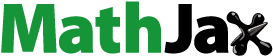
ABSTRACT
Unfounded child sexual abuse (CSA) allegations take investigative resources from real cases and have detrimental consequences for the people involved. The Finnish Investigative Instrument of Child Sexual Abuse (FICSA) supports investigators by estimating the probability of a CSA allegation being true based on the child’s background information. In the current study, we aimed at making FICSA resistant to deception. Two gender-specific questionnaires with FICSA questions and additional “trap” questions were constructed. The trap questions were designed to seem statistically related to CSA although they were not. Combining the answers of 278 real victims and 275 16–year-old students, instructed to simulate being CSA victims, we built a Naïve Bayes classifier that successfully separated the two groups (AUC = 0.91 for boys and 0.92 for girls). By identifying false allegations early in the investigation, authorities’ resources can be directed towards allegations that are more likely true, effectively helping actual CSA victims.
Introduction
The Finnish law defines child sexual abuse (CSA) according to the child’s age and level of development, the relationship between the perpetrator and the child, and the severity of the act. According to chapter 20, section 6 of the Finnish Criminal Code (540/2011), intercourse with a person under the age of 16 is considered to be CSA. Additionally, touching, or any other act aimed at sexual excitement or satisfaction that are harmful for the child’s development, are defined as CSA. However, an act is not considered punishable if both parties are approximately the same age and on the same level of development, and both participate voluntarily. If the perpetrator is the child’s parent or a person in a comparable position and lives in the same household, sexual acts are considered child sexual abuse until the age of 18 years. After 18 years of age, any sexual act involving children and parents, even if consensual, is criminally prosecuted, although not as CSA.
In the last decades, the number of CSA allegations reported to the authorities has increased, likely as the result of a recent legal obligation for all professionals working with children to report any suspicion of CSA (Child Welfare Act 2007, section 25; Hietamäki, Citation2012). The overall number of CSA cases shown to be substantiated has, however, decreased (Laaksonen et al., Citation2011). Relying on self-reports, the Finnish Child Victim Survey, that examined violence in children’s and adolescents’ lives in 1988, 2008 and 2013, has shown this descending trend in the prevalence of CSA better than the official count of reported cases. The survey indicates that approximately 4% of girls and 1% of boys in the ninth grade had reported CSA experiences in the 2013 compared with 14% for girls and 5% for boys in 1988 (Fagerlund et al., Citation2014).
Reporting CSA
Although the prevalence rate of CSA is decreasing, it is estimated that the number of CSA cases suspected by the child protection services has more than quadrupled since 1980 in Finland (Kauppinen et al., Citation2000). Yet, no direct conclusion can be made about the prevalence rate based on the number of reported CSA cases, as research has shown both under- and overreporting of CSA (Bruck et al., Citation1998; London et al., Citation2005).
Underreporting means that not all CSA cases are reported to the authorities, and the actual prevalence of CSA is therefore higher than the number of reported cases. A review by London et al. (Citation2005) revealed that 60–70% of the adults who reported experiencing sexual abuse in their childhood also never disclosed the abuse to anyone. Even if children disclose the abuse, research indicates that this often is to a peer (48%), rather than to an adult (26%) or the authorities (12%; Lahtinen et al., Citation2018). More encouraging, the review by London et al. (Citation2005) also showed that most children disclose abuse if they are asked in a direct and correct manner. This suggests that underreporting can originate in adults failing to facilitate disclosure of an abused child and/or fail to report it correctly to the authorities (Dhooper et al., Citation1991).
There are also issues with overreporting of CSA cases, meaning that allegations of CSA are made even when no abuse has taken place. It is estimated that at least 5–35% of CSA allegations are unfounded (Ceci & Bruck, Citation1995). Recently, Korkman et al. (Citation2019) studied the prevalence of unfounded CSA allegations in Finland, concluding that 25–40% of allegations are possibly unfounded. In Finland, professionals working with children are now legislatively obliged to report any suspicion of CSA to the police and child welfare services (Ellonen & Rantaeskola, Citation2016). This naturally increases the number of vague and indefinite allegations that are made, which, in turn, is likely to increase unfounded allegations (Laajasalo et al., Citation2018). Unfounded CSA allegations can also rise from, for example, adults’ erroneous interpretations of the child’s physical, behavioural, or psychological symptoms and characteristics (Korkman et al., Citation2019; Tadei et al., Citation2019), or from suggestive and leading discussions with the child (Hershkowitz, Citation2001; Korkman et al., Citation2014). Additionally, children themselves can occasionally report abuse, even when abuse has not taken place (Hershkowitz, Citation2001).
Underreporting increases the dark figure (i.e. the number of unrecognised CSA cases), which means that abuse is not exposed and the victim cannot receive help or justice, and that, in the worst case, the perpetrator will continue abusing the same child or other children. On the other hand, overreporting increases the risk of false positives (i.e. defining non-abused children as victims and innocent suspects as perpetrators), meaning that unfounded CSA allegations are processed by the authorities, resources are wasted on false cases, and there can be delays in investigating real cases.
Deception in children’s CSA reports
Vrij (Citation2000) defined deception as an intentional attempt to make another person believe in something that the teller knows is untrue. This definition excludes unintentionally wrongful statements that, for example, are due to poor memory or suggestive questioning (Hartwig & Santtila, Citation2008). In CSA cases, the child’s statement is particularly influential in the investigative process because the CSA victim is usually the only witness (Goodman et al., Citation2006; Herman, Citation2010). Currently, the general view is that children can be a reliable source for information in forensic settings (Koriat et al., Citation2001; Poole & Lamb, Citation2004). Nevertheless, it should not be disregarded that children are prone to suggestion, memory decay, and other cognitive limitations (Ceci et al., Citation2007; Poole & Lamb, Citation2004). Hence, the child’s age and cognitive development level should always be taken into account when hearing the child as a witness (Lahtinen, Citation2008; Poole & Lamb, Citation2004).
The capacity to consciously lie starts developing around four years of age when the child’s theory of mind (Premack & Woodruff, Citation1978) develops. By the age of eight, children acquire a deeper capacity for intentional deception, and use it in different situations (Talwar & Crossman, Citation2012). Nevertheless, reporting a false story requires skills that most young children have not yet obtained (e.g. good working memory, proficient executive functions, and inhibition; Talwar & Lee, Citation2008). Linguistic development and lying skills are also tightly connected, and the ability to express oneself continues to develop until the age of 18 (Paul, Citation2006).
Although believed to be relatively rare, children and adolescents can be deceitful in their witness reports and produce false allegations, just like adults (Jones & McGraw, Citation1987). This said, not a lot of research exists concerning intentionally fabricated reports by children (Talwar & Crossman, Citation2012) and data on the prevalence of deceiving behaviour in children and adolescents are limited. Nevertheless, some estimates say that false CSA allegations made by children comprise 2–4% of the investigated reports (see e.g. Jones & McGraw, Citation1987; Oates et al., Citation2000; Trocmé & Bala, Citation2005). The motives behind children’s lies appear to be similar to adults’ motives (Newton et al., Citation2000; Wilson et al., Citation2003), who tend to lie to avoid punishment, enhance their own social status, or protect and please others (Talwar & Crossman, Citation2012; Warneken & Orlins, Citation2015).
Investigation of CSA
In Finland, the police usually turn to a multidisciplinary team consisting of forensic psychologists, social workers, child psychiatrists, and other mental health professionals working in specialised units of forensic child and adolescent psychiatry to conduct the evaluation of a CSA case (Laajasalo et al., Citation2018). These forensic units collect background information, conduct the interview of the alleged child victim (when possible), and produce a forensic expert report (Ellonen & Rantaeskola, Citation2016). Often these case reports serve as expert testimony during court proceedings (Gratz & Orsillo, Citation2003).
Expert evaluations play a crucial role in the investigation of CSA allegations and should be of the highest quality possible. These evaluations are, however, demanding because experts must integrate complex and extensive information in their conclusions, with physical evidence often lacking. Consequently, there are several issues concerning the quality of these expert evaluations. Often experts do not follow evidence-based practice, and this can easily lead to erroneous conclusions about the allegation. Whenever possible, experts should base their decisions on factual (e.g. statistical) data to avoid these errors. Importantly, as evaluating statistical evidence is complicated (Edwards & von Winterfeldt, Citation1986), there is a genuine need for valid, scientifically supported assessment methods to assist experts when dealing with complex information in the decision-making process of CSA evaluations (Laajasalo et al., Citation2018). Evidence that cannot be accounted for statistically, for example due to limited research, still should be considered when drawing expert conclusions. In this case, it should be transparent to the end-user of the forensic evaluation which evidence was only clinically evaluated and which the possible biases on the expert’s side are. We argue, however, that also clinical evaluations performed on individual level refer to group-level data, in the sense that the clinicians’ ideas about the meaning of any clue will necessarily be based on their inferences made by observing other cases.
Base-rate probabilities should be considered in the evaluation of a CSA allegation (Proeve, Citation2009; Wood, Citation1996). Base-rates give an initial probability of the abuse having occurred, and this probability should be updated as new evidence becomes available. Bayes’ theorem is well-suited for adjusting base-rates in this way (Herman, Citation2005; Wood, Citation1996). By obtaining an accurate and updated base-rate probability of CSA at an early stage of an investigation, investigators can organise and proritize the CSA allegations according to their probability of having actually occurred (Tadei et al., Citation2019b). This prioritisation of CSA cases is important to investigation success, as time passing between the alleged event and the investigation negatively affects the reliability and quality of the child’s report (Shrimpton et al., Citation1998; Tucker et al., Citation1990).
The Finnish Investigative Instrument of Child Sexual Abuse (FICSA)
Tadei et al. (Citation2019) created a computerised decision-making support tool to help CSA experts in dealing with statistical information. Using modern machine learning, the Finnish Investigative Instrument of Child Sexual Abuse (FICSA, Tadei et al., Citation2019) is a classifier based on Bayesian statistics that calculates the probability of a particular child having been a victim of CSA. To calculate this probability, FICSA combines observations of 42 risk and protective factors (25 factors only for girls, 14 only for boys, and 3 for both genders) of CSA. These factors are based on a large representative sample of over 11,000 Finnish children and adolescents’ reports (see Ellonen et al., Citation2013). None of these 42 variables are about the alleged CSA event itself, but instead about the child’s background (e.g. relationships with peers and family, substance abuse, experiences with crime and violence; for a detailed description of FICSA, see Tadei et al., Citation2019). As the information needed for FICSA is gathered by a questionnaire that can be answered by the child alone, this can decrease the known risks of verbal interaction between the interviewer and the child, such as suggestive questioning or improper language use (see e.g. Korkman et al., Citation2008). As a semi-automatic statistical instrument, FICSA integrates information objectively and helps limit the influence of cognitive biases (Tadei et al., Citation2019a, Citation2019b).
A simulation study by Tadei et al. (Citation2019) showed that FICSA has promising diagnostic utility as a classifier tool in estimating the probability of CSA having occurred. According to this study, FICSA showed high discriminatory effectiveness for both boys and girls (Area Under the Curve, AUC = .97 for boys and AUC = .88 for girls). In another study, Tadei et al. (Citation2019b) examined forensic experts’ ability to use FICSA in mock scenarios of CSA and whether FICSA improved their estimates of the probability for CSA and found that FICSA can aid CSA investigators by helping integrate evidence correctly.
One current limitation of FICSA is that it can potentially be misled by wilful false allegations. To the extent that it is apparent how the included questions are related to CSA, they could be answered deceptively, and FICSA cannot identify information that the child gives as true or false. FICSA can, threrefore,not identify children who might be deceiving and report a false allegation. Because of this, it is important to include a feature into FICSA that identifies false CSA allegations.
The current study
To address the current limitations of FICSA (Tadei et al., Citation2019) and the challenges posed by false CSA allegations, we aimed to enhance FICSA by developing a feature to help separate children reporting a false allegation from those actually abused. As the information for FICSA is gathered by a questionnaire, the goal was to include new questions which could serve as deception detectors. To do this, we asked our participants to answer a questionnaire which included the standard questions for FICSA, and an additional selection of “trap” questions gathered from the same victimisation study FICSA is based on (see Ellonen et al., Citation2013). These trap questions were not statistically related to CSA but could be perceived as being related to CSA due to commonly held beliefs about CSA victims. Participants were instructed to answer the questionnaire by imagining how a CSA victim would answer, that is, simulating being CSA victims.
We hypothesised that by combining the answers of real CSA victims identified from the victimisation study and the answers of simulators in our sample, it would be possible to find a set of questions that would let us discriminate between real CSA victims and CSA simulators with a high accuracy. We also built a model that could predict the probability of deception. For each gender, we built three different Naïve Bayes classifiers, including either (1) FICSA and trap questions together, (2) only FICSA questions, or (3) only trap questions. We expected that FICSA and trap questions together would be the most accurate classifier.
Methods
Participants
Data were gathered in five different Finnish high schools situated in Helsinki, Tampere, Turku, and Varkaus from first year students aged 16. Out of the 30 schools that were contacted, these were first to give their consent. To have approximately the same number of simulators as there were real CSA victims (n = 278) in the original victimisation study, data collection was stopped when an appropriate number of participants was reached. The questionnaire was answered, individually and on paper, by 277 first-year high school students in the classrooms and under the supervision of their teacher and a researcher. Two participants were on their second year of high school, and their responses were excluded from the final dataset. The final sample of simulators thus consisted of 275 participants. Of the participants, 60.7% (n = 167) were girls and 39.3% (n = 108) were boys. The final dataset used in the analyses was composed of the 275 simulators of the new data collection and 278 actual CSA victims from the original data file (Tadei et al., Citation2019) and consisted of 553 respondents altogether.
Ethical permission
The present study was first approved by the Institutional Review Board for Research Ethics at Åbo Akademi University, then by each City Administration, and finally by each of the included schools. Before participation, all respondents signed a consent form which stated the topic and content of the study, policies about the anonymity, and the participants’ right to withdraw from the study at any point. All participants were also given suitable contact information in case they needed to discuss any experiences concerning which the study could have triggered memories.
Questionnaire
The data collection employed two different questionnaires, one for boys and one for girls. The boys’ questionnaire consisted of 15 questions, and the girls’ questionnaire consisted of 26 questions. Each questionnaire was composed by a selection of the gender-specific questions from FICSA (8 for boys, 23 for girls) and by a number of trap questions (7 for boys, 3 for girls). We did not ask all the original questions from FICSA because some of them could not be answered by everyone since they required certain initial conditions to be fulfilled (e.g. having siblings, or having been victim of robbery or theft). To build a tool that could be applied to as many cases as possible, we retained only the questions that could be asked of children coming from any family situation and that did not require specific life experiences.
As with the FICSA questions, trap questions were not about the alleged CSA event itself but about the child’s background and previous experiences with crime, violence, and relationships. The trap questions were selected among the 903 variables used in the extensive data collection on which FICSA is based (see Ellonen et al., Citation2013). To be considered possible traps, the questions had to seem but not be statistically related to CSA (i.e. non-significant chi-square relationship with CSA). For example, boys answering “Yes” to “Have you ever used drugs?” might seem to make the CSA report sound more realistic, but drug use is actually not statistically related to CSA for boys. Two experts in forensic psychology and one psychology student selected, individually, all the questions that theoretically could work as traps. Finally, only trap questions chosen by all the three researchers became part of the two questionnaires used in the actual data collection. In the questionnaires, the FICSA questions and the trap questions were all put together in the same order as they were in the original data file of the victimisation study (see Ellonen et al., Citation2013). All included questions had answer options to choose from, and the participants were instructed to choose only one.
Additionally, three questions were asked from both boys and girls at the end of the questionnaire to identify real CSA victims among the new participants. CSA was defined as any sexual experience from receiving a proposal to do something sexual to sexual penetration of a person below the age of 17, by an offender that is at least five years older. Participants who identified as CSA victims according to this definition were excluded from the analyses, since they did not fit the role of a simulator. However, both participants that reported having experienced and not having experienced abuse in real life might not have declared the truth. Hence, we cannot be sure we perfectly separated real CSA victims from non-victims. Altogether 23 participants were identified as CSA victims in our sample. Of all the boys, 1.9% (n = 2) reported CSA experiences and of all the girls, 12.6% (n = 21) reported having experiences of CSA.
Statistical analyses
Classifier. A classifier is a statistical machine learning tool that provides the probability of a certain observation belonging to a precise class. In the current study, we chose the Naïve Bayes (NB) classifier to keep the same approach used for FICSA (see Tadei et al., Citation2019) and to guarantee a high interpretability. Just as with many other classifiers, the accuracy of a NB classifier directly depends on how informative the predictors are in discriminating between the different classes of the response variable. Furthermore, a NB classifier relies on the assumption that all the predictors are independent of each other, making the graphical representation of these models easy enough through the use of Bayesian networks (Fenton & Neil, Citation2012; Koller & Friedman, Citation2009). As predictors to estimate the CSA risk for boys and girls can be different (see Tadei et al., Citation2019), we created gender-specific classifiers. We fitted three different classifiers for each gender: (1) FICSA and trap questions together, (2) only the FICSA questions, and (3) only the trap questions. Among these three different classifiers, the one with the smallest test error rate would also be the most accurate.
Missing values. We asked participants to answer all the questions as they thought a real CSA victim would have. Hence, the number of unanswered questions was almost null. Contrariwise, the children who answered the questionnaire from 2013 were real CSA victims and did not answer questions that were not applicable to their real-life experiences. To balance the number of missing answers and keep the data as real as possible, we deleted entries from the 2018 data, rather than imputing missing values to the 2013 dataset. We randomly picked the entries to delete and chose the right amount in order to have the same percentage of missing answers for each question.
Feature selection. The feature selection is a dimension reduction procedure. We used it to identify the smallest set of informative and non-redundant features that would lead to the most accurate classifier possible, given the available data. We applied this technique on all six groups of questions. shows, for each gender and classifier, the number of predictors before and after the feature selection.
Table 1. Number of predictors for the six classifiers, before and after feature selection.
Cross-validation. To estimate the out-of-sample accuracy of the classifier, meaning its performance on children that were not part of the data collection, we implemented a repeated k-fold cross-validation procedure, with four folds and five repetitions. Having four folds means that our dataset was divided into four equal parts (a, b, c, and d). Three of them were used as a training set and the fourth, say d, as a test set. Hence, d was used to estimate the accuracy of the classifier. We then fitted new classifiers on the remaining three combinations of training sets and measured the accuracy using first c, then b, and finally a. To have an even more reliable measure of the average accuracy of the classifier, this entire procedure was repeated five times, changing the composition of a, b, c, and d each time. In the end, the final performances of all our built classifiers were the average accuracy measured on 20 different test sets.
Receiver Operating Characteristics. We used the Receiver Operating Characteristics (ROC) and the Area Under the Curve (AUC) to evaluate and graphically represent the classifiers’ performance. A ROC is a two-dimensional plot that has the true positive rate (TPR) on the y-axis and the false positive rate (FPR) on the x-axis, with both axes on a scale 0–1. Each point of the curve is created by varying the cut-off to assign an observation to the positive class. The AUC is a value between 0 and 1 that indicates the probability for a positive observation to receive a higher probability from the classifier than a negative observation. Simply put, AUC value provides information about how well the model can distinguish between different classes. Chance level is represented by an AUC of 0.5. Depending on the context, a good classifier should reach an AUC higher than 0.5, but a higher value is needed for practical utility in a forensic context. The use of AUC as a measure of diagnostic accuracy has been recommended in forensic psychology (Mossman, Citation1994; Rice & Harris, Citation2005; Swets et al., Citation2000).
Results
Three separate NB classifiers were built for both genders to predict whether the CSA allegation made by the child is true or false. We fitted three models for each gender which used different starting sets of predictors: both FICSA and trap questions together, only FICSA questions, and only trap questions. Each of these classifiers are presented as ROC curves and the AUC values, them being the average performance of the 20 different training sets measured with the cross-validation procedure. shows the mean ROC curves and the AUC values for the NB classifiers for both genders. The classifier that used both FICSA and trap questions as predictors had the highest AUC value, ∼.91 for boys and ∼.92 for girls, whereas AUC values for both genders were under .90 for the other two classifiers with only FICSA or only trap questions.
Figure 1. ROC curves and AUC values (mean ± 95% confidence interval) for the three classifiers for boys (a) and for girls (b). The mean ± SD of the classifier with highest AUC value is represented on the ROC curves for both genders. ROC: receiver operating characteristic; AUC: area under the curve; SD: standard deviation.
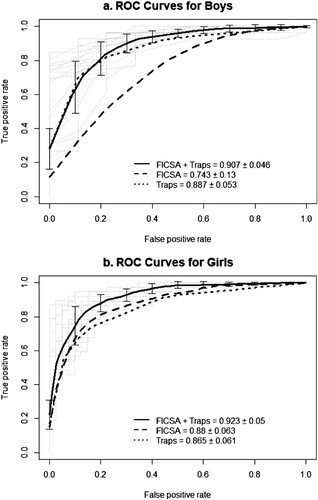
To statistically test the differences within gender between the three classifiers, we used a one-way ANOVA with Tukey post-hoc tests. We were particularly interested comparing the classifier with FICSA and trap questions to the two other classifiers, since it was hypothesised that this classifier would be the most efficient. For boys, there was a statistically significant difference between the three classifiers, F(2, 57) = 37.43, p < .001, = 0.57. The accuracy of the FICSA only classifier was lower than the other two (p < .001), which were not significantly different from each other (p = .598). Also for girls, there was a statistically significant difference between the three classifiers, F(2, 57) = 14.45, p < .001,
= 0.34. The accuracy of the classifier with both FICSA and trap questions outperformed the other two (p < .001). The classifier with only FICSA questions and the classifier with only traps did not differ significantly (p = .419).
Discussion
The goal of the current study was to add a deception resistant feature to FICSA, so that it could predict the probability of the CSA allegation being false. Previously, FICSA only estimated the probability of CSA being true and could not be used to identify whether the information used is truthful or not. Therefore, we aimed to find a selection of questions that could work as detectors of possible deception when using FICSA in CSA allegations. The results of the present study showed that the selection of these “trap” questions was successful, and that we were able to identify a set of questions that could predict the truthfulness of a CSA allegation. The classifiers built had excellent diagnostic utility in both genders.
Discriminatory effectiveness
Three different classifiers were built for each gender using different sets of questions as predictors. For boys, the accuracy of the classifiers with both FICSA and trap questions and with only traps was statistically equivalent, meaning that these two classifiers would be equally accurate in predicting false allegations based on our sample, and that both would outperform the FICSA only classifier. For girls, instead, the classifier with both FICSA and trap questions outperformed the other two. These results showed, for both genders, how the presence of trap questions made it possible to identify wilfully false CSA reports.
High AUC values do not translate automatically to high performance in real applications. Depending on the resources available, investigators will have to accept a certain percentage of false positives and false negative outcomes. These quantities, as shown by the ROC curves, are negatively correlated and, by varying the cut-off used to attribute an observation to one of the two classes, investigators will be able to choose which of the two errors to decrease and which to increase. Lowering the cut-off will get us a higher true positive rate (i.e. more simulators are correctly identified), but also a higher false positive rate (i.e. more real victims are classified as simulators). A relatively low cut-off could be useful, for example, if the authorities do not have sufficient resources on hand, as the FICSA then can effectively root out simulators. The higher the chosen cut-off is, the more of real victims will be identified as such, but then more simulators will be incorrectly identified also as real victims.
The participants of this study were chosen to be first year high school students, since high school is usually started at the age of 16 in Finland. CSA is a sensitive topic, and this age group was chosen because they were allowed by the schools to consent to participating in the study without informing their parents. Nevertheless, the minimum age for using FICSA is recommended to be 12 years, because this is the youngest participants in the original sample FICSA is based on (see Ellonen et al., Citation2013). Additionally, since the ability to form a false CSA allegation is generally acquired only by early adolescence, there is little need to identify wilful false allegations in younger children. It should also be noted that this tool was created only to identify wilful false CSA allegations. Hence, it might not perform equally well in identifying unfounded allegations that rise from false memories, suggestion, or misinterpretations. Finally, FICSA cannot serve as a screening tool to identify possible CSA cases yet unreported. FICSA’s false negative rate is relatively low, meaning that there is a low probability to wrongly define a child as a CSA victim. However, in case FICSA was used on all the children between 12 and 16 years, the absolute number of false negative cases would be so high to be impossible to investigate. We argue that FICSA is best suited for CSA allegations that adolescents are reporting themselves.
Participants were asked questions at the end of the questionnaire to identify and exclude participants with real CSA experiences and who would thus not fit the role of a simulator. In our sample, the 1.9% prevalence rate of CSA for boys go along with the previous prevalence rates in Finland, but the 12.6% prevalence rate for girls is high compared to population-based prevalence rates (see e.g. Fagerlund et al., Citation2014; Laaksonen et al., Citation2011).
Limitations
When using a machine learning approach, the issue of overfitting is important to consider. Overfitting is a common problem in the area of machine learning, and it can easily lead to over-optimistic results (Chicco, Citation2017). This problem occurs when the model does not generalise well to new data, although the model has performed well on the original data. Even though we used a cross-validation approach to reduce the risk of over-fitting, it is still unclear how well our classifiers would work on completely new samples.
Another relevant limitation concerns the ecological validity. When it comes to studying children’s lying in a forensic context, an issue is that the research conditions and experiments are not fully comparable to those in actual legal settings, and thus lack ecological validity (Talwar & Crossman, Citation2012). It is challenging to study deception, and identical conditions to what children might face when participating in legal processes are hard to create, at least due to ethical reasons (Talwar & Crossman, Citation2012). The setting of the current study lacked the emotional and social pressure which might be present when deceiving in a real police investigation. It is likely that when reporting a false allegation of CSA and becoming part of a police investigation, the pressure of getting caught affects the performance on filling out the FICSA questionnaire.
Additionally, participants in the simulator sample were asked to only imagine the situation in question and it is impossible to know participants’ thought process when answering the questionnaire. For example, an unmotivated participant could have just answered the questionnaire without giving any thought to it. Trying to keep all the requirements in mind and using imagination when filling out the questionnaire is admittedly difficult and replying to the questionnaire would inevitably be challenging.
Finally, not knowing the prevalence of false reports with certainty, we chose to have a 50–50 ratio between real victims and simulator for girls. For boys, instead, the number of real victims was too small to properly train a classifier, so we used a 25–75 ratio. These artificial base-rates should be corrected as soon as proper scientific data become available. Currently, a solution is to increase the threshold probability used to define a child as simulator. This correction would then decrease the number of reports considered false, and, consequently, the number of false negative decisions.
Future directions
More research is still needed before being able to validate FICSA and to implement its use in the real CSA investigations. The next step for the enhanced version of FICSA would be to test it on different samples and possibly on real CSA cases as well. To be able to validate and test FICSA on real CSA cases, we would first need cases with strong evidence that prove the alleged event having occurred. By testing FICSA on these cases we would be able to measure true positive and false negative rates. However, to be able to acquire the rates of false positives (i.e. defining the allegation as true, when it is false) and true negatives (i.e. defining the allegation as false, when it is false) for our tool is quite impossible. We would not be able to see how the tool would perform in real life when the allegation is false, since it is much harder to prove a case being false than true.
Conclusions
In forensic settings, deception is an important topic since legal processes can have serious consequences for all parties involved. Lie-detection is, therefore, of utmost relevance also in CSA cases in which physical evidence and eyewitnesses are often missing. After adding a deception resistant feature to it, FICSA can be considered an even more efficient tool for decision-making of CSA investigations. FICSA offers a statistical approach to be used in the complex CSA cases and can address the issues present at least to some extent. Although FICSA still possesses some important limitations, we argue that it could be used as a good starting point for a CSA investigation.
Disclosure statement
No potential conflict of interest was reported by the author(s).
References
- Bruck, M., Ceci, S. J., & Hembrooke, H. (1998). Reliability and credibility of young children’s reports: From research to policy and practice. American Psychologist, 53(2), 136–151. https://doi.org/https://doi.org/10.1037/0003-066X.53.2.136
- Ceci, S. J., & Bruck, M. (1995). Jeopardy in the courtroom: A scientific analysis of children’s testimony. American Psychological Association.
- Ceci, S. J., Kulkofsky, S., Klemfuss, J. Z., Sweeney, C. D., & Bruck, M. (2007). Unwarranted assumptions about children’s testimonial accuracy. Annual Review of Clinical Psychology, 3(1), 311–328. https://doi.org/https://doi.org/10.1146/annurev.clinpsy.3.022806.091354
- Chicco, D. (2017). Ten quick tips for machine learning in computational biology. BioData Mining, 10(1), 1–17. https://doi.org/https://doi.org/10.1186/s13040-017-0155-3
- Dhooper, S. S., Royse, D. D., & Wolfe, L. C. (1991). A statewide study of the public attitudes toward child abuse. Child Abuse and Neglect, 15(1–2), 37–44. https://doi.org/https://doi.org/10.1016/0145-2134(91)90088-U
- Edwards, W., & von Winterfeldt, D. (1986). On cognitive illusions and their implications. Southern California Law Review, 59(2), 401–451.
- Ellonen, N., Fagerlund, M., Kääriäinen, J., Peltola, M., & Sariola, H. (2013). Child Victim Survey 2013 [dataset]. Version 1.0 (2015-03-16). http://urn.fi/urn:nbn:fi:fsd:T-FSD2943
- Ellonen, N., & Rantaeskola, S. (2016). Lapsiin kohdistuvien väkivalta- ja seksuaali rikosepäilyjen tutkinta.
- Fagerlund, M., Peltola, M., Kääriäinen, J., Ellonen, N., & Sariola, H. (2014). Lasten ja nuorten väkivaltakokemukset 2013: Lapsiuhritutkimuksen tuloksia. Poliisiammattikorkeakoulu.
- Fenton, N., & Neil, M. (2012). Risk assessment and decision analysis with Bayesian networks. In Risk assessment and decision analysis with Bayesian networks. https://doi.org/https://doi.org/10.1201/b21982
- Goodman, G. S., Luten, T. L., Edelstein, R. S., & Ekman, P. (2006). Detecting lies in children and adults. Law and Human Behavior, 30(1), 1–10. https://doi.org/https://doi.org/10.1007/s10979-006-9031-2
- Gratz, K. L., & Orsillo, S. M. (2003). Scientific expert testimony in CSA cases: Legal, ethical, and scientific considerations. Clinical Psychology: Science and Practice, 10(3), 358–363. https://doi.org/https://doi.org/10.1093/clipsy/bpg035
- Hartwig, M., & Santtila, P. (2008). Valehtelun tunnistaminen ja epäillyn syyllisyyden arviointi poliisikuulustelussa. In Oikeuspsykologia (pp. 432-455). Edita Publishing Oy.
- Herman, S. (2005). Improving decision making in forensic child sexual abuse evaluations. Law and Human Behavior, 29(1), 87–120. https://doi.org/https://doi.org/10.1007/s10979-005-1400-8
- Herman, S. (2010). The role of corroborative evidence in child sexual abuse evaluations. Journal of Investigative Psychology and Offender Profiling, 7(3), 189–212. https://doi.org/https://doi.org/10.1002/jip.122
- Hershkowitz, I. (2001). A case study of child sexual false allegation. Child Abuse and Neglect, 25(10), 1397–1411. https://doi.org/https://doi.org/10.1016/S0145-2134(01)00274-5
- Hietamäki, J. (2012). Child protection in Finland. In Child protection systems: An international comparison of “good practice examples” of five countries (Australia, Germany, Finland, Sweden, United Kingdom) with recommendations for Switzerland (pp. 182–225). https://www.researchgate.net/publication/287735969_Child_protection_in_Finland
- Jones, D. P. H., & McGraw, J. M. (1987). Reliable and fictitious accounts of sexual abuse to children. Journal of Interpersonal Violence, 2(1), 27–45. https://doi.org/https://doi.org/10.1177/088626087002001002
- Kauppinen, S., Sariola, H., & Taskinen, S. (2000). Child sexual abuse suspicions quadrupled in 15 years. Stakes.
- Koller, D., & Friedman, N. (2009). Probabilistic graphical models: Principles and Techniques. MIT Press.
- Koriat, A., Goldsmith, M., Schneider, W., & Nakash-Dura, M. (2001). The credibility of children’s testimony: Can children Control the accuracy of their memory reports? Journal of Experimental Child Psychology, 79(4), 405–437. https://doi.org/https://doi.org/10.1006/jecp.2000.2612
- Korkman, J., Antfolk, J., Fagerlund, M., & Santtila, P. (2019). The prevalence of unfounded suspicions of child sexual abuse in Finland. Nordic Psychology, 71(1), 39–50. https://doi.org/https://doi.org/10.1080/19012276.2018.1470554
- Korkman, J., Juusola, A., & Santtila, P. (2014). Who made the disclosure? Recorded discussions between children and caretakers suspecting child abuse. Psychology, Crime and Law, 20(10), 994–1004. https://doi.org/https://doi.org/10.1080/1068316X.2014.902455
- Korkman, J., Santtila, P., Drzewiecki, T., & Sandnabba, N. K. (2008). Failing to keep it simple: Language use in child sexual abuse interviews with 3-8-year-old children. Psychology, Crime and Law, 14(1), 41–60. https://doi.org/https://doi.org/10.1080/10683160701368438
- Laajasalo, T., Korkman, J., Pakkanen, T., Oksanen, M., Tuulikki, L., Peltomaa, E., & Aronen, E. T. (2018). Applying a research-based assessment model to child sexual abuse investigations: Model and case descriptions of an expert center. Journal of Forensic Psychology Research and Practice, 18(2), 177–197. https://doi.org/https://doi.org/10.1080/24732850.2018.1449496
- Laaksonen, T., Sariola, H., Johansson, A., Jern, P., Varjonen, M., Von Der Pahlen, B., … Santtila, P. (2011). Changes in the prevalence of child sexual abuse, its risk factors, and their associations as a function of age cohort in a Finnish population sample. Child Abuse and Neglect, 35(7), 480–490. https://doi.org/https://doi.org/10.1016/j.chiabu.2011.03.004
- Lahtinen, H. M. (2008). Todistajapsykologia – Lapsitodistajat. In Oikeuspsykologia (pp. 176–198). Edita Publishing Oy.
- Lahtinen, H. M., Laitila, A., Korkman, J., & Ellonen, N. (2018). Children’s disclosures of sexual abuse in a population-based sample. Child Abuse and Neglect, 76(October 2017), 84–94. https://doi.org/https://doi.org/10.1016/j.chiabu.2017.10.011
- London, K., Bruck, M., Ceci, S. J., & Shuman, D. W. (2005). Disclosure of child sexual abuse: What does the research tell us about the ways that children tell? Psychology, Public Policy, and Law, 11(1), 194–226. https://doi.org/https://doi.org/10.1037/1076-8971.11.1.194
- Mossman, D. (1994). Assessing predictions of violence: Being accurate about accuracy. Journal of Consulting and Clinical Psychology, 62(4), 783–792. https://doi.org/https://doi.org/10.1037/0022-006X.62.4.783
- Newton, P., Reddy, V., & Bull, R. (2000). Children’s everyday deception and performance on false-belief tasks. British Journal of Developmental Psychology, 18(2), 297–317. https://doi.org/https://doi.org/10.1348/026151000165706
- Oates, R. K., Jones, D. P. H., Denson, D., Sirotnak, A., Gary, N., & Krugman, R. D. (2000). Erroneous concerns about child sexual abuse. Child Abuse and Neglect, 24(1), 149–157. https://doi.org/https://doi.org/10.1016/S0145-2134(99)00108-8
- Paul, R. (2006). Language disorders from infancy through adolescence: Assessement & intervention. In Language disorders from infancy through adolescence: Assessement & intervention. https://doi.org/https://doi.org/10.1016/c2015-0-04093-x
- Poole, D. A., & Lamb, M. E. (2004). Investigative interviews of children: A guide for helping professionals. American Psychological Association.
- Premack, D., & Woodruff, G. (1978). Does the chimpanzee have a theory of mind? Behavioral and Brain Sciences, 1(4), 515–526. https://doi.org/https://doi.org/10.1017/S0140525X00076512
- Proeve, M. (2009). Issues in the application of Bayes’ theorem to child abuse decision making. Child Maltreatment, 14(1), 114–120. https://doi.org/https://doi.org/10.1177/1077559508318395
- Rice, M. E., & Harris, G. T. (2005). Comparing effect sizes in follow-up studies: ROC area, Cohen’s d, and r. Law and Human Behavior, 29(5), 615–620. https://doi.org/https://doi.org/10.1007/s10979-005-6832-7
- Shrimpton, S., Oates, K., & Hayes, S. (1998). Children’s memory of events: Effects of stress, age, time delay and location of interview. Applied Cognitive Psychology, 12(2), 133–143. https://doi.org/https://doi.org/10.1002/(SICI)1099-0720(199804)12:2<133::AID-ACP502>3.0.CO;2-E
- Swets, J. A., Dawes, R. M., & Monahan, J. (2000). Psychological science can improve diagnostic decisions. Psychological Science in the Public Interest, 1(1), 1–26. https://doi.org/https://doi.org/10.1111/1529-1006.001
- Tadei, A., Pensar, J., Corander, J., Finnilä, K., Santtila, P., & Antfolk, J. (2019). A Bayesian decision-support tool for child sexual abuse assessment and investigation. Sexual Abuse: Journal of Research and Treatment, 31(4), 374–396. https://doi.org/https://doi.org/10.1177/1079063217732791
- Tadei, A., Santtila, P., & Antfolk, J. (2019a). The police use of background information related to alleged victims in mock evaluations of child sexual abuse. Journal of Police and Criminal Psychology, 35, 414–421. https://doi.org/https://doi.org/10.1007/s11896-019-9312-6
- Tadei, A., Santtila, P., & Antfolk, J. (2019b). The usability and effectiveness of the Finnish investigative instrument of child sexual abuse in mock evaluations. Nordic Psychology, 72(2), 127–145. https://doi.org/https://doi.org/10.1080/19012276.2019.1662323
- Talwar, V., & Crossman, A. M. (2012). Children’s lies and their detection: Implications for child witness testimony. Developmental Review, 32(4), 337–359. https://doi.org/https://doi.org/10.1016/j.dr.2012.06.004
- Talwar, V., & Lee, K. (2008). Social and cognitive correlates of children’s lying behavior. Child Development, 79(4), 866–881. https://doi.org/https://doi.org/10.1111/j.1467-8624.2008.01164.x
- Trocmé, N., & Bala, N. (2005). False allegations of abuse and neglect when parents separate. Child Abuse and Neglect, 29(12), 1333–1345. https://doi.org/https://doi.org/10.1016/j.chiabu.2004.06.016
- Tucker, A., Mertin, P., & Luszcz, M. (1990). The effect of a repeated interview on young children’s eyewitness memory. Australian & New Zealand Journal of Criminology, 23(2), 117–124. https://doi.org/https://doi.org/10.1177/000486589002300204
- Vrij, A. (2000). Detecting lies and deceit: The psychology of lying and implications for professional practice. Wiley.
- Warneken, F., & Orlins, E. (2015). Children tell white lies to make others feel better. British Journal of Developmental Psychology, 33(3), 259–270. https://doi.org/https://doi.org/10.1111/bjdp.12083
- Wilson, A. E., Smith, M. D., & Ross, H. S. (2003). The nature and effects of young children’s lies. Social Development, 12(1), 21–45. https://doi.org/https://doi.org/10.1111/1467-9507.00220
- Wood, J. M. (1996). Weighing evidence in sexual abuse evaluations: An introduction to Bayes’s theorem. Child Maltreatment, 1(1), 25–36. https://doi.org/https://doi.org/10.1177/1077559596001001004