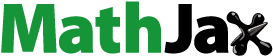
Abstract
The logical intuition hypothesis proposes a difference in the strength between logical and heuristic intuitions. The labels of logical and heuristic intuitions are exclusive to conventional reasoning research. This paper reports the result of testing intuition strength using the dot-probe methodology in a novel multiplication paradigm. Here, “logical intuition” and “heuristic intuition” were relabeled as “weaker intuition” (-1 × 5 = 5) and “stronger intuition” (1 × 5 = 5), respectively, to assess the assumptions about the difference in the strength of “Chinese multiplication mnemonics” intuitions. The dot-probe paradigm was utilized in the following experiments to measure attentional bias. This was assessed by calculating the difference in reaction time between incongruent (the target location is opposite to the probe location) and congruent (the target location is the same as the probe location) conditions. Experiment 1 found attentional bias directed attention to the correct answer for both weaker and stronger intuitions. Experiment 2 replicated and extended Experiment 1 with a secondary task to manipulate high and low cognitive loads. Consequently, stronger intuitions were unaffected by cognitive load and participants were biased toward the correct answer, whereas attentional bias for weaker intuitions only occurred in low load conditions. These results revealed that although both intuitions can guide attention, stronger intuition may guide attention bias in a high-load situation due to its robust automation. Our findings extend the logical intuition hypothesis that proposes a difference in the automated strengths of intuitions to mathematical and analytic thinking.
Introduction
According to dual-process theories, individuals have two distinct cognitive processing systems: intuitive (System 1 or Type 1) and analytical processing (System 2 or Type 2) (De Neys, Citation2012; Evans, Citation2003; Evans & Stanovich, Citation2013a, Citation2013b; Pennycook et al., Citation2015, Citation2018; Sloman, Citation1996). The conventional default-interventionist model (DI) assumes that the outcome of intuitive processing is often biased or erroneous, which subsequently activates analytical processing to intervene and override the biased answer using logic (Evans & Stanovich, Citation2013a, Citation2013b). However, the logical intuition hypothesis proposes that individuals can directly arrive at logical answers through automatic processing (De Neys, Citation2012, Citation2014, Citation2015; De Neys & Pennycook, Citation2019; Reyna & Rahimi-Golkhandan, Citation2017). This hypothesis suggests that individuals may have two different types of intuitive processing, i.e. heuristic intuition and logical intuition. Heuristic intuition aligns with the traditional intuition described in the DI model and automates the application of semantic associations from beliefs stored in the long-term memory (Betsch, Citation2008; De Neys, Citation2014; Williams et al., Citation2019). In contrast, logical intuition is based on simple logical knowledge, and may include matching and figural biases that may result in normative responses while considering probabilistic rules (Pennycook & Thompson, Citation2012; Stupple et al., Citation2013). This enables individuals to automatically generate logical answers without engaging the analytical processing system. The logical intuition hypothesis is supported by extensive research (Bago et al., Citation2018; Bago & De Neys, Citation2017a, Citation2020; Markovits et al., Citation2019, Citation2021; Raoelison et al., Citation2020).
The logical intuition hypothesis has an increasingly compelling empirical basis. For example, Bago et al. (Citation2018) conducted an event related potentials (ERP) study and found that a remarkable N2 component (reflecting conflict detection) was induced under time constraints where the base-rate information was inconsistent with stereotypical descriptions in a two-response paradigm with base-rate neglect problems. Their findings suggested that the participants may be intuitively sensitive to logical/probability rules. Participants’ initial response (in a two-response paradigm) under time pressure, along with a secondary load task, was normatively logical even though the engagement of analytical processing was minimized (Bago & De Neys, Citation2017a). Notably, Bago and De Neys (Citation2017a) proposed that logical and heuristic intuitions have different strengths, which influence performance. Several studies have explored the strength of intuitions described in the logical intuition model (Bago & De Neys, Citation2017a, Citation2017b, Citation2020; Pennycook et al., Citation2015). Pennycook et al. (Citation2015) proposed that when the strength of one intuition dominates the other, people have a tendency to respond with the intuitive response having a superior strength (Bago & De Neys, Citation2017a). Intuitive responses may not be generated at full strength, and different types of intuition may have a higher peak or faster activation. Therefore, studies of intuitive responses should consider their growth and decay (Bago & De Neys, Citation2017b). Furthermore, Bago and De Neys (Citation2020) manipulated the strength of logical intuition by modifying the base-rate information and recorded the participants’ confidence ratings for their initial responses in a two-response paradigm with base-rate neglect problems. The proportion of initial responses regarding the base-rate information (logic) dropped significantly as the base-rate information decreased, indicating a decline in the strength of logical intuition. In other words, each intuition may have a different strength and result in different performance.
The current experimental design utilized “Chinese multiplication mnemonics”Footnote1 ( displays the comparison of Chinese multiplication mnemonics and Times tables) that would elicit two separate intuitions to examine the different strengths of distinct automatic intuitions. Other studies have validated similar experimental manipulations (Yin et al., Citation2018; Yu et al., Citation2018). Multiplication mnemonics were selected as part of the experimental material since automatic intuitions are based on knowledge and associations. Chinese participants would have been exposed to multiplication mnemonics (e.g. 1 × 5 = 5) since pre-school and would have used them as basic mathematical knowledge during primary, middle, and high school (Zhou et al., Citation2007), resulting in the formation of strong semantic associations (De Neys, Citation2014). Children not only use multiplication mnemonics in class and for homework but to solve real-world problems in their daily lives as well. Long-term use of multiplication mnemonics ingrains it in their memory. They can automatically respond with 5 or 6 in answer to 1 × 5 or 2 × 3, respectively. The response 5 or 6 and other answers in multiplication mnemonics have become beliefs or knowledge that are stored in their memory, particularly semantic memory. Moreover, individuals can obtain answers to multiplication equations without deliberation. Therefore, a rapid response for an equation based on beliefs or knowledge is an application of heuristic intuition.
Negative multiplication mnemonics are analogous to logical intuition because the latter refers to normative and correct problem-solving responses that are derived from the automatic application of logical, probabilistic, and mathematical rules. De Neys (Citation2012) emphasized the term “logical intuition” to refer to an intuitive grasp of standard logical and probability theory principles, which arise when a logical solution or principle is easily and automatically activated. Raoelison et al. (Citation2021) highlighted the development of logical intuition as a learning process in which the use of corresponding logical rules is automatized. These applications of logico-mathematical rules are expected to be mastered at school, including the negative-calculation rule or principle. Furthermore, people have a long-term repetitive exposure to these principles in situations arising while teaching or in daily life. These rules have been repeatedly practiced and applied, thereby forming a cognitive architecture (e.g. mindware) (Stanovich, Citation2018). The mindware contains the rules or principles that are necessary to solve problems in which logical intuition can automatically access these rules. Boissin et al. (Citation2021) argued that intuitions could be regarded as a highly specialized set of procedures that are executed automatically when confronted with a corresponding stimulus. Accordingly, the current study included rules for calculating negative numbers, such as “multiplying a negative number by a positive number gives a negative number, and vice versa” (e.g. −1 × 5 = −5, 1 × −5 = −5), in the traditional multiplication mnemonics to elicit responses based on logical intuition.
The use of multiplication mnemonics in the current study is supported by the following reasons. First, the use of multiplication mnemonics instead of classical reasoning tasks mitigates the potential impact of familiarity and difficulty when assessing the strength of heuristic and logical intuitions. Conventional reasoning materials have different degrees of familiarity and interpretation among individuals. It is difficult to induce logical intuition for unfamiliar materials. For example, the familiarity with tasks for base-rate neglect and syllogisms varies amongst individuals (Raoelison et al., Citation2021), which makes it difficult to control individual differences. Considering the cultural background of Chinese participants, individual differences can be easily controlled using multiplication mnemonics since individuals should have automated logical-calculation rules for negative numbers. Therefore, the design issues associated with familiarity and difficulty in thinking and reasoning tasks can be avoided by using multiplication mnemonics.
Second, mathematical and conventional reasoning can be reasonably assumed to have some common ground. Morsanyi and colleagues have intensively studied the strong link between mathematics and reasoning (Morsanyi, Kahl, et al., Citation2017; Morsanyi, McCormack, et al., Citation2018; Morsanyi et al., Citation2013, Citation2014). Interestingly, their recent research showed that performance on conditional inference is moderately correlated with basic arithmetic skills (Morsanyi, McCormack, et al., Citation2018). Van Hoof et al. (Citation2020) further demonstrated that the natural-numbers bias exhibits intuitive characteristics. Specifically, they observed significantly decreased accuracy for incongruent items compared to congruent items under time constraints. This result supports our intention to use multiplication mnemonics for testing the logical intuition hypothesis and dual process theory.
Third, the key difference between heuristic and logical intuitions lies in the presence of logical rules. While heuristic intuitions rely on existing beliefs and may lack a logical basis, logical intuitions are embedded in the application of logical rules and principles. Therefore, two types of material were selected to elicit heuristic and logical intuitions. The positive multiplication mnemonic has been ingrained in memory as a stimulus-response association. When the multiplication mnemonic is repeatedly memorized, it eventually becomes ingrained knowledge or beliefs. When encountering 8 × 9 (i.e. stimulus), Chinese automatically generate 72 as the output (i.e. response) via heuristic intuition. Through repeated memorization and practice, multiplication mnemonics can become internalized as ingrained knowledge or beliefs. People can solve the problem, −8 × 9 = −72, by memorizing and applying this rule.
Labeling classical multiplication mnemonics versus negative multiplication mnemonics as heuristic versus logical intuitions is an analogy in conventional reasoning. Positive and negative multiplication can be associated with heuristic and logical intuitions, respectively, based upon their relative automatic strength, i.e. heuristic intuitions are stronger than logical intuitions (De Neys, Citation2012). Moreover, Fischbein (Citation1987, Citation1999) distinguished between primary intuitions derived from personal experience and secondary intuitions acquired through educational instructions. This perspective is a significant steppingstone in clarifying classical multiplication mnemonics and negative multiplication mnemonics as primary and secondary intuitions because primary intuition is associative and secondary intuition is the automatization of formal rules.
It should be acknowledged that there are some differences between multiplication mnemonics and canonical reasoning problems. Therefore, the current study relabeled “logical intuition” and “heuristic intuition” as “weaker intuition” and “stronger intuition” to test a more general assumption about the difference in the strength of different intuitions. This is consistent with primary and secondary intuitions being associated with stronger and weaker intuitions, respectively.
The next focus is on comparing the strength of the two types of intuitions, which may vary based on the extent of their automation. Attentional bias reflects the extent to which an individual automates information processing (Gladwin & Vink, Citation2018), which is typically manifested in the dot-probe paradigm when individuals respond faster to congruent conditions (where the probe stimulus is spatially congruent with the target) than to incongruent conditions (where the probe stimulus is spatially incongruent with the target) (Torrence & Troup, Citation2018). “Spatially congruent” refers to the probe location being the same as the target location, while “spatially incongruent” refers to the probe location being different from the target location. Attentional bias means that participants focus on the target and then direct their attention to the probe location, displaying a faster RT for the congruent than the incongruent condition. Specifically (see ), two stimuli are displayed on two sides of the screen, followed by the “probe” (i.e. arrow). If participants attend to the target (e.g. 2), their response to the probe will be faster in the congruent condition. If people rapidly and automatically produce the answer for a multiplication equation, they will attend to the target comprising the correct answer. In turn, the response to the probe stimulus would be faster in the congruent than in the incongruent condition. The difference in response time (RT) is used as an index of attentional bias (Torrence & Troup, Citation2018). The remarkable RT-difference between the incongruent and congruent conditions shows constant attention on the target.
Notably, intuitions may influence attention allocation (Evans, Citation2010; Evans et al., Citation1987; Teoh et al., Citation2020). Therefore, it can be inferred that intuitions can guide attentional bias, driving the corresponding behavioral response (de Chantal et al., Citation2020). Participants showed attentional bias toward the matching cards in the Wason selection task (Evans, Citation2010; Evans et al., Citation1987). Participants must test a logical rule, for example, if one side of a card is A, the other side is 3. Participants were required to pick cards from A, D, 3, and 7 to prove the correctness of this rule. Most of the participants chose cards A and 3 based on their intuition, applying a matching heuristic where responses matched the surface features. However, they should have chosen cards A and 7 according to the logical rules. This suggests that when responding using intuition, people selectively attend to the information that is consistent with the intuitive outcome, resulting in an attentional bias. Teoh et al. (Citation2020) proposed that under time pressure, intuitive social preferences drive individuals’ early attentional bias, thereby causing them to prioritize and consistently deploy attention to biased information. Therefore, attentional bias can effectively reflect automated intuitive responses, wherein intuitive top-down processes allocate more attention to the information that is consistent with the outcome of intuitive processing.
This argument leads to an interesting conjecture: the difference in the strength of intuitions would be reflected in the expression of attentional bias. The two types of intuition are activated by heuristic (e.g. stereotype or believability) and/or logical cues (e.g. base rate or validity) in a task, which can prompt different automated responses (Bago & De Neys, Citation2017a, Citation2017b, Citation2020). Since heuristic intuition is typically stronger or peaks more quickly than logical intuition (Bago & De Neys, Citation2017a, Citation2017b; De Neys, Citation2012), attentional bias is suggested to only occur for stronger (heuristic) intuitions. Additionally, previous studies imposed a secondary task on participants, in which a constant cognitive load was present to prevent Type 2 processing (Bago & De Neys, Citation2020). In the present study, the high and low cognitive loads were modulated using a secondary task. If Type 2 processing is involved in the implementation of heuristics, then differences should be observed between high and low loads. It is essential for the logical intuition model to account for any differences between logical and heuristic intuitions. Additionally, it is possible to determine whether Type 2 processing contributes to the strength of heuristic responses by varying the intensity of the cognitive load (Bago & De Neys, Citation2017a, Citation2020).
The present study primarily aimed to examine the different strengths of stronger and weaker intuitions due to a difference in their extent of automation, reflecting on attentional bias via dot-probe paradigm. This indirect method of measuring automatic processing is explorative. First, whether both stronger and weaker intuitions were automatic was verified. Second, the extent to which automatization was different for stronger and weaker intuitions when cognitive load tasks were added was assessed. The intuitive heuristic response might still be stronger than the intuitive logical response (De Neys, Citation2012). In other words, the weaker intuition triggered by negative multiplications may not be as robust as the stronger intuition triggered by canonical multiplications.
In previous studies, researchers conducted two experiments with the goal of reducing the involvement of analytical processing (Bago & De Neys, Citation2017a, Citation2020; De Neys & Pennycook, Citation2019). Both experiments included response deadlines (see Evans & Curtis-Holmes, Citation2005 for an example of this methodology prompting Type 1 thinking in a reasoning paradigm) to limit the participants’ time for calculations. Experiment 1 used a standard dot-probe paradigm to examine whether weaker and stronger intuitions could affect attention when attention was biased toward the correct answer of a multiplication question. Experiment 2 included a cognitive load task to explore whether the attentional bias disappeared due to changes in the cognitive load. Therefore, the hypothesis for Experiment 1 was that only stronger intuition will bias participants’ attention toward the correct answer, indexed by the RT, which should be faster in the congruent than in the incongruent condition. Moreover, the hypothesis for Experiment 2 was that the effect of attentional bias would be replicated under high cognitive load if it is guided by stronger intuitions, which means that the RT would be faster in the congruent than in the incongruent condition under high load.
Experiment 1
Hypothesis: Only stronger intuition will bias participants’ attention toward the correct answers, indexed by the RT, which should be faster in the congruent than in the incongruent condition.
Method
Participants
Considering the 2 × 2 mixed design in Experiment 1, the expected sample size was calculated by G-Power 3.1.9 (Faul et al., Citation2007) using f = 0.25 as the effect size with a power of 0.8 and alpha of 0.05 to detect a medium-sized effect. The analysis revealed an expected sample size of 24. Therefore, about 38 postgraduates (17 males, 21 females) were recruited for this experiment. They were divided into two groups with 19 (9 males, 10 females) in the weaker intuition group and 19 (8 males, 11 females) in the stronger intuition group, falling in an age range of 21 to 26 years (M = 23.42 years, SD = 1.45 years). All participants were native Chinese speakers, healthy, right-handed, with normal or corrected vision, and no neurological disorders. The ethics committee of the author’s institution approved this experiment. Participation was voluntary. Informed consent was obtained from the participants prior to the experiment and monetary compensation was provided at the end.
Design
The experiment utilized a 2 (intuition type: weaker intuition/stronger intuition) × 2 (trial type: congruency/incongruency) mixed design, wherein intuition type was the between-group variable and trial type was the within-group variable.
Materials
The materials were taken from the “multiplication mnemonic.” After removing the nine equations 1 × 1 = 1, 2 × 2 = 4, ……, 8 × 8 = 64, and 9 × 9 = 81, the remaining 36 equations were used as the base material and extended. Specifically (see ), the congruent condition for the stronger intuition group was that the correct answer to the equation appeared in the same position as the arrow. When both conditions were met, the correct answer appeared in either the left or right position and the arrow had an up or down orientation. Accordingly, a total of 144 (36 × 2 × 2) trials were formed under this condition. Half of the trials were presented in the normal order of the equation (e.g. 1 × 2), while the other half were presented in reverse (e.g. 2 × 1). The incongruent condition was that the correct answer to the equation appeared in a different position than the arrow. Other elements in this condition were the same as in the congruent condition. Accordingly, a total of 144 trials were formed under this condition. The non-attention condition was that both answers were correct or incorrect answers; it also contained 144 trials. For the weaker intuition group, it was necessary to not only satisfy the same elements as in the stronger intuition group, but also to balance the position of the presentation of the negative sign in the equation, where it was necessary for the sign to accompany the presentation of the first or second digit in the equation (e.g. −1 × 2 or 1 × −2).
Table 1. Example of material.
In summary, participants completed 432 trials in two blocks in this experiment. To guarantee the same number of trials for each condition under each block, the program was set to randomize the selection based on the condition and the position and arrow orientation of the answer within it. For example, when the correct answer appeared on the left side, the program randomly selected 18 out of 36 trials. The right side was drawn in the same way, making a total of 72 (18 × 4) trials for the congruent condition. Trials in the incongruent and non-attention conditions were drawn in the same way as in the congruent condition; therefore, a total of 216 (72 × 3) trials were drawn in the three conditions under this block. The remaining trials were allocated to another block.
To avoid potential confusion regarding the relative difficulty of the two types of multiplication, we conducted a detailed pilot study by administering a questionnaire. We used a Likert scale ranging from 1 to 5 (1 = completely different, 2 = relatively different, 3 = uncertain, 4 = relatively similar, 5 = completely similar) to assess the consistency of difficulty between the two types. For example, [“Do you think 1 × 2 = 2 is as difficult as −1 × 2=-2?”] [“Do you think 8 × 9 = 72 is as difficult as −8 × 9=-72?”].
About 71 participants were recruited for the pilot study, of which 53 provided valid responses (43 for females and 10 for males) and had a mean age of 23.53 years (SD = 1.38). The one-sample t-test was performed with a criterion of 3 for scores. The results showed that the participants perceived the similarity of difficulty for the positive and negative multiplication mnemonics to be significantly greater than 3 [(M = 3.59, SD = 0.93), t(52) = 4.64, p < .001, Cohen’s d = .64]. This indicated that participants tended to perceive the difficulty of the two materials as the same. Accordingly, the effect of the material’s difficulty was controlled.
Apparatus & procedure
The experiment was completed individually in a sound-attenuated, warm, and softly lit room with each participant seated in a comfortable chair approximately 60 cm from the monitor, which was a 24" DELL U2412M with a resolution of 1920 × 1080 at 16:9. Body and head movements were minimized during the experiment by gazing at the center of the screen with both eyes.
The stimulus presentation and behavioral data collection for the dot-probe task were controlled using E-prime 2.0 software (Psychology Software Tools, Inc., Pittsburgh, PA; http://www.pstnet.com/eprime). In each trial (see ), a “●,” randomly presented for 800–1000 ms, appeared in the center of the screen, followed by the successive presentation of the first and second multipliers for 100 ms. After the second multiplier disappeared, the “●” continued to appear in the center of the screen for a random duration of 400–500 ms to allow the participants to perform calculations under time pressure. Subsequently, the product of the previous equations appeared to the left or right side of the fixation for 500 ms. Thereafter, a probe was presented for 100 ms, which was an arrow pointing up or down. On the disappearance of the probe, the participant was required to respond within 1100 ms according to the orientation of the arrows by pressing the “P” key upwards and “L” key downwards while ensuring accuracy. If the participant did not respond within 1100 ms, the response was considered incorrect, and the participant was automatically moved to the next trial. Overall, participants required approximately 12 min to complete each block, followed by a 1-min break before proceeding to the second block, resulting in a total completion time of approximately 25 min.
Results
The correct reaction times (RT) were analyzed using the R 4.1.2 (R Core Team, Citation2021) and its bruceR package (Bao, Citation2021), and the figures were plotted using ggplot2 (Wickham, Citation2016). Trials with a correct RT of less than 150 ms or more than 750 ms were removed from the analysis (Carlson & Mujica-Parodi, Citation2015; Carlson & Reinke, Citation2014). A 2 (intuition type: weaker intuition/stronger intuition) × 2 (trial type: congruency/incongruency) repeated-measures ANOVA for RT was conducted to examine whether participants exhibited an attentional bias toward the correct answer. There was a significant main effect of trial type, with significantly longer RT for the incongruent condition than for the congruent condition [F(1, 36) = 39.62, p<.001, = .52]. Additionally, a significant interaction was observed between trial type and intuition type [F(1, 36) = 6.05, p=.02,
= .14]. Simple main effects analysis (see ) revealed that participants in each condition had a significantly longer RT to the incongruent condition than the congruent condition. However, this effect was larger in the stronger intuition group [F(1, 36) = 38.31, p < .001,
= .52, 90% CI [.321, .651] for
], but was medium-sized in the weaker intuition group [F(1, 36) = 7.36, p =.01,
= .17, 90% CI [.025, .352] for
]. No significant main effect of intuitive type was observed [F(1, 36) = .18, p = .67].
Discussion
Experiment 1 explored the guidance of attentional bias by stronger (heuristic) and weaker (logical) intuitions using a dot-probe paradigm including calculations from the multiplication mnemonic as the stimuli . In both groups, participants in each condition had significantly shorter RTs in the congruent than in the incongruent condition, indicating an attentional bias towards the correct answer. At the outset, participants would develop an attentional bias toward the correct answer (the target), which was consistent with the results of previous studies on attentional bias using the dot-probe paradigm (Carlson & Mujica-Parodi, Citation2015; Carlson & Reinke, Citation2014; Koster et al., Citation2004). More importantly, the results also support Evans’ view (2010) that intuition motivates individuals to do what feels right to them. Since the participants had an intuitive understanding of multiplication, they were able to focus on identifying the correct answer (the target). Interestingly, participants in both the stronger and weaker intuition groups showed attentional bias toward the correct answer; however, the effect was smaller for the weaker intuition group than for the stronger intuition group. This suggests that participants were able to effectively utilize both weaker and stronger intuitions, enabling them to direct their attention toward the correct answer using top-down attentional guidance. This mechanism helps individuals to selectively attend to relevant stimuli in the environment and filter out the irrelevant information once the correct answer has been established in their minds.
The multiplication mnemonics employed in Experiment 1 were familiar to participants from an early age and were ingrained in their memory to the extent that it allowed them to automatically generate the correct answer based on a stronger intuition. Moreover, participants were rapidly and automatically able to produce the correct answer based on the weaker intuition even with the addition of negative rules, which is comparable to the rule-based secondary intuition, as suggested by Fischbein (Citation1987, Citation1999). This implies that both stronger and weaker intuitions are capable of correctly solving specific problems (Bago & De Neys, Citation2020; Newman et al., Citation2017), and that the automated use of logic may be the consequence of individual learning and training over time (De Neys & Pennycook, Citation2019). Further investigation may be required to determine the extent to which beliefs and logic are automated. Pennycook et al. (Citation2015) proposed that intuition is somewhat dependent on automated stimulus-response associations, and that the strength of the association determines the fluency of intuitive processing, referred to as the strength of intuition (Bago & De Neys, Citation2017a). One possibility that can be deduced from this is that the level of automation determines the strength of weaker and stronger intuitions or the speed at which their strength peaks (Bago & De Neys, Citation2017b). Following the hypothesis and results of Experiment 1, Experiment 2 included a cognitive load task with high and low levels in a dot-probe paradigm, and further explored whether cognitive load affected the guidance of attentional bias by different strength of intuitions.
Experiment 2
Hypothesis: The effect of attentional bias would be replicated under high cognitive load if it is guided by stronger intuitions, which means that the RT would be faster in the congruent than in the incongruent condition under high load.
Method
Participants
Considering the 2 × 2 × 2 mixed design in Experiment 2, the expected sample size was calculated by G-Power 3.1.9 (Faul et al., Citation2007) using f = 0.25 as an effect size with a power of 0.8 and alpha of 0.05 to detect a medium-sized effect for a within-subjects effect. The analysis revealed an expected sample size of 16. 34 postgraduates (12 males, 22 females) were recruited for this experiment. They were divided into two groups with 17 (6 males, 11 females) in the weaker intuition group and 17 (6 males, 11 females) in the stronger intuition group, falling in an age range of 21 to 27 years (M = 22.97 years, SD = 1.47 years). All participants were native Chinese speakers, healthy, right-handed, with normal or corrected vision, and no neurological disorders. The ethics committee of the author’s institution approved this experiment. Participation was voluntary. Written informed consent was obtained from the participants prior to the experiment and monetary compensation was provided at the end.
Design
The experiment was a 2 (intuition type: weaker intuition/stronger intuition) × 2 (trial type: congruency/incongruency) × 2 (cognitive load: high/low) mixed design, where intuition type was the between-group variable, and cognitive load and trial type were within-group variables.
Materials
A cognitive load task was added to the dot-probe paradigm of Experiment 1. High and low cognitive loads were formed through the memorization of randomly arranged and meaningless sequences of letters having different lengths (Naert et al., Citation2018; Qi et al., Citation2014). Under high load, participants were asked to memorize letter sequences of length 6 (e.g. DSFLIN), while participants were asked to memorize a sequence of letters of length 2 (e.g. FP) under low cognitive load. At the end of the dot-probe task, participants were asked whether the presented letter (e.g. N) had appeared in the previously presented letter sequence.
Experiment 2 contained 216 trials; the congruent, incongruent, and non-attention conditions contained 72 trials each, which were split into 2 blocks to avoid participant fatigue. Trials were presented in the same way as in Experiment 1, and the number of high- and low-load trials in each block was equivalent.
Apparatus & procedure
The experiment was completed individually in a sound-attenuated, warm, and softly lit room where each participant was seated in a comfortable chair approximately 60 cm from the monitor, which was a 24" DELL U2412M with a resolution of 1920 × 1080 at 16:9. Participants were asked to minimize their body and head movements as much as possible during the experiment by gazing at the center of the screen with both eyes.
Experiment 2 utilized a dual-task paradigm (see ). The cognitive load task required the participants to memorize a sequence of letters presented for 2500 ms and then perform the same dot detection task as described in Experiment 1. After the participants had completed the arrow orientation judgement, a letter was presented on the screen for 5000 ms. They were asked to determine if the letter had occurred in the previously presented sequence of letters by pressing “1” if they thought it did, or “2” if it did not. Theoretically, the participants took approximately 18 min to complete each block, followed by a 1-min break before the second block, resulting in a final completion time of approximately 37 min.
Results
The RTs were analyzed using the R 4.1.2 (R Core Team, Citation2021) and its bruceR package (Bao, Citation2021), and the figures were plotted using ggplot2 (Wickham, Citation2016). Trials with a correct RT of less than 150 ms or more than 750 ms were removed (Carlson & Mujica-Parodi, Citation2015; Carlson & Reinke, Citation2014). A repeated-measures ANOVA was conducted on RT, using a 2 (intuition type: weaker intuition/stronger intuition) × 2 (cognitive load: high/low) × 2 (trial type: consistent/inconsistent) design to examine whether participants showed an attentional bias toward the correct answer. The main effect of trial type was found to be significant, with a significantly longer RT in the incongruent condition than in the congruent condition [F(1, 32) = 20.13, p < .001, = .39]. The main effect of cognitive load was found to be significant, with a significantly longer RT in the low load than in the high load condition [F(1, 32) = 4.44, p = .04,
= .12]. The three-way interaction between trial type, intuition type, and cognitive load was significant [F(1, 32) = 5.55, p =.03,
= .15]. The remaining main effects and interactions were not significant [Fs(1, 32) < 1.99, ps >.16].
On further simple-simple main effects analysisFootnote2 (see ), there were significantly longer RTs for the incongruent condition than for the congruent condition in the stronger intuition group at low load [F(1, 32) = 5.88, p = .02, = .16, 90%CI [.014, .347], medium effect]. Additionally, a longer RT for the incongruent condition than the congruent condition was found at high load [F(1, 32) = 17.37, p < .001,
= .35, 90%CI [.140, .529], large effect]. However, in the weaker intuition group, responses were significantly longer for the incongruent condition than the congruent condition only at low load [F(1, 32) = 8.84, p = .006,
= .22, 90%CI [.043, .409], medium effect]; no significant difference was found between the congruent and incongruent conditions at high load [F(1, 32) = .02, p=.90].
Discussion
The results revealed that cognitive load affected performance since responses were significantly longer at low loads than at high loads, indicating that the consumption of cognitive resources increased the speed of responses to the primary task (Hockey, Citation1997). Notably, participants in the stronger (heuristic) intuition group took longer to respond to the incongruent condition than the congruent condition in both high and low loads, suggesting an attentional bias toward the correct answer. However, in the weaker (logical) intuition group, attentional bias was only observed in the low load condition. This implies that stronger intuition is less affected by cognitive load, whereas weaker intuition may be inefficient under high load. Differences in the strength of intuition may be an essential factor influencing performance (Bago & De Neys, Citation2017a, Citation2017b; Citation2020; Reyna & Rahimi-Golkhandan, Citation2017). The results of the current study support this and suggest that the strength of stronger intuition may be more powerful than weaker intuition. Heuristic intuitions are generally stronger than logical intuitions (De Neys, Citation2012). Bago and De Neys (Citation2017a) adopted a two-response paradigm, which permitted two responses for the same question. The first response was a rapid response given in a time-limited condition along with a secondary load task. The second response followed immediately after the first response, where the participants could respond in an untimed condition without the cognitive load task. There were four combinations of responses in their study: 00 (incorrect response both times), 01 (incorrect first response, correct second response), 10 (correct first response, incorrect second response), and 11 (correct response both times). For example, the proportion of “00” response was higher than the proportion of “11” response in the base-rate neglect and syllogism tasks.
While Experiment 2 did not examine stronger and weaker intuitions from the perspective of cognitive conflict, it developed materials that activated the two intuitive processes separately, in terms of their characteristics. In the present study, the difference between the strength of weaker and stronger intuitions is supported by the fact that stronger intuitions may have a higher strength or reach their peak strength faster than weaker intuitions (Bago & De Neys, Citation2017b). Furthermore, Yu et al. (Citation2018) argued that intuitions may not be entirely independent of cognitive resources; the present study supports and extends this perceptive. Weaker intuitions can achieve higher activation intensities or peak more quickly when elicited by cues because of their highly automated semantic associations and are therefore not affected by variations in cognitive load. In contrast, weaker intuitions may not be able to achieve the same degree of automation in its application as stronger intuitions, and therefore, may only function in low load. One caveat to this explanation is that low cognitive load may allow brief access to Type 2 processes. Such a possibility should be considered in future investigations that explore the boundaries between Type 1 and Type 2 processing.
General discussion
This study explored the guidance of stronger (heuristics) and weaker (logical) intuitions on attentional bias through two experiments using dot-probe and cognitive load tasks. The current study used multiplication mnemonics to elicit two different intuitions, which varies from the traditional reasoning problems used in the previous literature. In a standard reasoning study, the labels of heuristic and logical intuitions align with the incorrect and correct responses. The current study used two types of stimuli to elicit weaker and stronger intuitions to facilitate the generalizability of the logical intuition hypothesis. The crucial characteristic that distinguishes logical intuition from heuristic intuition may not be the correctness or incorrectness of the response, but rather the ability to apply logical, probabilistic, and mathematical rules in an automated manner considering the definition. In other words, it is distinguished based on the sensitivity to perceive the rules contained in the problem. Thus, the current study labeled the two materials as weaker and stronger intuitions analogous to logical and heuristic intuitions, respectively, to maintain consistency with the previous literature.
The results from Experiments 1 and 2 showed that participants had an attentional bias toward the correct answer in different experimental setups, such as a response deadline with and without a cognitive load. These results indicated that stronger and weaker intuitions worked effectively when elicited by the corresponding cues and direct attention to the process outcomes. Under time constraints, individuals tend to terminate the information search process after identifying the target (Roberts et al., Citation2022). Therefore, only stronger intuition functions under the response deadline and high-load condition. The present results not only endorse the ability of stronger and weaker intuitions to automate the use of their corresponding beliefs and logics (Bago & De Neys, Citation2017a, Citation2017b, Citation2020; Pennycook et al., Citation2015), but also provide support for the existence of differences in the strength between the two intuitions from an attentional bias perspective. It may be relatively easier for individuals to produce answers through stronger intuitions in high-load situations than through weaker intuitions. Engaging in Type 2 processing can be challenging in the presence of a secondary task. However, individuals are more likely to rely on their intuitions when their cognitive load is elevated (Bago & De Neys, Citation2017a; Citation2020), which can lead to biased attention toward the correct answer. The reason for this may be the higher strength of the intuition evoked by the heuristic cue, which is more fluent in producing answers and allows them to have stronger expectations of the upcoming stimuli based on the correct answers formed by them (Summerfield & Egner, Citation2009). However, the possibility of the involvement of brief Type 2 processing where it is permitted by one’s cognitive capacity cannot be eliminated.
Different levels of automation may be an influential reason for the different strengths of stronger and weaker intuitions. Stanovich (Citation2018) has emphasized that mindware containing beliefs and logical intuitions can be automatically activated in a task through enough repetition and practice. Moreover, intuitions rely on stimulus-response associations (Pennycook, Citation2017; Pennycook et al., Citation2015) that enhance performance on visual search tasks that contain material with the same associations (Roche & O’Mara, Citation2003). Stronger intuition materials in the current study were selected from multiplication mnemonics, which is the foundation of Chinese mathematics education, in line with the characteristics of heuristic intuition based on beliefs, knowledge, and semantic associations (De Neys, Citation2014). Since multiplication mnemonics are learned and applied repetitively over time, individuals develop stable automated S-R semantic associations (e.g. S is 3 × 5, R is 15). In contrast, although individuals can automate the application of logical rules to process numbers and negative signs as a whole, they may not be automated to the same extent as positive numbers (Shaki & Petrusic, Citation2005). Thus, although logical intuition is automatic (Raoelison et al., Citation2021), its inferior level of automation may be one reason why it is not as strong as heuristic intuition. If not fully automated, then logical intuition may entail the initiation (but not completion) of some deliberative processing.
Additionally, researchers have proposed that semantic and structural features of problems can induce knowledge-based and logic-based intuitive responses, respectively (Handley & Trippas, Citation2015; Trippas & Handley, Citation2017). The multiplication mnemonic evokes a stronger intuitive response through its semantic features, while the addition of the negative number rule gives it a structural feature that evokes a weaker intuitive response. Attention, as a fundamental cognitive mechanism, gives individuals the essential ability to selectively process environmental inputs. What an individual is trying to accomplish and the resulting expectations influence the deployment of attention, which is known as top-down goal-direction (Akyurek et al., Citation2010; Akyurek & Schubo, Citation2011; Hutchinson & Turk-Browne, Citation2012).
A possible alternative explanation for the greater proportion of “00” responses (i.e. incorrect first and second responses) than “11” responses (i.e. both correct responses) in the previous study by Bago and De Neys (Citation2017a) is also offered by the current study results. Bago and De Neys allowed two responses to the same question; the first response being a rapid response in a time-limited condition, followed by the second response in an unrestricted condition. Attention is mostly attracted to information that is consistent with stronger intuitions and rarely to information that is consistent with weaker intuitions. This interpretation may support the results from Bago and De Neys’ study (2020) that the proportion of conflict problems in which the initial response was a logical intuition were fewer than the heuristic intuition outcome, even when the strength of logical intuition was enhanced by increasing the base rate.
Utilizing the attentional bias of the dot-probe paradigm as an index for the strength of intuitions is an important methodological innovation that provides new avenues for exploring intuition-related indicators in future studies on reasoning. Additionally, this method can be extended to explore belief bias (Evans & Curtis-Holmes, Citation2005; Handley et al., Citation2011), which is similar to the stronger intuition phenomenon observed in the present study. In traditional reasoning research, belief bias is often viewed as a bias that arises from existing beliefs or knowledge. The attentional bias training has been found to modify certain specific biases (Cisler & Koster, Citation2010), such as difficulty in disengaging. Therefore, future studies could explore the potential of combining attentional bias training with belief bias effects to investigate whether such training can help individuals alleviate the impact of their existing beliefs and improve their performance.
While the results of Experiment 2 indicated that cognitive load had a significant effect on weaker intuitions, the experiment had a limitation in that it did not record the accuracy of the secondary load task. This aspect should be addressed in future studies to enrich the findings. Additionally, although the difficulty of the two types of stimuli used in the experiments is similar, the ratings from the pilot study were not equal to 4 or 5 (which would indicate a high degree of similarity). This suggests that while the participants perceived the two types of stimuli to have the same difficulty, the objective degree of difficulty may have been different. Therefore, the potential influence of the degree of difficulty should be explored in the future.
In conclusion, no impact of cognitive load on the attentional bias guided by stronger intuitions was observed, potentially because of the higher strength of stronger intuitions. Moreover, due to the high occupancy of cognitive resources, participants rarely allocated their attention to information that was consistent with the results of their weaker intuition. This study explored the guidance of attention by stronger and weaker intuitions from the perspective of attentional bias. It was found that the different activation strengths of the two intuitions may induce distinct operating patterns in the high cognitive load condition. The higher level of automation of stronger intuitions may be one reason behind its robustness when compared to weaker intuitions in terms of strength.
Ethics approval statement
This study was conducted following the recommendations of the Ethics Committee of Shanghai Normal University. The protocol was approved by the Ethics Committee of Shanghai Normal University.
Acknowledgments
We also thank all the participants in our experiments, without them this research would not be possible.
Data availability statement
The datasets generated during and/or analyzed during the current study are not publicly available due to their confidential contents that could compromise the privacy of the research participants but are available from the corresponding author on reasonable request.
Disclosure statement
No potential conflict of interest was reported by the authors.
Additional information
Funding
Notes
1 Chinese multiplication mnemonics are simple rules of multiplication operations, usually comprising a rhythmically presented equation for multiplying numbers between 1 and 9. For example, one-one gets one, one-two gets two …… eight-nine-seventy-two, nine-nine-eighty-one, etc. Chinese as a monosyllabic morphemic language makes multiplication mnemonics rhythmic when read and recited, making them easy to remember and apply.
2 Another direction of simple-simple main effects analysis was conducted. Results showed that RT at low load was significantly longer than at high load in the weaker intuition group for the incongruent condition [F(1, 32) = 7.25, p = .01, = .19, 90%CI [.027, .378]], but other comparisons were not significant [Fs(1, 32) < 2.82, ps > .10].
References
- Akyurek, E. G., Dinkelbach, A., & Schubo, A. (2010). The neural processing fate of singleton target and nontarget stimuli. Brain Research, 1307, 115–133. https://doi.org/10.1016/j.brainres.2009.10.007
- Akyurek, E. G., & Schubo, A. (2011). The allocation of attention in displays with simultaneously presented singletons. Biological Psychology, 87(2), 218–225. https://doi.org/10.1016/j.biopsycho.2011.02.022
- Bago, B., & De Neys, W. (2017a). Fast logic?: Examining the time course assumption of dual process theory. Cognition, 158, 90–109. https://doi.org/10.1016/j.cognition.2016.10.014
- Bago, B., & De Neys, W. (2017b). Rise and fall of conflicting intuitions during reasoning. In Proceedings of the 39th Annual Meeting of the Cognitive Science Society (pp. 87–92). Cognitive Science Society. https://mindmodeling.org/cogsci2017/papers/0028/index.html
- Bago, B., & De Neys, W. (2020). Advancing the specification of dual process models of higher cognition: A critical test of the hybrid model view. Thinking & Reasoning, 26(1), 1–30. https://doi.org/10.1080/13546783.2018.1552194
- Bago, B., Frey, D., Vidal, J., Houde, O., Borst, G., & De Neys, W. (2018). Fast and slow thinking: Electrophysiological evidence for early conflict sensitivity. Neuropsychologia, 117, 483–490. https://doi.org/10.1016/j.neuropsychologia.2018.07.017
- Bao, H. W. S. (2021). bruceR: Broadly useful convenient and efficient R functions. R package version 0.8.2. https://CRAN.R-project.org/package=bruceR
- Betsch, T. (2008). The nature of intuition and its neglect in research on judgment and decision making. In: H. Plessner, C. Betsch & T. Betsch (Eds.), Intuition in judgment and decision making (pp. 3–22). Lawrence Erlbaum Associates Inc.
- Boissin, E., Caparos, S., Raoelison, M., & De Neys, W. (2021). From bias to sound intuiting: Boosting correct intuitive reasoning. Cognition, 211, 104645. https://doi.org/10.1016/j.cognition.2021.104645
- Carlson, J. M., & Mujica-Parodi, L. R. (2015). Facilitated attentional orienting and delayed disengagement to conscious and nonconscious fearful faces. Journal of Nonverbal Behavior, 39(1), 69–77. https://doi.org/10.1007/s10919-014-0185-1
- Carlson, J. M., & Reinke, K. S. (2014). Attending to the fear in your eyes: Facilitated orienting and delayed disengagement. Cognition and Emotion, 28(8), 1398–1406. https://doi.org/10.1080/02699931.2014.885410
- Cisler, J. M., & Koster, E. H. W. (2010). Mechanisms of attentional biases towards threat in anxiety disorders: An integrative review. Clinical Psychology Review, 30(2), 203–216. https://doi.org/10.1016/j.cpr.2009.11.003
- de Chantal, P. L., Newman, I. R., Thompson, V., & Markovits, H. (2020). Who resists belief-biased inferences? The role of individual differences in reasoning strategies, working memory, and attentional focus. Memory & Cognition, 48(4), 655–671. https://doi.org/10.3758/s13421-019-00998-2
- De Neys, W. (2012). Bias and conflict: A case for logical intuitions. Perspectives on Psychological Science : A Journal of the Association for Psychological Science, 7(1), 28–38. https://doi.org/10.1177/1745691611429354
- De Neys, W. (2014). Conflict detection, dual processes, and logical intuitions: Some clarifications. Thinking & Reasoning, 20(2), 169–187. https://doi.org/10.1080/13546783.2013.854725
- De Neys, W. (2015). Heuristic bias and conflict detection during thinking. In: B.H. Ross (Ed.), Psychology of learning and motivation (Vol. 62, pp. 1–32). Academic Press. https://doi.org/10.1016/bs.plm.2014.09.001
- De Neys, W., & Pennycook, G. (2019). Logic, fast and slow: Advances in dual-process theorizing. Current Directions in Psychological Science, 28(5), 503–509. https://doi.org/10.1177/0963721419855658
- Evans, J. S. B. T. (2003). In two minds: Dual-process accounts of reasoning. Trends in Cognitive Sciences, 7(10), 454–459. https://doi.org/10.1016/j.tics.2003.08.012
- Evans, J. S. B. T. (2010). Intuition and reasoning: A dual-process perspective. Psychological Inquiry, 21(4), 313–326. https://doi.org/10.1080/1047840X.2010.521057
- Evans, J, S. B. T., Ball, L. J., & Brooks, P. G. (1987). Attentional bias and decision order in a reasoning task. British Journal of Psychology, 78(3), 385–394. https://doi.org/10.1111/j.2044-8295.1987.tb02256.x
- Evans, J. S. B. T., & Curtis-Holmes, J. (2005). Rapid responding increases belief bias: Evidence for the dual-process theory of reasoning. Thinking & Reasoning, 11(4), 382–389. https://doi.org/10.1080/13546780542000005
- Evans, J. S. B. T., & Stanovich, K. E. (2013a). Dual-process theories of higher cognition: Advancing the debate. Perspectives on Psychological Science: A Journal of the Association for Psychological Science, 8(3), 223–241. https://doi.org/10.1177/1745691612460685
- Evans, J. S. B. T., & Stanovich, K. E. (2013b). Theory and metatheory in the study of dual processing: Reply to comments. Perspectives on Psychological Science: A Journal of the Association for Psychological Science, 8(3), 263–271. https://doi.org/10.1177/1745691613483774
- Faul, F., Erdfelder, E., Lang, A.-G., & Buchner, A. (2007). G*Power 3: A flexible statistical power analysis program for the social, behavioral, and biomedical sciences. Behavior Research Methods, 39(2), 175–191. https://doi.org/10.3758/BF03193146
- Fischbein, E. (1987). Intuition in science and mathematics: An educational approach. D. Reidel Publishing Company.
- Fischbein, E. (1999). Intuitions and schemata in mathematical reasoning. Educational Studies in Mathematics, 38, 11–50. https://doi.org/10.1023/A:1003488222875
- Gladwin, T. E., & Vink, M. (2018). Alcohol-related attentional bias variability and conflicting automatic associations. Journal of Experimental Psychopathology, 9(2), 204380871877963. https://doi.org/10.5127/jep.062317
- Handley, S. J., Newstead, S. E., & Trippas, D. (2011). Logic, beliefs, and instruction: A test of the default interventionist account of belief bias. Journal of Experimental Psychology: Learning, Memory, and Cognition, 37(1), 28–43. https://doi.org/10.1037/a0021098
- Handley, S. J., & Trippas, D. (2015). Dual processes and the interplay between knowledge and structure: A new parallel processing model. In: B.H. Ross (Ed.), Psychology of learning and motivation (Vol. 62, pp. 33–58). Academic Press. https://doi.org/10.1016/bs.plm.2014.09.002
- Hockey, G. R. J. (1997). Compensatory control in the regulation of human performance under stress and high workload: A cognitive-energetical framework. Biological Psychology, 45(1–3), 73–93. https://doi.org/10.1016/S0301-0511(96)05223-4
- Hutchinson, J. B., & Turk-Browne, N. B. (2012). Memory-guided attention: Control from multiple memory systems. Trends in Cognitive Sciences, 16(12), 576–579. https://doi.org/10.1016/j.tics.2012.10.003
- Koster, E. H., Crombez, G., Verschuere, B., & De Houwer, J. (2004). Selective attention to threat in the dot probe paradigm: Differentiating vigilance and difficulty to disengage. Behaviour Research and Therapy, 42(10), 1183–1192. https://doi.org/10.1016/j.brat.2003.08.001
- Markovits, H., de Chantal, P. L., Brisson, J., Dube, E., Thompson, V., & Newman, I. (2021). Reasoning strategies predict use of very fast logical reasoning. Memory & Cognition, 49(3), 532–543. https://doi.org/10.3758/s13421-020-01108-3
- Markovits, H., de Chantal, P. L., Brisson, J., & Gagnon-St-Pierre, E. (2019). The development of fast and slow inferential responding: Evidence for a parallel development of rule-based and belief-based intuitions. Memory & Cognition, 47(6), 1188–1200. https://doi.org/10.3758/s13421-019-00927-3
- Morsanyi, K., Busdraghi, C., & Primi, C. (2014). Mathematical anxiety is linked to reduced cognitive reflection: A potential road from discomfort in the mathematics classroom to susceptibility to biases. Behavioral and Brain Functions : BBF, 10(1), 31. 13. https://doi.org/10.1186/1744-9081-10-31
- Morsanyi, K., Devine, A., Nobes, A., & Szűcs, D. (2013). The link between logic, mathematics and imagination: Evidence from children with developmental dyscalculia and mathematically gifted children. Developmental Science, 16(4), 542–553. https://doi.org/10.1111/desc.12048
- Morsanyi, K., Kahl, T., & Rooney, R. (2017). The link between math and logic in adolescence: The effect of argument form. In M. E. Toplak & J. Weller (Eds.), Individual differences in judgment and decision making from a developmental context (pp. 166–185). Psychology Press.
- Morsanyi, K., McCormack, T., & O’Mahony, E. (2018). The link between deductive reasoning and mathematics. Thinking & Reasoning, 24(2), 234–257. https://doi.org/10.1080/13546783.2017.1384760
- Naert, L., Bonato, M., & Fias, W. (2018). Asymmetric spatial processing under cognitive load. Frontiers in Psychology, 9, 583. https://doi.org/10.3389/fpsyg.2018.00583
- Newman, I. R., Gibb, M., & Thompson, V. A. (2017). Rule-based reasoning is fast and belief-based reasoning can be slow: Challenging current explanations of belief-bias and base-rate neglect. Journal of Experimental Psychology: Learning, Memory, and Cognition, 43(7), 1154–1170. https://doi.org/10.1037/xlm0000372.supp
- Pennycook, G. (2017). A perspective on the theoretical foundation of dual process models. In W. De Neys (Ed.), Dual process theory 2.0 (pp. 47–65). Routledge.
- Pennycook, G., De Neys, W., Evans, J., Stanovich, K. E., & Thompson, V. A. (2018). The mythical dual-process typology. Trends in Cognitive Sciences, 22(8), 667–668. https://doi.org/10.1016/j.tics.2018.04.008
- Pennycook, G., Fugelsang, J. A., & Koehler, D. J. (2015). What makes us think? A three-stage dual-process model of analytic engagement. Cognitive Psychology, 80, 34–72. https://doi.org/10.1016/j.cogpsych.2015.05.001
- Pennycook, G., & Thompson, V. A. (2012). Reasoning with base rates is routine, relatively effortless, and context dependent. Psychonomic Bulletin & Review, 19(3), 528–534. https://doi.org/10.3758/s13423-012-0249-3
- Qi, S., Zeng, Q., Luo, Y., Duan, H., Ding, C., Hu, W., & Li, H. (2014). Impact of working memory load on cognitive control in trait anxiety: An ERP study. Plos One, 9(11), e111791. https://doi.org/10.1371/journal.pone.0111791
- R Core Team. (2021). R: A language and environment for statistical computing. R Foundation for Statistical Computing. https://www.R-project.org/
- Raoelison, M., Boissin, E., Borst, G., & De Neys, W. (2021). From slow to fast logic: The development of logical intuitions. Thinking & Reasoning, 27(4), 599–622. https://doi.org/10.1080/13546783.2021.1885488
- Raoelison, M., Thompson, V. A., & De Neys, W. (2020). The smart intuitor: Cognitive capacity predicts intuitive rather than deliberate thinking. Cognition, 204, 104381. https://doi.org/10.1016/j.cognition.2020.104381
- Reyna, V. F., & Rahimi-Golkhandan, S. (2017). The fuzzy-trace dual process model. In W. De Neys (Ed.), Dual process theory 2.0 (pp. 47–65). Routledge.
- Roberts, I. D., Teoh, Y. Y., & Hutcherson, C. A. (2022). Time to pay attention? Information search explains amplified framing effects under time pressure. Psychological Science, 33(1), 90–104. https://doi.org/10.1177/09567976211026983
- Roche, R. A. P., & O’Mara, S. M. (2003). Behavioural and electrophysiological correlates of visuomotor learning during a visual search task. Brain Research. Cognitive Brain Research, 15(2), 127–136. https://doi.org/10.1016/S0926-6410(02)00146-5
- Shaki, S., & Petrusic, W. M. (2005). On the mental representation of negative numbers: Context-dependent SNARC effects with comparative judgments. Psychonomic Bulletin & Review, 12(5), 931–937. https://doi.org/10.3758/BF03196788
- Sloman, S. A. (1996). The empirical case for two systems of reasoning. Psychological Bulletin, 119(1), 3–22. https://doi.org/10.1037/0033-2909.119.1.3
- Stanovich, K. E. (2018). Miserliness in human cognition: The interaction of detection, override and mindware. Thinking & Reasoning, 24(4), 423–444. https://doi.org/10.1080/13546783.2018.1459314
- Stupple, E. J., Ball, L. J., & Ellis, D. (2013). Matching bias in syllogistic reasoning: Evidence for a dual-process account from response times and confidence ratings. Thinking & Reasoning, 19(1), 54–77. https://doi.org/10.1080/13546783.2012.735622
- Summerfield, C., & Egner, T. (2009). Expectation (and attention) in visual cognition. Trends in Cognitive Sciences, 13(9), 403–409. https://doi.org/10.1016/j.tics.2009.06.003
- Teoh, Y. Y., Yao, Z., Cunningham, W. A., & Hutcherson, C. A. (2020). Attentional priorities drive effects of time pressure on altruistic choice. Nature Communications, 11(1), 3534. https://doi.org/10.1038/s41467-020-17326-x
- Torrence, R. D., & Troup, L. J. (2018). Event-related potentials of attentional bias toward faces in the dot-probe task: A systematic review. Psychophysiology, 55(6), e13051. https://doi.org/10.1111/psyp.13051
- Trippas, D., & Handley, S. J. (2017). The parallel processing model of belief bias: Review and extensions. In W. De Neys (Ed.), Dual process theory 2.0 (pp. 47–65). Routledge.
- Van Hoof, J., Verschaffel, L., De Neys, W., & Van Dooren, W. (2020). Intuitive errors in learners’ fraction understanding: A dual-process perspective on the natural number bias. Memory & Cognition, 48(7), 1171–1180. https://doi.org/10.3758/s13421-020-01045-1
- Wickham, H. (2016). ggplot2: Elegant graphics for data analysis. Springer-Verlag. https://ggplot2.tidyverse.org
- Williams, C. C., Kappen, M., Hassall, C. D., Wright, B. C., & Krigolson, O. E. (2019). Thinking theta and alpha: Mechanisms of intuitive and analytical reasoning. NeuroImage, 189, 574–580. https://doi.org/10.1016/j.neuroimage.2019.01.048
- Yin, Y., Yu, T., Wang, S., Zhou, S., Tang, X., Stupple, E. J. N., & Luo, J. (2018). Event-related potentials support a dual process account of the Embedded Chinese Character Task. Neuropsychologia, 121, 186–192. https://doi.org/10.1016/j.neuropsychologia.2018.10.021
- Yu, T., Yin, Y., Wang, S., Zhou, S., Tang, X., & Luo, J. (2018). Positive effect of intuitive processing is modulated by cognitive resources under different levels of consciousness. Acta Psychologica Sinica, 50(6), 583–591. https://doi.org/10.3724/SP.J.1041.2018.00583
- Zhou, X., Chen, C., Zhang, H., Chen, C., Zhou, R., & Dong, Q. (2007). The operand-order effect in single-digit multiplication: An ERP study of Chinese adults. Neuroscience Letters, 414(1), 41–44. https://doi.org/10.1016/j.neulet.2006.06.078