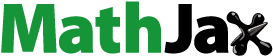
ABSTRACT
Each year, students graduate from schools, colleges and universities. Some find a job quickly – others do not. Delays in the transition from education to work have been associated with scarring effects and detrimental effects on later employment and career progression. Increasing numbers of graduates from universities and colleges are descendants of immigrants. The labour market performance of children of immigrants arguably constitutes an important test of the long-term structural integration of ethnic minorities. Using comprehensive Norwegian administrative data on complete birth cohorts, we apply discrete time hazard regression to examine ethnic inequalities in relative transition rates from education to work, comparing majority graduates to 10 groups of Norwegian-born second-generation immigrants from the 1973 to 1997 birth cohorts (N = 964,450 persons with 1,901,171 person-years). We find clear evidence of ethnic inequalities in transition rates, where children of immigrants or African origin fare the worst. Second, the overall patterns mostly remain unaffected when adjusted for factors like educational field, marriage status, children and parental background. Third, we find that the minority disadvantages are less marked at lower levels of education for some groups.
Introduction
Each school year, students graduate from schools, colleges and universities. Increasing numbers of those who enter the labour market are descendants of immigrants (Schröder Citation2010). Most graduates find a job quickly – others do not. Individuals who experience longer time spans than others before they find a job are not only losing out in terms of forgone earnings; studies show that the transition from education to work also matters to their labour market outcomes later in life (Bradley and Nguyen Citation2004; Steijn, Need, and Gesthuizen Citation2006; Eriksson and Rooth Citation2014). An unsuccessful transition is associated with substantial negative outcomes, while delays have been associated with scarring effects and detrimental effects on later employment and career progression (Korpi Citation1997; Arulampalam, Booth, and Taylor Citation2000; Cockx and Picchio Citation2013).
In this study, we examine ethnic inequalities in transition rates from education to work. Existing research generally provides convincing evidence of ethnic disadvantages in employment rates (Heath, Rothon, and Kilpi Citation2008; Algan et al. Citation2010; Crul, Schneider, and Lelie Citation2012). Inspired by the general literature on transitions from school to work and early labour market experiences (Wolpin Citation1987; Jensen and Westergard-Nielsen Citation1987; Bratsberg and Nilsen Citation2000), earlier studies, using hazard regression models, have addressed ethnic inequality in transition rates from education to work (Nielsen et al. Citation2003; Kalter and Kogan Citation2006; Brekke Citation2007; Tasiran and Tezic Citation2007; Vanoverberghe et al. Citation2008; Gupta and Kromann Citation2014; Toma Citation2015). A recent study also explores ethnic employment differences, comparing British graduates 6 months and 3 years after graduation (Zwysen and Longhi Citation2018). A general finding is that non-western immigrants and their children, especially individuals of African origin, are spend longer time in non-employment after they graduate from the educational system.Footnote1
This research is important and well crafted, yet most studies relying on a longitudinal perspective include only a small set of aggregated groups of second-generation immigrants (such as western/non-western, or world-regions), whereas studies including more detailed origin-based groups lack a proper longitudinal perspective. There is also a need for updated research, as well as studies looking in more detail at the potential importance of educational sorting. In this study, using hazard regression models, we examine relative transition rates from education to the first job for several groups of graduates from Norwegian universities, colleges and secondary schools. We utilise detailed individual-based longitudinal information with yearly observations from registry data covering the years 1990–2014 for the 1973–1997 birth cohorts. We classify all native-born children of immigrants into 10 groups and compare them with the majority population.
We first compare the job attainment rate of all graduates of the same age and educational cohort in the same year. These graduates have also spent the time searching for a job since they graduated from school. We then examine whether the same patterns hold when adjusting for important supply-side factors related to the graduates’ educational level and field, their parental background, marriage status, and, if they have any, their children. We provide clear evidence of ethnic inequalities in relative transition rates. With four exceptions (men with Turkish background and women with Turkish, Vietnamese and Moroccan backgrounds all perform on par with the majority population), all other minority groups are disadvantaged. Adjusting for important supply side factors such as educational qualifications, marriage status and children do not significantly affect this overall pattern of substantial minority disadvantage. We discuss potential mechanisms that could explain these residual disadvantages, including discrimination.
Furthermore, we analyse ethnic differences in job attainment rate for those who completed (a) lower secondary school, (b) upper secondary education and (c) higher education separately. While some studies indicate that the performance of minority groups compared to the majority varies across educational levels, more comprehensive studies of educational heterogeneity are lacking (but see Gupta and Kromann Citation2014). This is an important research question, as factors that potentially affect the job prospects of many minority groups in a negative way, such as reliance on social networks or higher levels of discrimination, may differ across segments of the labour market oriented towards specific levels and types of human capital. We find that for most minority groups, their relative transition rates do not vary by educational level. However, for some groups, we document a tendency for graduates with lower levels of schooling (especially lower secondary school to obtain employment faster relative to the majority) population, when compared to co-ethnics with longer educations.
We hope to make four important contributions to the literature. First, we include more ethnic groups than previous longitudinal research on relative transition rates. Second, we also include graduates who have lower secondary school as their highest level of educational attainment, many of whom are secondary school dropouts. These analyses add to the knowledge of labour market outcomes of a particular vulnerable group. Third, we include a large number of supply-side variables, such as educational level, educational field, marital status, number of children, and parental background. To our knowledge, this is one of the first studies addressing the importance of fields of education for the labour market transition of second-generation immigrants. Fourth, we study the relative transition rates of individuals from the same minority groups compared to the majority population at different levels of education. Very little previous work has considered this issue.
Why should we expect to find ethnic inequalities in education to work transitions?
Based on previous research and theory, we mainly study three factors that are likely to affect ethnic inequalities in labour market transitions: human capital, family obligations, and parental background.
Human capital and education
Human capital theory emphasises the positive causal association between education and work-related skills on labour market performance. In the classical formulation, the level and quality of education is directly linked to productivity (Becker Citation1993). If there is competition for the good jobs, we might expect the more productive and well educated to find a job earlier than the others. According to signalling theory, however, education works as a screening device for employers, as a signal they use to rank job applicants. An educational diploma gives a positive signal of the applicant’s unobserved qualities, such as intelligence, work effort and motivation (e.g. Stiglitz Citation1975). Thus, we can expect shorter transition periods for better educated graduates than others.
Though often downplayed in traditional human capital theory, evidence indicates that field of study also matters for work-related outcomes (Kalmijn and van der Lippe Citation1997; Klein Citation2010). Different kinds of education provide different specialised skills, relevant for different types of jobs. Klein (Citation2010) suggests that the effect of field of specialisation on performance in the labour market is mediated by specificity and selection. The first can be related to the classical formulation of human capital theory, while the second is more congruent with the signalling approach.
In this study, we measure human capital by including educational level and field, as well as, for a reduced sample, secondary school grades. We expect these factors are likely to explain some of the group differences in transition rates. Previous research typically shows substantial educational heterogeneity between different groups of children of immigrants, depending on country and region of origin. This is true for both educational attainment (c.f. Heath, Rothon, and Kilpi Citation2008; Fekjær Citation2007) and educational field (Jonsson and Rudolphi Citation2011).
Marriage status and children
For many young graduates, the period they finish their education overlaps with other life events, such as finding a partner, having children, and taking up loans to buy their first apartment (Bratsberg and Nilsen Citation2000). Family obligations affect individuals’ labour supply. Traditional gender norms, which were dominant in many western countries in the 1950s–1960s (Parsons and Bales Citation1956), prescribe that men should be responsible for providing their family with an income, while women should take the main responsibility for children and housework. Today, gender norms have changed and many societies, including Norway, are characterised by dual-income families (Ellingsæter Citation1998; Lewis Citation2001). Yet, traditional gender roles are still supported in many immigrant groups (Heath, Rothon, and Kilpi Citation2008). Studies indicate, though, that adherence to traditional gender norms are weaker among native-born children of immigrants (Dale, Lindley, and Dex Citation2006; Lappegård Citation2006; Heath, Rothon, and Kilpi Citation2008; Nadim Citation2016). We expect the transition rates from education to work to be influenced by such family-related factors. Thus, intermediate variables such as marital status and, if any, number of children are likely to be associated with transition rates, especially for women. Importantly, differences between ethnic groups in family obligations and adherence to traditional gender norms could potentially explain differences in their labour market success.
Parental background
Parental background is related to ethnic differences in labour market outcomes between descendants of immigrants and the majority population (Heath, Rothon, and Kilpi Citation2008; Jonsson Citation1987; Fekjær Citation2007; Hermansen Citation2013), and we therefore include information on the educational level of parents in our analysis.
To sum up, youths’ transition from education to work is likely to be associated with their level and field of education, their school grades, family obligations, and parental background. We will include these factors as control variables in our analyses. Previous research on the transition from education to work have found that adjusting for these factors makes a difference: Nielsen et al. (Citation2003) document that the inclusion of parental education made the disadvantages in waiting time for second-generation immigrants to Denmark somewhat less marked. Tasiran and Tezic (Citation2007) find that the inclusion of educational level of the individual as well as for the parents reduces disadvantages for some groups of second-generation immigrants in Sweden, but that significant disadvantages remain in most cases. Toma (Citation2015) includes a broader set of variables, more similar to the ones used here, and find that educational level, social background and having a spouse and children reduces some of the disadvantages in the timing of the school to work transition for descendants of immigrants in France. Also, the study by Gupta and Kromann (Citation2014) uses propensity score matching; found that educational level, parental characteristics as well as characteristics of the local labour market reduce; and in some cases reverses the disadvantages in the arrival rate of job offers for second-generation immigrants in Denmark.
Demand-side factors, such as employers treating job applicants with similar characteristics differently, are probably also relevant. Our data does not allow us to measure labour market discrimination, yet we will get back to this interpretation in the discussion.
The Norwegian context
Modern immigration history into Norway started in the 1970. Since then, the immigrant population has grown steadily, from 1.5% in the 1970, 11.4% in 2010, to 18.2% of the population in 2020 (Statistics Norway 2020). Though not a full member of the European Union, Norway has experienced a large influx of Polish immigrant workers after the EU25 enlargement. This is because Norway is a member of the European Economic Area Agreement, and thus in economic terms member of the free market inside EU. Thus, Poles have become the largest migrant group. From non-western countries, the largest immigrant group today are the Somalis, whereas the Pakistanis are the most established non-western group, with the largest group of Norwegians born to immigrant parents (Andreassen and Dzamarija Citation2011).
The employment rate for immigrants from the European Union, including eastern Europeans, and other western countries, are on par with the overall rate, yet immigrants from non-western countries have lower labour market participation; particularly Somalis, Iraqis and Afghanis. In some groups, such as Pakistanis and Somalis, there are also large gender gaps in employment (Olsen Citation2011). Field experiments have documented hiring discrimination against second-generation immigrants with Pakistani/Muslim names in the labour market (Birkelund, Heggebø, and Rogstad Citation2017; Midtbøen Citation2014). Still, attitudinal studies, such as the European Social Surveys, show that compared to other Europeans, Norwegians are relatively liberal and tolerant towards the immigrant population (Blom Citation2011).
indicates that the unemployment rate has generally declined throughout the period we study (1990–2014) and has stayed comparatively low. The rate peaked at around 6% during the early 1990s before stabilising at around 3.5% in the early 2000s with some fluctuations. Due to the country’s oil and gas revenues, the global recession in 2008 was less severe in Norway. Thus, compared to other European countries, the Norwegian labour market has been relatively tight, which in general terms should indicate a comparatively smooth transition from education to work for the newly graduated.
Figure 1. Unemployment ratea 1990–2014. percent.
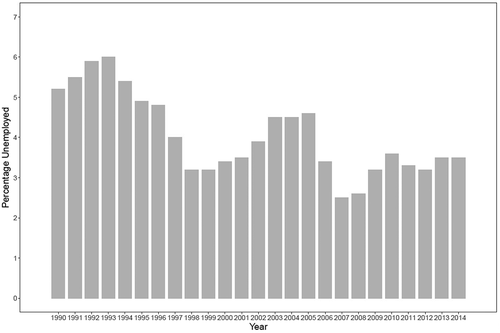
Data and variables
We utilise panel data on individuals from Statistics Norway’s comprehensive registries, which are linked using a unique and non-identifiable ID number for each person. Our data include information on 24 birth cohorts, i.e. individuals born between 1973 and 1997, who completed their education (at different levels) in the period 1990–2014. In each cohort, we include all children of immigrants as well as the complete majority population. Our data include 1,901,171 person-years (964,450 persons) and extend to observations in 2014, so we can measure whether people graduating in 2013 were employed in 2014 at the latest. All the time-varying variables, such as number of children and marriage status, have separate values for each person-year in the data set.
Definitions of variables
Employment: We combine information about annual employment status and income to define employment, and we have set a relatively low earnings threshold for annual employment: All individuals with earnings equal to or above twice the (consumer price adjusted) National Insurance Basic AmountFootnote2 as employed. In 2010, this corresponded to an annual income of about NOK 150,000, which is about 17.000 Euro, which is around one-third of the average income in the period studied. We also estimated the results with a lower threshold (1 G) with very similar results (Figures S1 and S2, Supplementary information).
Country of origin: We study Norwegian-born second-generation immigrants, which we define as individuals whose parents were born abroad. Country of origin is based on the country of birth of the mother. Children with one parent born in Norway and one born abroad are excluded from the analyses. We differentiate between descendants with parents originating in five countries of origin: Morocco, Vietnam, Turkey, India and Pakistan. The other groups are smaller, and we merged them into five regions: Europe and the West, Africa (excluding Morocco), Asia (excluding Vietnam, India and Pakistan; including Oceania), The Middle East (excluding Turkey), and Latin America. The majority is coded zero as the reference category.
Education: The data include detailed educational information from the National Education Database (NUDB). Educational level is a categorical variable with the following categories: (a) Completed lower secondary school; (b) Completed upper secondary education; (c) Higher education (all courses at University/college level, including also BA and MA/PhD). Lower secondary education is mandatory in the Norwegian educational system and covers grades 8–10. Upper secondary education generally consists of 3 years of study. Upper secondary school is not mandatory, though within each birth cohort, the vast majority enter upper secondary school. Higher degrees at colleges and universities conform to European standards (3-year bachelor’s degree, 5-year master’s degree). Field of education is a categorical variable with the following categories: humanistic and esthetical subjects; teaching and pedagogy; social sciences and law; economy and administration; science, crafts and technical subjects; health, social subjects and sports; agriculture, hunting and fishing; transportation, safety and service, and general subjects. These categories cover all parts of the Norwegian education system; some of them are only relevant at higher levels of the educational system. The term general subjects deserves further comment. This is the most common field of education in Norway and particularly in upper secondary school. This field provides access to higher education without requiring extra courses, and is therefore a safe choice for students who want to continue into higher education. In Norwegian it is refered to as ‘studieforberedende’ (preparatory for higher studies). A continuous measure of average lower secondary school grades (only available for a subset of the 1984–1997 cohorts) is also included in an additional analysis (Figure S5) in order to capture additional facets of individual skill level (c.f Miller Citation1998; Gracia, Vázquez-Quesada, and Van de Werfhorst Citation2016).
Social background is measured by parental education when the child was 16 years old. We use information on father’s education and only include mother’s education if father’s is not available. This variable includes the following categories: Lower secondary education (reference category), upper secondary education, short higher education and long higher education. In the analyses performed in STATA, all categorical variables are coded as sets of dummies with the following categories as the reference category: completed upper secondary (for educational level), general subjects (for educational fields) and lower secondary education (for parental education).
Family-related factors: Married is coded as 1, with unmarried/others (0) as reference category. Number of children includes the following categories: No children (reference category); one child; and, two or more children. We have merged two or more children into one category due to low numbers. In the analyses, this variable is implemented as a set of dummies, with no children as the reference category.
We also include the following control variables in all models. Age: Year of birth is included as a categorical variable, dummy coded with 1973 as reference category. Historical time: Due to economic fluctuations over time, we include a categorical variable with each year, from 1990 to 2014, implemented as a set of dummies with 1990 as the reference category. Duration refers to time at risk; i.e. the time between a person’s graduation and until he/she finds a job. To allow for curvilinear patterns, we include both a first order and a second order continuous variable of duration.
Analytical approach
We apply discrete-time hazard regression (Cleves, Gould, and Gutierrez Citation2002); a standard method for investigating relative transition rates. Each person is represented by x number of person years, which constitutes the unit of analysis. In our models, a person-year refers to the number of years in which a person is at risk of gaining his or her first post-graduation job. In our preferred model, we include every year from the individual obtained his or her highest educational level (lower secondary, upper secondary or higher education) including the year when he/she graduated, and in which the person’s earnings are under the specified earnings threshold. Years in which the person pursued full-time education, resided abroad, or gave birth to a child are excluded. This makes no difference to our findings. It should also be mentioned that our data does not include information on whether a person is actively looking for a job. Still, we have attempted to come as close to this as possible given the data at hand, by dealing with it in a way that is standard in the extant literature. Some possible implications of this limitation will be further discussed later.
Some individuals might be unable to find employment after they finished their education at a given level and may therefore decide to return to the educational system. If there are group differences in this behaviour, the exclusion of person-years between different levels of educations might bias the group estimates (see also Neal and Johnson Citation1996; Urban Citation2012). In an alternative specification, we therefore included years spent without employment between educational levels by counting all years since competing lower secondary school as years under risk (again excluding years spent in full-time education, residing abroad or giving birth to a child). This makes very little difference to the main results (see figure A3 and A4 in the appendix).
As we use the complementary logistical log function, we can interpret coefficient estimates as changes in relative rates. By raising Euler’s constant to the coefficient of the X-variable, we obtain the percentage increase in the relative transition rate from education to employment, given that the X-variable increased by one value. We use cluster-robust standard errors to correct for correlations resulting from the fact that many individuals are represented with several observations (person-years).
We perform all analyses separately for women and men, and we estimate three main models. In all models, we control for individual year of birth, duration and duration-squared, historical time and place of residence.
Our full model (3) of which the other models are subsets is represented as follows:
where is the probability of obtaining employment for an individual i at time t.
represents country of origin, our main explanatory variable of interest (where Norwegian natives are the reference category).
represents a vector of time-constant variables (educational level; educational field; parental education at age 16).
represents a vector of time-varying variables (marital status; number of children; place of residence; age; duration and duration squared; year).
is the error term, and
(1 –
)].
This basic approach is implemented in three main models: Model 1 estimates the transition rates to employment for the minority origin groups compared with the majority population. Model 2 includes human capital variables (level and field of education) as well as parental education.Footnote3 Model 3 also includes family-related variables (marital status and number of children). Interactions between all variables and the main groups were also tested (available on request), but they did not significantly affect the main results.
Results
We present our results in two parts. First, we provide descriptive statistics for the transition process by country of origin. We report the overall unadjusted transition rate as well as the timing of successful transitions (see ). Second, we describe the results of the three main models of the event history analyses (see ). Third, we present results from the analysis of heterogeneity in transition rates by educational level ().
Figure 2. Relative rate of transition from education to work for male and female minorities compared to the majority. discrete time hazard regression models.
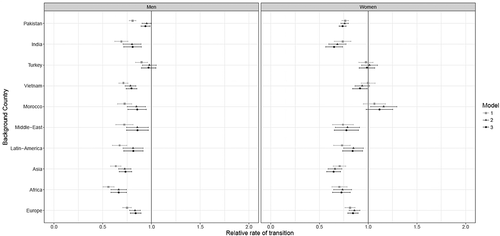
Figure 3. Relative rate of transition from education to work for male and female minorities compared to the majority, by educational level. discrete time hazard regression models.
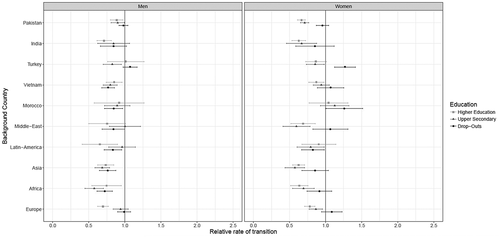
Table 1. Summary statistics for the transition from education to work, by country/region and sex
We begin by looking at group differences in the transition to work. The first rows of show the number of individuals in each origin group, the proportion who transition to work within the observation period, as well as the timing for those who transition. Some patterns are worth noting here. First, all minority groups display a higher percentage without work (right censored) at the end of the period compared to the majority (see , third row). Moreover, 14.8% of majority women and 13.6% of majority men are right-censored. Men of African descent (53%) and females of Middle-Eastern descent (48.8%) display the largest disadvantages, while women and men of Pakistani descent are closest to the majority, with 21.9% and 21% being right-censored, respectively.
A second point concerns the timing of successful transitions into employment. The next three rows of display the percentage who transitioned to work in the first year after graduating, the second year and the third year or later, for the individuals who obtained work during the observation period. We note that for all groups, transitions to employment are most likely to occur in the first year after graduation and then become less likely for each subsequent year without work. About 58% of the individuals of the majority population who are not right-censored obtain employment during their first year under risk. Compared to the majority, obtaining employment in the first year is less likely to happen for most groups of second-generation immigrants. However, female children of immigrants from Vietnam and India are slightly more likely to obtain employment during their first year under risk compared to the majority population.
The last rows of show the proportion of person-years ending in a transition to employment, which is the unit of analysis used in this article. We note that transition percentages vary across the groups, mirroring the results for individuals. For female and male natives, the percentage of person-years in which a transition takes place is 43.2 and 44, respectively. The lowest transition percentages for each gender are found for male children of African immigrants (22.3%) and female children of immigrants originating in The Middle-East (26.4%).
To summarise our discussion so far, most minority groups of second-generation immigrants seem to be disadvantaged in two related ways. Children of immigrants from most of the groups are less likely to be employed overall, and in the cases where they obtain employment, the transition is delayed compared to the majority population. Some of these observed disparities might be due to differences in year of birth, when they were outside the educational system (historical time), and for how long (duration), their level and field of education, parental characteristics, and their family situation. We document the full distribution of these variables for each group in tables S1a and S1b in Supplementary information, and we note a substantial variation between the groups. To address the consequences of adjusting for these factors, we now present the results from three regression models on relative transition rates, based on discrete time hazard regressions. provides the relevant results for men and women separately. Details on individual coefficients are found in Table S2.
Model 1 includes country of origin, with controls for age, year and duration. The results confirm our previous findings of slower relative rates of transition for all minority groups with the exception of female children of Turkish, Vietnamese and Moroccan immigrants, as well as male children of Turkish immigrants, all of which perform on par with the majority. The relative rates for the remaining groups varies between roughly 0.5 and 0.8 of that of the majority. Again, we see that male and female children of African immigrants display the lowest relative transition rates.
In Model 2, we also include individuals’ level and field of education, as well as the parental educational level. The inclusion of these variables leads to the reduction of some of the disadvantages, but this change is only statistically significant in one case: The relative transition rate of male children of immigrants from Pakistan is closer to t(0.9) in this model, reflecting disadvantages in their pre-market resources, related to educational attainment and social origin compared to the majority. The same logic (disadvantages in pre-market resources) implies that the relative transition rate for males of Turkish descent are no longer significantly different from the majority when these factors are adjusted for. Perhaps surprisingly, in this model, women of Moroccan descendants experience a slightly higher rate of transition compared to the majority.
In Model 3, we also include measures of marital status and number of children. For both men and women, these variables have only minor impact on the relative transition rates. While it makes very little difference for men overall, the inclusion of these variables does lead to a lower relative rate compared to that estimated in the second model for females, but the change is not statistically significant for any of the groups. While the changes in the coefficients for the countries and regions from model 2 to 3 are not statistically significant, it is worth noting that male children of Pakistani immigrants as well as female children of Vietnamese immigrants no longer perform on par with the majority (as indicated in model 2) when controls for the family situation are included. Overall, the pattern presented in are in many ways similar to the conclusions based on the findings in , based on the multivariate analyses, though the adjustments affect the relative disadvantages of some groups.
Educational levels
As noted above, the transition rates for the minority groups relative to the majority is likely to vary with qualifications. We therefore estimated the coefficients for each country of origin/region of origin separately at each educational level (). That is, we rely on the same formula as presented earlier, with two modifications: (a) we exclude the educational level variable from the set of individual characteristics and (b) we perform the analyses on three sub-sets of the data, separated by their highest level of education. This means that we have three overall groups: those with lower secondary as their highest educational level, those with higher secondary as their highest educational level, and, those with higher education. We first consider whether the relative transition rates varies between educational levels for each minority group, and then look more closely at the disadvantages – or advantages – in the relative transition rates associated with each level of education for each group. The main results are presented in .
For 8 of 10 groups of male descendants (children of immigrants from Pakistan, India, Vietnam, Morocco, The Middle-East, Latin-America, Asia and Africa), and 6 of 10 groups for the women (children of immigrants from India, Vietnam, Morocco, Latin-America, Asia and Africa), the differences in the relative transition rates do not vary significantly by educational levels, as indicated by overlapping confidence intervals. These results indicate the same relative disadvantage at all three levels of education (while taking the potential for type 2 errors into account).
For the remaining groups, the most common, statistically significant finding is that children of immigrants who have only completed lower secondary education generally have higher transition rates compared to one of or both the other two educational levels. Three main patterns can be discerned. First, for female children of Turkish immigrants, graduates from lower secondary education have the highest overall transition rates. In all groups except Latin America, graduates from lower secondary education have higher transition rates relative to the majority compared to those who have completed upper secondary or higher education. This is the case for women of Middle-Eastern descent, and men of Turkish descent.
Second, for some minority groups, graduates from lower secondary education experience a higher rate of transition compared to graduates from higher education, but perform on par with graduates from upper secondary education. This is the case for both female and male children of European immigrants. However, in the case of males with this background, those who have upper secondary education as their highest educational level obtain work faster than graduates from higher education do. For females of European descent, graduates from upper secondary education perform on par with the two other educational groups.
also indicates that some subgroups obtain employment on par with or faster than the majority population with the same educational qualifications, thereby providing a more detailed picture compared to our previous analyses.
Several subgroups perform on par with the majority population. For men, this is the case for children of immigrants from Pakistan (lower secondary education), India (lower and upper secondary education), Turkey (lower secondary and higher education), Morocco (upper secondary and higher education), Middle-East (all levels), Latin-America (upper secondary education) and Europe (lower and upper secondary education). Male children of immigrants originating in Vietnam, Asia and Africa are disadvantaged at all educational levels.
For women, children of immigrants from Pakistan (lower secondary), India (lower secondary), Turkey (higher education), Vietnam (lower and upper secondary), Morocco (upper secondary and higher education), Middle-East (lower secondary), Latin-America (higher education), Asia (lower secondary), Africa (lower secondary) and Europe (lower secondary) display transition rates that are not significantly different from that of the majority. Females of Turkish and Moroccan descent, who have completed lower secondary as their highest education, actually display faster transition rates compared to individuals of the majority with similar educational qualifications.
Thus, a clear overall pattern is that minority groups at the two lowest levels of education are more likely to transition to the labour market on par with or even faster than the majority, though the latter is rare. However, it is important to keep in mind that when we analyse smaller subgroups in this way the standard errors, and the chance of making type 2-errors, increases. In this case, a type 2 error would imply that we are unable to detect an existing difference, most often a minority disadvantage, due to the low number of observations in each subgroup. The evidence reviewed in this paragraph should therefore be treated as preliminary.
Discussion
The findings presented in this paper are based on a comparison of second-generation immigrants and native Norwegian born 1973–1997, who graduated from schools, colleges and universities and entered the labour market between 1990 and 2013. We have provided new and important insight into the critical transition between the labour market and the job market. Importantly, we have expanded upon previous research by including a larger set of country- and region- based groupings. We have also analysed each group separately at different levels of educational attainment. Consistent with the findings in previous research, we found clear evidence of disadvantages in the relative transition rates for almost all minority groups, especially those of non-western descent. Although the transition rates did not differ by level of education for the most of the groups, some minority groups displayed a tendency for higher relative rates at lower levels of education. Our more detailed results show the following:
Africa, The Middle-East and Latin-America: Previous research has documented that clearest minority disadvantages in the rate of transition from school to work for immigrants and descendants of immigrants originating in non-western countries (Vanoverberghe et al. Citation2008; Gupta and Kromann Citation2014); the regions Africa, Latin-America and The Middle East (Tasiran and Tezic Citation2007), as well as for Maghreb and Africa south of Sahara (Toma Citation2015). In congruence with this evidence, we find that descendants of immigrants originating in Africa, The Middle-East and Latin-America are all disadvantaged compared to the majority population. We also document that children of immigrants from the African region have the lowest relative rate overall for both genders, which is consistent with the last two studies. On the other hand, we find that female descendants of Moroccan immigrants obtain employment faster than the majority; which is not consistent with general evidence for children of African immigrants in Sweden (Tasiran and Tezic Citation2007), but in line with findings for Moroccan first-generation immigrants in Belgium and Spain (Kalter and Kogan Citation2006).
Europe: We also document some disadvantages for second-generation immigrants from Europe, while Vanoverberghe et al. (Citation2008) and Tasiran and Tezic (Citation2007) find no such disparity for ethnic minorities in Flanders and Sweden, respectively. However, Gupta and Kromann (Citation2014) also find some disadvantage for second-generation western immigrants to Denmark, and Toma (Citation2015) documents a disadvantage for men of South-European origin in France.
South-East Asia: Toma (Citation2015) also provides evidence for slower transitions to employment for children of immigrants from South-East Asia on the regional level compared to the French majority population. Our findings are partially consistent with this. We document disadvantages for both male and female children of immigrants from Asia, as well as male children of Vietnamese immigrants. On the other hand, female children of Vietnamese immigrants perform on par with the majority.
How can we explain these disparities? We have included information on individual educational level and – field, parental education, marital status and number of children as intermediate variables, to see if these variables effect the original association between immigrant status and the relative transition rates. We found that the intermediate variables reduced the disadvantages somewhat, yet made almost no difference to the overall results.Footnote4
When supply side factors, such as human capital, parental background and family responsibility, seem to be of relatively little relevance, future research on relative transition rates from education to work would probably benefit from inclusion of demand side factors, related to characteristics of employers and firms, labour market structure, etc. Our results are clearly consistent with the existence of labour market discrimination of ethnic minority groups in job hiring processes. Previous field experiments have documented discrimination of second-generation Pakistani-Norwegians (Midtbøen Citation2014; Birkelund, Heggebø, and Rogstad Citation2017), and it is therefore reasonable to believe that employer discrimination could explain at least some of the remaining disadvantages of minority groups in their relative transition rates (see also Booth, Leigh, and Varganova Citation2012; Connor and Koenig Citation2015). In addition, differential access to and quality of social networks could also be relevant for the topic explores here (Schröder Citation2010). While we have emphasised the importance of looking at factors on the demand side in future studies, yet there might also be other relevant supply side factors that we have been unable to include, such as differences in motivation, search behaviour, or preferences.
Another issue in our analytical design is endogeneity. Most of the intermediate factors included here are likely to be affected by such problems. For example, having a child and getting married or choosing to return to the educational system could be affected by one’s success in the labour market, implying reverse causality. We attempt to shed some light on this issue by including a measure of school grades in a supplementary analysis.
Figure S3 in Appendix provides results from two models; a reference model similar to model 1 in , and another model that also includes information about individuals’ grades when they finished lower secondary school (available only for a subset of our main sample – most individuals from the 1984–1997 cohorts). Students usually complete lower secondary school when they are 16 years old, so the grades should be unaffected by their future experiences in the labour market, thus – to some degree – mitigating problems related to reverse causality as well as certain types of endogeneity, such as correlated unobservable characteristics (Neal and Johnson Citation1996).Footnote5 These results provide some evidence indicating that early educational performance and correlated unobservable characteristics do not account for the group differences in relative transition rates. Again, the main conclusion seems to point towards the demand side of the labour market.
Less ethnic disadvantage at lower levels of education: The second part of our analysis looked at whether the disadvantages we have documented for most minorities varied with their educational attainment. Our analyses indicated that minority disadvantages were in some cases less marked at lower levels of education, though for most of the minority groups there were no significant differences in relative rates between the different educational levels. While previous research on this topic is sparse, Gupta and Kromann (Citation2014) find that the disadvantages in the arrival rate of job offers for second-generation immigrants in Denmark are less marked for those with lower levels of education (primary school and secondary/high school). In fact, they find that minority women with primary school as their highest level of qualifications are advantaged compared to the majority. The findings of Støren, Opheim, and Helland (Citation2009) based on Norwegian data are also relevant here. They look at the probability of being employed in a certain year after graduation. They study non-western immigrants and children of immigrants with low levels of education, i.e. from lower secondary to completed upper secondary, and find that the disadvantage in employment for non-western immigrants is less marked at lower levels of education.
Several explanations can potentially account for these findings, and we will suggest three. First, they may be a result of negative selection of natives at the lower educational levels. That is, natives could be more negatively selected in terms of health or other characteristics compared to minority individuals in the same situation (Støren, Opheim, and Helland Citation2009). Second, the results could also be due to a different kind of selection mechanism, where the minorities who are least successful in the labour market chose to return to the educational system (Urban Citation2012). As reported in supplementary analysis (see Figure S2), we have also tested models where we instead of relying on information on individuals’ highest educational level (our preferred measure) include all years they were under risk for finding employment, such as when they were between different educational levels. We found that the results did not differ much from the other outcomes. Third, the mechanisms that are relevant for the job opportunities of ethnic minorities might operate in different ways in different parts of the labour market. For example, discrimination could play a less important role if employers find few job applicants who are willing to work, for instance, in low-skilled jobs in service and manufacture.
While we have provided robust evidence for an overall disadvantage for minorities and for some variations by educational level, several limitations remain. First, we have information on a yearly basis, thus masking potentially group differences on a finer temporal level. Second, the registry data do not provide information on important labour supply mechanisms, such as work commitment (Gorinas Citation2014). Another important limitation concerns the issue of causality. In our main analyses, we introduce intermediate variables that might shed light on potential group disparities in relative transition rates. This strategy has been descriptively important and useful for testing hypotheses about possible reasons for ethnic disadvantages; these variables may introduce forms of endogeneity, thus making causal interpretations difficult. We have made some headway by introducing high-school grades, which are arguably less prone to such problems. Still, our results should not be interpreted as causal findings, and other types of research designs would be highly useful to complement existing studies.
Supplemental Material
Download MS Word (270.8 KB)Acknowledgments
The authors are grateful for comments from seminar audiences in Philadelphia (RC28) and Hafjell. The data required for this project were made available through the SEGREGATION project funded by the Research Council of Norway (RCN project #202479) as well as the FirmStrat project (Grant 287016).
Disclosure statement
No potential conflict of interest was reported by the author(s).
Supplementary material
Supplemental data for this article can be accessed here
Additional information
Funding
Notes on contributors
Mats Lillehagen
Mats Lillehagen is a post-doc in Sociology at the University of Oslo. His main research interests include social inequalities, immigration and population dynamics. He has published articles in European Sociological Review, Demography and Population Studies. See https://www.sv.uio.no/iss/english/people/aca/matslil/index.html
Gunn Elisabeth Birkelund
Mats Lillehagen is a post-doc in Sociology at the University of Oslo. His main research interests include social inequalities, immigration and population dynamics. He has published articles in European Sociological Review, Demography and Population Studies. See https://www.sv.uio.no/iss/english/people/aca/matslil/index.html
Gunn Elisabeth Birkelund is a Professor of Sociology at University of Oslo. Her main research interests include analytical sociology, labor market studies, social inequalities and population dynamics. She is a Fellow at The European Academy of Sociology, and Vice President of the Norwegian Academy of Science and Letters. Her articles have appeared in European Societies, European Sociological Review, Social Forces, International Migration Review, and, earlier, in American Journal of Sociology and American Sociological Review. See https://www.sv.uio.no/iss/english/people/aca/gunnb/index.html
Notes
1. This pattern is documented for the following groups: Immigrants from Morocco and outside the EU in Belgium, and for Moroccan immigrants in Spain (Kalter and Kogan Citation2006); immigrants (with master degree) from Western Europe, Eastern Europe, North America, Asia and Africa in Norway (Brekke Citation2007); non-western immigrants and their children in Flanders (Vanoverberghe et al. Citation2008); second-generation immigrants in Denmark (Nielsen et al. Citation2003); descendants of immigrants originating in Africa, The Middle East and Latin-America in Sweden (Tasiran and Tezic Citation2007); and male second-generation immigrants from Maghreb, previous French colonies in Africa, Sub-Saharan Africa, South-East Asia and Turkey in France (Toma Citation2015). Gupta and Kromann (Citation2014) document lower rates of job-offers for unemployed children of western and non-western immigrants in Denmark. Exceptions to the pattern of disadvantages are found for EU immigrants to Spain (Kalter and Kogan Citation2006), minority women with the lowest level of education in Denmark (Gupta and Kromann Citation2014) and male children of South-European immigrants in France (Toma Citation2015).
2. This threshold implies eligibility for unemployment benefits as well as pension related to disability and old age.
3. We first included individual education (level and field) separately, and then included parental education, but these models did not differ significantly (available on request). We therefore combine these two models into Model 2.
4. There are two exceptions: Male descendants of immigrants from Turkey perform on par with the majority population when these intermediate variables were included, indicated their educational disadvantage (see Table 1Sb). On the other hand, female descendants of Vietnamese immigrants are more disadvantaged after adjustments were made, which is related to their relative lower level of family obligations (see Table 1Sa).
5. While including grades for upper secondary and subsequent education or a measure of general ability would be interesting, such information unfortunately is not available, nor would grades at this level arguably constitute a true pre-market measure.
References
- Algan, Y., C. Dustmann, A. Glitz, and A. Manning. 2010. “The Economic Situation of First and Second‐generation Immigrants in France, Germany and the United Kingdom.” The Economic Journal 120 (542): 4–30. doi:https://doi.org/10.1111/j.1468-0297.2009.02338.x.
- Andreassen, K.K., and M.T. Dzamarija. 2011. “SSB) ‘Population.” In Immigration and Immigrants 2010, Statistical Analyses, edited by K. Henriksen, L.Østby, and D. Ellingsen, 15–46. Oslo: Statistics Norway.
- Arulampalam, W., A. Booth, and M. Taylor. 2000. “Unemployment Persistence.” Oxford Economic Papers 52 (1): 24–50. doi:https://doi.org/10.1093/oep/52.1.24.
- Becker, G. S. 1993. Human Capital: A Theoretical and Empirical Analysis with Special Reference to Education. Third ed. Chicago: University of Chicago Press.
- Birkelund, G. E., K. Heggebø, and J. Rogstad. 2017. “Additive or Multiplicative Disadvantage the Scarring Effects of Unemployment for Ethnic Minorities.” European Sociological Review 33: 17–29.
- Blom, S. 2011. “Comparison of Attitudes in Norway and Other European Countries.” In Immigration and Immigrants, edited by K. Henriksen, L. Østby, and D. Ellingsen, 151–167. Oslo: Statistics Norway.
- Booth, A. L., A. Leigh, and E. Varganova. 2012. “Does Ethnic Discrimination Vary across Minority Groups? Evidence from a Field Experiment.” Oxford Bulletin of Economics and Statistics 74 (4): 547–573. doi:https://doi.org/10.1111/j.1468-0084.2011.00664.x.
- Bradley, S., and A. N. Nguyen. 2004. “The School to Work Transition.” In International Handbook of Education Economics, edited by G. Johnes and J. Johnes, 484–521. Cheltenham: Edward Elgar.
- Bratsberg, E., and O. A. Nilsen. 2000. “Transitions from School to Work and the Early Labour Market Experience.” Oxford Bulletin of Economics and Statistics 62 (s1): 909–929. doi:https://doi.org/10.1111/1468-0084.0620s1909.
- Brekke, I. 2007. “Ethnic Background and the Transition from Education to Work among University Graduates.” Journal of Ethnic and Migration Studies 33 (8): 1299–1321. doi:https://doi.org/10.1080/13691830701614080.
- Cleves, M., W. Gould, and R. Gutierrez. 2002. An Introduction to Survival Analysis Using Stata. College Station, TX: Stata Press.
- Cockx, B., and M. Picchio. 2013. “Scarring Effects of Remaining Unemployed for Long‐term Unemployed School‐leavers.” Journal of the Royal Statistical Society: Series A (Statistics in Society) 176 (4): 951–980. doi:https://doi.org/10.1111/j.1467-985X.2012.01086.x.
- Connor, P., and M. Koenig. 2015. “Explaining the Muslim Employment Gap in Western Europe: Individual-level Effects and ethno-religious Penalties.” Social Science Research 49: 191–201. doi:https://doi.org/10.1016/j.ssresearch.2014.08.001.
- Crul, M., J. Schneider, and F. Lelie, eds. 2012. The European Second Generation Compared: Does the Integration Context Matter? Amsterdam: Amsterdam University Press.
- Dale, A., J. Lindley, and S. Dex. 2006. “A Life-course Perspective on Ethnic Differences in Women’s Economic Activity in Britain.” European Sociological Review 22 (3): 323–337. doi:https://doi.org/10.1093/esr/jci060.
- Ellingsæter, A. L. 1998. “Dual Breadwinner Societies: Provider Models in the Scandinavian Welfare States.” Acta Sociologica 41 (1): 59–73. doi:https://doi.org/10.1177/000169939804100105.
- Eriksson, S., and D.O. Rooth. 2014. “Do Employers Use Unemployment as a Sorting Criterion When Hiring? Evidence from a Field Experiment.” American Economic Review 104 (3): 1014–1039. doi:https://doi.org/10.1257/aer.104.3.1014.
- Fekjær, S. N. 2007. “New Differences, Old Explanations: Can Educational Differences between Ethnic Groups in Norway Be Explained by Social Background?” Ethnicities 7 (3): 367–389. doi:https://doi.org/10.1177/1468796807080234.
- Gorinas, C. 2014. “Ethnic Identity, Majority Norms, and the native–immigrant Employment Gap.” Journal of Population Economics 27 (1): 225–250. doi:https://doi.org/10.1007/s00148-012-0463-3.
- Gracia, P., L. Vázquez-Quesada, and H. G. Van de Werfhorst. 2016. “Ethnic Penalties the Role of Human Capital and Social Origins in Labour Market Outcomes of second-generation Moroccans and Turks in the Netherlands.” Journal of Ethnic and Migration Studies 42 (1): 69–87. doi:https://doi.org/10.1080/1369183X.2015.1085800.
- Gupta, N. D., and L. Kromann. 2014. “Differences in the Labor Market Entry of second-generation Immigrants and Ethnic Danes.” IZA Journal of Migration 3 (1): 16. doi:https://doi.org/10.1186/s40176-014-0016-5.
- Heath, A.F., and S. Y. Cheung, Eds. 2007. Unequal Chances: Ethnic Minorities in Western Labour Markets. Oxford: British Academy/Oxford University Press.
- Heath, A., C. Rothon, and E. Kilpi. 2008. “The Second Generation in Western Europe: Education, Unemployment, and Occupational Attainment.” Annual Review of Sociology 34 (1): 211–235. doi:https://doi.org/10.1146/annurev.soc.34.040507.134728.
- Hermansen, A. S. 2013. “Occupational Attainment among Children of Immigrants in Norway: Bottlenecks into Employment – Equal Access to Advantaged Positions?” European Sociological Review 29 (3): 517–534. doi:https://doi.org/10.1093/esr/jcr094.
- Jensen, P., and N.C. Westergard-Nielsen. 1987. “A Search Model Applied to the Transition from Education to Work.” Review of Economic Studies 54 (3): 461–472. doi:https://doi.org/10.2307/2297569.
- Jonsson, J.O. 1987. “Class Origin, Cultural Origin and Educational Attainment. The Case of Sweden.” European Sociological Review 3 (3): 229–242. doi:https://doi.org/10.1093/oxfordjournals.esr.a036451.
- Jonsson, Jan O, and Frida Rudolphi. 2011. “Weak Performance—strong Determination: School Achievement and Educational Choice Among Children of Immigrants in Sweden.” European Sociological Review 27 (4): 487–508. doi:https://doi.org/10.1093/esr/jcq021.
- Kalmijn, M., and T. van der Lippe. 1997. “Type of Schooling and Sex Differences in Earnings in the Netherlands.” European Sociological Review 13 (1): 1–15. doi:https://doi.org/10.1093/oxfordjournals.esr.a018198.
- Kalter, F., and I. Kogan. 2006. “Ethnic Inequalities at the Transition from School to Work in Belgium and Spain: Discrimination or self-exclusion?” Research in Social Stratification and Mobility 24 (3): 259–274. doi:https://doi.org/10.1016/j.rssm.2005.10.002.
- Klein, M. 2010. “Mechanisms for the Effect of Field of Study on the Transition from Higher Education to Work.” Mannheimer Zentrum für Europäische Sozialforschung Arbeitspapiere nr, no. 130. https://strathprints.strath.ac.uk/57477/.
- Korpi, T. 1997. “Is Utility Related to Employment Status? Employment, Unemployment, Labor Market Policies and Subjective well-being among Swedish Youth.” Labour Economics 4 (2): 125–147. doi:https://doi.org/10.1016/S0927-5371(97)00002-X.
- Lappegård, T. 2006. “Family Formation and Education among Pakistani, Turkish and Vietnamese Women in Norway.” Genus 62: 75–95.
- Lewis, J. 2001. “The Decline of the Male Breadwinner Model: Implications for Work and Care.” Social Politics: International Studies in Gender, State and Society 8 (2): 152–169. doi:https://doi.org/10.1093/sp/8.2.152.
- Midtbøen, A. H. 2014. “Discrimination of the Second Generation: Evidence from a Field Experiment in Norway.” Journal of International Migration and Integration 17 (1): 253–272. doi:https://doi.org/10.1007/s12134-014-0406-9.
- Miller, S. R. 1998. “High School Grades as a Signal of Human Capital.” Educational Evaluation and Policy Analysis 20 (4): 299–311. doi:https://doi.org/10.3102/01623737020004299.
- Nadim, M. 2016. “Undermining the Male Breadwinner Ideal? Understandings of Women’s Paid Work among second-generation Immigrants in Norway.” Sociology 50 (1): 109–124. doi:https://doi.org/10.1177/0038038514560259.
- Neal, D. A., and W. R. Johnson. 1996. “The Role of Premarket Factors in black-white Wage Differences.” Journal of Political Economy 104 (5): 869–895. doi:https://doi.org/10.1086/262045.
- Nielsen, H. S., M. Rosholm, N. Smith, and L. Husted. 2003. “The school-to-work Transition of 2nd Generation Immigrants in Denmark.” Journal of Population Economics 16 (4): 755–786. doi:https://doi.org/10.1007/s00148-003-0164-z.
- Olsen, B. 2011. “Labour.” In Immigration and Immigrants, edited by K. Henriksen, L. Østby, and D. Ellingsen, 77–102. Oslo: Statistics Norway.
- Parsons, T., and R. F. Bales. 1956. Family Socialization and Interaction Process. Milton Park, Oxfordshire: Routledge Press.
- Schröder, L. 2010. “Labour Market Characteristics and Their Impact on the Integration of Immigrant Offspring.” In Equal Opportunities: The Labour Market Integration of the Children of Immigrants, 129–160. Paris: OECD Publishing.
- Statistics Norway. 2017. [Immigrant. Statistics for Norway] URL: https://www.ssb.no/befolkning/statistikker/innvbef
- Steijn, B., A. Need, and M. Gesthuizen. 2006. “Well Begun, Half Done? Long-term Effects of Labour Market Entry in the Netherlands, 1950-2000.” Work, Employment and Society 20 (3): 453–472. doi:https://doi.org/10.1177/0950017006066996.
- Stiglitz, J. E. 1975. “The Theory of “Screening”, Education, and the Distribution of Income.” American Economic Review 65: 283–300.
- Støren, L. A., V. Opheim, and H. Helland. 2009. “Getting through Closed Doors? Labour Market Entry among Ethnic Minority and Majority Youth with Low Levels of Formal Education.” Journal of Education and Work 22 (4): 261–282. doi:https://doi.org/10.1080/13639080903290413.
- Tasiran, A., and K. Tezic. 2007. “Early labour-market Experiences of second-generation Immigrants in Sweden.” Applied Economics 39 (7): 809–824. doi:https://doi.org/10.1080/00036840500461915.
- Toma, S. 2015. “The School-to-Work Transitions of Second Generation Immigrants in France.” International Journal of Migration and Border Studies 1 (4): 372–397. doi:https://doi.org/10.1504/IJMBS.2015.070786.
- Urban, S. 2012. “University Education as a Compensation Strategy among Second‐Generation Immigrants.” International Migration Review 46 (4): 919–940. doi:https://doi.org/10.1111/imre.12005.
- Vanoverberghe, J., D. Verhaest, E. Verhofstadt, and E. Omey. 2008. “The Transition from School to Work in Flanders: A Duration Analysis.” Journal of Education and Work 21 (4): 317–331. doi:https://doi.org/10.1080/13639080802379895.
- Wolpin, K.I. 1987. “Estimating a Structural Search Model: The Transition from School to Work.” Econometrica 55 (4): 801–817. doi:https://doi.org/10.2307/1911030.
- Zwysen, W., and S. Longhi. 2018. “Employment and Earning Differences in the Early Career of Ethnic Minority British Graduates: The Importance of University Career, Parental Background and Area Characteristics.” Journal of Ethnic and Migration Studies 44 (1): 154–172. doi:https://doi.org/10.1080/1369183X.2017.1338559.