Abstract
This study constitutes the first longitudinal exploration of consent to link survey and administrative data. It examines variations in consent over time and explores the influence of the respondents’ characteristics (both observed and latent) and the impact of the interviewers on consent co-operation. Respondent inclination to consent is modelled as a latent construct. Most respondents behave consistently over time. However, this consistency is not driven by a strong inclination to consent but rather by the circumstances of the respondents at the time of the interview and by the impact of the interviewers themselves. The findings also show that the change in consent behaviour over time is a clear indication that consent should be treated as a dynamic phenomenon at the individual level.
1. Introduction
Longitudinal surveys face significant challenges due to the rise in survey costs, attrition over time, and non-coverage of the target population. All these challenges have the potential to undermine the quality of the collected data. One method of reducing the costs of data collection and maintaining coverage of the survey record is to link selected individual administrative information to the survey. Administrative data linkage leads to shorter interviews, less respondent burden and an overall reduction in costs (Sakshaug, Couper, Ofstedal, & Weir, Citation2012) in addition to the gain of valuable information on respondents. However, access to administrative records will suffer from non-consent whenever respondents refuse permission to have their records linked to the survey. Non-consent will obviously result in smaller sample sizes and possibly bias the sample composition if the likelihood of consent is related to the characteristics of the respondents.
The existing literature on consent is dominated by studies arising from the medical profession and epidemiology. Much of the early work (Baker, Shiels, Stevenson, Fraser, & Stone, Citation2000; Dunn, Jordan, Lacey, Shapley, & Jinks, Citation2004; Nelson et al., Citation2002; Kho, Duffett, Willison, Cook, & Brouwers, Citation2009; Silva, Smith, & Bammer, Citation2002; Huang, Shih, Chang, & Chou, Citation2007) focused on linking patients’ administrative records to medical surveys. More recently, the work of Jenkins, Cappellari, Lynn, Jäckle, and Sala (Citation2006) opened the door to a new wave of studies focusing on multi-topic social surveys. All these studies examined the impact of various socio-demographic characteristics on consent and some focused on particular attributes of the respondents such as their personality (Sala, Burton, & Knies, Citation2012; Jenkins et al., Citation2006; Olson, Citation1999; Woolf, Rothemich, Johnson, & Marsland, Citation2000; Armstrong et al., Citation2008; Sakshaug & Kreuter, Citation2012; Sakshaug et al., Citation2012; Schröder et al., Citation2015; and Al Baghal, Citation2016). Other studies explored the impact of interviewers on consent (Sala et al., Citation2012; Sakshaug et al., Citation2012; Korbmacher & Schroeder, Citation2013; Sakshaug, Tutz, & Kreuter, Citation2013) while few have provided experimental evidence on the effect of survey features such as question wording and placement (Sakshaug et al., Citation2013; Sala, Burton, & Knies, Citation2014).
To-date the emphasis has been on the nature of consent arising in cross-sectional surveys and very little is known about the patterns of consent over time when respondents are asked to provide consent on repeat occasions. Exceptions include Sala et al. (Citation2012) and Schröder et al. (Citation2015). Sala et al. (Citation2012) used data from previous waves of the British Household Panel Survey to explain consent to link health and benefit records in subsequent waves. The authors examined the impact of respondent and interviewer characteristics and interview features. Schröder et al. (Citation2015), on the other hand, studied selectivity and bias resulting from obtaining consent in a longitudinal survey. In comparison, our study draws upon the UK Millennium Cohort Study (MCS) in order to explore any changes in respondents’ circumstances and their decision to consent to agree to provide access to health records over time. The paper addresses three research questions:
RQ1 | Is consent behaviour consistent over time? | ||||
RQ2 | Is it possible to identify a latent inclination to consent? and | ||||
RQ3 | What are the factors that influence consent? |
Consent behaviour is said to be consistent if respondents behave in exactly the same way over time. We suggest that this consistency (or inconsistency) might be the result of three types of factors: the respondent’s inclination to consent which may be driven by unmeasured aspects of the individual such as their personality or a general disposition or attitude towards the value of survey research or satisficing behaviour, the respondent’s observed characteristics which are largely socio-demographic but also include response histories and any reported concerns about confidentiality, and the effect of the interviewer’s attempts to elicit consent.
The paper advances our knowledge in three ways. First, it investigates the existence of an inclination to consent. Secondly, it examines both the cross-sectional and longitudinal variations in consent. Thirdly, it measures the impact of interviewers on the respondents’ consent patterns. Moreover, the growing popularity of longitudinal cohort studies and the expanding practice of administrative and survey data linkage highlight the value of this study for both data users concerned about non-consent bias and survey professionals interested in improving fieldwork practices.
The paper is organised as follows. Section II discusses consent mechanisms over time. Section III presents the data, consent procedures, and methods. Section IV presents the findings, and the final section concludes.
2. Consent mechanisms over time
In longitudinal and birth cohort studies, consent for survey and administrative data linkage has to be sought repeatedly over time for ethical reasons in order to give respondents the chance to re-consider their previous decision about whether or not to release their administrative records. Therefore, it is quite possible that respondents’ consent behaviour will change. Those who have consented in the past might refuse to consent in the future and vice versa. In other words, some respondents will behave consistently over time while others will not. We argue that these variations in consent behaviour can be linked to three types of influences: the respondent’s characteristics both unobserved and observed and the interviewers.
From a theoretical perspective, consistency in one’s attitudes and behaviours is at the core of human conduct (Festinger, Citation1957; Heider, Citation1958; Newcomb, Citation1953). People in general are inclined to be consistent with what they said or did in the past. Thus, after committing themselves to a particular action or set of behaviours, they are likely to act in ways that reflect this commitment especially if it is freely made (Cialdini, Wosinska, Barrett, Butner, & Gornik-Durose, Citation1999, p. 1244). In this study, the consistency principle implies that respondents who have consented to link their survey and administrative data in the past are likely to consent in subsequent waves of data collection. However, since most research was largely based on cross-sectional data, it was not possible to test this assertion. Our study sets out to examine consent behaviour for the same respondents when asked to consent to health record linkage on three separate occasions in the context of a longitudinal birth cohort study.
Given the fact that consent was sought on different occasions, it is possible to estimate a latent inclination to consent that reflects unobserved respondent characteristics which might include personality, personal convictions, and certain predispositions. In a cross-sectional survey it is not possible to separate out the latent inclination to consent from the unobserved conditions of the interview. Whereas, in a longitudinal survey, the circumstances of the interview are likely to be different from wave to wave; whilst certain latent personal characteristics and attitudes will remain stable. By using multivariate probit models (Cappellari & Jenkins, Citation2003), it will be possible to identify whether the unobserved parts of consent outcomes sought in different waves are correlated over time. If the correlation is strong, it will be possible to conclude that consent is driven by latent attitudes or personal characteristics which tend to be stable over time.
Furthermore, consent is likely to be affected by the respondent’s observed characteristics and any changes in these characteristics over time. These include social class, marital status, ethnic group, religion, language spoken at home, response history, self-reported health, and reported confidentiality concerns, some of which may well vary over time. Our choice of respondent characteristics was motivated by the existing literature, e.g. Sheldon, Graham, Pothecary, and Rasul (Citation2007) argue that disadvantaged social groups and some ethnic minorities are less likely to cooperate in surveys due to low levels of literacy, disengagement from government, and communication barriers. In contrast, respondents practicing a religion which is demanding in its rituals are more likely to cooperate according to Levy and Razin (Citation2012). Moreover, respondents who have a history of taking part in a survey with few occasions of non-response are also likely to cooperate with in-survey requests (Mostafa, Citation2016). Similarly, respondents with health problems are more likely to consent for linking health records due to their previous relations with the authorities holding the data (Sakshaug et al., Citation2012), while respondents who are worried about data confidentiality are less likely to consent. Confidentiality concerns were proxied by income item non-response in Jenkins et al. (Citation2006), Sala et al. (Citation2012) and Singer, Hoewyk, and Neugebauer (Citation2003) and by a measure of ‘being a private person’ in Mostafa (Citation2016).
Finally, we test the influence of the interviewers who are in charge of administering the consent questions and explaining what consent to data linkage is. In general, since interviewers are incentivised to minimize unit non-response, obtaining respondent co-operation to consent to administrative data linkage is not their main goal. However, we are in a position to examine the role of the interviewers in obtaining consent.
In the next section, we present a brief outline of our data source, the MCS, the consent procedures, and the chosen methods designed to examine the influences affecting consent.
3. Data, consent procedures and methods
3.1. The Millennium Cohort Study
The MCS is the most recent of the British Cohort studies. It follows the lives of a nationally representative sample of more than 19,000 children born in the UK in 2000–2001. MCS has a complex survey design (Plewis, Citation2007). The sample is stratified by country, clustered at the electoral ward level, and oversamples minorities and disadvantaged groups. The primary sampling unit is the electoral ward. These were disproportionally stratified to ensure adequate representation of all countries of the UK (i.e. England, Scotland, Wales and Northern Ireland), of disadvantaged areas and of areas with high concentration of ethnic minorities. Survey data has been collected on five occasions when the cohort members (CMs) were 9 months, three, five, seven, and eleven years old. The main respondents (MRs) were mostly the mothers although very few have swapped with the fathers or other members of the household over time. Unsurprisingly, the sample has also experienced attrition over time.
The original study had 19,244 families who were interviewed at least once in waves 1 and 2, some of which had twins and triplets. Our analytical sample consists of 11,745 MRs who were present in waves 1, 2, and 4. Presence in wave 3 was not a qualifier for inclusion as the consent question from wave 2 was administered to non-consenters in this wave and has led to a consent rate close to 100%. Therefore wave 3 was discarded. The sample also excluded MRs who have changed over time (e.g. a switch from mother to father and vice versa). The participating MRs were interviewed by 328 interviewers in wave 1, by 334 interviewers in wave 2, and by 443 interviewers in wave 4.Footnote1 The sample design features are included in all analyses that follow.
The membership of our analytical sample remains constant over time, however there are changes in the distribution of key socio-demographic characteristics of this sample. These changes are provided in Table A1 of our Appendix 1. Broadly speaking, there is evidence for an increase in the number of single MRs over time, a rise in the proportion of MRs exercising managerial and self-employed jobs, and a decline in the proportion of those doing routine and technical jobs. The number of households where both parents are unemployed or at least one is employed has declined whereas the number of households with two working parents has increased. Similarly, and as expected, after 7 years, the number of house owners has increased while the number of those renting, living with parents, or living free of rent has declined. Moreover, the number of MRs reporting that they spoke only English at home has increased.
Regarding health, the number of MRs reporting excellent health has declined by just over 8% indicating that over time more MR’s have begun to report health problems as they age. The opposite happens for the CMs, where health concerns are more frequent in infancy (i.e. by age 9 months) and tend to subside in early childhood (i.e. after age 9 months). We now go on to describe the procedures for obtaining consent.
3.2. Consent procedures
Written consent was sought from MRs for linking their children’s health records in three waves (at age 9 months, 3 and 7 years). Consent was never sought directly from the CMs because they were too young. Prior to the interview, leaflets explaining what consent to administrative data linkage consists of were posted out to the MRs. All interviews were face to face, and all consent questions were administrated at the end of the main interview. Respondents who were willing to give consent were asked to tick a box containing two options: ‘yes’ or ‘no’, then sign print their names and date the form. The wording and the content of the consent question changed between waves 1 and 2. In wave 1, consent was sought to link information on pregnancy and birth and to follow the CM’s National Health Service (NHS) registration. In wave 2, consent was sought to link health records from birth to age 7. All consent forms made it clear that respondents could refuse to participate or withdraw from any part of the survey by simply expressing the wish to do so. All consent questions included a confirmation statement.Footnote2 The procedures, the leaflets and consent forms are presented in detail in the technical report on the Millennium Cohort Study, Ethical Review and Consent (Citation2012). The outcomes of interest are presented below in Table .
Table 1. Consent to link CM’s health records.
Wave 5 (age 11) was not included in the sequence of consent above because the health consent question was not asked in this wave as the consent obtained in wave 4 was valid until age 14. Also as mentioned earlier, the wave 3 consent question was a repeat of wave 2 and therefore was not included. In terms of fieldwork organisation, it is also worth emphasising that the survey agency carrying out the fieldwork changed between waves 1 and 2. This disruption might have affected the levels of consent since the interviewers and the survey agency fieldwork management procedures would also have changed. Finally, it is worth emphasising that consent was sought for the same outcome (i.e. health) for the same respondents over time.
3.3. Methods
The analytical methods used in this study are adopted to address the three research questions as described in the introduction. Firstly, the consistency of consent over three occasions can be described as a pattern of co-operation or not. Secondly, the exploration of the influences upon individual’s willingness to consent is more challenging and forms the strategy for addressing the second and third research questions. We set-out to achieve this in three ways: (i) by jointly estimating the three consent outcomes in order to reveal any association between them using a multivariate probit model (Cappellari & Jenkins, Citation2003) and by interpreting the cross-sectional influence of socio-demographic factors on consent; (ii) by applying a conditional probit model of consent to explore the changes in consent behaviour across two consecutive waves of data collection; and (iii) by considering the influence of the interviewers assigned to our study on consent. This is achieved by adopting a linear probability model in order to examine the impact of interviewers as ‘fixed effects’. All analyses were carried out in Stata 13. We now describe the analysis strategy in more detail.
The first analysis consists of a joint estimation of the three consent outcomes using a multivariate probit specification (i.e. three consent equations estimated jointly) closely adhering to the methodology adopted by Cappellari and Jenkins (Citation2003). This analysis allows for the computation of the cross-equation correlations (the strength of the association between the unobserved factors or error terms explaining each consent outcome) and the estimation of the effects of the correlates. The M-equation multivariate probit model is the following:
where yim is the binary consent outcome for respondent i and consent outcome m with m = 1, …, 3. x is a vector of independent variables for respondent i. ɛim, are error terms distributed as multivariate normal, each with a mean of zero and a variance-covariance matrix V, where V has values of 1 on the diagonal and values different to 1 off-diagonal. Note that Stata does not provide a ready-for-use command to estimate multivariate probit models. For this reason, we estimated the model using a maximum simulated likelihood procedure (MSL) similar to the one used in Cappellari and Jenkins (Citation2003), (Citation2006) and Mostafa (Citation2016). This procedure was adapted to take into account the complexity of the MCS survey design throught use of the svy command in Stata 13. Details on the procedure and the Stata syntax can be found in Cappellari and Jenkins (Citation2003, p. 178).
Since the unobserved circumstances of the interview are unlikely to be the same over time, it is possible to attribute the cross-equation correlations to the presence of a latent inclination to consent. In other words, the existence of significant associations between the latent parts of the different consent outcomes indicates the presence of unobserved factors (e.g. strong belief in the importance of scientific research, certain predispositions, satisficing behaviour, etc.) affecting consent over time.
The second analysis consists of conditional probit models designed to analyse the switch of behaviour between two consecutive waves. The dependent variable is a binary variable taking the value of 0 if the respondent had the same behaviour over two consecutive waves (was a consenter and remained a consenter, or was a non-consenter and remained a non-consenter) and 1 if the respondent switched behaviour (was a consenter and became a non-consenter or was a non-consenter and became a consenter). All four models are estimated with the right-hand side variables being the respondent’s characteristics in the initial waves (i.e. waves 1 or 2 depending on the model).
The first and second analyses do not consider the presence or influence of the interviewers on consent. This is addressed in our final analysis. The third analysis consists of three linear probability models designed to measure the rise in the models’ explanatory power after the inclusion of interviewer fixed effects (i.e. rise in adjusted R2). However, any rise in the adjusted R2 cannot be completely attributed to the impact of interviewers because their workload allocation is likely to be on a ‘nearest-to-home’ basis. Therefore, interviewer effects will be confounded by the social and geographical characteristics of interviewer assignment areas (e.g. some assignment areas may have large proportions of minorities, high levels of poverty or unemployment or ‘hard-to-reach’ respondents, whereas other areas may not). This challenge was overcome in an analysis by Mostafa (Citation2016) by controlling for the characteristics of assignment areas in addition to the inclusion of interviewers as fixed effects in the modelling. Three linear probability models (one for each wave) are estimated in three steps:
(1) | Base model: it included the MRs’ observed characteristics (same as previous analyses). | ||||
(2) | Model with area effects = Base model + characteristics of the interviewer’s assignment area. These are computed as averages of MRs’ characteristics at the level of the interviewer. They include the proportion of minorities, proportion unemployed, average log income, and social class composition. | ||||
(3) | Fixed effects model = Base model + interviewer fixed effects. The model excludes the assignment area characteristics since they are collinear with the fixed effects. |
We decided to use linear probability models for three reasons: first, because they allow for the measurement of the adjusted R2. Secondly, because probit models do not converge when hundreds of interviewer fixed effects are included (computational time also rises dramatically), and thirdly because interviewer identifiers are not consistent over time and would preclude using such identifiers in a longitudinal manner. Therefore, we measured the effect of interviewers separately instead of adding them to the aforementioned probit models. By modelling interviewers as ‘fixed effects’ we formally acknowledge that CMs are not randomly assigned to interviewers. Therefore, examining interviewer effect as a variance component in the modelling is ruled out.
The choice of covariates was motivated by the literature and by the fact that some of these characteristics were expected to vary over time. For instance, after seven years in the life of the survey, adult respondents are expected to have higher incomes, higher positions in their jobs, and a growing professional experience. It is also likely that some respondents have experienced divorce or separation and have started new relationships. Similarly, the number of house owners is expected to grow as young parents grow older. In terms of health, respondents are likely to have more health issues as they age while the reverse is likely to be true for children since most of the health problems happen after birth and progressively decline. In addition to time-varying socio-demographic characteristics, time-invariant characteristics include gender, ethnicity, personality (i.e. being a private person), and response history on the survey. Response history is a binary variable taking the value of 1 if the respondent was absent in at least one wave, it is used as a proxy for the respondent’s willingness to cooperate. For an in-depth description of the motivation behind the choice of the covariates, refer to Mostafa (Citation2016). All models take into account the MCS survey design features.
4. Findings
In what follows, we begin with a description of consent patterns over time, followed by interviewer’s success rates in obtaining consent. These descriptive accounts are followed by the regression results which are presented in the same order as outlined above as analyses (i)–(iii).
4.1. Consent patterns
Figure shows the existence of variations in consent over time. Consent rates for linking the CMs’ health records are the highest in wave 1 followed by wave 4 and the lowest in wave 2. There are a number of possible explanations. Firstly, there may be a tendency for less fieldwork effort to be put into obtaining consent per se when all of the focus of fieldwork management is on minimising unit non-response. This might well have been exacerbated by a change in fieldwork agencies between waves 1 and 2. Secondly, the drop in consent in wave 2 could also be attributed to the change in the content of the consent question. Wave 2 was the first time MRs were asked to link their children’s hospital records over a long period from birth to age 7. In wave one it was only birth records, and NHS registration used for tracing purposes.Footnote3
Figure 1. Consent rates for health record linkage in the three waves.
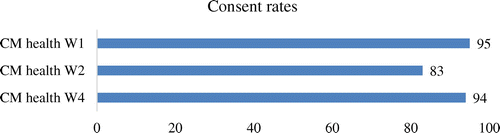
Table presents the consent patterns over time. In this table, a ‘1’ indicates that a respondent consented and ‘0’ otherwise. For instance, a pattern of 101 indicates that a respondent consented in wave 1, refused to consent in wave 2 and consented again in wave 4. The figures show that the data is dominated by two patterns: the majority (75.8%) of respondents who consented in all three waves (i.e. 111), and those who did not consent in wave 2 (i.e. 101, representing a significant minority (14%) of the sample). The remaining 10% illustrate other patterns of switching behaviour, whilst only a small minority of respondents (.5%) are non-consenters in all waves. It is also worth noting that from wave 1 to wave 2, 15.4% of consenters became non-consenters and 3.3% did the opposite. Similarly, from wave 2 to wave 4, 15% of non-consenters became consenters and 4.6% did the opposite. Respondents who switched from consenters to non-consenters between waves 1 and 2 are almost the same individuals as those who did the opposite between waves 2 and 4. Based on this evidence it is possible to say that there are sufficient changes in consent behaviour and in the characteristics of the sample over time to warrant exploring the temporal dimension of consent. Moreover, consent behaviour seems to be consistent over time since most respondents consented in all three waves.
Table 2. Consent patterns in waves 1, 2, and 4 of MCS.
4.2. Interviewer success rates in obtaining consent
Figure presents boxplots depicting interviewer success rate in obtaining consent to CM health data linkage in each of the three waves. The success rate is defined as the number of obtained consents for each interviewer divided by the number of achieved interviews, it ranges between 0 and 1. The number of interviews per interviewer ranged from 2 to 105 in wave 1 with an average number of 49; 2–145 in wave 2 with an average number of 57; and 2–71 in wave 4 with an average number of 33. Very few interviewers had a workload lower than 5 interviews (less than 5% of the interviewers in each wave). The 11,745 MRs were interviewed by 328 interviewers in wave 1, by 334 interviewers in wave 2, and by 443 interviewers in wave 4.
For each wave we see considerable dispersion in success rates amongst the bottom quartile of interviewers and the least dispersion in the upper quartile. This could reflect the dispersion in interviewers’ experience, with the less experienced having more variations in their success to obtain consent. Alternatively, interviewers with a limited number of achieved interviews were more likely to have low success rates. Secondly, for all three attempts to obtain consent, the outliers belong to the lowest quartile. Thirdly, the success rates in wave 2 are more dispersed for all quartiles than in the two other waves. This could be due to the change in the survey agency in wave 2 which could have led to higher dispersions. In summary, the existence of wide variations in success rates between individual interviewers warrants the measurement of their collective impact.
4.3. Regression findings
This section presents the results from the regression analyses. In Figure , the estimated cross-equation correlations from the first analysis are presented. As mentioned earlier, these correlations are obtained through the joint modelling of the three consent outcomes using a multivariate probit model. The correlations measure the strength of the association between the unobserved factors explaining each consent. Since the consent outcomes were sought in different waves, the circumstances surrounding the interviews are likely to be different. Therefore, these correlations can be attributed to the existence of a latent inclination to consent reflecting stable respondent characteristics such as strongly held convictions, predispositions and satisficing behaviour.
Figure 3. Cross-equation correlations based on a multivariate probit model.
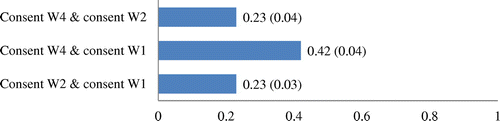
Our evidence suggests that the correlations between the unobserved parts of the consent outcomes are not very strong ranging between .2 and .4 across adjacent and non-adjacent waves, even though they are statistically significant at p < .01. Hence, there is some indication that a weak/moderate latent inclination to consent exists. This finding does not contradict the fact that most respondents consistently consented in all three waves. However, what it indicates is that such a consistent behaviour is weakly driven by latent characteristics and predispositions. A possible explanation is that respondents behave passively and simply consent when prompted by the interviewer. They could have also forgotten what they did in the past especially that consent is not an important decision in their lives. Note that the correlation between consent in wave 1 and wave 4 is higher than the other two combinations. One possible explanation is that the change of the survey agency in wave 2 has affected the collection of consent in a way that inhibited the influence of respondents’ latent characteristics.
In Table , the estimated regression coefficients from the first analysis (i.e. multivariate probit model) are presented to show the impact of our selected socio-demographic characteristics on the willingness to consent in each of waves 1, 2, and 3. In general, the results in Table show that socially disadvantaged groups (those MRs with low SES and members of ethno-linguistic minorities), are less likely to consent. This is in line with previous empirical evidence (Jenkins et al., Citation2006; Mostafa, Citation2016 and Sakshaug et al., Citation2012). Sheldon et al. (Citation2007) suggest that disadvantaged respondents tend to be less cooperative and provide an argument that this represents disengagement from government to official institutions, low literacy, and communication barriers in the case of some ethnic minorities.
Table 3. Cross-sectional influences of socio-demographic characteristics on the willingness to consent using a multivariate probit model.
In Table , the probability of switching consent behaviour between two consecutive waves is modelled using a conditional probit approach (second analysis). The dependent variable is a binary variable taking the value of 0 if the respondent had the same behaviour over two consecutive waves and 1 if the respondent switched behaviour. Note that the analytical samples were restricted to consenters or non-consenters in the initial wave depending on the model. Table shows that some of the covariates have a strong and significant impact on the likelihood of switching behaviour over time.
Table 4. Conditional probit regressions of changing consent decisions over consecutive waves.
Taking the results of the conditional probit model (Table ) together with those in Table we find the latter largely supports the former.
The results of Table show that respondents holding routine, technical and supervisory jobs are less likely to consent than those doing managerial and professional jobs. The presence of enough variation in the dependent variable in wave 2 (wave 2 has the lowest consent rate of 83%), could be the reason why the results were only significant in this wave. The results are confirmed by the conditional probit model (Table ) in which respondents from the three lower SES groups are more likely to switch from being consenters to non-consenters between waves 1 and 2.
Respondents from non-White backgrounds are less likely to consent in waves 1 and 4. Table also shows that ethnic minority respondents are more likely to switch from being consenters to being non-consenters between waves 2 and 4.
When it comes to religion, non-Christians were found to be more likely to consent in wave 2. Similarly, non-Christian respondents are less likely to switch from being consenters to being non-consenters between waves 1 and 2, while respondents with no religious affiliation were more likely to do the opposite between waves 2 and 4. It is worth noting that ethnicity and religion work in opposite directions. The reason could be that, after controlling for ethnicity, religion will account for certain predispositions and attitudes. This coincides with the finding that respondents belonging to religious groups which are more demanding in their rituals, are more likely to cooperate (Levy & Razin, Citation2012).
Those who had a translated interview were less likely to consent in wave 1. The negative effect of this variable is an indication that communication barriers might hinder consent. They were also likely to switch (Table ) from being non-consenters to being consenters between waves 2 and 4.
MRs who report no health problems for the CM in wave 1 are less likely to consent than those who report some problems. They are also more likely to switch from being non-consenters in wave 1 to being consenters in wave 2. This finding is in line with the results of Mostafa (Citation2016). MR’s with CMs suffering from health problems have previous experiences with the healthcare system (i.e. the institutions holding the health records). Therefore, they are more likely to cooperate since providing access to their children’s medical records might help advance medical research and improve services. The significance of the effect only in wave 1 in addition to the switch in behaviour between waves 1 and 2 is possibly the result of variations in health status over time. As shown in Table A1, more CMs suffer from health issues during the first 9 months after birth (about 42% of the sample). These concerns tend to decline over time with only 13% of MRs reporting that the CM had health problems by age 7. Hence, fewer CMs had health issues in waves 2 and 4. The decline in variations in health status could have led to the non-significant effect in waves 2 and 4 and to the switch in behaviour. This finding highlights the value added of considering changes in the sample composition over time. This feature would be lost in a cross-sectional analysis.
When it comes to privacy concerns, the effects are mostly non-significant in the multivariate probit regressions. In the conditional probit model, those who report that they are less private (i.e. agree) were less likely to switch from being consenters to being non-consenters (between waves 1 and 2). Moreover, MRs who missed at least one wave of MCS are less likely to consent in wave 4 while those who have higher incomes are slightly more likely to consent in wave 2.
All other covariates such as CM’s gender, housing tenure, language spoken at home, and MR’s self-reported health tend not to have any statistically significant impact on consent. It is also worth noting that no one covariate has a consistently significant effect in all waves. The effects and significance of individual covariates vary over time. These variations in significance levels could be due to the fluctuations in consent rates caused by the change in the survey agency in wave 2 (note that the data is dominated by two patterns 111 and 101). Therefore, it is possible to say that in the event of an external shock to the survey, such as the change of the survey agency, the loss of consenters is more likely to happen among the socially disadvantaged (low SES, ethnic minority groups) as shown in Table . This indicates that fieldwork practices might have differential effects according to the characteristics of respondents. Furthermore, since social disadvantage is directly related to health outcomes, this will lead to bias in sample composition in the linked survey and administrative data. In other words, we will lose CMs with health problems since they are more likely to come from disadvantaged groups whose probability of consent is lower.
In the third strand of analyses, the impact of interviewers is measured using three linear probability models. All models are cross-sectional and examine each consent outcome separately. The base model includes the aforementioned covariates without interviewers fixed effects. The model with area effects is identical to the base model and includes the characteristics of the interviewer’s assignment area. The fixed effects (FE) model is equivalent to the base model and includes the interviewers’ fixed effects.
In Table , the comparison of the first three columns indicates by how much the explanatory power of the model (as measured by the adjusted R2) has changed after the inclusion of assignment area characteristics (third column) and interviewer fixed effects (fourth column). The results show that the explanatory power of the base model is limited (adjusted R2 varies between 1 and 3.2% depending on the wave). When the area characteristics were included, the explanatory power only rose by a small amount. However, when interviewer’s fixed effects were added, the explanatory power rose by a much larger amount (i.e. 2–10 times) even though the adjusted R2 is still modest in magnitude. The dramatic rise in wave 2 is possibly the result of the change of the fieldwork agency. In other words, in wave 2, new interviewers from a different agency were contracted to the study. This has resulted in more between-interviewer variation and in a rise in their impact. This rise has persisted in wave 4. Moreover, interviewers were probably incentivised to minimize unit non-response. Therefore, consent was not the main priority and this has led to more between-interviewer variation in obtaining consent.
Table 5. Interviewers’ effects for health record consent outcomes.
5. Conclusion
Despite the growing number of studies dealing with consent to link survey and administrative data, there is very limited knowledge of how consent works over time. This study constitutes the first exploration of consent mechanisms using three waves of data collection from the MCS spanning 7 years of the lives of the cohort members. The study examines consistency in consent behaviour over time and explores three factors which affect consent: the respondents’ latent characteristics and predispositions, the respondents’ observed characteristics, and the impact of the interviewers.
Firstly, consent rates show that most respondents (i.e. 76.5%) do behave consistently over time. Secondly, the cross-equation correlations from the first analysis show that the unobserved parts of the consent outcomes are weakly associated over time, and therefore, cannot really be held to indicate the existence of a strong latent inclination to consent reflecting the influence of latent personal characteristics and predispositions. In other words, once the observed respondent characteristics are taken into account there is very little to suggest that there are time-stable unobserved factors which influence a MR’s inclination to consent. Thirdly, the likelihood of consent and the likelihood of switching behaviour over time are related to the respondents’ circumstances, and to the variation in the impact interviewers have on the MRs willingness to consent. Taken together these three findings indicate that, for the majority of respondents, consent is not driven by stable latent characteristics and predispositions, but rather depends on the circumstances of the respondents at the time of the interview and on the potential influence of the interviewers and changes to the survey fieldwork practices.
Our findings also show that when consent rates drop because of an ‘external shock’ to the survey procedures, in this case a change in the survey agency, the loss of consenters is more likely to happen among the socially disadvantaged. This highlights the importance of maintaining stability in survey fieldwork and emphasising the need to obtain consent. Moreover, given the effect of the respondents’ social background on the likelihood of consent there may be a case for oversampling certain groups or making statistical adjustments for changing sample composition over time.
Our findings suggest that interviewers have an important role to play in securing consent. Future research could examine the interaction between the interviewer characteristics and those of the respondents in addition to exploring the effect of interviewer training. Ideally, this would require experimental designs where CMs are randomly assigned to interviewers (Dijkstra, Citation1987). In addition, it would be informative to ascertain whether the existence of motivational or attitudinal factors distinguish the willingness to consent to administrative record linkage from the willingness to co-operate as respondents in general.
Disclosure statement
No potential conflict of interest was reported by the author.
Funding
This work was funded by the Economic and Social Research Council and the National Centre for Research Methods (Research Grant No. R00960-01-001-1) and the Economic and Social Research Council’s Centre for Longitudinal Studies (Grant No. ES/M001660/1).
Notes on contributors
Tarek Mostafa is a research officer in Centre for Longitudinal Studies, UCL, at Institute of Education. Mostafa’s interests include Economics of education, survey and quantitative methods.
Richard D Wiggins is a professor, UCL, at Institute of Education. Wiggin’s interests include Survey and quantitative methods.
Acknowledgement
The authors would like to thank Professors John Micklewright and Lucinda Platt for their valuable comments.
Notes
1. For more information on sampling, response, and on how to use MCS refer to: the MCS technical report on sampling, the MCS technical report on Response, and the MCS user guide for analysing MCS data in Stata. Note that 6918 respondents were excluded because they dropped out from the survey in one or more waves. Respondents who have changed over time were also excluded (420 cases) in addition to twins and triplets (161 cases).
2. Example of wave 4 confirmation statement: I have read or heard the information leaflet about information from other sources and have had the opportunity to ask questions. I understand the information released will be treated in strict confidence in accordance with the Data Protection Act and used for research purposes only. I understand that this consent will remain valid unless revoked by me in writing and that I may withdraw my consent at any time by contacting the Child of the New Century in writing to the address below, without giving any reasons. (MCS4 consent forms).
3. Table B1 in the Appendix 1 provides weighted estimates of percentages of consenters for some of the key variables included in the analyses. In general, it shows that consenters and non-consenters differ along the lines of SES, employment status, housing tenure, ethnicity, language spoken at home, self-reported health, and whether the interview was translated.
References
- Al Baghal, T. (2016). Obtaining data linkage consent for children: Factors influencing outcomes and potential biases. International Journal of Social Research Methodology, 19, 623–643.10.1080/13645579.2015.1064635
- Armstrong, V., Julie, B., Helen, C., Michelle, M., Moran-Ellis, J., & Shepherd, R. (2008). Public perspectives on the governance of biomedical research: A qualitative study in a deliberative context. London: Wellcome Trust.
- Baker, R., Shiels, C., Stevenson, K., Fraser, R., & Stone, M. (2000). What proportion of patients refuse consent to data collection from their records for research purposes? British Journal of General Practice, 50, 655–656.
- Cappellari, L., & Jenkins, S. (2003). Multivariate probit regression using simulated maximum likelihood. The Stata Journal, 3, 278–294.
- Cappellari, L., & Jenkins, S. (2006). Calculation of multivariate normal probabilities by simulation, with applications to maximum simulated likelihood estimation. The Stata Journal, 6, 156–189.
- Cialdini, R., Wosinska, W., Barrett, D., Butner, J., & Gornik-Durose, M. (1999). Compliance with a request in two cultures: The differential influence of social proof and commitment/consistency on collectivists and individualists. Personality and Social Psychology Bulletin, 25, 1242–1253.10.1177/0146167299258006
- Dijkstra, W. (1987). Interviewing style and respondent behaviour: An experimental study of the survey-interview. Sociological Methods and Research, 16, 309–334.10.1177/0049124187016002006
- Dunn, K., Jordan, K., Lacey, R., Shapley, M., & Jinks, C. (2004). Patterns of consent in epidemiologic research: Evidence from over 25,000 responders. American Journal of Epidemiology, 159, 1087–1094.10.1093/aje/kwh141
- Festinger, L. (1957). A theory of cognitive dissonance. Stanford, CA: Stanford University Press.
- Heider, F. (1958). The psychology of interpersonal relations. New York, NY: Wiley.10.1037/10628-000
- Huang, N., Shih, S., Chang, H., & Chou, Y. (2007). Record linkage research and informed consent: Who consents? BMC Health Services Research, 7, 18.10.1186/1472-6963-7-18
- Jenkins, S., Cappellari, L., Lynn, P., Jäckle, A., & Sala, E. (2006). Patterns of consent: Evidence from a general household survey. Journal of the Royal Statistical Society Series A, 169, 701–722.10.1111/rssa.2006.169.issue-4
- Kho, M., Duffett, M., Willison, D., Cook, D., & Brouwers, M. (2009). Written informed consent and selection bias in observational studies using medical records: Systematic review. British Medical Journal, 338, b866.10.1136/bmj.b866
- Korbmacher, J., & Schroeder, M. (2013). Consent when linking survey data with administrative records: the role of the interviewer. Survey Research Methods, 7, 115–131.
- Levy, G., & Razin, R. (2012). Religious beliefs, religious participation, and cooperation. American Economic Journal: Microeconomics, 4, 121–151.
- MCS Technical Report on Response. (2010). (3rd ed.), CLS. Retrieved from http://www.cls.ioe.ac.uk/shared/get-file.ashx?id=607&itemtype=document
- MCS Technical Report on Sampling. (2007). (4th ed.), CLS. Retrieved from http://www.cls.ioe.ac.uk/shared/get-file.ashx?id=409&itemtype=document
- Millennium Cohort Study, Ethical Review and Consent. (2012). Retrieved from http://www.cls.ioe.ac.uk/shared/get-file.ashx?id=1601&itemtype=document
- Mostafa, T. (2016). Variation within households in consent to link survey data to administrative records: Evidence from the UK Millennium Cohort Study. International Journal of Social Research Methodology, 19, 355–375.10.1080/13645579.2015.1019264
- Nelson, K. Elena, Garcia, R., Brown, J., Mangione, C. M., Louis, T. A., Keeler, E., & Cretin, S. (2002). Do patient consent procedures affect participation rates in health services research? Medical Care, 40, 283–288.10.1097/00005650-200204000-00004
- Newcomb, T. M. (1953). An approach to the study of communicative acts. Psychological Review, 60, 393–404.10.1037/h0063098
- Olson, J. A. (1999). Linkages with data from social security administrative records in the health and retirement study. Social Security Bulletin, 62, 73–85.
- Plewis, I. (2007). (Ed). The Millennium Cohort Study: Technical report on sampling (4th ed.), London: Centre for Longitudinal Studies. Retrieved from http://www.cls.ioe.ac.uk/shared/get-file.ashx?id=409&itemtype=document
- Sakshaug, J. & Kreuter, F. (2012). Assessing the magnitude of non-consent biases in linked survey and administrative data. Survey Research Methods, 6, 113–122.
- Sakshaug, J., Couper, M., Ofstedal, M., & Weir, D. (2012). Linking survey and administrative records mechanisms of consent. Sociological Methods and Research, 41, 535–569.10.1177/0049124112460381
- Sakshaug, J., Tutz, V., & Kreuter, F. (2013). Placement, wording, and interviewers: Identifying correlates of consent to link survey and administrative data. Survey Research Methods, 7, 133–144.
- Sala, E., Burton, J., & Knies, G. (2012). Correlates of obtaining informed consent to data linkage: respondent, interview and interviewer characteristics. Sociological Methods and Research, 41, 414–439.10.1177/0049124112457330
- Sala, E., Burton, J., & Knies, G. (2014). Inclination to consent to data linkage: Experimental evidence on the role of three survey design features in a UK longitudinal panel. International Journal of Social Research Methodology, 17, 455–473.10.1080/13645579.2014.899101
- Schröder, H., Kleudgen, M., Steinwede, J., March, S., Swart, E., & Stallmann, C. (2015). Data linkage – respondents consent without selectivity? Gesundheitswesen, 77, 57–62.
- Sheldon, H. Graham, C. Pothecary, N. and Rasul, F. (2007). Increasing response rates amongst black and minority ethnic and seldom heard groups. Picker Institute Europe. Retrieved from http://www.nhssurveys.org/Filestore/documents/Increasing_response_rates_literature_review.pdf
- Silva, M. S., Smith, W. T., & Bammer, G. (2002). The effect of timing when seeking permission to access personal health services utilization records. Annals of Epidemiology, 12, 326–330.10.1016/S1047-2797(01)00261-7
- Singer, E. Van, Hoewyk, J., & Neugebauer, R. J. (2003). Attitudes and behaviour: The impact of privacy and confidentiality concerns on participation in the 2000 Census. Public Opinion. Quarterly, 67, 368–384.10.1086/377465
- User Guide to Analysing MCS Data Using STATA. (2000). 1st ed., CLS. Retrieved from http://www.cls.ioe.ac.uk/shared/get-file.ashx?id=1372&itemtype=document
- Woolf, S. H., Rothemich, S. F., Johnson, R. E., & Marsland, D. W. (2000). Selection bias from requiring patients to give consent to examine data for health services research. Archives of Family Medicine, 9, 1111–1118.10.1001/archfami.9.10.1111
Appendix
Table A1. Changes in the characteristics of the sample over time.
The analytical sample consists of 11,745 MRs observed three times (35,235 observations in total).
Table B1. Health record consent rates for each socio-demographic group.
Table b provides weighted estimates of percentages of consenters for some of the key variables included in the analyses. Note that the percentage of non-consenters for each category is equal to 100 minus the percentage of consenters. Figures in bold indicate that consenters are statistically different from non-consenters according to the variable of interest, and this difference is significant at least at the level of p < .1.