 By leveraging expertise and network of outside directors to make better decision, utilize more efficiently resources and monitor more effectively
11 The SHELDUS database is provided by Center for Emergency Management and Homeland Security at the Arizona State University Carolina https://cemhs.asu.edu/sheldus)
12 The National Climatic Data Center, the National Geophysical Data Center, and the Storm Prediction Center on fatalities and direct losses in the form of property damages associated with 18 types of natural hazards (e.g. hurricanes, floods, severe storms, tornados, and wildfires, etc.).
13 13 = e5.3
14 Also, we are able to estimate the parameter γ by a propensity score matching methodology, where banks in county c affected by natural disasters are the "treated", otherwise they are the "controls" (see the results Table A5).
15 We also report another version of IV-GMM without lag of natural disasters in Table A6.
16 This measure is different with Spread_DealScan variable, reported in Table A2.
17 The results from alternative measures of banking stability confirm on this, see Table A2, with Spread_DealScan.
18 Both traditional banking activities and other non-banking activities are included for the revenue calculation.
19 The percentage annual change in gross loans reported on the balance sheet.
21 See, e.g. Chavaz (Citation2016), Noth and Schüwer (Citation2018), Skidmore and Toya (Citation2002).
22 Total damage is the sum of property damage and crop damage, reported in US dollars.
References
- Abbott, L. J., S. Parker, and T. J. Presley. 2012. “Female Board Presence and the Likelihood of Financial Restatement.” Accounting Horizons 26 (4): 607–629.
- Adams, Hermalin, B. E., and M. S. Weisbach. 2010. “The Role of Boards of Directors in Corporate Governance: A Conceptual Framework and Survey.” Journal of Economic Literature 48 (1): 58–107.
- Allen, F., and D. Gale. 2001. Comparing Financial Systems. Cambridge, MA: MIT Press.
- Almeida, H., M. Campello, and C. Liu. 2006. “The Financial Accelerator: Evidence from International Housing Markets.” Review of Finance 10 (3): 321–352.
- Baldauf, M., L. Garlappi, and C. Yannelis. 2020. “Does Climate Change Affect Real Estate Prices? Only if you Believe in it.” The Review of Financial Studies 33 (3): 1256–1295.
- Barrot, J.-N., and J. Sauvagnat. 2016. “Input Specificity and the Propagation of Idiosyncratic Shocks in Production Networks.” The Quarterly Journal of Economics 131 (3): 1543–1592.
- Barth, J. R., Y. Sun, and S. Zhang. 2003. Is Corporate Governance Different for Bank Holding Companies? SSRN 387561.
- Barth, J. R., Y. Sun, and S. Zhang. 2019. Banks and Natural Disasters. SSRN 3438326.
- Batten, S., R. Sowerbutts, and M. Tanaka. 2016. Let’s Talk About the Weather: The Impact of Climate Change on Central Banks. SSRN 2783753.
- Berg, G., and J. Schrader. 2012. “Access to Credit, Natural Disasters, and Relationship Lending.” Journal of Financial Intermediation 21 (4): 549–568.
- Bernanke, B. S., and M. Gertler. 1995. “Inside the Black Box: The Credit Channel of Monetary Policy Transmission.” Journal of Economic Perspectives 9 (4): 27–48.
- Bernile, G., V. Bhagwat, and P. R. Rau. 2017. “What Doesn’t Kill you Will Only Make You More Risk-Loving: Early-Life Disasters and CEO Behavior.” The Journal of Finance 72 (1): 167–206.
- Bernstein, A., M. T. Gustafson, and R. Lewis. 2019. “Disaster on the Horizon: The Price Effect of Sea Level Rise.” Journal of Financial Economics 134 (2): 253–272.
- Bertram-Huemmer, V., and K. Kraehnert. 2018. “Does Index Insurance Help Households Recover from Disaster? Evidence from IBLI Mongolia.” American Journal of Agricultural Economics 100 (1): 145–171.
- Bos, J., and R. Li. 2017. “Understanding the Trembles of Nature: How do Disaster Experiences Shape Bank Risk Taking?” Maastricht University, Graduate School of Business and Economics (GSBE).
- Boyd, J. H., and S. L. Graham. 1986. “Risk, Regulation, and Bank Holding Company Expansion Into Nonbanking.” Quarterly Review 10 (Spr): 2–17.
- Boyd, J. H., S. L. Graham, and R. S. Hewitt. 1993. “Bank Holding Company Mergers with Nonbank Financial Firms: Effects on the Risk of Failure.” Journal of Banking & Finance 17 (1): 43–63.
- Brahmana, R., C. Puah, and M. Chai. 2016. “Natural Disaster and Local Bank Non-Performing Loan: Case of Nias Tsunami 2004.” Economics Bulletin 36 (4): 2413–2421.
- Brei, M., P. Mohan, and E. Strobl. 2019. “The Impact of Natural Disasters on the Banking Sector: Evidence from Hurricane Strikes in the Caribbean.” The Quarterly Review of Economics and Finance 72: 232–239.
- Breitenstein, M., D. K. Nguyen, and T. Walther. 2021. “Environmental Hazards and Risk Management in the Financial Sector: A Systematic Literature Review.” Journal of Economic Surveys 35 (2): 512–538.
- Bushman, R. M., and C. D. Williams. 2012. “Accounting Discretion, Loan Loss Provisioning, and Discipline of Banks’ Risk-Taking.” Journal of Accounting and Economics 54 (1): 1–18.
- Calomiris, C. W., and D. Nissim. 2007. “Activity-Based Valuation of Bank Holding Companies.” National Bureau of Economic Research. Working Paper. http://www.nber.org/papers/w12918.
- Cetorelli, N., and L. S. Goldberg. 2011. “Global Banks and International Shock Transmission: Evidence from the Crisis.” IMF Economic Review 59 (1): 41–76.
- Chavaz, M. 2016. Dis-Integrating Credit Markets: Diversification, Securitization, and Lending in a Recovery. SSRN 2843683.
- Chen, X., and C.-P. Chang. 2021. “The Shocks of Natural Hazards on Financial Systems.” Natural Hazards 105 (3): 2327–2359.
- Cheng, Y., J. Park, S. Pierce, and T. Zhang. 2019. Big Bath Accounting Following Natural Disasters. SSRN 3305478.
- Coles, J. L., N. D. Daniel, and L. Naveen. 2008. “Boards: Does One Size Fit All?” Journal of Financial Economics 87 (2): 329–356.
- Collier, B., A. L. Katchova, and J. R. Skees. 2011. “Loan Portfolio Performance and el niño, an Intervention Analysis.” Agricultural Finance Review 71 (1): 98–119. doi:10.1108/00021461111128183.
- Collier, B., and J. Skees. 2012. “Increasing the Resilience of Financial Intermediaries Through Portfolio- Level Insurance Against Natural Disasters.” Natural Hazards 64 (1): 55–72.
- Core, J. E., R. W. Holthausen, and D. F. Larcker. 1999. “Corporate Governance, Chief Executive Officer Compensation, and Firm Performance.” Journal of Financial Economics 51 (3): 371–406.
- Cornille, D., F. Rycx, and I. Tojerow. 2019. “Heterogeneous Effects of Credit Constraints on Smes’ Employment: Evidence from the European Sovereign Debt Crisis.” Journal of Financial Stability 41: 1–13.
- Correa, R., A. He, C. Herpfer, and U. Lel. 2020. The Rising Tide Lifts Some Interest Rates: Climate Change, Natural Disasters and Loan Pricing. SSRN: 3710451.
- Cortés, K. R. 2014. Rebuilding After Disaster Strikes: How Local Lenders Aid in the Recovery.
- Cortés, K. R., and P. E. Strahan. 2017. “Tracing out Capital Flows: How Financially Integrated Banks Respond to Natural Disasters.” Journal of Financial Economics 125 (1): 182–199.
- Davis, T. L., M. C. Thurber, and F. A. Wolak. 2020. An Experimental Comparison of Carbon Pricing Under Uncertainty in Electricity Markets. Tech. Rep. National Bureau of Economic Research. http://www.nber.org/papers/w27260.
- Delis, M. D., and G. P. Kouretas. 2011. “Interest Rates and Bank Risk-Taking.” Journal of Banking & Finance 35 (4): 840–855.
- DellAriccia, G., L. Laeven, and R. Marquez. 2014. “Real Interest Rates, Leverage, and Bank Risk-Taking.” Journal of Economic Theory 149: 65–99.
- Dell’Ariccia, G., L. Laeven, and G. A. Suarez. 2017. “Bank Leverage and Monetary Policy’s Risk-Taking Channel: Evidence from the United States.” The Journal of Finance 72 (2): 613–654.
- De Nicolo, G. 2000. “Size, Charter Value and Risk in Banking: An International Perspective.” International Finance Discussion Papers. Board of Governors of the Federal Reserve System (US), No 689, Accessed (13 February 2013). https://econpapers.repec.org/paper/fipfedgif/689.htm.
- Denis, D. K., and J. J. McConnell. 2003. “International Corporate Governance.” Journal of Financial and Quantitative Analysis 38 (1): 19–41.
- EM-DAT. 2019. OFDA/CRED International Disaster Database [Accessed 24.06.2021].
- Faiella, I., and F. Natoli. 2018. Natural Catastrophes and Bank Lending: The Case of Flood Risk in Italy. Bank of Italy Occasional Paper (457). SSRN 3429210.
- Ferris, S. P., M. Jagannathan, and A. C. Pritchard. 2003. “Too Busy to Mind the Business? Monitoring by Directors with Multiple Board Appointments.” The Journal of Finance 58 (3): 1087–1111.
- Fouejieu, A. 2017. “Inflation Targeting and Financial Stability in Emerging Markets.” Economic Modelling 60: 51–70.
- Gall, M., K. A. Borden, and S. L. Cutter. 2009. “When do Losses Count? six Fallacies of Natural Hazards Loss Data.” Bulletin of the American Meteorological Society 90 (6): 799–810.
- Gallagher, J., and D. Hartley. 2017. “Household Finance After a Natural Disaster: The Case of Hurricane Katrina.” American Economic Journal: Economic Policy 9 (3): 199–228.
- Garbarino, N., and B. Guin. 2021. “High Water, No Marks? Biased Lending After Extreme Weather.” Journal of Financial Stability 54: 100874.
- Garcia-Meca, E., I.-M. Garcia-Sanchez, and J. Martinez-Ferrero. 2015. “Board Diversity and Its Effects on Bank Performance: An International Analysis.” Journal of Banking and Finance 53: 202–214.
- Gassebner, M., A. Keck, and R. Teh. 2010. “Shaken, Not Stirred: The Impact of Disasters on International Trade.” Review of International Economics 18 (2): 351–368.
- Graff Zivin, J., and M. Neidell. 2014. “Temperature and the Allocation of Time: Implications for Climate Change.” Journal of Labor Economics 32 (1): 1–26.
- Green, C. P., and S. Homroy. 2018. “Female Directors, Board Committees and Firm Performance.” European Economic Review 102: 19–38.
- Hannan, T. H., and G. A. Hanweck. 1988. “Bank Insolvency Risk and the Market for Large Certificates of Deposit.” Journal of Money, Credit and Banking 20 (2): 203–211.
- Hong, H., G. A. Karolyi, and J. A. Scheinkman. 2020. “Climate Finance.” The Review of Financial Studies 33 (3): 1011–1023.
- Hong, H., F. W. Li, and J. Xu. 2019. “Climate Risks and Market Efficiency.” Journal of Econometrics 208 (1): 265–281.
- Hosono, K., D. Miyakawa, T. Uchino, M. Hazama, A. Ono, H. Uchida, and I. Uesugi. 2016. “Natural Disasters, Damage to Banks, and Firm Investment.” International Economic Review 57 (4): 1335–1370.
- Houston, J. F., C. Lin, P. Lin, and Y. Ma. 2010. “Creditor Rights, Information Sharing, and Bank Risk Taking.” Journal of Financial Economics 96 (3): 485–512.
- Huang, Q., F. Jiang, Y. Xuan, and T. Yuan. 2020. Do Banks Overreact to Disaster Risk? SSRN 3871505.
- Huynh, T. D., T. H. Nguyen, and C. Truong. 2020. “Climate Risk: The Price of Drought.” Journal of Corporate Finance 65: 101750.
- IJtsma, P., L. Spierdijk, and S. Shaffer. 2017. “The Concentration–Stability Controversy in Banking: New Evidence from the EU-25.” Journal of Financial Stability 33: 273–284.
- Iliev, P., and L. Roth. 2021. Do Directors Drive Corporate Sustainability? SSRN 3575501.
- Ioannidou, V., S. Ongena, and J.-L. Peydró. 2015. “Monetary Policy, Risk-Taking, and Pricing: Evidence from a Quasi-Natural Experiment.” Review of Finance 19 (1): 95–144.
- Ivanov, I., M. Macchiavelli, and J. A. Santos. 2020. Bank Lending Networks and the Propagation of Natural Disasters. SSRN 3541335.
- Ivanov, I., M. Macchiavelli, and J. A. Santos. 2020. The Impact of Natural Disasters on the Corporate Loan Market (tech. rep.). Federal Reserve Bank of New York.
- Killins, R. N. 2020. “Real Estate Prices and Banking Performance: Evidence from Canada.” Journal of Economics and Finance 44 (1): 78–98.
- Kiyotaki, N., and J. Moore. 1997. “Credit Cycles.” Journal of Political Economy 105 (2): 211–248.
- Klomp, J. 2014. “Financial Fragility and Natural Disasters: An Empirical Analysis.” Journal of Financial Stability 13: 180–192.
- Klomp, J., and J. De Haan. 2012. “Banking Risk and Regulation: Does One Size Fit All?” Journal of Banking & Finance 36 (12): 3197–3212.
- Koetter, M., F. Noth, and O. Rehbein. 2020. “Borrowers Under Water! Rare Disasters, Regional Banks, and Recovery Lending.” Journal of Financial Intermediation 43: 100811.
- Koller, T., M. Goedhart, and D. Wessels. 2010. Valuation: Measuring and Managing the Value of Companies. Vol. 499. Hoboken, NJ: John Wiley & Sons.
- Kong, D., Z. Lin, Y. Wang, and J. Xiang. 2021. “Natural Disasters and Analysts’ Earnings Forecasts.” Journal of Corporate Finance 66: 101860.
- Kousky, C. 2019. “The Role of Natural Disaster Insurance in Recovery and Risk Reduction.” Annual Review of Resource Economics 11: 399–418.
- Kress, J. C. 2018. “Board to Death: How Busy Directors Could Cause the Next Financial Crisis.” BCL Review. 59: 877.
- Kurz, W., S. Hayne, M. Fellows, J. MacDonald, J. Metsaranta, M. Hafer, and D. Blain. 2018. “Quantifying the Impacts of Human Activities on Reported Greenhouse gas Emissions and Removals in Canada’s Managed Forest: Conceptual Framework and Implementation.” Canadian Journal of Forest Research 48 (10): 1227–1240.
- Laeven, L., and R. Levine. 2009. “Bank Governance, Regulation and Risk Taking.” Journal of Financial Economics 93 (2): 259–275.
- Laeven, L., and G. Majnoni. 2003. “Loan Loss Provisioning and Economic Slowdowns: Too Much, Too Late?” Journal of Financial Intermediation 12 (2): 178–197.
- Liang, S., F. Moreira, and J. Lee. 2020. “Diversification and Bank Stability.” Economics Letters 193: 109312.
- Linnerooth-Bayer, J., and R. Mechler. 2006. “Insurance for Assisting Adaptation to Climate Change in Developing Countries: A Proposed Strategy.” Climate Policy 6 (6): 621–636.
- Low, D. C., H. Roberts, and R. H. Whiting. 2015. “Board Gender Diversity and Firm Performance: Empirical Evidence from Hong Kong, South Korea, Malaysia and Singapore.” Pacific-Basin Finance Journal 35: 381–401.
- Luo, Y. 2005. “How Does Globalization Affect Corporate Governance and Accountability? A Perspective from MNEs.” Journal of International Management 11 (1): 19–41.
- Maddaloni, A., and J.-L. Peydró. 2011. “Bank Risk-Taking, Securitization, Supervision, and Low Interest Rates: Evidence from the Euro-Area and the us Lending Standards.” The Review of Financial Studies 24 (6): 2121–2165.
- Mohanram, P., S. Saiy, and D. Vyas. 2018. “Fundamental Analysis of Banks: The Use of Financial Statement Information to Screen Winners from Losers.” Review of Accounting Studies 23 (1): 200–233.
- Moussu, C., and A. Petit-Romec. 2014. Roe in Banks: Myth and Reality. SSRN 2374068.
- Murfin, J., and M. Spiegel. 2020. “Is the Risk of sea Level Rise Capitalized in Residential Real Estate?” The Review of Financial Studies 33 (3): 1217–1255.
- Nguyen, D. D., S. Ongena, S. Qi, and V. Sila. 2020. “Climate Change Risk and the Costs of Mortgage Credit.” Swiss Finance Institute Research Paper, (20-97).
- Nguyen, L., and J. O. Wilson. 2020. “How Does Credit Supply React to a Natural Disaster? Evidence from the Indian Ocean Tsunami.” The European Journal of Finance 26 (7–8): 802–819.
- Niinimäki, J.-P. 2009. “Does Collateral Fuel Moral Hazard in Banking?” Journal of Banking & Finance 33 (3): 514–521.
- Noth, F., and U. Schüwer. 2018. Natural Disaster and Bank Stability: Evidence from the U.S Financial System. SSRN 2921000.
- Noy, I. 2009. “The Macroeconomic Consequences of Disasters.” Journal of Development Economics 88 (2): 221–231.
- Omri, A., S. Daly, C. Rault, and A. Chaibi. 2015. “Financial Development, Environmental Quality, Trade and Economic Growth: What Causes What in MENA Countries.” Energy Economics 48: 242–252.
- Pathan, S., and R. Faff. 2013. “Does Board Structure in Banks Really Affect Their Performance?” Journal of Banking Finance 37 (5): 1573–1589.
- Phalkey, R., and V. Louis. 2016. “Two Hot to Handle: How Do We Manage the Simultaneous Impacts of Climate Change and Natural Disasters on Human Health?” The European Physical Journal Special Topics 225 (3): 443–457.
- Rehbein, O., and S. Ongena. 2020. “Flooded Through the Back Door: The Role of Bank Capital in Local Shock Spillovers.” Swiss Finance Institute Research Paper (20-07).
- Ritchie, H., and M. Roser. 2020. CO and Greenhouse Gas Emissions. Our World in Data.
- Sawada, Y., and Y. Takasaki. 2017. “Natural Disaster, Poverty, and Development: An Introduction.” World Development 94: 2–15.
- Skidmore, M., and H. Toya. 2002. “Do Natural Disasters Promote Long-run Growth?” Economic Inquiry 40 (4): 664–687.
- Strobel, F. 2011. “Bank Insolvency Risk and Different Approaches to Aggregate Z-Score Measures: A Note.” Applied Economics Letters 18 (16): 1541–1543.
- Strömberg, D. 2007. “Natural Disasters, Economic Development, and Humanitarian Aid.” Journal of Economic Perspectives 21 (3): 199–222.
- Trinh, V. Q., M. Elnahass, A. Salama, and M. Izzeldin. 2020. “Board Busyness, Performance and Financial Stability: Does Bank Type Matter?” The European Journal of Finance 26 (7–8): 774–801.
- Uhde, A., and U. Heimeshoff. 2009. “Consolidation in Banking and Financial Stability in Europe: Empirical Evidence.” Journal of Banking & Finance 33 (7): 1299–1311.
- Van Aalst, M. K. 2006. “The Impacts of Climate Change on the Risk of Natural Disasters.” Disasters 30 (1): 5–18.
- Vural-Yavaş, Ç. 2020. “Corporate Risk-Taking in Developed Countries: The Influence of Economic Policy Uncertainty and Macroeconomic Conditions.” Journal of Multinational Financial Management 54: 100616.
- Wu, K., H. Zhang, S. Wang, Y. Qiu, and M. S. Seasholes. 2021. How Do Firms Manage Earnings Following Natural Disasters? SSRN 3676848.
- Zhang, B., Z. Wang, and B. Wang. 2018. “Energy Production, Economic Growth and CO2 Emission: Evidence from Pakistan.” Natural Hazards 90 (1): 27–50.