Abstract
As the population of the world gets older, cognitive and mental health decline in older adults has become one of the most pressing scientific problems of the 21st century. It has been well-established that multiple pathways exist that can lead to future cognitive decline and the development of age-related mental health conditions. Making matters more complicated is the fact that aging is not a homogenous or simple process; rather, each person brings a diverse set of circumstances to the table, leading to differences in biologically aging. Research is needed to identify how the intersection of environmental and biological factors may protect older adults or put them at future risk for cognitive decline and the development of age-related mental health conditions. Furthermore, each potential factor may impact different domains of cognition and mental health or may impact individuals differentially based on genetic, environmental, social, emotional, and behavioral factors. Neuroimaging is one key tool that can be used to discover and assess markers of cognitive and mental health. The goal of this special issue is to expand the science on how biological markers can aid the understanding of aging and mental health through the lens of the individual from an international perspective, representing Germany, Taiwan, Thailand, the United Kingdom, and the United States. Common themes from these articles are used to support the new Seed and Soil Model of Neurocognitive Disorders.
Introduction
The increased attention to the aging population has also given rise to an increased focus on age-related neurocognitive disorders such as Alzheimer’s disease. This concern is exemplified by the 21st Century Cures Act in the United States that was recently signed into law by President Barack Obama. This law was designed, in part, to provide funding for research involved in Precision Medicine and the BRAIN initiatives. These initiatives recognize that combatting cognitive decline and the onset of mental illnesses might best be achieved by early detection and prevention methods. While a surge of clinical trials has attempted to stop or reverse pathology in those with the disorders such as Alzheimer’s disease, these trials have failed (Schneider et al., Citation2014). Ultimately, these failures suggest that preventing rather than treating such neurocognitive disorders might be the only way to effectively combat them. Targeting people who will eventually develop neurocognitive disorders requires understanding how normal aging differs from pathological aging. These two different trajectories, however, are difficult to disentangle through cognitive or emotional measures because preclinical stages of a disorder, by definition, do not carry the hallmark features of the disorder.
Brain measures, however, can reveal alterations sometimes decades before cognitive or emotional changes such as Alzheimer’s disease (Sperling et al., Citation2011; Jack et al., Citation2013), thus placing these tools at the forefront of research investigating early detection of disorders. By detecting individuals at preclinical stages, individuals can be targeted for treatments once they become available to potentially prevent disorders before symptoms ever manifest (Sperling et al., Citation2014). While this progress is promising, more research is needed to better understand the range of normal brain aging. Furthermore, many brain markers often are small to moderately correlated with progression to various cognitive disorders, resulting in imperfect predictive models. Nevertheless, the evolution of the science of neurocognitive disorders using neuroimaging keeps pushing the edge of research on early detection of age-related mental illnesses.
For decades (and still to this day), hippocampal volume has been the most well-studied structural marker that differentiates between Alzheimer’s disease and normal aging (e.g., Hedden & Gabrieli, Citation2004; Jack et al., Citation1998; Raz & Rodrigue, Citation2006). Within the past decade, a set of new structural markers assessed using cortical thickness have grown in popularity also to separate different aging trajectories (Bakkour et al., Citation2009; Dickerson et al., Citation2009; McDonough, Citation2017; McDonough et al., Citation2016). While measures of volume and thickness have dominated research questions on successful aging, other measures of shape have been relatively less explored. In this issue, Madan explored the relationship between novel markers of age-related neuroanatomical decline in relation to well-known volumetric measures in subcortical structures including the hippocampus. Across two large independent samples and six different measures of brain morphometry, two markers predicted the majority of age-related variance: fractal dimensionality and surface-to-volume ratio. The current findings clarify the age-related differences in the shape, not just volume, of subcortical structures in the brain and provide strong evidence for additional biological markers of aging. Thus, preserved fractal dimensionality and surface-to-volume ratio might serve as new markers of successful brain aging.
Whereas the volume and shape of subcortical structures often correspond with differences in memory performance (Kaup et al., Citation2011), the connections between brain regions also may be useful markers of aging. Here, Fan and colleagues sought to explore different white matter pathways using diffusion tensor imaging through which aging-related declines in cognition occur. Using mediation analyses, they found a shared brain-cognition pathway in white matter tracts connecting hemispheres of the frontal cortex that explained much of the age-related decline in cognition. This shared pathway might be a mechanism through which both younger and older adults recruit additional neural resources through the contralateral hemisphere as tasks become more taxing. However, they also found a brain-cognition pathway that was unique to older adults that connected the medial temporal lobe (MTL) to the parietal lobe. They surmised that this pathway is preserved into late adulthood and helps to compensate for frontal cortex declines. This combination of declining frontal pathways and preserved posterior pathways offers hints as to which brain regions might need additional protection and which might serve as the first line of ‘neural defense.’
Many factors can accelerate age-related declines and potentially short-circuit potential compensatory pathways including one’s genetic predisposition and health behaviors. In this issue, Aniwattanapong and colleagues investigated some of these risk factors in a group of cognitively normal older adults, those with mild cognitive impairment (MCI), and those with Alzheimer's disease. They showed evidence that the detrimental APOE ε4 allele, folate levels, and triglyceride/high density lipoprotein-cholesterol (TG/HDL-C) ratio cumulatively and independently predict performance on a newly created Thai version of the Boston Naming Test. While the precise role that each of these potential mechanisms plays in the Alzheimer's trajectory is still unclear, evidence thus far suggests that each may be involved in repairing or maintaining neuronal or metabolic pathways. In addition, these findings suggest that folate levels and TG/HDL-C ratio might be modifiable targets for behavioral and pharmaceutical interventions.
To maintain cognition and mental health in both normal and pathological aging, many studies have investigated the effects of acute bouts of physical exercise (e.g., Colcombe et al., Citation2006; Kramer & Erickson, 2007; Rogers, Meyer, & Mortel, Citation1990). However, acute exercise may be more likely to benefit older adults that have become more sedentary in late life. Furthermore, recent clinical trials have found that acute exercise may not be a suitable intervention strategy to combat cognitive decline after a diagnosis of dementia (Lamb et al., Citation2018). While these studies create doubt surrounding the long-term benefits of exercise on cognitive and brain health, few studies have actually measured the long-term benefits through lifelong physical activity. In this issue, Engeroff and colleagues investigated how lifelong physical activity might serve as a cumulative protective factor for cognitive and brain function in late adulthood. They found that physical exercise throughout one’s lifetime increased attention and control over interference. Independently, lifetime physical activity also enhanced measures of neurochemical metabolites using MR spectroscopy. These results provide insight into protective factors that help maintain neuronal integrity and possibly prevent neurodegeneration in neurocognitive disorders such as Alzheimer’s disease. This study also supports recent research that found a reduced incidence of dementia over a 10-year timespan for older adults who self-reported engaging in moderate to vigorous physical activity both at baseline and throughout the 10-year period (Soni et al., Citation2017).
Complementary to a cognitive perspective, neuroimaging also is frequently being used to gain insight into mental health in late life. Individuals with subclinical depression are at increased risk for major depression and other psychiatric conditions. In this issue, Szymkowicz and colleagues aimed to identify preclinical markers of depression using measures of brain volume. The strongest relationship they found was between depressive symptoms and smaller hippocampal volumes. They also found a relationship between somatic depressive symptoms and the posterior cingulate cortex. Indeed, evidence is mounting that depression is linked to a decline in neurogenesis (the birth of new neurons) in the hippocampus (Fang, Demic, & Cheng, Citation2018; Jacobs, van Praag, & Gage, Citation2000). While Szymkowicz and colleagues provide evidence that such risk factors might accelerate age-related declines in brain structure, these risks also might put some older adults at greater risk for dementia (e.g., Diniz et al., Citation2013).
Converging themes in this special issue
Each of these studies illustrates how individual differences can have a large impact on brain health. While the first structural MRI measures of the aging brain occurred in the early 1990’s (e.g., Jernigan et al., Citation1990), one common theme across these studies is the continuing role that measures of brain structure have on informing how people age. As highlighted in this issue, some of these new markers are sensitive indicators of age-related decline (e.g., fractal dimensionality and surface-to-volume; Madan, this issue). The need for such macro-level measures emphasize the impact that structural analyses have already had and continue to have in the Gerosciences for understanding factors that may precede cognitive and functional decline indicative of pathological aging.
This special issue places emphasis on MTL structures such as the hippocampus, suggesting that this particular region plays a critical role in maintaining brain health. The hippocampus is, of course, well studied in regard to its role in episodic memory and its degeneration in neurocognitive disorders such as Alzheimer’s disease. However, the role of the hippocampus and other MTL structures is growing such as its involvement in working memory (Axmacher et al., Citation2010), reasoning (Fan et al., this issue), and even modulation of emotional control (Corcoran et al., Citation2005; Milad et al., Citation2007). The hippocampus is notable because it is closely tied to the default mode network (DMN)—sometimes being characterized within the DMN (Greicius, Srivastava, Reiss, & Menon, Citation2004; Vincent et al., Citation2006) and other times being separate from, but closely interacting with regions within the DMN (Westlye et al., Citation2011). The brain map in the center of illustrates a functional connectivity map using a seed in the medial prefrontal cortex that connects to other parts of the DMN like the parietal cortex, but also shows its high level of connectivity with the hippocampus. Unlike the selective cognitive role that the hippocampus is known for, the DMN has been associated with a variety of cognitive domains including episodic memory, working memory, and self-reflection (e.g., Hampson et al., Citation2006; Buckner et al., Citation2008; Spreng et al., Citation2009). It has been postulated that the DMN might be involved in so many cognitive domains because the regions that comprise this network are highly connected to many other brain networks, thus serving as critical hubs for information processing (Buckner et al., Citation2009; McDonough & Nashiro, Citation2014).
Figure 1. Seed and Soil Model of Neurocognitive Disorders. Many environmental, social, emotional, and behavioral factors based on one’s genes or accrued throughout one’s lifetime experiences impacts the balance of neuroprotection vs. toxicity of the brain’s microenvironment. The more toxic the brain’s microenvironment, the more vulnerable the brain is to pathogenesis, thus increasing the likelihood of the onset of a neurocognitive disorder including Alzheimer’s disease. To the extent that critical brain hubs like the DMN and/or the hippocampus are impacted, the higher the risk of such a disorder. These brain structure and functional insults result in observable changes in cognition (e.g., episodic memory) and emotions (e.g., depression). Prevention of surpassing the disorder threshold can occur by (1) reducing the toxic brain load, (2) increasing neuroprotection, or (3) implementing interventions that strengthen the at-risk brain hubs. The brain map in the center of the figure displays resting-state functional connectivity using the MPFC as a seed region (coordinates = −4, 34, −4) in a sample of 1,000 subjects using Neurosynth (http://neurosynth.org). The top brain image shows the connectivity between the MPFC and the hippocampus and the bottom brain image shows the connectivity between the MPFC and the precuneus/posterior cingulate. DMN = Default mode network; MPFC = Medial prefrontal cortex.
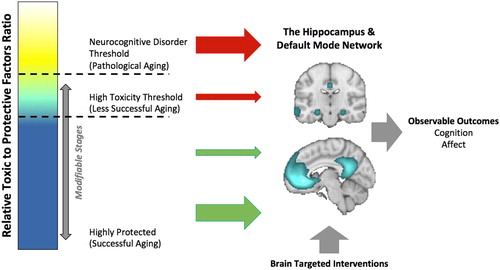
Researchers have proposed that the DMN consumes such a high level of energy relative to other brain networks that it might become overworked and subsequently “burnout,” thus making it vulnerable in neurocognitive disorders such as Alzheimer’s disease (Buckner et al., Citation2009; Jones et al., Citation2016). Perhaps not surprisingly then, additional negative factors like having low lifetime physical activity levels or elevated depressive symptoms might exacerbate these network vulnerabilities. For example, DMN dysfunction has been suggested to precede clinical episodes of depression, thus serving as a marker of affective vulnerability (for review, see Marchetti et al., Citation2012). Importantly, risk factors like subclinical depressive symptoms are immediately treatable, suggesting that these age-related accelerations in atrophy need not occur at all.
Seed and soil model of neurocognitive disorders
As comorbid health conditions accompany normal aging, the risk of neurocognitive disorders might also increase. These health conditions may set the stage for a toxic brain environment that fosters pathologic processes like the accumulation of beta-amyloid or neurofibrillary tangles found in diseases like Alzheimer’s disease. Such ideas are well-known in cancer metastasis, where the beginning cancer cell acts as a seed and the microenvironment of a specific organ serves as the soil that promotes tumor-cell growth—known as the ‘seed and soil’ theory (Paget, Citation1889). In this analog, the soil (or microenvironment) is just as critical as the initial seed because without the right environment, the seed will not grow. In regard to Alzheimer’s disease, the ‘seed’ is not yet clear, but could include genetic factors such as the detrimental APOE ε4 allele or beginning accumulation of beta-amyloid and tau. Regardless of the exact ‘seed,’ research has repeatedly shown that many factors must converge before the cascade of Alzheimer’s disease substantially progresses. For example, a person might have the APOE ε4 allele, but that person may never be diagnosed with the disease. It has been estimated that as many as 50% of people who have two ε4 allele’s (i.e., at most genetic risk) do not develop Alzheimer’s disease even into their 80’s (e.g., Henderson et al., Citation1995; Myers et al., Citation1996). Similarly, about 25%–45% of cognitively normal older adults harbor beta-amyloid deposits (e.g., Bennett et al., Citation2006; Price and Morris, Citation1999) and also may not develop the disease.
In the context of Alzheimer’s disease, the ‘soil’ might be interpreted as the many environmental, social, emotional, and behavioral factors accrued throughout one’s lifetime that impacts the balance of neuroprotection vs. toxicity of the brain’s microenvironment (see ). Critically, it is the soil that allows the seed of pathology to flourish. That is, the more toxic the brain’s microenvironment, the more vulnerable the brain is to pathogenesis. Long-term toxic brain environments will likely compromise neuronal processes that help restore and maintain functioning, leading to a systemic network breakdown. For example, these toxic factors could impair the ability of astrocytes and microglia from clearing away waste, debris, and protein collections that keep neurons healthy, prevent the delivery of sufficient blood and nutrients via the vascular system, may limit the amount of glucose needed to provide energy to neurons, or could lead to chronic inflammation (e.g., Jacobs et al., Citation2012; Schwartz et al., Citation2013). Furthermore, multiple toxic factors might be synergistic in their negative effect on the brain environment. For example, having Type 1 diabetes and depressive symptoms might interact to impact the brain environment that over time leads to progressive cognitive impairment and a diagnosis of dementia (Gilsanz et al., Citation2018). The aging process also might serve as a toxic factor, in part, because the aging brain might be less resilient to brain stressors (McEwen & Morrison, Citation2013). In this intermediate stage of high toxicity, dynamic functional systems are likely to be recruited to compensate or provide scaffolding for the systems that are beginning to breakdown (Park & McDonough, Citation2013; Reuter-Lorenz & Park, Citation2014). To the extent that critical brain hubs like the DMN and/or the hippocampus are impacted, the higher the likelihood of passing a critical threshold that triggers an apparent inevitable series of cell death and detectable behavioral, emotional, and cognitive declines.
The notion of a threshold is being used here differently from that of existent theories. For instance, neural reserve theory posits that a person with greater neural reserve (e.g., brain size or neuronal count) can accumulate more pathology until a threshold is reached to negatively impact cognition and daily functions (e.g., Steffener & Stern, Citation2012; Stern, Citation2009). This notion implements a threshold in the context of a passive model of brain capacity and does not take into account active compensatory neural processes. On the other hand, cognitive reserve suggests that lifestyle factors (e.g., education, socioeconomic status, job occupation) enables one to ‘make flexible and efficient use’ of available neural resources (Steffener & Stern, Citation2012). This more active model assumes that no threshold exists (Stern, Citation2009). Instead, the threshold in our model refers to the level at which the brain can operate normally while retaining sufficient neural plasticity to be modifiable through interventions. Thus, in the context of these other theories, not only might neural reserve be depleted, but flexible and efficient use of brain networks also would be working past capacity. Once the threshold is reached and irreversible neurodegeneration has started, clearly observable changes in cognition (e.g., episodic memory) and affect (e.g., depression) would manifest, thus leading to a diagnosis of a neurocognitive disorder such as Alzheimer’s disease.
A recent study discovering a link between two common herpes viruses and Alzheimer’s disease provides a concrete example. Readhead et al. (Citationin press) found that HHV-6 and HHV-7, which are known for causing a rash in children, were up to two times more abundant in brain tissue of those with Alzheimer’s disease compared with non-Alzheimer’s brain tissue. Rather than inferring that herpes causes Alzheimer’s disease, this viral activity in the brain might constitute a toxic brain factor serving as the ‘soil.’ Further investigation found that the herpes virus genes interacted with genes associated with Alzheimer’s disease such that only the combination was associated with increased beta-amyloid, tau, and immune responses to brain cells (potential ‘seeds’). Thus, having one or more of these herpes viruses as a child might make the brain more vulnerable to pathology later in life and potentially accelerate the disease process—analogous to putting gas on a flame. At the same time, an antiviral medication is not going to be the solution to prevent all cases of Alzheimer’s disease. Rather, such pursuits would only prevent an additional increase in the level of brain toxicity, but still leaves room for numerous other toxic factors to proliferate a negative brain environment (i.e., the soil).
Given the ‘seed’ being planted, the best avenue toward prevention then comes from altering the ‘soil’ before the threshold is reached. Prevention methods can target (1) a reduction of the toxic brain load, (2) an increase in neuroprotection, or (3) interventions that fortify the at-risk brain hubs. Current evidence suggests that treatment efforts are in vain after surpassing this neuronal dysfunction threshold (e.g., Frisoni et al., Citation2017; Lamb et al., Citation2018; Sperling et al., Citation2011). In fact, some researchers are now proposing that this ratio of protective to toxic factors should be optimized by tailoring lifestyle interventions in midlife. By changing behavior early in the lifespan, one can hope to reduce brain damage accumulation and avoid long-term exposure to these toxic factors (Deckers et al., Citation2017; Lafortune et al., Citation2016).
One critically important—and under investigated—area of inquiry is how the many different protective and toxic factors might interact to impact normal brain functioning. The answer is likely not a simple subtraction of the number of toxic from protective factors, nor is a given protective factor likely to ‘cancel out’ a toxic factor. In fact, each factor likely operates on different neural or metabolic pathways and levels of analysis. The take home point is that the accumulation of toxic factors, especially when not counteracted by protective factors, will culminate in the same macro-structure endpoint: systemic burden on critical brain hubs. The insidious effect of these toxic factors on the brain’s microenvironment set the stage to cultivate pathological processes that lead to irreversible neurocognitive disorders. While we have focused on Alzheimer’s disease here, this model is potentially generalizable to different types of neurocognitive disorders and possibly different brain hubs (cf. Seeley et al., Citation2009).
Brain-targeted interventions
The converging threads in this special issue provide an example of how structural neuroimaging can bridge cognitive and emotional systems through a single brain network—in this case, the DMN. While originally derived to test the effectiveness of promoting cognitive and emotional well-being, some interventions in older adults have begun to shed light on how brain regions are altered as a result of interventions. Not only does neuroimaging provide a mechanism for intervention effects, it can also provide a new target for similar or future novel interventions (Alderman et al., Citation2016; Dahlin et al., Citation2008; Shors et al., Citation2014). For example, aerobic exercise training in cognitively normal older adults has been found to increase hippocampal volume, thus reversing age-related brain atrophy by an estimated 1–2 years (Erickson et al., Citation2011). Similar results of aerobic exercise on hippocampal volumes have been found in older women with MCI (Ten Brinke et al., Citation2014).
Another growing area of intervention research is through the implementation of mindfulness-based stress reduction (MBSR). MBSR courses consist of multiple techniques aimed at focusing one’s attention to improve one’s well-being and reduce one’s levels of perceived stress. MBSR in neuroimaging studies often includes practicing mentally scanning one’s body, mindful yoga, and sitting meditation. In one of the earliest studies to test MBSR on brain anatomy, Hölzel et al. (Citation2011) found that a group of young to middle-aged adults evidenced increases in gray matter in the hippocampus and regions of the DMN over 8-weeks. Wells et al. (Citation2013) found similar increases in a small sample of older adults with MCI. In that study, both the MBSR group and the control group showed hippocampal atrophy over the 8 weeks, but the MBSR group showed signs of atrophy slowing relative to the control group. This evidence suggests that while MBSR successfully targeted the hippocampus, participants with MCI might have surpassed the critical brain threshold to reverse the progression of the negative toxic brain state, consistent with the proposed Seed and Soil Model of Neurocognitive Disorders. Similarly, Pickut et al. (Citation2013) showed increased gray matter density in bilateral hippocampi after 8 weeks of MBSR in a small sample of adults with a mean age of 61 with Parkinson’s disease.
Conclusion
Scientific knowledge is steadily being cultivated around those factors that might influence the ratio of protective and toxic factors that threaten successful aging. This knowledge has already impacted (directly or indirectly) the incidence of neurocognitive disorders such as dementia in some countries such as the U.S. and the U.K. Even though the exact causes of these reductions are not known, researchers have speculated that they could be due to the general improvement in health and lifestyle factors, including greater average levels of education, physical activity, better control over cardiovascular diseases, and cognitively stimulating leisure activities across the lifespan (d’Orsi et al., Citation2017; Matthews et al., Citation2016; Wu et al., Citation2016). These lifestyle modifications promote neuroprotection and reduce toxic brain factors that might reduce the brain’s vulnerability to pathogenesis. Without the proper brain environment to promote disease states, genetic or other seeds to disease are less likely to flourish. As outlined here, a central tenet to the Seed and Soil Model of Neurocognitive Disorders is that both the ‘seed’ and the ‘soil’ are necessary components that cause neurocognitive disorders like Alzheimer’s disease. The combination of the two compromises neuronal processes in key brain hubs, thus leading to systemic network breakdown. We propose that such a breakdown can be prevented by reducing one’s toxic brain load, increasing one’s neuroprotection, and engaging in interventions that fortify the at-risk brain hubs. However, more research is needed to identify (a) which combinations of behavioral factors would be most optimal for an individual to pursue to prevent neurocognitive disorders, (b) when during one’s lifetime such changes should be initiated, and (c) what neural pathways each work through.
Disclosure statement
The authors declare that there is no conflict of interest.
References
- Alderman, B. L., Olson, R. L., Brush, C. J., & Shors, T. J. (2016). MAP training: combining meditation and aerobic exercise reduces depression and rumination while enhancing synchronized brain activity. Translational Psychiatry, 6(2), e726.
- Axmacher, N., Henseler, M. M., Jensen, O., Weinreich, I., Elger, C. E., & Fell, J. (2010). Cross-frequency coupling supports multi-item working memory in the human hippocampus. Proceedings of the National Academy of Sciences, 107(7), 3228–3233.
- Bakkour, A., Morris, J. C., & Dickerson, B. C. (2009). The cortical signature of prodromal AD Regional thinning predicts mild AD dementia. Neurology, 72(12), 1048–1055.
- Bennett, D. A., Schneider, J. A., Arvanitakis, Z., Kelly, J. F., Aggarwal, N. T., Shah, R. C., & Wilson, R. S. (2006). Neuropathology of older persons without cognitive impairment from two community-based studies. Neurology, 66(12), 1837–e1844.
- Buckner, R. L., Andrews‐Hanna, J. R., & Schacter, D. L. (2008). The brain's default network: anatomy, function, and relevance to disease. Annals of the New York Academy of Sciences, 1124(1), 1–38.
- Buckner, R. L., Sepulcre, J., Talukdar, T., Krienen, F. M., Liu, H., Hedden, T., … Johnson, K. A. (2009). Cortical hubs revealed by intrinsic functional connectivity: mapping, assess- ment of stability, and relation to Alzheimer’s disease. Journal of Neuroscience, 29(6), 1860–1873.
- Colcombe, S. J., Erickson, K. I., Scalf, P. E., Kim, J. S., Prakash, R., McAuley, E., ... & Kramer, A. F. (2006). Aerobic exercise training increases brain volumein aging humans. The Journals of Gerontology Series A: Biological Sciences and Medical Sciences, 61(11), 1166–1170.
- Corcoran, K. A., Desmond, T. J., Frey, K. A., & Maren, S. (2005). Hippocampal inactivation disrupts the acquisition and contextual encoding of fear extinction. Journal of Neuroscience, 25(39), 8978–8987.
- d'Orsi, E., Xavier, A. J., Rafnsson, S. B., Steptoe, A., Hogervorst, E., & Orrell, M. (2017). Is use of the internet in midlife associated with lower dementia incidence? Results from the English Longitudinal Study of Ageing. Aging & Mental Health, 10, 1–9.
- Dahlin, E., Neely, A. S., Larsson, A., Bäckman, L., & Nyberg, L. (2008). Transfer of learning after updating training mediated by the striatum. Science, 320(5882), 1510–1512.
- Deckers, K., Köhler, S., van Boxtel, M., Verhey, F., Brayne, C., & Fleming, J. (2017). Lack of associations between modifiable risk factors and dementia in the very old: findings from the Cambridge City over-75s cohort study. Aging & Mental Health, 2, 1–7.
- Dickerson, B. C., Bakkour, A., Salat, D. H., Feczko, E., Pacheco, J., Greve, D. N., … Buckner, R. L. (2009). The cortical signature of Alzheimer's disease: regionally specific cortical thinning relates to symptom severity in very mild to mild AD dementia and is de- tectable in asymptomatic amyloid-positive individuals. Cerebral Cortex, 19(3), 497–510.
- Diniz, B. S., Butters, M. A., Albert, S. M., Dew, M. A., & Reynolds, C. F. (2013). Late-life depression and risk of vascular dementia and Alzheimer’s disease: systematic review and meta-analysis of community-based cohort studies. British Journal of Psychiatry, 202(5), 329–335.
- Erickson, K. I., Voss, M. W., Prakash, R. S., Basak, C., Szabo, A., Chaddock, L., … Kramer, A. F. (2011). Exercise training increases size of hippocampus and improves memory. Proceedings of the National Academy of Sciences, 108(7), 3017–3022.
- Fang, J., Demic, S., & Cheng, S. (2018). The reduction of adult neurogenesis in depression impairs the retrieval of new as well as remote episodic memory. PLoS One, 13(6), e0198406. https://doi.org/10.1371/journal.pone.0198406
- Frisoni, G. B., Boccardi, M., Barkhof, F., Blennow, K., Cappa, S., Chiotis, K., … Winblad, B. (2017). Strategic roadmap for an early diagnosis of Alzheimer's disease based on biomarkers. The Lancet Neurology, 16(8), 661–676.
- Gilsanz, P., Schnaider Beeri, M., Karter, A. J., Quesenberry, C. P., Jr, Adams, A. S., & Whitmer, R. A. (2018). Depression in type 1 diabetes and risk of dementia. Aging & Mental Health, 4, 1–7.
- Greicius, M. D., Srivastava, G., Reiss, A. L., & Menon, V. (2004). Default-mode network activity distinguishes Alzheimer's disease from healthy aging: evidence from functional MRI. Proceedings of the National Academy of Sciences of the United States of America, 101(13), 4637–4642.
- Hampson, M., Driesen, N. R., Skudlarski, P., Gore, J. C., & Constable, R. T. (2006). Brain connectivity related to working memory performance. Journal of Neuroscience, 26(51), 13338–13343.
- Hedden, T., & Gabrieli, J. D. (2004). Insights into the ageing mind: a view from cognitive neuroscience. Nature Reviews Neuroscience, 5(2), 87
- Henderson, A. S., Easteal, S., Jorm, A. F., Mackinnon, A. J., Korten, A. E., Christensen, H., … Jacomb, P. A. (1995). Apolipoprotein E allele epsilon 4, dementia, and cognitive decline in a population sample. Lancet (London, England), 346(8987), 1387–1390.
- Hölzel, B. K., Carmody, J., Vangel, M., Congleton, C., Yerramsetti, S. M., Gard, T., & Lazar, S. W. (2011). Mindfulness practice leads to increases in regional brain gray matter density. Psychiatry Research: Neuroimaging, 191(1), 36–43.
- Jack, C. R., Knopman, D. S., Jagust, W. J., Petersen, R. C., Weiner, M. W., Aisen, P. S., … Trojanowski, J. Q. (2013). Tracking pathophysiological processes in Alzheimer's disease: an updated hypothetical model of dynamic biomarkers. The Lancet Neurology, 12(2), 207–216.
- Jack, C. R., Petersen, R. C., Xu, Y., O'Brien, P. C., Smith, G. E., Ivnik, R. J., … Kokmen, E. (1998). Rate of medial temporal lobe atrophy in typical aging and Alzheimer's disease. Neurology, 51(4), 993–999.
- Jacobs, A. H., & Tavitian, B. & INMiND Consortium. (2012). Noninvasive molecular imaging of neuroinflammation. Journal of Cerebral Blood Flow & Metabolism, 32(7), 1393–1415.
- Jacobs, B. L., Van Praag, H., & Gage, F. H. (2000). Adult brain neurogenesis and psychiatry: a novel theory of depression. Molecular Psychiatry, 5(3), 262
- Jernigan, T. L., Press, G. A., & Hesselink, J. R. (1990). Methods for measuring brain morphologic features on magnetic resonance images: validation and normal aging. Archives of Neurology, 47(1), 27–32.
- Jones, D. T., Knopman, D. S., Gunter, J. L., Graff-Radford, J., Vemuri, P., Boeve, B. F., … Jack, C. R. (2016). Cascading network failure across the Alzheimer’s disease spectrum. Brain, 139(2), 547–562.
- Kaup, A. R., Mirzakhanian, H., Jeste, D. V., & Eyler, L. T. (2011). A review of the brain structure correlates of successful cognitive aging. The Journal of Neuropsychiatry and Clinical Neurosciences, 23(1), 6–15.
- Kramer, A. F., & Erickson, K. I. (2007). Capitalizing on cortical plasticity: influence of physical activity on cognition and brain function. Trends in cognitivesciences, 11(8), 342–348.
- Lafortune, L., Martin, S., Kelly, S., Kuhn, I., Remes, O., Cowan, A., & Brayne, C. (2016). Behavioural risk factors in mid-life associated with successful ageing, disability, dementia and frailty in later life: A rapid systematic review. PLoS One, 11(2), e0144405. doi:10.1371/journal.pone.0144405
- Lamb, S. E., Sheehan, B., Atherton, N., Nichols, V., Collins, H., Mistry, D., … Lall, R. (2018). Dementia And Physical Activity (DAPA) trial of moderate to high intensity exercise training for people with dementia: randomised controlled trial. BMJ, 361, k1675.
- Marchetti, I., Koster, E. H., Sonuga-Barke, E. J., & De Raedt, R. (2012). The default mode network and recurrent depression: a neurobiological model of cognitive risk factors. Neuropsychology Review, 22(3), 229–251.
- Matthews, F. E., Stephan, B. C. M., Robinson, L., Jagger, C., Barnes, L. E., Arthur, A., … Forster, G. (2016). A two decade dementia incidence comparison from the Cognitive Function and Ageing Studies I and II. Nature Communications, 7, 11398.
- McDonough, I. M. (2017). Beta-amyloid and Cortical Thickness Reveal Racial Disparities in Preclinical Alzheimer's Disease. NeuroImage: Clinical, 16, 659–667.
- McDonough, I. M., Bischof, G. N., Kennedy, K. M., Rodrigue, K. M., Farrell, M. E., & Park, D. C. (2016). Discrepancies between fluid and crystallized ability in healthy adults: a behavioral marker of preclinical Alzheimer's disease. Neurobiol Aging, 46, 68–75.
- McDonough, I. M., & Nashiro, K. (2014). Network complexity as a measure of information processing across resting-state networks: evidence from the Human Connectome Project. Frontiers in Human Neuroscience, 8, 409.
- McEwen, B. S., & Morrison, J. H. (2013). The brain on stress: vulnerability and plasticity of the prefrontal cortex over the life course. Neuron, 79(1), 16–29.
- Milad, M. R., Wright, C. I., Orr, S. P., Pitman, R. K., Quirk, G. J., & Rauch, S. L. (2007). Recall of fear extinction in humans activates the ventromedial prefrontal cortex and hippocampus in concert. Biological Psychiatry, 62(5), 446–454.
- Myers, R. H., Schaefer, E. J., Wilson, P. W., D'Agostino, R., Ordovas, J. M., Espino, A., … Wolf, P. A. (1996). Apolipoprotein E epsilon4 association with dementia in a population-based study: The Framingham study . Neurology, 46(3), 673–677.
- Paget, S. (1889). The distribution of secondary growths in cancer of the breast. Lancet, 133(3421), 571–573.
- Park, D. C., & McDonough, I. M. (2013). The dynamic aging mind: Revelations from functional neuroimaging research. Perspectives on Psychological Science, 8(1), 62–67.
- Pickut, B. A., Van Hecke, W., Kerckhofs, E., Mariën, P., Vanneste, S., Cras, P., & Parizel, P. M. (2013). Mindfulness based intervention in Parkinson's disease leads to structural brain changes on MRI: a randomized controlled longitudinal trial. Clinical Neurology and Neurosurgery, 115(12), 2419–2425.
- Price, J. L., & Morris, J. C. (1999). Tangles and plaques in nondemented aging and “ ‘preclinical’ Alzheimer's disease”. Annals of Neurology, 45(3), 358–368.
- Raz, N., & Rodrigue, K. M. (2006). Differential aging of the brain: patterns, cognitive correlates and modifiers. Neuroscience & Biobehavioral Reviews, 30(6), 730–748.
- Readhead, B., Haure-Mirande, J.-V., Funk, C. C., Richards, M. A., Shannon, P., Haroutunian, V., … Dudley, J. T. (in press). Multiscale Analysis of Independent Alzheimer’s Cohorts Finds Disruption of Molecular, Genetic, and Clinical Networks by Human Herpesvirus. Neuron, (0)DOI:https://doi.org/10.1016/j.neuron.2018.05.023
- Reuter-Lorenz, P. A., & Park, D. C. (2014). How does it STAC up? Revisiting the scaffolding theory of aging and cognition. Neuropsychology Review, 24(3), 355–370.
- Rogers, R. L., Meyer, J. S., & Mortel, K. F. (1990). After reaching retirement age physical activity sustains cerebral perfusion and cognition. Journal of the American Geriatrics Society, 38(2), 123–128.
- Schneider, L. S., Mangialasche, F., Andreasen, N., Feldman, H., Giacobini, E., Jones, R., … Kivipelto, M. (2014). Clinical trials and late-stage drug development for Alzheimer's disease: an appraisal from 1984 to 2014 . Journal of Internal Medicine, 275(3), 251–283.
- Schwartz, M., Kipnis, J., Rivest, S., & Prat, A. (2013). How do immune cells support and shape the brain in health, disease, and aging? The Journal of Neuroscience: The Official Journal of the Society for Neuroscience, 33(45), 17587–17596.
- Seeley, W. W., Crawford, R. K., Zhou, J., Miller, B. L., & Greicius, M. D. (2009). Neurodegenerative diseases target large-scale human brain networks. Neuron, 62(1), 42–52.
- Shors, T. J., Olson, R. L., Bates, M. E., Selby, E. A., & Alderman, B. L. (2014). Mental and Physical (MAP) Training: a neurogenesis-inspired intervention that enhances health in humans. Neurobiology of Learning and Memory, 115, 3–9.
- Soni, M., Orrell, M., Bandelow, S., Steptoe, A., Rafnsson, S., d'Orsi, E., … Hogervorst, E. (2017). Physical activity pre-and post-dementia: English Longitudinal Study of Ageing. Aging & Mental Health, 10, 1–7.
- Sperling, R. A., Aisen, P. S., Beckett, L. A., Bennett, D. A., Craft, S., Fagan, A. M., …., Park, D. C. (2011). Toward defining the preclinical stages of Alzheimer’s disease: Recommendations from the National Institute on Aging-Alzheimer's Association workgroups on diagnostic guidelines for Alzheimer's disease. Alzheimer's & Dementia, 7, 280–292.
- Sperling, R. A., Jack, C. R., & Aisen, P. S. (2011). Testing the right target and right drug at the right stage. Science Translational Medicine, 3(111), 111cm33–111cm33.
- Sperling, R., Mormino, E., & Johnson, K. (2014). The evolution of preclinical Alzheimer's disease: implications for prevention trials. Neuron, 84(3), 608–622.
- Spreng, R. N., Mar, R. A., & Kim, A. S. (2009). The common neural basis of autobiographical memory, prospection, navigation, theory of mind, and the default mode: a quantitative meta-analysis. Journal of Cognitive Neuroscience, 21(3), 489–510.
- Steffener, J., & Stern, Y. (2012). Exploring the neural basis of cognitive reserve in aging. Biochimica et Biophysica Acta, 1822(3), 467–473.
- Stern, Y. (2009). Cognitive reserve. Neuropsychologia, 47(10), 2015–2028.
- Ten Brinke, L. F., Bolandzadeh, N., Nagamatsu, L. S., Hsu, C. L., Davis, J. C., Miran-Khan, K., & Liu-Ambrose, T. (2014). Aerobic exercise increases hippocampal volume in older women with probable mild cognitive impairment: a 6-month randomised controlled trial. Br J Sports Med, 49(4), 248–254.
- Vincent, J. L., Snyder, A. Z., Fox, M. D., Shannon, B. J., Andrews, J. R., Raichle, M. E., & Buckner, R. L. (2006). Coherent spontaneous activity identifies a hippocampal-parietal memory network. Journal of Neurophysiology, 96(6), 3517–3531.
- Wells, R. E., Yeh, G. Y., Kerr, C. E., Wolkin, J., Davis, R. B., Tan, Y., … Kong, J. (2013). Meditation's impact on default mode network and hippocampus in mild cognitive impairment: a pilot study. Neuroscience Letters, 556, 15–19.
- Westlye, E. T., Lundervold, A., Rootwelt, H., Lundervold, A. J., & Westlye, L. T. (2011). Increased hippocampal default mode synchronization during rest in middle-aged and elderly APOE ε4 carriers: relationships with memory performance. Journal of Neuroscience, 31(21), 7775–7783.
- Wu, Y. T., Fratiglioni, L., Matthews, F. E., Lobo, A., Breteler, M. M., Skoog, I., & Brayne, C. (2016). Dementia in western Europe: epidemiological evidence and implications for policy making. The Lancet Neurology, 15(1), 116–124.