Abstract
Introduction. Dreams might represent a window on altered states of consciousness with relevance to psychotic experiences, where reality monitoring is impaired. We examined reality monitoring in healthy, non-psychotic individuals with varying degrees of dream awareness using a task designed to assess confabulatory memory errors – a confusion regarding reality whereby information from the past feels falsely familiar and does not constrain current perception appropriately. Confabulatory errors are common following damage to the ventromedial prefrontal cortex (vmPFC). Ventromedial function has previously been implicated in dreaming and dream awareness.
Methods. In a hospital research setting, physically and mentally healthy individuals with high (n = 18) and low (n = 13) self-reported dream awareness completed a computerised cognitive task that involved reality monitoring based on familiarity across a series of task runs.
Results. Signal detection theory analysis revealed a more liberal acceptance bias in those with high dream awareness, consistent with the notion of overlap in the perception of dreams, imagination and reality.
Conclusions. We discuss the implications of these results for models of reality monitoring and psychosis with a particular focus on the role of vmPFC in default-mode brain function, model-based reinforcement learning and the phenomenology of dreaming and waking consciousness.
Introduction
The faculty by which we are subject to illusion when affected by disease is identical with that which produces illusory effects in sleep. (Aristotle, trans. Citation1908)
Psychotic symptoms like hallucinations and delusions (Simons, Henson, Gilbert, & Fletcher, Citation2008) as well as spontaneous confabulation (Nahum, Ptak, Leemann, & Schnider, Citation2009; Pihan, Gutbrod, Baas, & Schnider, Citation2004; Schnider, Citation2001, Citation2003; Schnider, Bonvallat, Emond, & Leemann, Citation2005) are all associated with disruptions in reality monitoring. Although spontaneous confabulation is a consequence of neurological damage, confabulation more generally refers to false or erroneous memories that may occur in persons with or without clear neurological injury. These memories may be either false in themselves or “real” memories jumbled in temporal context and retrieved inappropriately (Kopelman, Citation2010). Korsakoff (Citation1955) described neurological patients who confused “old recollections with present impressions”.
Case example (from Schnider et al., Citation2005, Neurology): A 63-year-old woman, a psychiatrist until 15 years previously, experienced haemorrhage from an anterior communicating artery aneurysm. Computed tomography at 4 months revealed destruction of the right orbitofrontal cortex (OFC) and basal forebrain bilaterally. At 10 weeks, she was extremely amnesic but unaware of it. She thought she was 50 years old and hospitalized because of a ruptured vessel in her leg (operated on 15 years previously) or that she was a medical staff member. She recounted visits from her mother, who had died 13 years previously (Schnider et al., Citation2005).
Spontaneous confabulation is commonly associated with focal neurological damage to OFC (particularly medial regions) (Nahum, Bouzerda-Wahlen, Guggisberg, Ptak, & Schnider, Citation2012). Furthermore, confabulation is associated with strokes of the anterior communicating artery that disconnect the OFC from the striatum (Schnider, Citation2001). Schnider argues that spontaneous confabulation involves a failure of reality monitoring resulting from malfunctioning of a very rapidly acting (200–300 ms) filter located in OFC that brings to bear memories of prior experiences on the current prevailing context (Schnider, Citation2001). Without this mechanism, patients become trapped in an inappropriate time place as mentioned earlier, habitually responding as if current perceptual inputs do not modulate the reality they are experiencing (Schnider, Citation2001).
The reality monitoring process is dopaminergically mediated (Schnider, Guggisberg, Nahum, Gabriel, & Morand, Citation2010) and may involve prediction error signalling – the mismatches between expectation and experience (Rescorla & Wagner, Citation1972) that are signalled by dopamine neurons in the midbrain, as well as the striatum and OFC, amongst other regions (Schultz & Dickinson, Citation2000). These signals provide a computational motif for learning the reward structure of the environment (Dickinson, Citation2001). Phasic bursts of dopamine activity accompany unanticipated rewards (the first taste of chocolate ice cream); once those rewards are learnt, the activation shifts back in time to accompany recognition of a stimulus which predicts reward (opening the fridge) and decreases if the reward does not arrive (the fridge is empty) (Schultz & Dickinson, Citation2000).
Reality monitoring is the ability to determine for any representation whether it is consistent with an available model of a domain (Johnson, Citation2006). Thus, predictive learning and reality monitoring are interdependent (Grossberg, Citation2000). An internal model of the reward structure of the world is to some extent a model of the organism's reality (Grossberg, Citation2000). When expected rewards do not arrive or unpredicted rewards occur the model needs to be updated (Corlett, Taylor, Wang, Fletcher, & Krystal, Citation2010). Equally, models of the world need to be consulted to determine a response (Takahashi et al., Citation2013). Since the OFC and striatum are involved in prediction error signalling, their disconnection is deleterious for reality monitoring and manifest as spontaneous confabulation (Schnider, Citation2001).
Aberrant reality monitoring (Simons, Davis, Gilbert, Frith, & Burgess, Citation2006; Simons et al. Citation2008) and aberrant prediction error have been evoked to explain psychotic symptoms (Corlett et al., Citation2010). In this work, we explore how they pertain to lucid dreaming, which is a potential model of psychosis.
Recent empirical work suggests that OFC function may be related to the capacity for lucid dreaming. In particular, persons with a higher propensity to have lucid dreams were found to be particularly competent at performing a task that engages the OFC, the Iowa Gambling Task (Neider, Pace-Schott, Forselius, Pittman, & Morgan, Citation2011). This task involves reinforcement-based decision-making and responding to variations in reinforcement contingency, valence and magnitude. These data provided a preliminary indication that OFC may be likewise implicated in dream awareness. However, the relationship between OFC function, gambling behaviour and lucid dreaming required clarification. In the present study we measured the reality monitoring of neurologically healthy subjects who reported high and low levels of dream awareness. Specifically, we examined their susceptibility to memory confabulations with a task that engages OFC but has more face and construct validity for lucid dreaming. Given the role of OFC in reality monitoring and its disruption in spontaneous confabulation, we predicted an effect of dream awareness on reality monitoring errors.
However, the direction of this effect was less easy to predict. Two considerations suggest that heightened dream awareness might be associated with fewer monitoring errors. First, dream lucidity – the experience of meta-awareness during sleep – may appear to be a heightened state of reality monitoring and could be associated with greater reality monitoring during wakefulness. Second, persons with high dream awareness performed better on the Iowa Gambling Task, perhaps indicating a propensity for fewer monitoring errors.
However, dreaming and wakefulness are distinct states, so the phenomenon that promotes reality monitoring during dreaming may be expressed differently during wakefulness. Supporting this possibility is the association between dream lucidity and a personality characteristic called “thin boundaries”. (Galvin, Citation1990). Persons with “thin” versus “thick” boundaries are characterised by a tendency to confuse (or experience an overlap in the perception of) reality and fantasy. During wakefulness, this overlap admits some fantasy into the perceived realm of reality. During sleep, it is possible that this overlap may similarly allow a heightened sense of reality, or lucidity, to permeate the more fantastical dream state. Because of this association, and the tendency for persons with higher dream awareness to report lucid dreams, we hypothesised that greater dream awareness could be associated with more reality monitoring errors.
We used signal detection theory to adjudicate between these two possibilities (Green & Swets, Citation1966). According to signal detection theory (Green & Swets, Citation1966), people's decisions are based on a number of parameters, including their sensitivity to real differences in familiarity and their bias (their propensity to give a particular response). In the present context (a recognition memory task), d-prime is a measure of sensitivity to familiarity. If lucid dreamers have better OFC functions and are more sensitive to familiarity in their recognition memory, we would expect d-prime to be higher in lucid than in non-lucid dreamers (see ). On the other hand, criterion (see ) is an estimate for the threshold subjects use to decide whether to respond that an item is a repeat (Green & Swets, Citation1966). If dream lucidity is associated with an overlap in the perception of reality with imagination or imagination, people with high lucidity should have a more negative criterion (a bias towards liberal acceptance – see ).
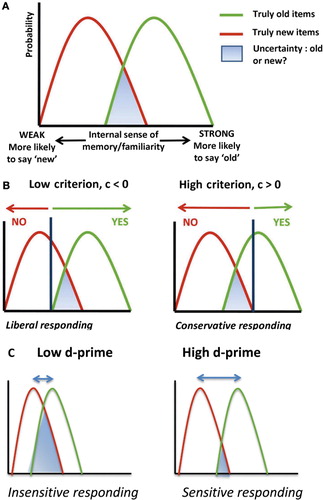
Signal detection theory (Green & Swets, Citation1966) has also been applied to explain behaviour during familiarity-based memory tasks (Banks, Citation1970), reinforcement learning (Dayan & Daw, Citation2008) and associative learning (Allan et al., Citation2007; Allan, Siegel, & Tangen, Citation2004; Allan, Siegel, & Tangen, Citation2005), but what does it have to do with prediction error?
In prior work sensory physiologists have related hierarchical predictive coding models of perception [in which top-down priors encounter bottom-up evidence – and any prediction errors update future priors (Friston, Citation2009)] to signal detection theory by considering the precision of prediction error (Hesselmann, Sadaghiani, Friston, Kleinschmidt, & Lauwereyns, Citation2010) [held to be mediated by dopamine signalling and to drive the degree to which a given prediction error updates future belief (Adams, Stephan, Brown, Frith, & Friston, Citation2013)]. Precision of prediction error is signalled in the period prior to an event and, when higher, it can encourage false alarm responses (Hesselmann et al., Citation2010). If a sensory or cognitive system has aberrant prediction errors, it will send a false alarm to irrelevant stimuli (Miller, Citation1976). In our case, we are asking subjects to judge the familiarity of a stimulus in a recognition memory task. We suspect subjects do this by making a prediction about upcoming stimulus familiarity and comparing that prediction with their experience of the stimulus (Whittlesea, Masson, & Hughes, Citation2005). The surprising familiarity of a stimulus from a prior run makes subjects false alarm. Hence biased responding rather than sensitivity.
One example of how this might arise would be hyperactivity in OFC, driving spurious predictions in dopamine cells in the midbrain, predictions that might lead expectation, imagination and experience to be confused – this is one possibility for what might be happening in lucid dreamers (Gerrans, Citation2014).
In summary, we employed signal detection theory analysis of behavioural performance on a recognition memory task on which false familiarity may drive responding to examine the neuropsychological differences between people who report a high awareness of their dreams when dreaming and those who do not.
Method
Subjects
In all, 57 healthy subjects (22 males, aged 29.59 ± 9.21 years) were recruited via local advertisement. They responded to a telephone questionnaire interview regarding their dream awareness. This baseline lucidity assessment (Neider et al., Citation2011) (BLA, indicating high dream awareness and/or lucid dream experiences) was used to assay subjects’ level of dream awareness. It consists of five statements regarding memory for dreams, dream consciousness and control. Endorsement of each statement was rated on 5-point Likert scale, anchored at 1: Strongly Agree and 5: Strongly Disagree. Hence, lower scores were associated with higher dream awareness (range of possible scores 5–25). All subjects gave written informed consent prior to participation.
Memory selection task
The memory selection task measures the ability to distinguish between memories that relate to ongoing reality and those that do not (Schnider et al., Citation2010). It was presented on a PC running Windows 7 and E-Prime 2.0 software (Psychology Software Tools Inc, Sharpsburg, PA). The task had a single practice run followed by 12 experimental runs of 27 trials of a continuous recognition test composed of a series of abstract line drawings (total 324 trials). Pictures were presented on a white background on a computer screen for 500 ms; interstimulus interval was 2000 ms. The subject had to respond using a two-button forced-choice response on a keypad to indicate whether a stimulus on a particular trial is a repeat of a stimulus seen previously in that task run. The only difference between the runs is that pictures are presented in a different order. Across runs, participants again have to indicate picture recurrences within the run and disregard familiarity with items from the previous run. Thus, all experimental runs require the ability to distinguish between items’ previous occurrence in the currently ongoing rather than the previous runs. Monitoring errors involve responding to a picture that was not repeated in that run, but that had been presented in prior runs of the task (including the practice run). Subjects also completed a series of further neuropsychological tests to be reported elsewhere.
Planned analyses
Based on prior work with the BLA scale (Neider et al., 2010), we designated those with high dream awareness as scoring less than or equal to 12 and those with low dream awareness as scoring greater than or equal to 18 on the scale. This gave us two groups of subjects: high dream awareness (n = 18) and low dream awareness (n = 13). In studies of reality monitoring memory errors, two error types form the focus: first, claims that new items in that run are repetitions because they were experienced in a previous run (false alarms) and second, claims that old items in a run are actually new (misses; see ). We conducted a signal detection theory analysis that incorporated further responses types (Hits and Correct Rejections – thus making use of all of the behavioural data we gathered). We computed the rate of hits (correctly identifying a repeat), false alarms (incorrectly claiming that a novel item was a repeat), correct rejections (correctly claiming an item was not a repeat) and incorrect rejections (incorrectly claiming that) for each subject averaged across the 12 task runs (see ).
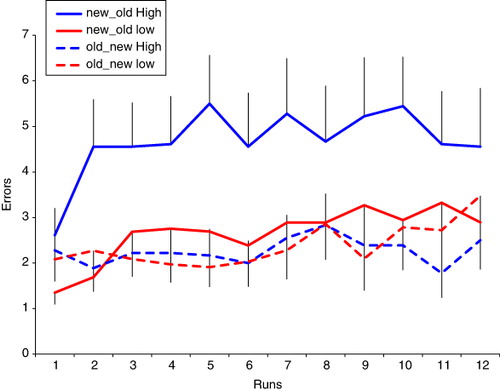
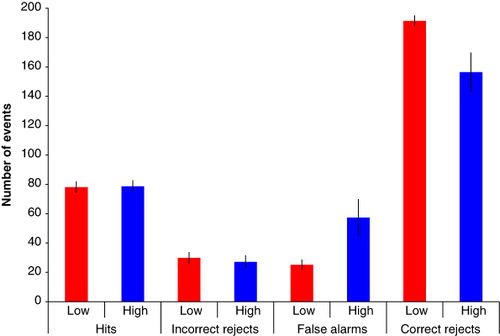
Using these data we computed d-prime and criterion d-prime was calculated as the difference between the normalised hit rate (correctly endorsing a drawing that was presented previously in that run) and the normalised false alarm rate (incorrectly endorsing a drawing that was presented in a previous run but not a repeat in that run). The more sensitive the participant is at discriminating between repeats and lures, the larger the d-prime value will be (Fox, Citation2004; MacMillan & Creelman, Citation1991; Shapiro, Citation1994).
We calculated criterion by multiplying the sum of the normalised scores of the hit rates and the false alarm rates by −0.5 (Fox, Citation2004; MacMillan & Creelman, Citation1991; Shapiro, Citation1994). When the false alarm rate for lures equals the miss rate for repeats, a participant is just as likely to say “yes” as to say “no” in making judgements (Fox, Citation2004; MacMillan & Creelman, Citation1991; Shapiro, Citation1994). When this happens, the value for c is zero. The criterion is then considered unbiased (Fox, Citation2004; MacMillan & Creelman, Citation1991; Shapiro, Citation1994). When the false alarm rate is greater than the miss rate, the bias is towards answering “yes”, indicating a liberal criterion bias with a negative value (Fox, Citation2004; MacMillan & Creelman, Citation1991; Shapiro, Citation1994). When the miss rate is greater than the false alarm rate, the bias is towards answering “no”, indicating a conservative criterion bias with a positive value (Fox, Citation2004; MacMillan & Creelman, Citation1991; Shapiro, Citation1994; see ).
We compared d-prime and criterion in the high and low dream awareness individuals using independent samples t-tests using in SPSS 19.0 for Mac (IBM).
Results
There was no significant difference in d-prime between the groups [t = 1.53, degrees of freedom (d.f.) = 29, p = .137].
The two groups did differ in their response criteria (t = 2.656, d.f. = 29, p = .013). The criterion was more negative in the high dream awareness group – indicating more liberal acceptance bias – they were more likely to indicate that a picture was familiar to them, even if it was novel.
Discussion
We find that individuals with high dream awareness make a pattern of memory errors consistent with an impairment in a reality monitoring process involving the function of the OFC (Nahum et al., Citation2009; Schnider, Citation2001, Citation2003; Schnider et al., Citation2005).
This pattern of errors was previously reported in patients with neurological damage to the OFC and its connections who spontaneously confabulate – that is, they let old memories override or govern current perceptual inputs and they allow memory fragments to intrude upon their current conceptual understanding of the world, generating a set of beliefs about themselves that is bizarre and insensitive to change (Nahum et al., Citation2009; Schnider, Citation2001, Citation2003; Schnider et al., Citation2005).
Such neurological confabulation is a model for the neural understanding of psychotic symptoms, particularly delusional beliefs – the fixed false beliefs that characterise schizophrenia (Turner & Coltheart, Citation2010). We believe this is the first empirical study to examine the relationship between confabulation, dreams and delusions that has been theorised (Pace-Schott, Citation2013).
Dreams involve creating a story – weaving a coherent narrative from the elements that happen to be present in consciousness, generating the experience of immersion in an alternate reality (Pace-Schott, Citation2013). The same has been said of delusions (Currie, Citation2000; Gerrans, Citation2012, Citation2013; Sass, Citation1994; Whitfield-Gabrieli & Ford, Citation2012) that they represent an alternate world in which patients are immersed and for which the rules of belief formation, updating and coherence do not apply (Currie, Citation2000; Gerrans, Citation2012, Citation2013; Sass, Citation1994).
Intriguingly, delusions, dreams and confabulation have all been considered to represent the unconstrained engagement of the brain's default mode (Pace-Schott, Citation2013). The default mode has been identified by examining cognitive subtraction – that is, by studies that involve task periods and rest or control periods in which less mental engagement is required (Anticevic et al., Citation2012). Task-positive regions are identified by looking at the contrast of task and baseline. However, the default-mode circuitry – incorporating the ventromedial prefrontal cortex (vmPFC), and posterior cingulate cortex, amongst other regions – is more engaged at rest than during a demanding task (Raichle et al., Citation2001) and is consistent across tasks (Raichle & Snyder, Citation2007). This pattern of engagement has been related to self-reflected processing, particularly the use of autobiographical memory to make predictions about the future (Spreng & Grady, Citation2010; Spreng, Mar, & Kim, Citation2009; Spreng, Stevens, Chamberlain, Gilmore, & Schacter, Citation2010). The inappropriate engagement of this simulation process might generate false familiarity for novel items (Corlett et al., Citation2009), leading to the pattern of errors we report presently. False familiarity signals have also been invoked to explain Déjà vu and Déjà vecu experiences, which bear phenomenological similarity to lucid dreams – people report the uncanny (and surprising) experience of having had an experience before in their past (O'Connor & Moulin, Citation2010). This false familiarity is believed to emanate from fronto-hippocampal dys-interaction (O'Connor & Moulin, Citation2010). These models of comparable phenomena perhaps point to the generality of predictive learning mechanisms in the brain (Friston, Citation2009) and the consequences of disrupted predictive learning across brain systems (Corlett et al., Citation2010).
Prior work has related delusion severity to the inappropriate engagement of the default-mode circuitry, perhaps as a result of its unconstrained operation in the absence of control from task-positive regions like Dorsolateral Prefrontal Cortex (DLPFC) (Whitfield-Gabrieli & Ford, Citation2012). Default thinking represents a means for processing oneself, reflecting on the past and projecting into the future, simulating novel, potential associations with self (Gerrans, Citation2013). The DLPFC signals an explanatory gap – or prediction error (Corlett et al., Citation2010) – and the OFC and other default regions generate a narrative to explain that gap (Carhart-Harris & Friston, Citation2010; Devinsky, Citation2009; Gerrans, Citation2013).
This notion of OFC function has much in common with preclinical rodent work on model-based reinforcement learning (McDannald, Lucantonio, Burke, Niv, & Schoenbaum, Citation2011; McDannald et al., Citation2012; McDannald, Lucantonio, Burke, Niv, & Schoenbaum, Citation2011) in which OFC function is involved in the simulation of expectations based on action–outcome associations and learned stimulus values. These expectations are communicated to structures like the striatum and midbrain to synchronise motivation with action in service of goal-directed behaviour (McDannald, Lucantonio, et al., Citation2011). Disruptions to these functions have been implicated in various mental illnesses (Lee, Citation2013) including delusions in schizophrenia (Whitfield-Gabrieli & Ford, Citation2012). Delusions, confabulation and dreaming involve the release from usual constraint a tendency to create stories that organise past, present and future reality (Pace-Schott, Citation2013). Dreaming then is a naturally occurring confabulation in which imagined events are believed and vividly experienced (Pace-Schott, Citation2013).
Taking these observations together with our prior work on individuals with high baseline lucidity – who were particularly adept with the Iowa Gambling Task (Neider et al., Citation2011), but who made confabulatory errors on the present task – there is an apparent disconnect: enhanced and impaired OFC function in the same population (Pace-Schott, Citation2013). We believe we can reconcile these observations. We propose that confabulatory errors and gambling performance are driven by a similar process – that of mental simulation (Lee, Citation2013). In the case of the gambling task, subjects simulate and use a probability space for the task that is sculpted by feedback and their own visceral responses to the task (Gerrans, Citation2007; Gerrans & Kennett, Citation2010). Without OFC, subjects suffer from a myopia for the future and do not construct an internal model of their world based on their experiences (Gerrans, Citation2007; Gerrans & Kennett, Citation2010). With increased OFC function (and perhaps elevated tonic dopamine, discussed later), they have hyperopia for the future. This helps Iowa Gambling Task performance. However, in the memory task, being too facile with mental simulation is actually deleterious, it encourages one to believe in the model and confuse it with reality, engaging a false familiarity for memoranda for previous task runs, such that they are erroneously taken to be repeats of trials within that run. Future work will explore the possibility that simulation underlies both competent and erroneous performance on different tasks.
Neurobiologically, Schnider et al. (Citation2010) have shown that dopamine modulates reality monitoring. They gave L-dopa, a drug that increases synaptic dopamine release (since it is the precursor for presynaptic dopamine production). L-dopa administration induces a similar increase in confabulatory memory errors as observed presently (Schnider et al., Citation2010). L-dopa also modulates prediction error brain signals during reward learning (Pessiglione, Seymour, Flandin, Dolan, & Frith, Citation2006). The L-dopa effects on the present study point to the tension between two modes of dopamine signalling – positive prediction errors mediated by phasic dopamine release and negative prediction errors mediated by a pause in dopamine firing, causing a dip below baseline in the firing of dopamine cells (Frank, Seeberger, & O'Reilly, Citation2004; Schultz, Dayan, & Montague, Citation1997; Schultz & Dickinson, Citation2000; Waltz, Frank, Robinson, & Gold, Citation2007). It is important to note that midbrain dopamine cells have at least two firing modes (Grace, Citation1991); phasic firing is rapidly cleared from the synaptic cleft and may mediate prediction errors. On the other hand, there is a slower tonic mode of firing that may be related to motivated behaviour. It may even mediate the sort of future-oriented simulations involved in model-based reinforcement learning (Smith, Li, Becker, & Kapur, Citation2006).
The L-dopa data of Schnider et al., Citation2010 suggest that reality monitoring requires good signal-to-noise differentiation in the dopamine system, such that pauses in firing can be resolved from the tonic baseline and phasic increases. L-dopa engenders dopamine release and alters this signal-to-noise ratio. Perhaps our opposite pattern results in lucid dreaming: facile gambling performance and poor reality monitoring, are explicable in these terms. If lucid dreamers have a sensitised phasic positive prediction error signal or elevated tonic dopamine [involved in goal-directed motivation (Smith et al., Citation2006)], they would be competent at gambling and reward learning, but their reality monitoring, dependent on negative prediction error and a pause in the firing of dopamine cells (Schnider et al., Citation2010), would be impaired. With relevance to our model-based explanation discussed earlier, the OFC seems to govern dopaminergic prediction error signalling in the ventral tegmental area dopamine cells in experimental animals by specifying top-down, model-based expectations (Takahashi et al., Citation2009). Further, patients with schizophrenia, in whom delusions are prevalent, also fail to learn from negative prediction errors (Waltz et al., Citation2007), a deficit that has also been linked to excessive engagement of the default-mode network (Waltz et al., Citation2013). Future work will explore the role of dopamine in lucid dreaming.
The observation of liberal acceptance bias in subjects with high dream awareness is consistent with source memory–based explanations for the delusional memories formed by patients with narcolepsy (Wamsley, Donjacour, Scammell, Lammers, & Stickgold, Citation2014) whose vivid dreams become confused with reality and become the basis for delusional memories [for example, having been assaulted in reality when in fact they dreamt being assaulted (Wamsley et al., Citation2014)]. The point here is that criterion, or bias, is the parameter through which our prior beliefs are manifest (Dube, Rotello, & Heit, Citation2010). If people with high dream awareness have particular preconceptions about familiarity or memory, then they would affect the criterion rather than their sensitivity. We believe our data support the idea that dream awareness involves the intrusion of reality onto the dreaming state and that this overlap is also manifest during waking, whereby high dream awareness subjects experience false familiarity for memoranda causing them to make false alarm responses.
In previous experiments using the Deese-Roediger-McDermott recognition memory task, we found that healthy individuals with delusion-like beliefs also had a more negative criterion/liberal acceptance bias (Corlett et al., Citation2009). This will form an important kernel for our future investigations of the links between learning, memory, dreams and delusions.
In conclusion we feel these data represent the first empirical link between dreaming and confabulation. Future work with deluded patients, other cognitive tasks and functional neuroimaging will allow us to test the hypothesis that all three phenomena share a neural basis – the dys-interaction between lateral frontal task-positive circuitry and the default-mode circuitry, particularly the OFC. Phenomenologically, this drives the involuntary generation of salient experiences that demand explanation by a narrative production mechanism that is not under usual inhibitory control.
Acknowledgements
The authors thank Dr. Armin Schnider for helpful comments on an earlier version of this manuscript.
Funding
This work was supported by a research award from the Mind Science Foundation, and by the Connecticut State Department of Mental Health and Addiction Services. P.R.C. was funded by an International Mental Health Research Organization (IMHRO)/Janssen Rising Star Translational Research Award and Clinical and Translational Science Award (CTSA) [grant number UL1 TR000142] from the National Center for Research Resources and the National Center for Advancing Translational Science, components of the National Institutes of Health (NIH), and NIH road map for Medical Research. Its contents are solely the responsibility of the authors and do not necessarily represent the official view of NIH.
Additional information
Funding
References
- Adams, R. A., Stephan, K. E., Brown, H. R., Frith, C. D., & Friston, K. J. (2013). The computational anatomy of psychosis. Frontiers in Psychiatry, 4, 47. doi:10.3389/fpsyt.2013.00047
- Allan, L., Siegel, S., Hannah, S., & Crump, M. (2007). Merging associative and signal-detection accounts of contingency assessment. Canadian Journal of Experimental Psychology-Revue Canadienne De Psychologie Experimentale, 61, 362–362.
- Allan, L., Siegel, S., & Tangen, J. (2004). Judgment of causality: Contingency learning or signal detection?International Journal of Psychology, 39, 186–186.
- Allan, L. G., Siegel, S., & Tangen, J. M. (2005). A signal detection analysis of contingency data. Animal Learning and Behavior, 33, 250–263. doi:10.3758/BF03196067
- Anticevic, A., Cole, M. W., Murray, J. D., Corlett, P. R., Wang, X.-J., & Krystal, J. H. (2012). The role of default network deactivation in cognition and disease. Trends in Cognitive Sciences, 16, 584–592. doi:10.1016/j.tics.2012.10.008
- Aristotle. (350 B.C./1908). On dreams. (J. I. Beare, Trans.). Oxford: Clarendon Press.
- Banks, W. P. (1970). Signal detection theory and human memory. Psychological Bulletin, 74, 81–99. doi:10.1037/h0029531
- Carhart-Harris, R. L., & Friston, K. J. (2010). The default-mode, ego-functions and free-energy: A neurobiological account of Freudian ideas. Brain, 133(Pt 4), 1265–1283. doi:10.1093/brain/awq010
- Corlett, P. R., Simons, J. S., Pigott, J. S., Gardner, J. M., Murray, G. K., Krystal, J. H., & Fletcher, P. C. (2009). Illusions and delusions: Relating experimentally-induced false memories to anomalous experiences and ideas. Frontiers in Behavioral Neuroscience, 3, 53. doi:10.3389/neuro.08.053.2009
- Corlett, P. R., Taylor, J. R., Wang, X. J., Fletcher, P. C., & Krystal, J. H. (2010). Toward a neurobiology of delusions. Progress in Neurobiology, 92, 345–369.
- Currie, G. (2000). Imagination, delusion and hallucinations. In M. Coltheart & M. Davies (Eds.), Pathologies of belief (pp. 167–182). Oxford: Blackwell.
- Dayan, P., & Daw, N. D. (2008). Decision theory, reinforcement learning, and the brain. Cognitive, Affective, and Behavioral Neuroscience, 8, 429–453. doi:10.3758/CABN.8.4.429
- Devinsky, O. (2009). Delusional misidentifications and duplications: Right brain lesions, left brain delusions. Neurology, 72, 80–87. doi:10.1212/01.wnl.0000338625.47892.74
- Dickinson, A. (2001). The 28th Bartlett memorial lecture causal learning: An associative analysis. The Quarterly Journal of Experimental Psychology B, 54, 3–25. doi:10.1080/02724990042000010
- Dube, C., Rotello, C. M., & Heit, E. (2010). Assessing the belief bias effect with ROCs: It's a response bias effect. Psychological Review, 117, 831–863. doi:10.1037/a0019634
- Fox, J. R. (2004). A signal detection analysis of audio/video redundancy effects in television news video. Communication Research, 31, 524–536. doi:10.1177/0093650204267931
- Frank, M. J., Seeberger, L. C., & O'Reilly R,C. (2004). By carrot or by stick: Cognitive reinforcement learning in parkinsonism. Science, 306, 1940–1943. doi:10.1126/science.1102941
- Friston, K. (2009). The free-energy principle: A rough guide to the brain? Trends in Cognitive Sciences, 13, 293–301. doi:10.1016/j.tics.2009.04.005
- Galvin, F. (1990). The boundary characteristics of lucid dreamers. Psychiatric Journal of the University of Ottawa: Revue de psychiatrie de l'Universite d'Ottawa, 15, 73–78.
- Gerrans, P. (2007). Mental time travel, somatic markers and “myopia for the future”. Synthese, 159, 459–474. doi:10.1007/s11229-007-9238-x
- Gerrans, P. (2012). Dream experience and a revisionist account of delusions of misidentification. Consciousness and Cognition, 21, 217–227. doi:10.1016/j.concog.2011.11.003
- Gerrans, P. (2013). Delusional attitudes and default thinking delusional attitudes and default thinking. Mind & Language, 28, 83–102. doi:10.1111/mila.12010
- Gerrans, P. (2014). Pathologies of hyperfamiliarity in dreams, delusions and déjà vu. Frontiers in Psychology, 5, 97. doi:10.3389/fpsyg.2014.00097
- Gerrans, P., & Kennett, J. (2010). Neurosentimentalism and moral agency. Mind, 119, 585–614. doi:10.1093/mind/fzq037
- Grace, A. A. (1991). Phasic versus tonic dopamine release and the modulation of dopamine system responsivity: A hypothesis for the etiology of schizophrenia. Neuroscience, 41, 1–24. doi:10.1016/0306-4522(91)90196-U
- Green, D. M., & Swets, J. A. (1966). Signal detection theory and psychophysics. New York, NY: Wiley.
- Grossberg, S. (2000). How hallucinations may arise from brain mechanisms of learning, attention, and volition. Journal of the International Neuropsychological Society, 6, 583–592. doi:10.1017/S135561770065508X
- Hesselmann, G., Sadaghiani, S., Friston, K. J., Kleinschmidt, A., & Lauwereyns, J. (2010). Predictive coding or evidence accumulation? False inference and neuronal fluctuations. PloS One, 5, e9926. doi:10.1371/journal.pone.0009926.g002
- Johnson, M. K. (2006). Memory and reality. American Psychologist, 61, 760–771. doi:10.1037/0003-066X.61.8.760
- Johnson, M. K., Foley, H. J., Raye, C. L., & Foley, M. A. (1981). Cognitive operations and decision bias in reality monitoring. The American Journal of Psychology, 94, 37–64. doi:10.2307/1422342
- Johnson, M. K., Kahan, T. L., & Raye, C. L. (1984). Dreams and reality monitoring. Journal of Experimental Psychology. General, 113, 329–344. doi:10.1037/0096-3445.113.3.329
- Kopelman, M. D. (2010). Varieties of confabulation and delusion. Cognitive Neuropsychiatry, 15(1), 14–37.
- Korsakoff, S. S. (1955). Psychic disorder in conjunction with peripheral neuritis. Neurology, 5, 394–406.
- Langdon, R., & Turner, M. (2010). Delusion and confabulation: Overlapping or distinct distortions of reality? Cognitive Neuropsychiatry, 15, 1–13. doi:10.1080/13546800903519095
- Lee, D. (2013). Decision making: From neuroscience to psychiatry. Neuron, 78, 233–248. doi:10.1016/j.neuron.2013.04.008
- MacMillan, N., & Creelman, C. (1991). Detection theory: A user's guide. Cambridge: Cambridge University Press.
- McDannald, M. A., Lucantonio, F., Burke, K. A., Niv, Y., & Schoenbaum, G. (2011). Ventral striatum and orbitofrontal cortex are both required for model-based, but not model-free, reinforcement learning. Journal of Neuroscience, 31, 2700–2705. doi:10.1523/JNEUROSCI.5499-10.2011
- McDannald, M. A., Takahashi, Y. K., Lopatina, N., Pietras, B. W., Jones, J. L., & Schoenbaum, G. (2012). Model-based learning and the contribution of the orbitofrontal cortex to the model-free world OFC in model-based learning. European Journal of Neuroscience, 35, 991–996. doi:10.1111/j.1460-9568.2011.07982.x
- McDannald, M. A., Whitt, J. P., Calhoon, G. G., Piantadosi, P. T., Karlsson, R.-M., O'Donnell, P., & Schoenbaum, G.. (2011). Impaired reality testing in an animal model of schizophrenia. Biological Psychiatry, 70, 1122–1126. doi:10.1016/j.biopsych.2011.06.014
- Miller, R. (1976). Schizophrenic psychology, associative learning and the role of forebrain dopamine. Medical Hypotheses, 2, 203–211. doi:10.1016/0306-9877(76)90040-2
- Nahum, L., Bouzerda-Wahlen, A., Guggisberg, A., Ptak, R., & Schnider, A. (2012). Forms of confabulation: Dissociations and associations. Neuropsychologia, 50, 2524–2534. doi:10.1016/j.neuropsychologia.2012.06.026
- Nahum, L., Ptak, R., Leemann, B., & Schnider, A. (2009). Disorientation, confabulation, and extinction capacity: Clues on how the brain creates reality. Biological Psychiatry, 65, 966–972. doi:10.1016/j.biopsych.2009.01.007
- Neider, M., Pace-Schott, E. F., Forselius, E., Pittman, B., & Morgan, P. T. (2011). Lucid dreaming and ventromedial versus dorsolateral prefrontal task performance. Consciousness and Cognition, 20, 234–244. doi:10.1016/j.concog.2010.08.001
- O'Connor, A. R., & Moulin, C. J. A. (2010). Recognition without identification, erroneous familiarity, and déjà vu. Current Psychiatry Reports, 12, 165–173. doi:10.1007/s11920-010-0119-5
- Pace-Schott, E. F. (2013). Dreaming as a story-telling instinct. Frontiers in Psychology, 4, 159. doi:10.3389/fpsyg.2013.00159
- Pessiglione, M., Seymour, B., Flandin, G., Dolan, R. J., & Frith, C. D. (2006). Dopamine-dependent prediction errors underpin reward-seeking behaviour in humans. Nature, 442, 1042–1045. doi:10.1038/nature05051
- Pihan, H., Gutbrod, K., Baas, U., & Schnider, A. (2004). Dopamine inhibition and the adaptation of behavior to ongoing reality. Neuroreport, 15, 709–712. doi:10.1097/00001756-200403220-00027
- Raichle, M. E., MacLeod, A. M., Snyder, A. Z., Powers, W. J., Gusnard, D. A., & Shulman, G. L. (2001). A default mode of brain function. Proceedings of the National Academy of Sciences, 98, 676–682. doi:10.1073/pnas.98.2.676
- Raichle, M. E., & Snyder, A. Z. (2007). A default mode of brain function: A brief history of an evolving idea. Neuroimage, 37, 1083–1090; discussion 1097–1089. doi:10.1016/j.neuroimage.2007.02.041
- Rescorla, R. A., & Wagner, A. R. (1972). A theory of Pavlovian conditioning: Variations in the effectiveness of reinforcement and non-reinforcement. In A. H. Black & W. F. Prokasy (Eds.), Classical conditioning II: Current research and theory (pp. 64–99). New York, NY: Appleton-Century-Crofts.
- Sass, L. A. (1994). Paradoxes of delusion: Wittgenstein, Schreber, and the schizophrenic mind. Ithaca, NY: Cornell University Press.
- Schnider, A. (2001). Spontaneous confabulation, reality monitoring, and the limbic system—a review. Brain Research Reviews, 36, 150–160. doi:10.1016/S0165-0173(01)00090-X
- Schnider, A. (2003). Spontaneous confabulation and the adaptation of thought to ongoing reality. Nature Reviews Neuroscience, 4, 662–671. doi:10.1038/nrn1179
- Schnider, A., Bonvallat, J., Emond, H., & Leemann, B. (2005). Reality confusion in spontaneous confabulation. Neurology, 65, 1117–1119. doi:10.1212/01.wnl.0000178900.37611.8d
- Schnider, A., Guggisberg, A., Nahum, L., Gabriel, D., & Morand, S. (2010). Dopaminergic modulation of rapid reality adaptation in thinking. Neuroscience, 167, 583–587. doi:10.1016/j.neuroscience.2010.02.044
- Schultz, W., Dayan, P., & Montague, P. R. (1997). A neural substrate of prediction and reward. Science, 275, 1593–1599. doi:10.1126/science.275.5306.1593
- Schultz, W., & Dickinson, A. (2000). Neuronal coding of prediction errors. Annual Review of Neuroscience, 23, 473–500. doi:10.1146/annurev.neuro.23.1.473
- Shapiro, M. (1994). Signal detection measures of recognition memory. In A. Lang (Ed.), Measuring psychological responses to media messages (pp. 133–148). Hillsdale, NJ: Lawrence Erlbaum.
- Simons, J. S., Davis, S. W., Gilbert, S. J., Frith, C. D., & Burgess, P. W. (2006). Discriminating imagined from perceived information engages brain areas implicated in schizophrenia. Neuroimage, 32, 696–703. doi:10.1016/j.neuroimage.2006.04.209
- Simons, J. S., Henson, R. N. A., Gilbert, S. J., & Fletcher, P. C. (2008). Separable forms of reality monitoring supported by anterior prefrontal cortex. Journal of Cognitive Neuroscience, 20, 447–457. doi:10.1016/j.tics.2005.07.001
- Smith, A., Li, M., Becker, S., & Kapur, S. (2006). Dopamine, prediction error and associative learning: A model-based account. Network, 17, 61–84. doi:10.1080/09548980500361624
- Spreng, R. N., & Grady, C. L. (2010). Patterns of brain activity supporting autobiographical memory, prospection, and theory of mind, and their relationship to the default mode network. Journal of Cognitive Neuroscience, 22, 1112–1123. doi:10.1037/0033-2909.121.3.331
- Spreng, R. N., Mar, R. A., & Kim, A. S. N. (2009). The common neural basis of autobiographical memory, prospection, navigation, theory of mind, and the default mode: A quantitative meta-analysis. Journal of Cognitive Neuroscience, 21, 489–510. doi:10.1016/j.neuroimage.2004.12.013
- Spreng, R. N., Stevens, W. D., Chamberlain, J. P., Gilmore, A. W., & Schacter, D. L. (2010). Default network activity, coupled with the frontoparietal control network, supports goal-directed cognition. Neuroimage, 53, 303–317. doi:10.1016/j.neuroimage.2010.06.016
- Takahashi, Y. K., Chang, C. Y., Lucantonio, F., Haney, R. Z., Berg, B. A., Yau, H.-J., … Schoenbaum, G. (2013). Neural estimates of imagined outcomes in the orbitofrontal cortex drive behavior and learning. Neuron, 80(2), 507–518. doi:10.1016/j.neuron.2013.08.008
- Takahashi, Y. K., Roesch, M. R., Stalnaker, T. A., Haney, R. Z., Calu, D. J., Taylor, A. R., … Schoenbaum, G. (2009). The orbitofrontal cortex and ventral tegmental area are necessary for learning from unexpected outcomes. Neuron, 62(2), 269–280. doi:10.1016/j.neuron.2009.03.005
- Turner, M., & Coltheart, M. (2010). Confabulation and delusion: A common monitoring framework. Cognitive Neuropsychiatry, 15, 346–376. doi:10.1080/13546800903441902
- Turner, M. S., Cipolotti, L., & Shallice, T. (2010). Spontaneous confabulation, temporal context confusion and reality monitoring: A study of three patients with anterior communicating artery aneurysms. Journal of the International Neuropsychological Society, 16, 984–994. doi:10.1017/S1355617710001104
- Vaitl, D., Birbaumer, N., Gruzelier, J., Jamieson, G. A., Kotchoubey, B., Kübler, A., … Thomas, W. (2005). Psychobiology of altered states of consciousness. Psychological Bulletin, 131, 98–127. doi:10.1037/0033-2909.131.1.98
- Waltz, J. A., Frank, M. J., Robinson, B. M., & Gold, J. M. (2007). Selective reinforcement learning deficits in schizophrenia support predictions from computational models of striatal-cortical dysfunction. Biological Psychiatry, 62, 756–764. doi:10.1016/j.biopsych.2006.09.042
- Waltz, J. A., Kasanova, Z., Ross, T. J., Salmeron, B. J., McMahon, R. P., Gold, J. M., & Stein, E. A. (2013). The roles of reward, default, and executive control networks in set-shifting impairments in schizophrenia. PloS One, 8, e57257. doi:10.1371/journal.pone.0057257.s010
- Wamsley, E., Donjacour, C. E., Scammell, T. E., Lammers, G. J., & Stickgold, R. (2014). Delusional confusion of dreaming and reality in narcolepsy. Sleep, 37, 419–422.
- Whitfield-Gabrieli, S., & Ford, J. M. (2012). Default mode network activity and connectivity in psychopathology. Annual Review of Clinical Psychology, 8, 49–76. doi:10.1146/annurev-clinpsy-032511-143049
- Whittlesea, B. W., Masson, M. E., & Hughes, A. D. (2005). False memory following rapidly presented lists: The element of surprise. Psychological Research, 69, 420–430.