Abstract
Purpose: The diagnosis of tuberculous meningitis (TBM) in children is often delayed due to diagnostic difficulties. New tools are urgently needed to improve the diagnosis of the disease in this vulnerable group. The present study aimed to validate the accuracy of recently identified host cerebrospinal (CSF) biomarkers as candidates for the diagnosis of TBM in children.
Materials and methods: We collected CSF samples from 87 children aged 3 months to 13 years, that were consecutively admitted at a tertiary hospital in Cape Town, South Africa, on suspicion of having TBM. We evaluated the concentrations of 67 selected host protein biomarkers using a multiplex platform.
Results: Previously identified 3-marker (VEGF-A + IFN-γ + MPO) and 4-marker (IFN-γ + MPO + ICAM-1 + IL-8) signatures diagnosed TBM with AUCs of 0.89 (95% CI, 0.81–0.97) and 0.87 (95% CI, 0.79–0.95) respectively; sensitivities of 80.6% (95% CI, 62.5–92.5%) and 81.6% (95% CI, 65.7–92.3%), and specificities of 86.8% (71.9–95.6%) and 83.7% (70.4–92.7%) respectively. Furthermore, a new combination between the analytes (CC4b + CC4 + procalcitonin + CCL1) showed promise, with an AUC of 0.98 (95% CI, 0.94–1.00).
Conclusions: We have shown that the accuracies of previously identified candidate CSF biomarkers for childhood TBM was reproducible. Our findings augur well for the future development of a simple bedside test for the rapid diagnosis of TBM in children.
Background
Globally, tuberculosis (TB) is among the leading causes of death and the second leading cause of death by a single infectious agent after COVID-19 (WHO Citation2021). An estimated 10 million people fell ill with TB and 1.5 million died from the disease in 2020 worldwide (WHO Citation2021). Children under the age of 15 years represented 11% of the worldwide TB burden in 2020 (WHO Citation2021). Tuberculous meningitis (TBM), the most severe form of TB, mostly affects young children and has an increased risk of death (WHO Citation2018). The true global burden of TBM is not known as many individuals with TBM remain undiagnosed, untreated, and are not reported to the surveillance systems (Seddon and Thwaites Citation2019). It is, however, estimated that 2–5% of new cases of TB are TBM (Seddon and Thwaites Citation2019). Despite the availability of anti-tuberculosis therapy, TBM results in death in up to 20% of affected children, and severe neurological sequelae in more than half of the survivors (Chiang et al. Citation2014). Delay in diagnosis is one of the major concerns in TBM, leading to poor outcomes.
The clinical features seen in TBM may be similar to those in many forms of sub-acute meningoencephalitis, often resulting in diagnostic confusion (Thwaites et al. Citation2002). Cerebrospinal fluid (CSF) cell count and biochemistry profiles lack sensitivity and are non-specific to use as the primary method of diagnosis (Bahr and Boulware Citation2014). Although clinical criteria have been developed to improve the diagnosis of TBM, performance variability may occur due to atypical clinical features, especially with prevalence of tuberculosis and human immunodeficiency virus (HIV) co-infection (Thwaites et al. Citation2002). The visualisation of acid-fast bacilli by microscopy of the CSF and mycobacterial culture remains the traditional methods for definite diagnosis of TBM. Although microscopy is cheap and rapid, the test is notoriously insensitive (∼10 to 15%) in routine practice for the diagnosis of TBM (Bahr and Boulware Citation2014). Mycobacterial culture has better sensitivity (∼60 to 70%) but is too slow (2–6 weeks) to provide results for timely clinical intervention. Furthermore, culture requires a biosafety level 3 laboratory which is difficult to implement in resource-limited settings (Bahr and Boulware Citation2014, Bahr et al. Citation2019).
Recent molecular technologies, particularly the nucleic acid amplification tests, have shown improved accuracy and turn-around time for TBM diagnosis, but none of the existing tests is adequate as a stand-alone method for diagnosis of TBM (Pormohammad et al. Citation2019). The Xpert MTB/RIF assay is a rapid molecular test that is based on a real-time polymerase chain reaction (PCR) cartridge system that allows for simultaneous detection of Mycobacterium tuberculosis (M.tb) and rifampicin resistance within 2 hours (Lawn and Nicol Citation2011). A sensitivity of only around 50–60% for the diagnosis of TBM is achieved with Xpert MTB/RIF test, although varying performances have been reported (Patel et al. Citation2013, Nhu et al. Citation2014, Bahr, Nuwagira, et al. Citation2018, Bahr et al. Citation2019). The re-engineered assay, termed Xpert MTB/RIF Ultra (Xpert Ultra) has shown higher sensitivity than that of either the initial Xpert or culture in the diagnosis of TBM in recent studies (Bahr, Nuwagira, et al. Citation2018, Chin et al. Citation2019, Cresswell et al. Citation2020). Consequently, the WHO recommends Xpert Ultra as an alternative to Xpert as the initial test for TBM (WHO Citation2017). Despite improved accuracy, both Xpert MTB/RIF and Xpert Ultra are not adequate to confidently rule out TBM due to imperfect negative predictive value (Cresswell et al. Citation2020). Other important challenges of Xpert assays are the relatively high operational cost and infrastructural requirements which limit their implementation or wide use in resource-limited settings (Lawn and Nicol Citation2011, Creswell et al. Citation2014). New methods for early diagnosis and management of TBM are therefore urgently needed. In line with the target to end the TB epidemic globally by 2030, the WHO encourages the development of child-friendly point of care (POC) tests with good accuracy for diagnosis of TB in children (WHO Citation2018).
As the detection of bacilli or their products can be challenging, CSF host response biomarkers that may play an adjunctive role in improving the diagnosis of TBM have been explored (Visser et al. Citation2015, Manyelo et al. Citation2019a). The WHO has recommended the development of a rapid biomarker-based non-sputum-based test for detection of extrapulmonary TB (EPTB), including detection in CSF specimens, and specified the TTP for such a test in adult patients, in which the sensitivity of at least 80% and specificity of 98% (or similar to that of GeneXpert MTB/RIF test) are required (WHO Citation2014). A rapid CSF biomarker-based test would facilitate the diagnosis of TBM and guide clinicians to quickly initiate proper treatment to reduce the chances of death or long-term disability.
Recent studies have identified host protein biomarkers with potential as diagnostic candidates for pulmonary (Chegou et al. Citation2016, Jacobs et al. Citation2016a, Jacobs et al. Citation2016b, MacLean et al. Citation2019) and EPTB, including TBM (Peng et al. Citation2014, Visser et al. Citation2015, Chen et al. Citation2016, Bahr, Halupnick, et al. Citation2018, Manyelo et al. Citation2019a, Manyelo et al. Citation2019b). In a previous study, we identified a 3-marker CSF biosignature comprising VEGF-A, IL-13, and cathelicidin LL-37, which diagnosed TBM in children with sensitivity of 52% and specificity of 95% (Visser et al. Citation2015). In a more recent report, we showed that refinement of this 3-marker signature by the replacement of IL-13 and cathelicidin LL-37 with new biomarkers; IFN-γ and MPO respectively, improved the diagnostic accuracy of the signature (78.3% sensitivity and 91.7% specificity after leave-one-out cross-validation) (Manyelo et al. Citation2019a). Furthermore, we identified other candidate biomarkers and biomarker combinations which also showed strong potential as childhood TBM diagnostic candidates (Manyelo et al. Citation2019a). Given the promising accuracy of the biosignatures plus the many other candidate biomarkers which showed potential, we proposed that further replication of the identified biomarkers in more children was necessary, to confirm their diagnostic potentials (Manyelo et al. Citation2019a). In the present study, we sought to replicate the performance of our recently identified three- and four- marker CSF biosignatures (Manyelo et al. Citation2019a) in more children with suspected TBM, and to further refine the biosignatures and explore combinations between other candidate biomarkers that also showed potential in our previous study.
Methods
Study setting and participants
Study participants were recruited at Tygerberg Academic Hospital, a large tertiary referral hospital that is based in Cape Town, South Africa. Children presenting with signs and symptoms suggestive of meningitis seen at primary community health centres, district, and secondary level hospitals from within the referral drainage area are referred to Tygerberg Hospital to establish TBM diagnosis, and to treat complications associated with advanced stage TBM disease (stages 2 and 3). The study protocol was reviewed and approved by the Health Research Ethics Committee of Stellenbosch University (N16/11/142).
We prospectively enrolled children who were admitted to Tygerberg Hospital on suspicion of meningitis between 2016 and 2020. The inclusion criteria for the study were age between 3 months and 13 years and suspicion of having meningitis. Written informed consent was provided by parents or legal guardians/caregivers of the participants. Assent was obtained if children were older than 7 years, provided that they had a normal level of consciousness based on the Glasgow Coma Score (GCS), wherein a GCS of 15/15 was considered as normal. Some of the severely ill children admitted to the hospital during the study period were not recruited owing to these children being too ill and requiring emergency treatment. All the study participants were TB treatment naïve at the time of enrolment.
Procedures
Comprehensive clinical investigation including assessment of the signs and symptoms, history of TB contact, HIV test, GCS, tuberculin skin test (TST), and chest radiography were carried out on all study participants. Routine computed Tomography (CT) of the brain was performed in all suspected TBM cases, and in other forms of meningitis when indicated. Magnetic resonance imaging (MRI) was performed when clinically indicated. Air- encephalography was performed in all TBM cases with hydrocephalus demonstrated on neuroimaging, provided there were no contra-indications to performing a lumbar puncture (LP).
After collection of a lumbar CSF specimen, routine CSF diagnostic investigations included colour and appearance, differential cell count determination, protein concentration and glucose levels. Each CSF sample was further microbiologically examined by gram staining, India ink staining, culture of the centrifuged sediment on blood agar plates for pyogenic bacteria, Auramine ‘O’ staining & fluorescence microscopy, culture using the mycobacterium growth indicator tubes (MGIT)™ method (Becton and Dickinson), GeneXpert MTB/RIF and HAIN Genotype MTBDRplus kit for M.tb DNA when the mycobacterial culture was positive. Other additional investigations included viral PCR and the determination of serum glucose levels. Following the collection of specimens for routine diagnostic purposes, additional CSF samples were collected and transported to the research laboratory for this study. The CSF samples were centrifuged at 4000 xg for 15 minutes, after which the supernatants were harvested and stored at −80 °C until the protein biomarkers were measured.
Classification of study participants
Definite TBM, probable TBM, and no-TBM were defined using a uniform research case definition for TBM (Marais et al. Citation2010), consistent with our previous studies (Manyelo et al. Citation2019a, Manyelo et al. Citation2019b): briefly, (1) Children with microbiologically-confirmed TBM by either the detection of acid-fast bacilli in the CSF, positive CSF culture or a positive CSF GeneXpert MTB/RIF result were classified as having definite TBM; (2) Probable TBM cases were defined based on a scoring system that combines clinical criteria, CSF criteria, neuroimaging criteria, and evidence of TB elsewhere; (3) children with alternative diagnoses including bacterial meningitis, viral meningitis, and no meningitis were defined as ‘no-TBM’.
Immunoassays
We measured the concentrations of 67 host protein biomarkers in CSF samples from all the study participants using either ELISA (transthyretin) or the Luminex multiplex immunoassay platform (all other biomarkers). We included the biomarkers comprising our previously identified 3-and 4-marker CSF biosignatures, along with the other biomarkers that showed potential as TBM diagnostic candidates in our previous study (Manyelo et al. Citation2019a, Manyelo et al. Citation2019b), and also promising biomarkers identified by other studies (Xu et al. Citation2015, Jacobs et al. Citation2016a, Ahmad et al. Citation2019, Sudbury et al. Citation2019).
CSF transthyretin concentrations were measured using a commercially available kit (Novus Biologicals, Biotechne, USA, Catalog #NBP2-60516) according to the manufacturer’s instructions. The absorbance at 450 nm was read using an automated microplate reader (iMarkTM Microplate Reader, Bio-Rad Laboratories), and the generated standard curve was used to determine the concentrations in each sample.
The concentrations of the other 66 host protein biomarkers were determined using Luminex multiplex kits supplied by Merck Millipore (Billerica, MA, USA) and R&D systems (Bio-Techne, Minneapolis, USA), according to the manufacturer’s instructions (Supplementary Table 1). Luminex plates were read on either a Bio-Plex 200 or Magpix instrument (Bio-Rad Laboratories), in an ISO 15189 accredited laboratory. Bead acquisition and analysis of median fluorescence intensity were done using the Bio-Plex Manager 6.1 software (Bio-Rad Laboratories). The laboratory staff performing the experiments were blinded to the clinical classification of the children. The quality control samples included in the assays yielded values that were within the expected ranges.
Statistical analysis
Analyses were performed using Statistica (TIBCO Software Inc., CA, USA) and GraphPad Prism version 9 (Graphpad Software Inc., CA, USA). Differences in the concentrations of single biomarkers were compared between the clinical groups (TBM and no-TBM) using the Mann-Whitney U test. p-values < 0.05 were considered significant. The diagnostic abilities of individual biomarkers were evaluated using receiver operator characteristic (ROC) curve analysis. Sensitivity and specificity values were determined by a selection of optimal cut-off values based on Youden’s index (Fluss et al. Citation2005). The diagnostic accuracies of combinations between biomarkers were assessed by general discriminant analysis (GDA), followed by leave-one-out cross-validation. Variable selection for the GDA was done using the all-subset regression method. V-fold cross validation was used for selecting best models. Consistency of markers to be selected was evaluated by counting how many times they appeared in the top 20 models.
Results
Between December 2016 and October 2020, 113 children were prospectively enrolled into the study, 87 of whom provided sufficient CSF samples for the present study (). Of these 87 children, 48 (55.2%) were males (). Among 81 with available HIV infection results, 8 (9.9%) were positive. Thirty-eight (43.7%) of the 87 children were diagnosed with TBM (11 definite TBM and 27 probable TBM) and 49 (56.3%) had alternative diagnoses including viral meningitis (n = 2), bacterial meningitis (n = 6), and no meningitis (n = 41). The 49 participants with alternative diagnoses were classified as no-TBM during data analysis. The baseline characteristics of the study participants are shown in .
Figure 1. STARD diagram depicting the study design and classification of study participants. The study participants were classified as definite TBM, probable TBM and no-TBM using a uniform research case definition (Marais et al. Citation2010). CRF: case report form; CSF: cerebrospinal fluid; ELISA: enzyme-linked immunosorbent assay; TBM: Tuberculous meningitis; no-TBM: children presenting with signs and symptoms suggestive of TBM, but finally diagnosed with other meningitis or no meningitis (see ).
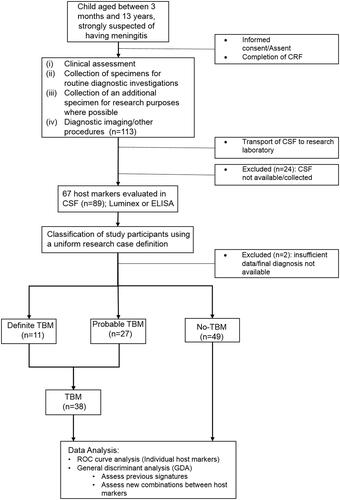
Table 1. Clinical and demographic characteristics of study participants.
Utility of previously identified 3-and 4-marker CSF biosignatures in the diagnosis of TBM in the current study
In previously published work (Manyelo et al. Citation2019a), we showed that 3-marker (IFN-γ + MPO + VEGF) and 4-marker (IFN-γ + MPO + ICAM-1 + IL-8) CSF biosignatures had strong potential as diagnostic candidates for childhood TBM (Manyelo et al. Citation2019a). To assess the replicability of these signatures, we evaluated their diagnostic accuracy in the current dataset.
3-Marker signature
Individually, all three biomarkers comprising the 3-marker signature showed diagnostic potential (AUC ≥ 0.83) in the current study (Supplementary table 2). When evaluated in study participants who had valid data for all three proteins comprising the signature (n = 69, n = 31 TBM and n = 38 no TBM), the 3-marker biosignature diagnosed TBM with an AUC of 0.89 (95% CI, 0.81–0.97), sensitivity of 80.6% (25/31) (95% CI, 62.5–92.5%) and specificity of 86.8% (33/38) (95% CI, 71.9–95.6%) (, ). The sensitivity and specificity of the signature remained the same after leave-one-out cross-validation. The positive predictive value (PPV) and negative predictive value (NPV) of the 3-makrer signature were 83.3% (95% CI, 68.5–92.0%) and 84.6% (95% CI, 72.6–91.9%), respectively (). When sensitivity was fixed at 80%, the cut-off value that is recommended by the World Health Organisation target product profiles (WHO TTP) for a rapid biomarker test for the diagnosis of extrapulmonary TB in adults (including TBM) (WHO Citation2014), the specificity of the signature (87%), was however, lower than the stipulated 98% (). When the cut-off values obtained for the 3 individual biomarkers in our previous study (Manyelo et al. Citation2019a) were applied to the current dataset, the most optimal approach (positivity for any two of the three biomarkers) yielded a sensitivity of 87.1% (95% CI, 71.2 − 94.9%) and specificity of 92.1% (95% CI, 79.2–97.3%), with NPV and PPV of 89.7% (95%, 77.7–95.6%) and 90.0% (95%, 75.1–96.4%), respectively ().
Figure 2. Performance of the previously identified host CSF protein biosignatures in the diagnosis of TBM in children. Receiver operator characteristics (ROC) curve showing the accuracy of the three-marker CSF biosignature (VEGF + IFN-γ + MPO) (Manyelo et al. Citation2019a) in diagnosing TBM (A), Scatter plot showing the ability of the three-marker biosignature in discriminating between TBM and no-TBM (B), ROC curve showing the accuracy of the four-marker CSF biosignature (IFN-γ + MPO + ICAM-1 + IL-8) (Manyelo et al. Citation2019a) in diagnosing TBM (C), Scatter plot showing the ability of the four-marker biosignature in discriminating between TBM and no-TBM (D).
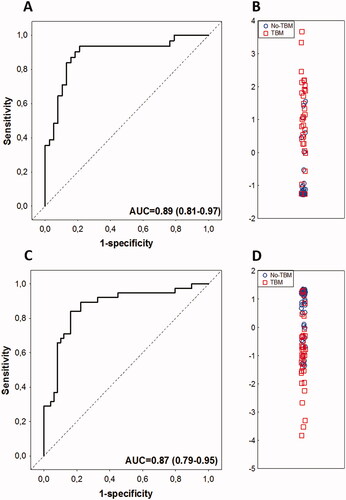
Table 2. Diagnostic accuracy of the host CSF protein biosignatures described in the present study in the diagnosis of TBM in children.
Table 3. The diagnostic accuracy obtained when the study participants were diagnosed as TBM based on data from one or more of the three proteins in the 3-marker signature (Manyelo et al. Citation2019a).
4-Marker signature
The two analytes from this previously identified 4-marker signature (MPO + IFN-γ + ICAM-1 + IL-8) that were not part of the 3-marker model (ICAM-1 and IL-8) also showed potential individually as TBM diagnostic candidates, with AUCs of 0.78 and 0.87, respectively (Supplementary table 2). When all participants with valid data for the four analytes (n = 87; n = 38 TBM and n = 49 no TBM) were considered, the 4-marker biosignature diagnosed TBM with an AUC of 0.87 (95% CI, 0.79–0.95), sensitivity of 81.6% (31/38) (95% CI, 65.7–92.3%) and specificity of 83.7% (41/49) (95% CI, 70.4–92.7%), with both the sensitivity and specificity remaining the same after leave-one-out cross-validation. The PPV and NPV of the 4-marker signature were 79.5% (95% CI, 66.9–88.1%) and 85.4% (95% CI, 74.8–92.0%), respectively (, ). At the WHO TPP recommended sensitivity threshold of ≥80% (WHO Citation2014), the specificity of the 4-marker model was 84% (). When the cut-off values obtained for the different biomarkers in our previous study (Manyelo et al. Citation2019a), were applied to this dataset, the best accuracy was obtained with positivity for any two out of the four biomarkers; which diagnosed TBM with a sensitivity of 89.5% (95% CI, 75.2–97.1), specificity of 85.7% (95% CI, 72.8–94.1), PPV of 82.9% (95% CI, 70.1–90.7) and NPV of 91.3% (95% CI, 80.5–96.4) ().
Table 4. The diagnostic accuracy obtained when the study participants were diagnosed as TBM based on data from one or more of the four proteins in the previously identified 4-marker signature (Manyelo et al. Citation2019a).
Utility of all individual host CSF protein biomarkers in the diagnosis of TBM
We assessed differences in the concentrations of the 67 protein biomarkers that were selected for evaluation in this study, between children diagnosed with (n = 38) and without TBM (n = 49). Out of the 67 biomarkers evaluated, 54 were significantly different (p < 0.05) between the two groups irrespective of HIV infection status, according to the Mann Whitney U test (Supplementary Table 2). After correcting for multiple testing using the Bonferroni method, 47 remained significantly different between the two groups (p < 0.0007) (Supplementary table 2).
When ROC curve analysis was used to investigate the abilities of individual biomarkers to diagnose TBM irrespective of HIV status of the study participant, the area under the curve (AUC) was between 0.80 and 0.95 for 34 of the 67 proteins, namely; CCL1 (I-309), IFN-γ, TNF-α, GM-CSF, VEGF-A, CXCL9 (MIG), IL-10, CXCL11 (I-TAC), CCL18 (MIP-4), IL-1β, CXCL8 (IL-8), MMP-8, CCL5 (RANTES), IL-18, IL-12/23p40, Complement component (CC) 2, PAI-1, CCL22 (MDC), CCL4 (MIP-1β), S100A9, CD40 ligand, IL-6, CC5, CC5a, MMP-1, IL-1RA, apolipoprotein AI, MBL, MPO, CC4b, CXCL10 (IP-10), CC4, Complement factor I, and IL-1α (Supplementary table 2). Scatter plots and ROC curves for the six most accurate biomarkers (AUC ≥ 0.90) are shown in .
Figure 3. Scatter plots showing the concentrations of CCL1 (A), IFN-γ (B), TNF-α (C), VEGF-A (G), GM-CSF (H), and CXCL9 (I) in CSF samples from children with TBM and no-TBM, and the receiver operator characteristics curves showing the accuracies of CCL1 (D), IFN-γ (E), TNF-α (F), VEGF-A (J), GM-CSF (K), and CXCL9 (L) in the diagnosis of TBM. The error bars depict the median concentrations and interquartile ranges. Uncorrected Mann-Whitney U test p-values for the differences between the medians of the groups are shown for each biomarker. Data obtained after correcting for multiple testing are shown in and Supplementary Table 2. Representative plots for the six most accurate individual biomarkers (AUC > 0.90) are shown.
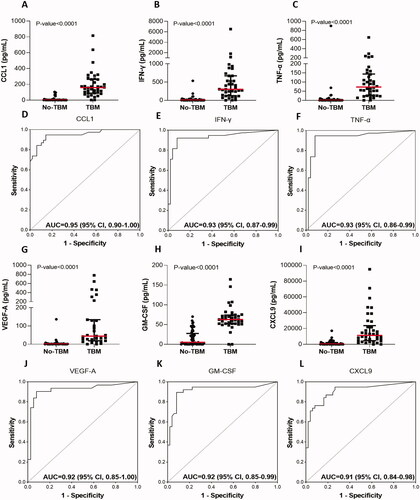
Identification of alternative CSF protein biosignatures for the diagnosis of TBM in children
Given that all candidate biomarkers which were significantly different in children with and without TBM in our previous studies (Manyelo et al. Citation2019a, Manyelo et al. Citation2019b) were included in the current study, we assessed combinations between biomarkers again, to ascertain whether alternative biosignatures that were better than the previously identified 3- and 4-marker models could be identified. Data obtained for all the 67 biomarkers investigated were fitted into general discriminant analysis (GDA) models irrespective of HIV infection status, and without any constraints on the identity and/or number of biomarkers in the model. Optimal diagnosis of TBM was shown to be achieved with a combination of up to 5 biomarkers. The most accurate biosignature identified in the current dataset was a combination between four biomarkers namely; CC4b, CC4, procalcitonin, and CCL1, which diagnosed TBM with an AUC of 0.98 (95% CI, 0.94–1.00), sensitivity of 89.5% (34/38) (95% CI, 75.2–97.1%) and specificity of 100.0% (49/49) (95% CI, 94.1–100%) (, ). After leave-one-out cross-validation, the sensitivity of the signature was 81.6% (31/38) (95% CI, 65.7–92.3%) and specificity was 100.0% (49/49) (95% CI, 94.1–100%), with PPV and NPV of 100.0% (95% CI, 92.7–100.0%) and 87.5% (95% CI, 78.2–93.2%), respectively. At the WHO TTP recommended sensitivity threshold of ≥80%, the specificity of the new 4-marker model was 100%, therefore meeting the optimal requirements for a new biomarker-based test for EPTB (). Another alternative 4-marker model comprising apolipoprotein-CIII, CC4, RANTES and TNF-α also showed promise in the diagnosis of TBM, with AUC of 0.92 (95% CI, 0.86–0.98) (, Supplementary figure 1).
Figure 4. Performance of the alternative CSF protein biosignature in the diagnosis of TBM in children. Receiver operator characteristic (ROC) curve showing the accuracy of the novel four-marker CSF biosignature (CC4b + CC4 + Procalcitonin + CCL1) in the diagnosing TBM (A), Scatter plot showing the ability of the four-marker biosignature in discriminating between TBM and no-TBM (B), Frequency of proteins in the top 20 general discriminant analysis (GDA) models that accurately classified children with TBM and no-TBM (C).
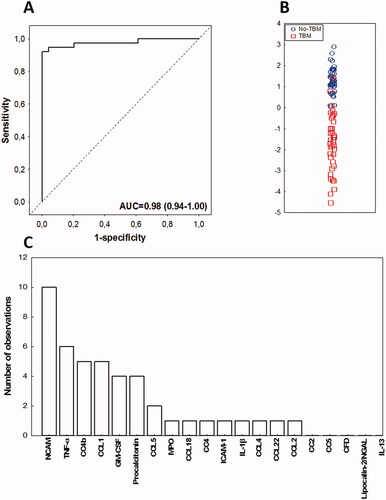
Discussion
The significant difficulty and delay in TBM diagnosis emphasizes the need for new tools to improve the diagnosis of the disease, in order to reduce the frequency of adverse outcomes. In the present study, we assessed the utility of host inflammatory biomarkers that were identified in previous studies as tools for the diagnosis of TBM in children. Individual biomarkers that were identified in previous work done in our research group demonstrated strong potential as diagnostic candidates for TBM in the current study (Manyelo et al. Citation2019a). We validated previously identified CSF host 3-marker (VEGF-A, IFN-γ, and MPO) and 4-marker (IFN-γ, MPO, ICAM-1 and IL-8) protein biosignatures for diagnosis of TBM in children and identified a novel 4-marker biosignature comprising CC4b, CC4, procalcitonin, and CCL1 which also showed promise.
The host CSF protein biosignatures investigated in the current study are promising for the development of a POC or bedside biomarker-based test for the early diagnosis of TBM. The three-marker CSF protein biosignature validated in this study provided a sensitivity of 84.0% and specificity of 87.0% when benchmarked against WHO TTP. Using the cut-off points from our previous study, we also demonstrated that the three-marker CSF biosignature achieved the best accuracy with a sensitivity of 87.1% and specificity of 92.1% when positivity for any two of the three biomarkers was considered. The four-marker CSF biosignature had a sensitivity of 84.0% and specificity of 84% when benchmarked against the WHO TPP and when the previous cut-off points were used, the signature provided the best accuracy (sensitivity of 89.5% and specificity of 85.7%) with positivity for any two of the four biomarkers. Thus, the overall sensitivity and specificity of the three-marker and four-marker biosignatures when using the cut-off points from our previous study or the new cut-off points from the current study approached the WHO TPP. The diagnostic performance of the three- and four-marker biosignatures in the current study was relatively closer to the accuracy achieved by these signatures in our previous study, thus demonstrating the replicability of these signatures in this larger cohort of children. The validated host protein biosignatures from this study are promising for incorporation into a rapid biomarker-based POC or bedside test for diagnosis of TBM in children. The promising alternative novel 4-marker CSF biosignature (CC4b, CC4, procalcitonin, and CCL1) and other combinations between analytes identified in this study, along with the candidate biomarkers that showed potential individually could be suitable for further optimisation of the biosignatures or as backup replacements during the possible development of a POC or bedside test in future.
A future rapid biomarker-based test could be based on a simple, cheap, and user-friendly technology to allow implementation at points of care or bedside, and may significantly reduce the delays currently incurred in the diagnosis of TBM in children. A previous study from the Western Cape Province of South Africa, which is a relatively better-resourced area showed that children from this setting still required an average of six primary health care visits before a proper TBM diagnosis could be made (Solomons et al. Citation2016). This emphasizes the need for improved rapid diagnostic tools for early diagnosis or screening of TBM, to guide physicians towards expeditious and correct treatment, to reduce poor outcomes. Such a POC biomarker-based test that makes use of simple and easy-to-use technology (for example, the lateral flow assay platform) would be more advantageous compared to the current available molecular methods such as PCR-based assays in the resource-limited settings, as it will have less infrastructural requirements. Such a simple test would be a game-changer especially in resource-limited settings, in which children may have limited access to facilities required to establish a diagnosis when using clinical criteria, including neuroimaging. A rapid and accurate biomarker-based test may also help in deciding whether to treat TBM or not when culture results are not available, due to the well-known limitation of long turn-around time that is applicable to culture, and will also be useful in settings with limited access to GeneXpert or Xpert Ultra. Although a CSF-based test would require an invasive sample collection through lumbar puncture by experienced personnel, the benefits are the possible quick turn-around time of such a test and thus timely management of TBM.
Our findings are in line with our previous work and studies from others, in which host immune response protein biomarkers with potential for diagnosis of TBM were identified (Peng et al. Citation2014, Visser et al. Citation2015, Chen et al. Citation2016, Manyelo et al. Citation2019a, Manyelo et al. Citation2019b). The potential of the CSF protein biomarkers investigated in the current study aligns with the fact that some of the biomarkers have already been associated with immune responses in TBM. For instance, VEGF, a potent mediator of vascular permeability and angiogenesis, has been implicated in brain edoema due to ischaemic stroke, tumours, and infections. Several studies reported increased VEGF concentrations in the CSF of TBM patients (Matsuyama et al. Citation2001, Flier et al. Citation2004, Husain et al. Citation2008, Misra et al. Citation2013, Visser et al. Citation2015) and VEGF consistently showed promise for the diagnosis of TBM in our previous studies (Visser et al. Citation2015, Manyelo et al. Citation2019a). Increased levels of pro-inflammatory cytokines (IFN-γ and TNF-α) and chemokines (IL-8 and RANTES) contributing to our biosignatures have been reported in patients with TBM (Misra et al. Citation2010, Visser et al. Citation2015). Procalcitonin, a calcitonin precursor, has been extensively described as a biomarker for diagnosis of bacterial meningitis (Wei et al. Citation2016, Reshi et al. Citation2017) and TBM (Kim et al. Citation2016), and notably, it was included in two new combinations between analytes in the current study. Complement C4 and its fragment peptide C4b are molecules of the complement system, which is involved in both the innate and adaptive immunity, defending against pathogens and maintaining homeostasis (Merle et al. Citation2015, Li et al. Citation2017, Wang and Liu Citation2021). CC4 is an essential complement system protein that plays a critical role in the induction of immune defences via the activation of the classical and lectin complement pathways (Li et al. Citation2017, Wang and Liu Citation2021). Complement proteins have been identified as markers for TB in other studies (Chegou et al. Citation2016, Jacobs et al. Citation2016a) and in other neurological diseases, such multiple sclerosis (an immune-mediated disease of the central nervous system) (Ingram et al. Citation2012). CCL1 (I-309), a member of CC chemokine family, is an inflammatory mediator that plays a role in monocyte migration, and has contributed to biosignatures for the diagnosis of TB in other recent studies (Chendi et al. Citation2021, Mutavhatsindi et al. Citation2021). A predictive model linking the 3-marker CSF biosignature (VEGF, IFN-γ, and MPO) and the downstream metabolic effects in the brain, as well as the expected urinary metabolic profiles, was proposed in a recent review (Isaiah et al. Citation2020). The suggested role of the 3-marker CSF biosignature in brain metabolic processes further supports the evidence that this may be a putative signature for the diagnosis of TBM.
Our study has some limitations. As only a small proportion of children with definite TBM was included, the accuracy of the biosignatures was determined only against a composite score (uniform research case definition) (Marais et al. Citation2010) and not against the microbiological reference standard. However, this was a relatively well-designed study as the children were enrolled on suspicion of TBM (TBM-suspect study), in routine clinical management conditions. Due to a small proportion (9.9%) of the study participants who were HIV-infected, we were not able to assess the effect of HIV infection on the performance of the biosignatures. The low percentage of HIV co-infected patients in our study is consistent with previous studies, including studies from the same study setting, which found that only a small proportion of children clinically diagnosed with TBM had HIV co-infection (Karande et al. Citation2005, Solomons et al. Citation2015). Future studies should investigate the effect of HIV infection status on the accuracies of the biosignatures. Although we evaluated the biomarkers in more children compared to our previous study, we were not able to investigate the accuracy of the biosignatures in a training and a test-set separately, but rather performed leave-one-out cross validation. Thus, further evaluations of the biomarkers are required in larger studies. Despite this limitation, we were able to confirm the diagnostic potential of candidate biomarkers and the biosignatures that were identified in previous studies (Visser et al. Citation2015, Manyelo et al. Citation2019a). The other main limitation was the few participants with meningitis of non-tuberculous aetiology. In future studies aimed at further validation and refinement of the biosignatures described in this study, more children with confirmed TBM and non-tuberculous meningitis, and those who are HIV infected should be included. Furthermore, the accuracy of the biosignatures in children from other geographical regions should be investigated in such studies. To our knowledge, target product profiles have not been set for the diagnosis of TBM in children, and thus benchmarks for diagnosis of EPTB in adults as set by the WHO were used to assess the biosignatures in this study. WHO TPP criteria set for diagnosis of TBM in children could aid in benchmarking the performance of the biosignatures specifically in the paediatric population.
In conclusion, we showed the replicability of previously identified three- and four-marker CSF biosignatures, which showed promise and approached the WHO’s target product profile benchmarks set for extrapulmonary TB in adults, in the current study. We furthermore, identified alternative new biosignatures with encouraging accuracy for the diagnosis of TBM. Our findings are promising for the future development of a simple POC or bedside test for the rapid diagnosis of TBM in children and warrant further evaluation in larger studies. Other promising biomarkers that showed potential individually or that were included in alternative biosignatures may be used for the improvement of the accuracy of such a bedside test during development, and should be included in future childhood TBM biomarker validation studies.
Competing interests
NC, CM, GW, and RS are listed as inventors on an International Patent Application entitled ‘Cerebrospinal fluid and blood-based biomarkers for the diagnosis of tuberculosis meningitis’ (PCT/IB2019/054259), filing date: 23 May 2019. NC and GW are listed as inventors on another patent application entitled ‘Method for diagnosing tuberculous meningitis’ (PCT/IB2015/052751), filing date: 15 April 2015. These applications do not generate any royalties for the inventors.
Author contributions
NC, GW and RS conceptualised and designed the study, and put together the study team. RS, YK, CC enrolled the patients, collected and captured the clinical data, and classified the study participants. CC and YK collected and transported the specimen to the research laboratory. KS designed and managed the study database. VL was responsible for the administration and management of the project. KR and CM processed and stored the samples in the laboratory. CS, KR and CM performed the immunoassays. MK and CM carried out the statistical analyses. NC, MC and RS interpreted the data. CM wrote the initial draft of the manuscript, while NC and RS critically reviewed and edited the manuscript. All authors critically reviewed the manuscript for important intellectual content, provided approval for publication of the content, and agreed to be accountable for all aspects of the work.
Supplemental Material
Download MS Word (93 KB)Acknowledgments
We thank the support staff and all the study participants for their contribution to the project.
Disclosure statement
No potential conflict of interest was reported by the author(s).
Additional information
Funding
References
- Ahmad, R., et al., 2019. A rapid triage test for active pulmonary tuberculosis in adult patients with persistent cough. Science translational medicine, 11 (515), eaaw8287.
- Bahr, N.C., Nuwagira, E., et al., 2018. Diagnostic accuracy of Xpert MTB/RIF Ultra for tuberculous meningitis in HIV-infected adults: a prospective cohort study. The lancet. Infectious diseases, 18 (1), 68–75.
- Bahr, N.C., Halupnick, R., et al., 2018. Delta-like 1 protein, vitamin D binding protein and fetuin for detection of Mycobacterium tuberculosis meningitis. Biomarkers in medicine, 12 (7), 707–716.
- Bahr, N.C., and Boulware, D.R., 2014. Methods of rapid diagnosis for the etiology of meningitis in adults. Biomarkers in medicine, 8 (9), 1085–1103.
- Bahr, N.C., Meintjes, G., and Boulware, D.R., 2019. Inadequate diagnostics: the case to move beyond the bacilli for detection of meningitis due to Mycobacterium tuberculosis. Journal of medical microbiology, 68 (5), 755–760.
- Chegou, N.N., et al., 2016. Diagnostic performance of a seven-marker serum protein biosignature for the diagnosis of active TB disease in African primary healthcare clinic attendees with signs and symptoms suggestive of TB. Thorax, 71 (9), 785–794.
- Chen, Y., et al., 2016. HMGB1 level in cerebrospinal fluid as a complimentary biomarker for the diagnosis of tuberculous meningitis. SpringerPlus, 5 (1), 1775–1775.
- Chendi, B.H., et al., 2021. A plasma 5-marker host biosignature identifies tuberculosis in high and low endemic countries. Frontiers in immunology, 12, 608846.
- Chiang, S.S., et al., 2014. Treatment outcomes of childhood tuberculous meningitis: a systematic review and meta-analysis. The lancet. Infectious diseases, 14 (10), 947–957.
- Chin, J.H., et al., 2019. Xpert MTB/RIF ultra for detection of mycobacterium tuberculosis in cerebrospinal fluid. Journal of clinical microbiology, 57 (6), e00249–19.
- Cresswell, F.V., et al., 2020. Xpert MTB/RIF ultra for the diagnosis of HIV-associated tuberculous meningitis: a prospective validation study. The lancet infectious diseases, 20 (3), 308–317.
- Creswell, J., et al., 2014. Results from early programmatic implementation of Xpert MTB/RIF testing in nine countries. BMC infectious diseases, 14 (1), 2.
- Flier, M. v d., et al., 2004. Vascular endothelial growth factor and blood-brain barrier disruption in Tuberculous meningitis. The pediatric infectious disease journal, 23 (7), 608–613.
- Fluss, R., Faraggi, D., and Reiser, B., 2005. Estimation of the Youden Index and its associated cutoff point. Biom J, 47 (4), 458–472.
- Husain, N., et al., 2008. Vascular endothelial growth factor as a marker of disease activity in neurotuberculosis. Journal of infection, 56 (2), 114–119.
- Ingram, G., et al., 2012. Systemic complement profiling in multiple sclerosis as a biomarker of disease state. Multiple sclerosis journal, 18 (10), 1401–1411.
- Isaiah, S., et al., 2020. Overview of brain-to-gut axis exposed to chronic CNS Bacterial Infection(s) and a predictive urinary metabolic profile of a brain infected by Mycobacterium tuberculosis. Frontiers in neuroscience, 14, 296–296.
- Jacobs, R., et al., 2016a. Identification of novel host biomarkers in plasma as candidates for the immunodiagnosis of tuberculosis disease and monitoring of tuberculosis treatment response. Oncotarget, 7 (36), 57581–57592.
- Jacobs, R., et al., 2016b. Host biomarkers detected in saliva show promise as markers for the diagnosis of pulmonary tuberculosis disease and monitoring of the response to tuberculosis treatment. Cytokine, 81, 50–56.
- Karande, S., et al., 2005. Tuberculous meningitis and HIV. The indian journal of pediatrics, 72 (9), 755–760.
- Kim, J., et al., 2016. Procalcitonin as a diagnostic and prognostic factor for Tuberculosis Meningitis. Journal of clinical neurology, 12 (3), 332–339.
- Lawn, S.D., and Nicol, M.P., 2011. Xpert® MTB/RIF assay: development, evaluation and implementation of a new rapid molecular diagnostic for tuberculosis and rifampicin resistance. Future microbiology, 6 (9), 1067–1082.
- Li, N., et al., 2017. Association between C4, C4A, and C4B copy number variations and susceptibility to autoimmune diseases: a meta-analysis. Scientific reports, 7 (1), 42628.
- MacLean, E., et al., 2019. A systematic review of biomarkers to detect active tuberculosis. Nature microbiology, 4 (5), 748–758.
- Manyelo, C.M., et al., 2019a. Application of cerebrospinal fluid host protein biosignatures in the diagnosis of Tuberculous meningitis in children from a high burden setting. Mediators of inflammation, 2019, 1–11.
- Manyelo, C.M., et al., 2019b. Potential of Host Serum Protein Biomarkers in the Diagnosis of Tuberculous Meningitis in Children. Frontiers in pediatrics, 7, 376–376.
- Marais, S., et al., 2010. Tuberculous meningitis: a uniform case definition for use in clinical research. The lancet. Infectious diseases, 10 (11), 803–812.
- Matsuyama, W., et al., 2001. Expression of vascular endothelial growth factor in tuberculous meningitis. Journal of the neurological sciences, 186 (1–2), 75–79.
- Merle, N.S., et al., 2015. Complement system Part I – molecular mechanisms of activation and regulation. Frontiers in immunology, 6, 262.
- Misra, U.K., et al., 2013. Vascular endothelial growth factor in tuberculous meningitis. Int J Neurosci, 123 (2), 128–132.
- Misra, U.K., et al., 2010. A study of cytokines in tuberculous meningitis: clinical and MRI correlation. Neuroscience letters, 483 (1), 6–10.
- Mutavhatsindi, H., et al., 2021. Validation and optimization of host immunological bio-signatures for a point-of-care test for TB disease. Frontiers in immunology, 12, 607827.
- Nhu, N.T.Q., et al., 2014. Evaluation of GeneXpert MTB/RIF for diagnosis of tuberculous meningitis. Journal of clinical microbiology, 52 (1), 226–233.
- Patel, V.B., et al., 2013. Diagnostic Accuracy of Quantitative PCR (Xpert MTB/RIF) for Tuberculous Meningitis in a high burden setting: a prospective study. PLoS medicine, 10 (10), e1001536.
- Peng, T., et al., 2014. Detection of Delta-like 1 ligand for the diagnosis of tuberculous meningitis: an effective and rapid diagnostic method. J Int Med Res, 42 (3), 728–736.
- Pormohammad, A., et al., 2019. A systematic review and meta-analysis of the diagnostic accuracy of nucleic acid amplification tests for Tuberculous meningitis. Journal of clinical microbiology, 57 (6), e01113–18.
- Reshi, Z., et al., 2017. Cerebrospinal fluid procalcitonin as a biomarker of bacterial meningitis in neonates. Journal of perinatology, 37 (8), 927–931.
- Seddon, J.A., and Thwaites, G.E., 2019. Tuberculous meningitis: new tools and new approaches required. Wellcome open research, 4, 181.
- Solomons, R., et al., 2016. IMCI indicators of childhood TBM at primary health care level in the Western Cape Province of South Africa. The international journal of tuberculosis and lung disease, 20 (10), 1309–1313.
- Solomons, R.S., et al., 2015. Chest radiograph findings in children with tuberculous meningitis. The international journal of tuberculosis and lung disease, 19 (2), 200–204.
- Sudbury, E.L., et al., 2019. Mycobacterium tuberculosis-specific cytokine biomarkers for the diagnosis of childhood TB in a TB-endemic setting. Journal of clinical tuberculosis and other mycobacterial diseases, 16, 100102.
- Thwaites, G., et al., 2002. Diagnosis of adult tuberculous meningitis by use of clinical and laboratory features. The lancet, 360 (9342), 1287–1292.
- Visser, D.H., et al., 2015. Host immune response to tuberculous meningitis. Clin Infect Dis, 60 (2), 177–187.
- Wang, H., and Liu, M., 2021. Complement C4, infections, and autoimmune diseases. Frontiers in immunology, 12, 694928.
- Wei, T.-T., et al., 2016. Diagnostic accuracy of procalcitonin in bacterial meningitis versus nonbacterial meningitis: a systematic review and meta-analysis. Medicine, 95 (11), e3079.
- World Health Organization. 2021. Global tuberculosis report 2021. Geneva: World Health Organisation.
- World Health Organization. 2018. Roadmap towards ending TB in children and adolescents, second edition. Geneva: World Health Organization.
- World Health Organization, 2017. Next-generation Xpert® MTB/RIF Ultra assay recommended by WHO [online]. Available from: https://www.who.int/news/item/25-03-2017-next-generation-xpert-mtb-rif-ultra-assay-recommended-by-who [Accessed 8 Jun 2021].
- World Health Organization. 2014., High-priority target product profiles for new tuberculosis diagnostics: report of a consensus meeting. Geneva, Switzerland: World Health Organization.
- Xu, D., et al., 2015. Serum protein S100A9, SOD3, and MMP9 as new diagnostic biomarkers for pulmonary tuberculosis by iTRAQ-coupled two-dimensional LC-MS/MS. Proteomics, 15 (1), 58–67.