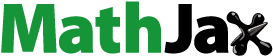
Abstract
This article analyzes two longitudinal datasets (October – December 2020; April 2021) of 1,000 and 900 women in Kenya and Nigeria, respectively, alongside in-depth qualitative interviews with women at risk of changes to time use, to study two pandemic issues: women’s substitution of paid for unpaid work and how these shifts compromise their mental health. Women devote more time to domestic care (30–38 percent), less time to employment (29–46 percent), and become unemployed (12–17 percent). A rise in domestic work is correlated with depressive (Nigeria) and anxiety symptoms (Kenya and Nigeria). Women with greater agency (Kenya) and fewer children (Nigeria) are less likely to report a domestic burden or loss in paid activities. Social protection programs may fill the void of assistance traditionally provided by informal networks in the short term, while campaigns shifting norms around household work may preserve women’s economic participation in the long term.
HIGHLIGHTS
Women in Kenya and Nigeria reported increases in domestic labor amid the pandemic.
Women’s agency is negatively associated with the domestic burden and a reduction in paid activities in Kenya.
Women in households with two or more children face greater domestic burdens and losses in paid activities in Nigeria.
Increases in domestic work render women more likely to be anxious (Kenya and Nigeria) and depressed (Nigeria).
INTRODUCTION
An emerging body of literature illustrates how the pandemic has disproportionately affected the time women spend on unpaid work relative to men, particularly within the home (Del Boca et al. Citation2020; Agarwal Citation2021; Desai, Deshmukh, and Pramanik Citation2021; Seck et al. Citation2021; Zamarro and Prados Citation2021). The increased demand for domestic work prompted by COVID-19 lockdown policies has coincided with more women exiting the labor market (Desai, Deshmukh, and Pramanik Citation2021; Seck et al. Citation2021) and greater declines in mental health (Xue, McMunn, and Tran Citation2021; Zamarro and Prados Citation2021) compared to men. It is well-established that cyclical economic shocks that temporarily displace workers from the labor market can generate a suite of long-term effects on their future livelihoods, socialization, and well-being (von Wachter Citation2020). Furthermore, a widening in the gender gap in labor market participation interferes with long-term development goals (Coulibaly Citation2020). Yet, relatively little has been documented on the time use and labor market effects of the pandemic on women in Africa to identify solutions for smoothing the transition and sustaining economic growth.
We collected two longitudinal datasets (October – December 2020; April 2021) of 1,000 and 900 women in Kenya and Nigeria alongside in-depth qualitative interviews with women at risk of changes to time use, respectively. Both countries are characterized as lower-middle income and represent significant shares of the Sub-Saharan Africa population (53 million in Kenya and 206 million in Nigeria; World Bank Citation2022). Moreover, the heterogeneity in ethnicities, religions, and regional labor markets suggests quite complex interactions between the role of norms and institutions in the interference of women’s engagement in economic activities (De Giusti and Kambhampati Citation2016; Efobi, Adejumo, and Atata Citation2021). The purpose of analyzing the longitudinal data was to study two key pandemic issues in these contexts: women’s substitution of paid for unpaid work, and how these shifts compromise their mental health.
Before the pandemic, African women already devoted more time on average to home production than paid employment. Using microdata from five African countries, Taryn Dinkelman and L. Rachel Ngai (Citation2022) show, on average, women spend between thirty-two to forty-seven hours on domestic activities, such as cooking, cleaning, and laundering the clothing of household members, while only seven to seventeen hours on market work. The market work performed by women is often unpaid, designating time to family farms or enterprises that provide flexibility in work location, hours, and access to older person or child care offered by family members at home. Dinkelman and Ngai (Citation2022) attribute two factors to women’s burden of domestic work before the pandemic: (i) the absence of infrastructure to quicken performance of domestic tasks (for example, transportation, electricity, refrigeration) and support affordable family care; and (ii) the presence of social norms that stymie women’s ability to devote more time to the labor market (Dinkelman and Ngai Citation2022). These constraints have been shown to influence women’s engagement in economic activities in Kenya (De Giusti and Kambhampati Citation2016; Muriithi, Mutegi, and Mwabu Citation2020) and Nigeria (Chimhowu et al. Citation2012).
We find women are increasingly vulnerable to the burden of domestic work, inactivity, and unemployment amid COVID-19. They self-report both an increase in time devoted to domestic care (38 percent in Kenya and 30 percent in Nigeria) and a decrease in time devoted to paid employment (46 percent in Kenya and 29 percent in Nigeria) between October – December 2020 and April 2021. The fact that the changes in domestic work and employment are similar in magnitude suggest substitution between unpaid and paid labor. We further observe their employment status changed: 12(17) percent of women who were employed in October – December were unemployed by April in 2021 in Kenya (Nigeria). We then estimate linear probability models (LPMs) to understand which behavioral factors discussed in the feminist economics literature drive women to substitute paid for unpaid work, focusing on agency, time constraints, demographic, wealth, and preferences.
The second issue relates to the contribution of the burden of domestic work on women’s well-being. Prior to the pandemic, mild to severe depression in Kenya was reported to be as high as 42 percent (Ndetei et al. Citation2009), and 34 percent among young women (15–24 years old; Kilburn et al. Citation2016). Depression rates of women remain lower in Nigeria than in Kenya at 6.3 percent (Adewuya et al. Citation2018b), although these figures may be subject to downward bias (Kessler et al. Citation2009; Adewuya et al. Citation2018a). According to our surveys, the mental health of women in Africa has deteriorated. Over a five-to-seven month period, we find a quarter to a third of women in Kenya and Nigeria report losing interest in doing things; feeling down, depressed, or hopeless; feeling nervous, anxious, or on edge; or feeling like they were not able to stop or control worrying. Our estimates are on par with prevalence rates for anxiety (31 percent) and depression (30 percent) symptoms collected in Sub-Saharan Africa amid the pandemic (Chen et al. Citation2021).
To determine the potential welfare benefits of alleviating the domestic burden on women, we estimate correlates of the change in domestic work with the tendency to report being depressed and anxious between rounds. Women who experience a greater burden in unpaid domestic work over time are 7.4 percentage points more likely to feel down, depressed, or hopeless in Nigeria, and 6.2 and 5.7 percentage more likely to be unable to stop or control worrying in Kenya and Nigeria, respectively. Protecting women’s well-being will involve lightening the burden of domestic or unpaid work for women, particularly where norms around the division of labor of household production and constraints on women’s labor force participation may be leading drivers of these outcomes amid the pandemic.
EXTENSIONS TO THE LITERATURE
Our research connects to the existing literature in three fundamental ways. First, there are a growing number of assessments that corroborate that women were encumbered by the pandemic due to national policies to prevent transmission of COVID-19 and gender inequities in the division of domestic labor (Del Boca et al. Citation2020; Agarwal Citation2021; Desai, Deshmukh, and Pramanik Citation2021; Zamarro and Prados Citation2021). Few offer the perspectives of African women. Contrary to the experiences of women in high-income settings, school closures were already removed in Kenya and Nigeria at the beginning of our study (Mueller et al. Citation2022). Similarly, workplace closures were relatively lenient in both countries (Figure A1) in part due to low numbers of confirmed cases relative to previous case studies (Figure A2). The pandemic created a unique set of challenges that might have contributed to the domestic burden of African women at this juncture. Disruptions in transport and value chains affected employment opportunities and also inflated prices, potentially reducing the disposable income available for households to spend on items or services considered “non-essential” (Mueller et al. Citation2022). Furthermore, according to our qualitative study, women claimed to spend more time than usual cleaning as a disease-control mechanism. These increased demands on their time would have worsened during the restrictions imposed (March 2021 in Kenya) on the movement of people in and out of urban areas, and under national curfews that were implemented intermittently (Hale et al. Citation2020). Such policies likely affected the accessibility of service labor for women to outsource their domestic tasks.
Second, our work bridges the existing literature on intrahousehold relations and their effect on the allocation of labor, paying particular attention to whether these relationships persist or change in pandemic settings (Doss and Meinzen-Dick Citation2015; Doss and Quisumbing Citation2020; Laszlo et al. Citation2020). We focus on how women’s agency affects substitution between paid and unpaid labor amid the pandemic. Agency, characterized as the ability to define and implement one’s goals (Kabeer Citation1999), is operationalized in previous studies using metrics that capture women’s control over resources and mobility (Hanmer and Klugman Citation2016) or over how they divide their time across activities (Eissler et al. Citation2021), among others. Our interest is to examine the extent the level of agency may worsen during hardships and, consequentially, how that affects decisions related to paid and unpaid work (Roy et al. Citation2015).
In addition to individual agency, we consider other known influential factors at the individual and household level on time spent in paid and unpaid work. In places with low marketization of home services, women face competing constraints on the time available to work. Norms around caregiving children and other members of the household suggest women with more children or who live in large households may be less likely to participate in the labor force (Gammage, Joshi, and van der Meulen Rodgers Citation2020). COVID-19 risk within the community can also elevate demand for the unpaid labor of women, particularly within contexts where women rely on exchange labor from neighboring kin for caregiving (Sear et al. Citation2002; Sear and Mace Citation2008). Finally, demographics, wealth, and preferences also factor into time-use decisions in distinct ways. The age of a household member, for example, may dictate who holds primary responsibility for specific activities within the household, while education can excuse members from domestic work given the opportunity cost of their time. Asset wealth which allows women to afford hired labor may be countered by other preferences, such as a women’s time preference (or discount rate). Aversion to COVID-19 risk may differ by gender (Oreffice and Quintana-Domeque Citation2021), and, thus, women may be willing to exit the labor force now in order to protect family members from contracting the disease, which has future benefits.
Our analysis also links to a third literature that evaluates how changes in the employment status of women due to economic shocks negatively impacts their mental health (Currie, Duque, and Garfinkel Citation2015; Cygan-Rehm, Kuehnle, and Oberfichtner Citation2017; Wang, Wang, and Halliday Citation2018; Briody, Doyle, and Kelleher Citation2020). The loss of employment can lead to both positive and negative health behaviors. On the one hand, the unemployed may have time to invest in healthier practices, such as exercise or visiting the doctor, and less income to consume vice goods. On the other hand, employment loss may create financial stress, which can prompt coping mechanisms that exacerbate one’s mental health. Liquidity constraints may preclude the unemployed from seeing medical attention to maintain their health. The emerging literature on the impacts of COVID-19 consistently finds declines in women’s mental health, yet the mechanism underlying the decline varies by context. Gemma Zamarro and María J. Prados (Citation2021) show that working women are much less likely to experience anxiety and depressive symptoms relative to unemployed women in the US. However, women who work remotely are more likely to deal with mental health setbacks, presumably due to the increased burden of unpaid work introduced by school closures and lockdowns (Zamarro and Prados Citation2021). Sonia Oreffice and Climent Quintana-Domeque (Citation2021), in contrast, attribute gender gaps in health concerns about COVID-19 to gender differentials in mental health rather than employment in the UK. Natalie Bau et al. (Citation2022) evaluate the effect of containment policies on India. Declines in mental health associated with containment comove with positive associations with food insecurity potentially corroborating the mechanism of financial stress. Although we examine how changes in domestic care influence employment decisions during the pandemic, our main objective is to understand the linkages proposed in Zamarro and Prados (Citation2021) in a low-income setting. That is, we want to understand the extent changes in mental health status during the pandemic are associated with an increased burden in domestic work in accordance with our qualitative study that identifies such as a major source of stress. Although the disproportionate burden of domestic work is documented in the feminist economics literature (Gammage Citation2010; Amarante and Rossel Citation2018), our study contributes to the understanding of the gender inequalities that arise from this burden focusing on one adverse effect, poor mental health (MacDonald, Phipps, and Lethbridge Citation2005; Liu, MacPhail, and Dong Citation2018).
DATA
Quantitative surveys
The quantitative analysis uses two individual panel surveys collected in Kenya and Nigeria in October – December 2020 (Round 1) and April 2021 (Round 2). The study was authorized by an Institutional Review Board at Simon Frasier University in agreement with Arizona State University (FWA 00009102; IRB Registration Number: IRB00000128). The sampling frame was based on Random Digit Dial (RDD) methods. Phone numbers are made available through third-party distributors and randomly called to build a sample of women respondents. Women are screened according to a few additional criteria besides gender: their age (at least 18 years of age), language, and willingness to be considered in follow-up surveys requiring additional identifying information (contact information) and name. Innovations for Poverty Action was responsible for the survey logistics and implementation (see Mueller et al. [Citation2022] for a more detailed description of the surveys and their protocols).
Although gender gaps in mobile phone ownership are small in Kenya (7 percent) and Nigeria (4 percent; Carboni et al. Citation2021), the RDD sample is unlikely representative of women at the national level. This is a function of the eligibility criteria imposed when screening women per above and the composition of women owning and using mobile phones. Our sample of women will tend to overrepresent literate, wealthy women who live in urban areas where connectivity is less of a problem (Alvi et al. Citation2020; LeFevre et al. Citation2020; Hersh et al. Citation2021).
A second source of sample selection bias may stem from attrition. The original samples comprised 1,296 women in Kenya and 1,146 women in Nigeria. Attrition rates between rounds were 20 and 21 percent in Kenya and Nigeria, respectively (Mueller et al. Citation2022).Footnote1 The panel sample, which includes those with non-missing responses for the outcomes and explanatory variables used in the analysis, consists of 1,027 women in Kenya and 882 women in Nigeria. Panel survey weights are utilized in all statistical analyses to correct for the representation of the sample along dimensions of gender, age, region, and phone ownership (World Bank Citation2020) as well as attrition (Himelein et al. Citation2020).
We analyze the correlates of four time-use outcomes. In Round 2, we asked the respondents to evaluate whether the time spent on various tasks changed since the time of their last interview (Round 1). There were five possible responses that the interviewees could select to project the amount of effort that changed per task over time: (i) gone down by a large amount; (ii) gone down by a small amount; (iii) stayed about the same; (iv) gone up by a small amount; or (v) gone up by a large amount. From their answers to these questions, we create three binary outcomes that reflects whether their time spent on cooking or other domestic tasks increased, whether their time spent on care devoted to children increased, and whether their time spent on paid employment decreased between rounds. For the domestic work and childcare outcomes, a value of one is assigned if the person indicated a (iv) or (v) for the activity, and zero otherwise. For the paid employment outcome, a value of one is assigned if the person indicated (i) or (ii) for the activity, and zero otherwise. Table 1 shows the proportion of women with increased amounts of childcare (41 and 34 percent in Kenya and Nigeria) and domestic work (38 and 30 percent in Kenya and Nigeria). The proportion of women exhibiting reductions in the time spent on paid employment are on par with those reporting gains in domestic work and childcare. Forty-six and 29 percent Kenyan and Nigeria women, respectively, report reducing their paid work hours.
Table 1 Time use, employment, and mental health outcomes
Information from the time-use module above allows us to observe which factors affect changes in paid employment decisions at the intensive margin. To capture modifications to paid employment at the extensive margin, we create a fourth binary outcome based on information from the employment module in the survey. Specifically, in both rounds, we asked the respondent: “In the last 7 days, did you work for someone else/self for pay, for one or more hours?” To reflect whether women became unemployed over the two rounds, we create a binary outcome that assigns women a value of one if they answered “yes” to the employment question in Round 1 and “no” to the employment question in Round 2, and zero otherwise. Table 1 shows that approximately 12 and 17 percent of women in Kenya and Nigeria who were active in the labor force in Round 1 became inactive or unemployed by Round 2.
In both survey rounds, we also asked each woman four standard questions predictive of depression and anxiety in the mental health literature (Löwe, Kroenke, and Gräfe Citation2005; Kroenke et al. Citation2007; Plummer et al. Citation2016). Specifically, we asked over the last two weeks how often the respondent has been bothered by the following problems: little interest or pleasure in doing things; feeling down, depressed, or hopeless; feeling nervous, anxious or on edge; and not being able to stop or control worrying. The possible responses to each of these problems are: (i) not at all; (ii) several days (1–7 days); (iii) more than half the days (8–12 days); and (iv) nearly every day (13–14 days). Based on the answers to these questions, we create four binary outcomes to quantify whether a women became depressed or anxious between rounds. Specifically, if the person gave a response of (i) to a question in Round 1 and a (ii), (iii), or (iv) to the same question in Round 2, we assigned her a value of one and zero otherwise. According to the statistics in Table 1, about a quarter to a third of women in Kenya and Nigeria account becoming more depressed and anxious according to these four metrics over the course of the study.
We categorize the explanatory variables into three main drivers of time-use decision making: agency, time constraints, and demographics, preferences, and wealth. To capture agency over a women’s decisions, we create variables that proxy a women’s control over resources, such as her relationship to the household head (indicators for head of the household, child or child-in law, other relationship; omitted category is head’s spouse), marital status (indicator for married), and a dichotomous variable for agency over COVID-protective behavior. Relationship to the household head and marital status were collected in Round 1. The COVID-protective behavior variable was created from the responses to a series of four questions asked during Round 2, regarding who makes decisions to: (i) wear face masks; (ii) purchase sanitizers/masks/gloves; (iii) stay at home to social distance; and (iv) travel within town/county/country. A binary variable was created from that information, assigning a value of one if the respondent is the sole decision maker of activities (i) – (iv), and zero otherwise. Approximately, 62 percent of women in Kenya and 48 percent of women in Nigeria expressed having agency over COVID-19 protective behavior (Table 2).
Time constraints are featured in the empirical model through the inclusion of the following variables. From Round 1, we create a set of dichotomous variables that indicate the number of primary-school-age children residing in the household (household has 1–2, >2; omitted category is household has no primary-school-age children). We also add variables that reflect the change in household size between rounds and COVID risk, using demographic information collected in Rounds 1 and 2. The latter is a binary variable that assigns a value of one, if the woman did not know a person with COVID-19 in Round 1 but did know a person with COVID-19 in Round 2.Footnote2 Fourteen percent of women in Kenya became aware of a person with COVID-19 in their network in Round 2, while only five percent of women in our Nigerian sample experienced a similar increase in risk (Table 2). A final set of time-constraint variables were generated using data from the twenty-four-hour time-use module administered in Round 1, where we ask women to report the hours they spent on a series of activities. The baseline time-use variables are: whether the women spent at least two hours on domestic tasks, whether the women spent at least two hours on childcare, and whether the women spent at least five hours on school, paid or unpaid work activities. Interestingly, while only 37 and 36 percent of women spent over two hours on caregiving at baseline in Kenya and Nigeria, the majority of women were spending that amount of time on domestic work (Table 2). This potentially suggests that the increased demand on domestic work may be creating a greater time burden than childcare in our sample of women.
The last group of explanatory variables is used to describe the demographic and wealth of the women’s household, as well as her preferences for sacrifice. The demographic and wealth variables are created from information collected in Round 1. Secondary and post-secondary education binary variables control for the highest level of education attended. We also add dichotomous variables to reflect the age category (26–35, 36–45, >45 years old; omitted category 18–25) and wealth (middle and high asset tercile; omitted category is low asset tercile) of women. The statistics in Table 2 indicate that the majority of women in our sample have at least attended some form of secondary school and are in the 18–35 age range. To capture a women’s time preference, we use a measure applied in the behavioral economics literature (Falk et al. Citation2018). In Round 2, we asked: “How willing are you to give up something that is beneficial for you today in order to benefit more from that in the future?” Women are asked to rank their willingness on a scale from 0 to 10. From this information, we create a binary variable, which assigns a value of one to those who report a value greater than 8. Table 2 indicates that half of the women in our sample expressed great willingness to sacrifice something beneficial now for greater future benefits.
Table 2 Means of explanatory variables
Qualitative survey
The quantitative survey data was supplemented with qualitative data collected from in-depth interviews with women in Kenya and Nigeria representative of particular constituent groups at risk of significant effects of the non-pharmaceutical interventions introduced by governments to mitigate the spread of COVID-19. These groups were identified through systematic mapping of gray and academic sources, utilizing the COVID-19 Gender Matrix framework (Smith et al. Citation2021). Such a matrix approach offers a structure to analyze the effects of public health interventions to understand how gendered power relations manifest as inequalities and the structures that determine such outcomes (Jhpiego Citation2020). This allowed us to sift through publicly available material, such as media reports, NGO reports, government policy documents, and academic literature to understand how the pandemic was manifesting amid societies in Kenya and Nigeria, and whether particular sub-sections of society, and indeed particular groups of women were particularly affected by government interventions. Searches were not exhaustive but aimed to offer a rapid scoping of the gendered effects of the outbreak in each country and to identify pertinent themes and groups for further analysis.
From this matrix approach, we subsequently identified key constituent groups in each country for in-depth interviews to explore the data generated by the surveys. In Kenya, the qualitative data collection focused on women community health volunteers (CHVs); women owners of small and medium size enterprises (SMEs); women domestic workers (those paid for work within households, for example, childcare, housework, cook, these are often internal migrants within Kenya); adolescent girls ages 13 to 19; and pregnant women and new mothers. Interviews were undertaken both in Nairobi (urban informal settlement) and Migori (rural county). Recruitment for interview participants in Kenya depends on the sample. For the women SMEs and domestic workers, they were recruited by disseminating requests through women’s groups and community stakeholders in the selected study areas. For the CHVs, those attached to the LVCT Health (a Kenyan non-governmental organization) site were approached and a snowballing technique was then used to generate additional names for this target population. Table 3 displays the final sample interviewed from urban (104 women) and rural (96 women) locations in Kenya by their profile. For the purpose of this analysis, the majority of data used were derived from the SME, domestic worker and adolescent sub-groups.
In Nigeria, the constituent groups were healthcare workers; adolescents; persons with disabilities; women in slum communities; and women working in informal markets. Interviews were undertaken in Lagos State, South West Nigeria. Participants were recruited through convenience sampling from identified groups, faith-based groups, non-governmental organizations, and government institutions. For the purpose of this analysis, the majority of data used were derived from comparable sub-groups to that of Kenya: adolescent girls, informal market workers, and women from slum communities. Table 3 illustrates the composition of the sample of forty-seven women who were interviewed in Lagos.
Table 3 Qualitative sample composition
Interviews were conducted between December 2020 and May 2021. Ethical approval was obtained from the Kenyan (AMREF ERSC P917/2020; NACOSTI research permit license number NACOSTI/P/21/8998) and Nigerian governments (Lagos State Government, Health Research Ethics Committee, Registration No. NHREC04/04/2008). Interviews were conducted by phone or in person. When conducted in person, these occurred in community spaces and with the necessary COVID-19 preventative measures in place, as defined by contemporary government regulations; informed consent was obtained from each participant, prior to the commencement of the interview. A thematic interview guide was developed based on gendered power relations seeking to interrogate women’s experience of the COVID-19 pandemic to understand who has what (access to resources); who does what (division of labor and household bargaining); how values are defined (social norms, ideologies, beliefs and perceptions) and who decides (decision making practices). Interviews were recorded, where permitted, and subsequently transcribed verbatim (Morgan et al. Citation2016). The interview guides are included in Section 2 of the Supplemental Online Appendix.Footnote3
Analysis of the data was performed using framework analysis (Ritchie and Spencer Citation2002). Nicola K. Gale et al. (Citation2013) explain that there are seven steps in the procedure for analysis: (1) transcription; (2) familiarization with the interview; (3) coding; (4) developing a working analytical framework; (5) applying the analytical framework; (6) charter data into the framework matrix; and (7) interpreting the data. Following steps 1 and 2, two researchers reviewed the transcripts to identify key trends in the data and create an iterative process for coding the transcripts. Thematic areas were identified, and subsequent second order analysis was conducted along the domains of the aforementioned COVID-19 gender matrix to identify key tenets of the data to explore themes identified in the surveys.Footnote4
QUANTITATIVE EMPIRICAL METHODS
Determinants of time allocation
The intent of the study is to understand which women increase their domestic workload as the pandemic ensues, and, then, the subsequent implications on their mental health. We first estimate a linear probability model (LPM) to investigate the selection of women into positive changes in time spent on domestic activities between Rounds 1 and 2:
(1)
(1)
In (1), the change in time use
is dichotomized, which allows us to measure the effect of each explanatory variable on the likelihood of having an increase in domestic responsibilities over time. All specifications also include an urban fixed effect
, allowing the magnitude of the intercept to vary by location. Standard errors are clustered at the county level for Kenya and state level for Nigeria, allowing for time use and labor activities to be correlated with unobserved labor market features or policy responses at the administrative level.
We estimate (1) using a LPM to identify whether women with specific attributes are more inclined to face a time burden during the pandemic and inform the targeting of programs designed to protect women from exiting the labor force. For this purpose, the explanatory variables are classified into three categories to address which aspect of decision-making affects the time burden: agency over time use, time constraints, and demographics, preferences, and wealth. Agency is captured through whether the women is the head of the household, a child or child-in-law, or has another relationship to the household head (omitted category is the head’s spouse); her marital status (); as well as her decision-making power over COVID-protective behavior (
). Time constraints are reflected by whether the household has 1–2, or greater than 2 primary-school-age kids in the household (
); whether the household size increased between rounds (
; and whether she spent at least two hours on domestic tasks at baseline
. Lastly, we also include a set of variables in the model that pertain to the woman’s demographic and wealth background, as well as preferences. Education (secondary and post-secondary education), age (26–35, 36–45, >45 years old), and wealth (middle and high asset tercile indicators) are implicit in
. We additionally capture a women’s preferences for sacrificing something beneficial today that has greater benefits realized in the future, included in vector
.
Although the focus in (1) is on changes in domestic activities, we estimate versions of the model replacing the main dependent variable with three other dichotomous variables: an indicator for whether a woman experienced (i) a reduction in paid activities; (ii) a loss in employment; and (iii) an increase in time spent on child caregiving. We analyze outcomes (i) and (ii) to learn whether factors that influence domestic work correspond with factors that influence employment at the extensive and intensive margin. If, for example, married women are more susceptible to increases in domestic work and similarly experience declines in their supply of labor, then we can triangulate that married women may be substituting paid labor for unpaid labor activities during the pandemic. Taken together, this would suggest that agency over the types of activities a woman engages in may be one possible root of the inequities at play. Similar interpretations could be made if the relationships between domestic care (and employment), time constraints, and demographic and wealth variables comove in identical directions. We additionally run parallel analyses evaluating changes in time spent on childcare to investigate whether alternative forms of substitution between paid and unpaid work occur.
There are limitations in the interpretation of our results. First, seasonality may be an important dimension to consider when evaluating the time use of rural women. The first round was collected following the lean season in Kenya and Nigeria, while the second round coincided with the main “long-rain” season in Kenya, the end of the dry season in northern Nigeria and the wet season elsewhere. The amount of tasks required to sustain household production might accrue with the demand for family labor devoted to land preparation and harvesting. Although the majority of the respondents come from urban areas in Nigeria, the issue might complicate the interpretation of findings in Kenya. We lack power to differentiate effects by location, but recognize this as a limitation of the study.
An additional shortcoming of our study is that we have few time-variant variables at the individual and household level that can be used in the model. The studies are only a few months apart, and therefore little differences exist in the demographic and wealth variable values across rounds. We therefore also included lagged time-use variables () as predictors of the change in time use. The rationale is that intrinsic to the lagged allocation of time are unobserved idiosyncratic factors that affect it and its trajectory. Thus, we aim to control for these unobserved factors in the domestic care specification, by adding an explanatory variable that depicts whether the person spent at least two hours on domestic work at baseline. Meanwhile, we use indicators for whether the person spent at least five hours on school or work and two hours on childcare in specifications of employment and childcare, respectively, to accomplish the same objective.
Nevertheless, the relationships we estimate may only be considered correlational due to the limited number of time-variant and time-invariant variables collected in the survey. Thus, concerns over the endogeneity of the change in domestic care variables remain and potentially leave room for bias in the parameter estimates.
Mental health-domestic burden nexus
We next estimate the relationship between the changes in mental health and domestic care
, using a separate specification for each of the four binary dependent variables (developing a lack of interest, feeling down, becoming nervous or worried between Rounds 1 and 2):
(2)
(2)
All explanatory variables are similar to those included in (1), except the change in domestic care
replaces the lagged time-use variable
. Standard errors remain clustered at the regional level, as before.
Our approach controls for a variety of factors that are likely to influence mental health, however, it may continue to introduce parameter bias due to the omission of important factors in the model that influence both adjustments in domestic care as well as well-being. We worry about the pervasiveness of shocks over the time period that is likely to affect well-being as well as the household allocation of labor. For example, if elderly members of the household contribute to domestic work and become vulnerable to transmission over the two periods, then a woman’s mental health might suffer and domestic responsibilities increase. While we control for the change in knowing a person with COVID-19 and household size in (2), there are likely a number of other external shocks (some related to policy responses or local economic resilience as a whole) left unaccounted for in our model.
RESULTS
Determinants of change in time spent on paid and unpaid activities
Figure 1 presents the parameter estimates of the agency, time constraint, demographic, preferences, and wealth variables included in (1) and their 90 percent confidence intervals. We present the results from models that employ four time-use dependent variables: a binary variable for whether the woman reduced the time spent on paid activities (PT), a binary variable for whether the woman became unemployed or inactive between Round 1 and 2 (EL), a binary variable for experiencing an increase in childcare burden (CT), and a binary variable for experiencing an increase in domestic work (DT).
Figure 1 Relationship between time-use outcomes and explanatory variables (90 percent CI)
Notes: KE: Kenya; NG: Nigeria; PT: Loss in paid time; EL: Loss of employment; DT: Gain in domestic time; CT: Gain in childcare time; DR: Discount rate.
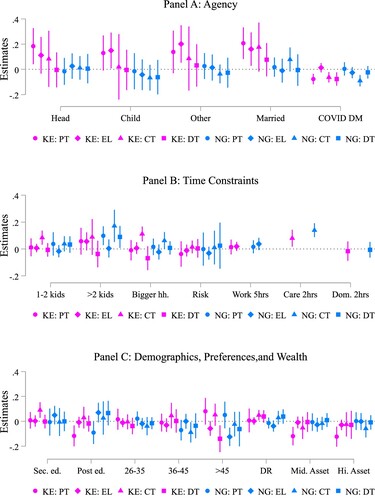
There are only a few factors that appear to be significantly correlated with women’s changes in domestic work. First, women with greater agency are 7.6 percentage points less likely to experience an increase in domestic work in Kenya (Online Appendix Table A.1). These findings are consistent with findings from the qualitative study. The burden of domestic tasks was often to women of the household, with limited authority over the intrahousehold allocation of labor. As one young woman in Nairobi stated, “I could be meeting my buddies, but first I have to do the housework. By the time I am through there is no time [to do anything else].” Another young woman in Nairobi echoed these sentiments: “I’m the one who does them because she believes she gave birth to a girl so it’s my job to clean.” Here, we identify adolescent girls and young women, with little household bargaining power/agency, as particularly vulnerable to these domestic time-use changes.
Women with greater agency were also less likely to reduce their paid activities in Kenya and experience gains in childcare. In particular, the point estimate in the paid activities regression is similar in magnitude to that in the domestic work regression (−0.077 in Online Appendix Table A.2 compared to – 0.079 in Online Appendix Table A.1). A woman miner in Migori, Kenya articulated the tradeoffs she made to maintain her employment schedule at the expense of maintaining the household, particularly in the midst of a national curfew which imposed additional frictions on the job search and commuting. She adds:
My house chores are affected because I would rather rush and work to get paid then come later and continue with my chores because I have to keep time so that the curfew time can get me back home. Sometimes you find that I will neglect my house chores like I will leave dirty clothes to pile while I am busy chasing the paid work because I don’t know when next the curfew time will be adjusted so you find that you tend to neglect your house chores while chasing the paid work.
It changed because they were always at home. I had to wash more now. Their (children) clothes are dirty because they are playing, even though its indoors, but they now have to wear more house clothes. So, because of that, my laundry workload increased. I have to wash more plates as well because everybody is eating. Then I have to tidy the house because the children will definitely play and during playing, they will scatter the house. So, all these things are contributed to the increase in my workload.
Relationship between poor mental health and the burden of domestic work
Figure 2 provides the parameter estimates and their 90 percent confidence intervals for model (2) using four binary dependent variables which capture increases in depression (whether women lost interest in doing things or felt down, depressed, or hopeless) and anxiety (whether women became nervous, anxious, or on edge or were not able to stop or control worrying) in between rounds. In Nigeria, there is a positive correlation between the burden of domestic work and depression. Women with a positive change in domestic care since round 1 are 7.4 percentage points more likely to report feeling down, depressed, or hopeless (Online Appendix Table A.3). In Kenya and Nigeria, there is a positive correlation between the burden of domestic work and anxiety. Women who report an increased burden in domestic care in Kenya (Nigeria) have an increased likelihood of losing the ability to stop or control worrying by 6.2 (5.7) percentage points (Online Appendix Table A.4). Increased domestic work appears to be correlated with poor mental health outcomes of women. Interestingly, no similar relationship is detected between the change in the burden of childcare and mental health (Online Appendix Figure A3).
Figure 1 Relationship between mental health outcomes and domestic activities (90 percent CI)
Notes: KE: Kenya; NG: Nigeria; Int: Lack of interest since round 1; Down: Felt down since round 1; Nerv.: Felt nervous since round 1; Worr.: Felt worried since round 1.
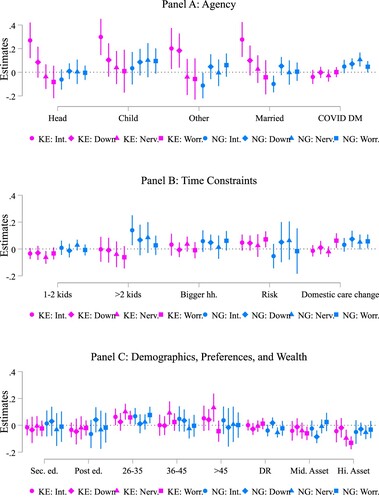
While the qualitative study did not include specific markers of anxiety or depression in their instruments (Section 2, Online Appendix), accounts from multiple women in Kenya and Nigeria corroborate their feelings of distress associated with an increase in domestic work. A separated, frozen food seller in Nigeria expressed details of why she feels down:
I’m tired of everything. I cannot lie to you my sister. Even in my house, if I want to sweep, everything I want to do with my children, it’s too heavy for me. I’m tired of it. Sometimes, I will wake up around 3 and do whatever I want to do before I will be going in the morning.
Yes, it (household responsibilities) increased, in the aspect that everybody is just depending on me. I am at home now and everybody is at home. They (family) put pressure on me in terms of cooking because now when everybody is home we have to be cooking every time.
Things that you used to enjoy, things that you used to do, now you can’t do them anymore because of this virus … this COVID-19, it has led to pressure because people are being stressed and stress leads to pressure. Now it has led to high rate of pressure.
DISCUSSION
Using qualitative and quantitative evidence, we establish women in Kenya and Nigeria are experiencing an increase in their domestic burden at home amid the pandemic. Substitution between paid and unpaid activities occurs in result. The change in the domestic burden was correlated with poor mental health outcomes among women (anxiety and depression in Nigeria only). Women have reported feeling at their “bandwidth” with the increase of domestic labor at a cost of or in parallel to paid work, with little respite available owing to social distancing requirements. The sudden occurrence of the pandemic without prior warning to women, combined with the lack of a foreseeable end to many non-pharmaceutical interventions make it particularly difficult to bear. Protecting women’s well-being will involve additional support to lighten the burden of domestic or unpaid work for women, particularly where norms around the division of labor of household production and time constraints on women’s labor force participation may be leading drivers of these outcomes amid the pandemic.
Models of time use suggest that agency (in Kenya) and the number of young children (in Nigeria) are consistently associated with changes in the time devoted to paid and unpaid activities. Women with greater agency may be delegating the increased burden to adolescent women, domestic workers, or migrant women. In situations, where young members of the household rely on women, the availability of resources and services that typically would be given by members in one’s informal network have become limited. Asset wealth mediates the effects on anxiety, which suggests that some women may be able to replace declines in informal exchange labor with hired labor to overcome the additional time constraints incurred by the pandemic (Figure 2). Social protection programs going forward may be necessary to protect the leisure time of adolescent women and to allow poorer segments of the population opportunities to afford private caregiving services while informal support systems are disrupted.
Our analysis suffers from a few limitations. Our sampling strategies pose concerns over the external validity of our findings. The quantitative and qualitative samples do not allow us to reflect on the state of crisis experienced by the average woman living in Kenya or Nigeria. In surveying women over the phone, we likely provide the perspectives of a more privileged class of women given gender discrepancies in mobile phone ownership in these countries. Furthermore, the sampling strategy of the qualitative study faces the opposite problem, as vulnerable groups identified in the pandemic literature established which women were interviewed. Given the sampling frames likely differ across qualitative and quantitative studies, we might tend to overrepresent insights from urban women, women working in certain sectors, and young women when providing evidence on the qualitative side to support the quantitative findings. As it becomes safer to implement field surveys at scale, future research would benefit from not only expanding the scope of women covered in a quantitative survey but also ensuring sufficient overlap in women represented in the qualitative surveys.
Second, the relationships estimated in the paper are purely descriptive as multiple sources of biases likely exist due to our inability to conduct field surveys during the pandemic at scale (geographically and temporally). Relying on a short survey instrument precluded incorporation of a sufficient number of controls in the regression analysis. An alternative empirical issue stems from the fact that our measure of changes in domestic care is based on perceptions rather than more objective panel measures on time use. In the second round, we opted to ask women how they perceived their allocation of time to change relative to baseline because of the length of time it took to administer the time-use module at baseline. The omission of important variables and the potential error in measurement of changes in domestic care are left unaddressed in our empirical strategy. This is because identifying exogenous sources of variation at the subnational level that might have contributed to changes in domestic care and be unrelated to changes in mental health outcomes was difficult (Altingdag, Erten, and Keskin Citation2022). The enforcement of COVID policy responses at the national level in addition to the discord in timing between school closures and our interviews rendered addressing the endogeneity bias explicitly in our regression model particularly challenging.
Finally, the fact that follow-up rates are lower than in most longitudinal surveys performed in the field also raises apprehensions over the extent attrition bias affects our conclusions (Dillon, Glazerman, and Rosenbaum Citation2021). The correlation between changes in domestic care and mental health may be influenced by the attrition of women with specific attributes in the follow-up surveys. While our panel survey weights adjust for differences in completion rates across dimensions related to gender, age, education, and region, there may be additional factors unaccounted for that determine attrition tendencies. Future research would benefit from the development of protocols to mitigate attrition in phone surveys, particular as the incidences of adverse events related to climate, political instability, or, in this case, global health grow prompting their use to monitor changes in welfare.
Supplemental Material
Download PDF (479.6 KB)ACKNOWLEDGMENTS
We thank Erika Lisco, Nina Liu, Alice Murage, Bianca Navia, Nicole Wu, and our partners at Innovations for Poverty Action (especially, Henry Atimone, Elliott Collins, Steven Glazerman, John Mungai, Debborah Muthoki Wambua, Michael Rosenbaum, Eric Tawiah, and Shana Warren) for research advice and technical support throughout the project.
SUPPLEMENTAL DATA
Supplemental data for this article can be accessed online at https://doi.org/10.1080/13545701.2023.2174566
Additional information
Funding
Notes
1 We estimated a linear probability model to evaluate the correlates of remaining in the sample. We included baseline covariates in the model, such as schooling, age, marital status, the number of children, whether the residence was urban, whether the person knew someone infected with COVID-19, and an asset index. In Nigeria, the only explanatory variable that was correlated with the probability of remaining in the sample and statistically significant (p < 0.01) was whether the woman reported knowing someone with COVID-19. In Kenya, age (p < 0.1) and the number of children (p < 0.05) were both positively correlated with remaining in the sample and statistically significant.
2 Valerie Mueller et al. (Citation2022) illustrate that the majority of risk stems at the community level, meaning respondents more often report knowing someone with COVID-19 in their community rather than within their household. We recognize that this variable may be flawed in capturing true COVID-19 risk given deficiencies in public testing at the national level and selection issues associated with voluntary testing. However, we use this variable as a proxy for potential disruptions in informal support systems at the community level due to COVID-19 related illness knowing that it is subject to measurement error for the reasons mentioned.
3 All personal information that would allow the identification of any person(s) described in the article has been removed.
4 Examples of applying gender matrices are available at: https://www.genderandcovid-19.org/matrix/.
5 The descriptive statistics for the sample are provided in Online Appendix Table A.5.
REFERENCES
- Adewuya, Abiodun O., Olayinka Atilola, Bolanle A. Ola, Olurotimi A. Coker, Mathew P. Zachariah, Olufemi Olugbile, Adedolapo Fasawe, and Olajide Idris. 2018a. “Current Prevalence, Comorbidity and Associated Factors for Symptoms of Depression and Generalized Anxiety in the Lagos State Mental Health Survey (LSMHS), Nigeria.” Comprehensive Psychiatry 81: 60–5.
- Adewuya, Abiodun O., Olurotimi A. Coker, Olayinka Atilola, Bolanle A. Ola, Mathew P. Zachariah, Tomilola Adewumi, Olufemi Olugbile, Adedolapo Fasawe, and Olajide Idris. 2018b. “Gender Difference in the Point Prevalence, Symptoms, Comorbidity, and Correlates of Depression: Findings from the Lagos State Mental Health Survey (LSMHS), Nigeria.” Archives of Women’s Mental Health 21(6): 591–9.
- Agarwal, Bina. 2021. “Livelihoods in COVID Times: Gendered Perils and New Pathways in India.” World Development 139: 105312.
- Altingdag, Onur, Blige Erten, and Pinar Keskin. 2022. “Mental Health Costs of Lockdowns: Evidence from Age-Specific Curfews in Turkey.” American Economic Journal: Applied Economics 14(2): 320–43.
- Alvi, Muzna, Shweta Gupta, Ruth Meinzen-Dick, and Claudia Ringler. 2020. “Phone Surveys to Understand Gendered Impacts of COVID-19: A Cautionary Note.” IFPRI Policies, Institutions and Markets (blog). July 14, 2020.
- Amarante, Verónica and Cecilia Rossel. 2018. “Unfolding Patterns of Unpaid Household Work in Latin America.” Feminist Economics 24(1): 1–34.
- Bau, Natalie, Gaurav Khanna, Corinne Low, Manisha Shah, Sreyashi Sharmin, and Alessandra Voena. 2022. “Women’s Well-Being During a Pandemic and Its Containment.” Journal of Development Economics 156: 102839.
- Briody, Jonathan, Orla Doyle, and Cecily Kelleher. 2020. “The Effect of Local Unemployment on Health: A Longitudinal Study of Irish Mothers 2001–2011.” Economics and Human Biology 37: 100859.
- Carboni, Isabelle, Nadia Jeffrie, Dominica Lindsey, Matthew Shanahan, and Claire Sibthorpe. 2021. “Connected Women: The Mobile Gender Gap Report 2021.” GSMA.
- Chen, Jiyao, Nusrat Farah, Rebecca Kechen Dong, Richard Z. Chen, Wen Xu, Jin Yin, Bryan Z. Chen, Andrew Yilong Delios, Saylor Miller, Xue Wan, Wenping Ye, and Stephen X. Zhang 2021. “Mental Health During the COVID-19 Crisis in Africa: A Systematic Review and Meta-Analysis.” International Journal of Environmental Research and Public Health 18(20): 10604.
- Chimhowu, Admos, Sushila Zeitlyn, Omowumi Asubiaro Dada, and British Council. 2012. Gender in Nigeria Report 2012: Improving the Lives of Girls and Women in Nigeria. Issues, Policies, Action. 2nd ed. British Council Nigeria.
- Coulibaly, Brahima, ed. 2020. Foresight Africa: Top Priorities for the Continent 2020 to 2030. Washington, DC: Brookings Institute.
- Currie, Janet, Valentina Duque, and Irwin Garfinkel. 2015. “The Great Recession and Mothers’ Health.” Economic Journal 125(588): F311–46.
- Cygan-Rehm, Kamila, Daniel Kuehnle, and Michael Oberfichtner. 2017. “Bounding the Causal Effect of Unemployment on Mental Health: Nonparametric Evidence from Four Countries.” Health Economics 26: 1844–61.
- De Giusti, Giovanna and Uma Sarada Kambhampati. 2016. “Women’s Work Choices in Kenya: The Role of Social Institutions and Household Gender Attitudes.” Feminist Economics 22(2): 87–113.
- Del Boca, Daniela, Naomi Oggero, Paola Profeta, and Mariacristina Rossi. 2020. “Women’s and Men’s Work, Housework and Childcare, Before and During COVID-19.” Review of Economics of the Household 18(4): 1001–17.
- Desai, Sonalde, Neerad Deshmukh, and Santanu Pramanik. 2021. “Precarity in Time of Uncertainty: Gendered Employment Patterns During the COVID-19 Lockdown in India.” Feminist Economics 27(1-2): 152–72.
- Dillon, Andrew, Steven Glazerman, and Michael Rosenbaum. 2021. “Understanding Response Rates in Random Digit Dial Surveys.” Working Paper ID 3836024. Global Policy Research Lab, Social Science Research Network.
- Dinkelman, Taryn, and L. Rachel Ngai. 2022. “Time Use and Gender in Africa in Times of Structural Transformation.” Journal of Economic Perspectives 36(1): 57–80.
- Doss, Cheryl R. and Ruth Meinzen-Dick. 2015. “Collective Action within the Household: Insights from Natural Resource Management.” World Development 74: 171–83.
- Doss, Cheryl R. and Agnes R. Quisumbing. 2020. “Understanding Rural Household Behavior: Beyond Boserup and Becker.” Agricultural Economics 51(1): 47–58.
- Efobi, Uchenna, Oluwabunmi Adejumo, and Scholastica Ngozi Atata. 2021. “Age at First and Current Marriage and Women’s Entrepreneurship in Nigeria.” Feminist Economics 27(4): 148–73.
- Eissler, Sarah, Jessica Heckert, Emily Myers, Greg Seymour, Sheela Sinharoy, and Kathryn M. Yount. 2021. “Exploring Gendered Experiences of Time-Use Agency in Benin, Malawi, and Nigeria as a New Concept to Measure Women’s Empowerment.” IFPRI Discussion Paper 02003. Washington, DC: International Food Policy Research Institute (IFPRI).
- Falk, Armin, Anke Becker, Thomas Dohmen, Benjamin Enke, David Huffman, and Uwe Sunde. 2018. “Global Evidence on Economic Preferences.” Quarterly Journal of Economics 133(4): 1645–92.
- Gale, Nicola K., Gemma Heath, Elaine Cameron, Sabina Rashid, and Sabi Redwood. 2013. “Using the Framework Method for the Analysis of Qualitative Data in Multi-Disciplinary Health Research.” BMC Medical Research Methodology 13(1): 117.
- Gammage, Sarah. 2010. “Time Pressed and Time Poor: Unpaid Household Work in Guatemala.” Feminist Economics 16(3): 79–112.
- Gammage, Sarah, Shareen Joshi, and Yana van der Meulen Rodgers. 2020. “The Intersections of Women’s Economic and Reproductive Empowerment.” Feminist Economics 26(1): 1–22.
- Hale, Thomas, Samuel Webster, Anna Petherick, Toby Phillips, and Beatriz Kira. 2020. “Oxford COVID-19 Government Response Tracker.” Blavatnik School of Government, University of Oxford. Github. Accessed April 15, 2021. Github.com/OxCGRT/covid-policy-tracker/raw/master/data/timeseries/OxCGRT_timeseries_all.xlsx.
- Hanmer, Lucia and Jenni Klugman. 2016. “Exploring Women’s Agency and Empowerment in Developing Countries: Where Do We Stand?” Feminist Economics 22(1): 237–63.
- Hersh, Skye, Divya Nair, Pradyot Bharadwaj Komaragiri, and Raghav Kapoor Adlakha. 2021. “Patchy Signals: Capturing Women’s Voices in Mobile Phone Surveys of Rural India.” BMJ Global Health 6(Suppl 5): e005411.
- Himelein, Kristen, Stephanie Eckman, Jonathan Kastelic, Kevin Mcgee, Michael Wild, Nobuo Yoshida, and Johannes Hoogeveen. 2020. “High-Frequency Mobile Phone Surveys of Households to Assess the Impacts of COVID-19 (Vol. 2): Guidelines on Sampling Design.” Report 148213. The World Bank.
- JHPIEGO. 2020. Gender Analysis Toolkit for Health Systems. Baltimore, MD: John Hopkins University.
- Kabeer, Naila. 1999. “Resources, Agency, Achievements: Reflections on the Measurement of Women’s Empowerment.” Development and Change 30(3): 435–64.
- Kessler, Ronald C., Sergio Aguilar-Gaxiola, Jordi Alonso, Somnath Chatterji, Sing Lee, Johan Ormel, T. Bedirhan Üstün, and Philip S. Wang. 2009. “The Global Burden of Mental Disorders: An Update from the WHO World Mental Health (WMH) Surveys.” Epidemiology and Psychiatric Sciences 18(1): 23–33.
- Kilburn, Kelly, Harsha Thirumurthy, Carolyn Tucker Halpern, Audrey Pettifor, and Sudhanshu Handa. 2016. “Effects of a Large-Scale Unconditional Cash Transfer Program on Mental Health Outcomes of Young People in Kenya.” Journal of Adolescent Health 58(2): 223–29.
- Kroenke, Kurt, Robert L. Spitzer, Janet B. W. Williams, Patrick O. Monahan, and Berndt Löwe. 2007. “Anxiety Disorders in Primary Care: Prevalence, Impairment, Comorbidity, and Detection.” Annals of Internal Medicine 146(5): 317–25.
- Laszlo, Sonia, Kate Grantham, Ecem Oskay, and Tingting Zhang. 2020. “Grappling with the Challenges of Measuring Women’s Economic Empowerment in Intrahousehold Settings.” World Development 132: 104959.
- LeFevre, Amnesty E., Neha Shah, Jean J. H. Bashwinga, Asha S. George, and Diwakar Mohan. 2020. “Does Women’s Mobile Phone Ownership Matter for Health? Evidence from 15 Countries.” BMJ Global Health 5(5): e002524.
- Liu, Lan, Fiona MacPhail, and Xiao-yuan Dong. 2018. “Gender, Work Burden, and Mental Health in Post-Reform China.” Feminist Economics 24(2): 194–217.
- Löwe, Bernd, Kurt Kroenke, and Kerstin Gräfe. 2005. “Detecting and Monitoring Depression with a Two-Item Questionnaire (PHQ-2).” Journal of Psychosomatic Research 58(2): 163–71.
- MacDonald, Martha, Shelley Phipps, and Lynn Lethbridge. 2005. “Taking its Toll: The Influence of Paid and Unpaid Work on Women’s Well-Being.” Feminist Economics 11(1): 63–94.
- Morgan, Rosemary, Asha George, Sarah Ssali, Kate Hawkins, Sassy Molyneux, and Sally Theobald. 2016. “How to Do (or Not to Do) … Gender Analysis in Health Systems Research.” Health Policy and Planning 31(8): 1069–78.
- Mueller, Valerie, Karen Grépin, Atonu Rabbani, Bianca Navia, Anne S. W. Ngunjiri, and Nicole Wu. 2022. “Food Insecurity and COVID-19 Risk in Low- and Middle-Income Countries.” Applied Economic Perspectives and Policy 44(1): 92–109.
- Muriithi, Moses K., Reuben G. Mutegi, and Germano Mwabu. 2020. “Counting Unpaid Work in Kenya: Gender and Age Profiles of Hours Worked and Imputed Wage Incomes.” Journal of the Economics of Ageing 17: 100120.
- Ndetei, David M., Lincoln I. Khasakhala, Mary Kuria, Victoria Mutiso, Fransica A. Ongecha- Owuor, and Donald A. Kokonya. 2009. “The Prevalence of Mental Disorders in Adults in Different Level General Medical Facilities in Kenya: A Cross-Sectional Study.” Annals of General Psychiatry 8(1): 1–8.
- Oreffice, Sonia and Climent Quintana-Domeque. 2021. “Gender Inequality in COVID-19 Times: Evidence from UK Prolific Participants.” Journal of Demographic Economics 87(2): 261–87.
- Plummer, Faye, Laura Manea, Dominic Trepel, and Dean McMillan. 2016. “Screening for Anxiety Disorders with the GAD-7 and GAD-2: A Systematic Review and Diagnostic Metaanalysis.” General Hospital Psychiatry 39: 24–31.
- Ritchie, Jane and Liz Spencer. 2002. “Qualitative Data Analysis for Applied Policy Research.” The Qualitative Researcher’s Companion 573: 305–29.
- Roy, Shalini, Jinnat Ara, Narayan Das, and Agnes R. Quisumbing. 2015. “‘Flypaper Effects’ in Transfers Targeted to Women: Evidence from BRAC’s ‘Tareting the Ultra Poor’ Program in Bangladesh.” Journal of Development Economics 117: 1–19.
- Sear, Rebecca and Ruth Mace. 2008. “Who Keeps Children Alive? A Review of the Effects of Kin on Child Survival.” Evolution and Human Behavior 29(1): 1–18.
- Sear, Rebecca, Fiona Steele, Ian A. McGregor, and Ruth Mace. 2002. “The Effects of Kin on Child Mortality in Rural Gambia.” Demography 39(1): 43–63.
- Seck, Papa, Jessamyn O. Encarnacion, Cecilia Tinonin, and Sara Duerto-Valero. 2021. “Gendered Impacts of COVID-19 in Asia and the Pacific: Early Evidence on Deepening Socioeconomic. Inequalities in Paid and Unpaid Work.” Feminist Economics 27(1–2): 117–32.
- Smith, Julia, Sara E. Davies, Huiyun Feng, Connie C. R. Gan, Karen A. Grépin, Sophie Harman, Asha Herten-Crabb, Rosemary Morgan, Nimisha Vandan, and Clare Wenham. 2021. “More Than a Public Health Crisis: A Feminist Political Economic Analysis of COVID- 19.” Global Public Health 16(8–9): 1364–80.
- von Wachter, Till. 2020. “The Persistent Effects of Initial Labor Market Conditions for Young Adults and Their Sources.” Journal of Economic Perspectives 34(4): 168–94.
- Wang, Huixia, Chenggang Wang, and Timothy J. Halliday. 2018. “Health and Health Inequality During the Great Recession: Evidence from the PSID.” Economics and Human Biology 29: 17–30.
- World Bank. 2020. “COVID-19 Rapid Response Phone Survey with Households 2020–2022, Panel: Waves 1–8.” Reference ID KEN–2020– COVIDRS– v07–M, The World Bank.
- World Bank. 2022. “World Development Indicators [Database].” The World Bank.
- Xue, Baowen, Anne McMunn, and Thach Tran. 2021. “Gender Differences in Unpaid Care Work and Psychological Distress in the UK COVID-19 Lockdown.” PLoS One 16(3): e0247959.
- Zamarro, Gemma and María J. Prados. 2021. “Gender Differences in Couples’ Division of Childcare, Work and Mental Health During COVID-19.” Review of Economics of the Household 19(1): 11–40.