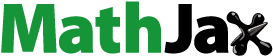
ABSTRACT
Nonprobability web surveys suffer from selection and coverage biases and are generally not representative of the target population. To carry out statistical modelling in a nonprobability web survey, we explore different methods of statistical adjustments to compensate for biases through the use of a probability-based reference sample. We also show that we need to account for these biases when imputing missing data. The methods for statistical adjustments include propensity score weighting with post-stratification and a technique called sample matching. For the substantive study, we use a nonprobability online web-survey taken from the WageIndicator (WI) programme (www.wageindicator.org) to estimate the gender pay gap (GPG) using the 2016 WI survey data from the Netherlands. We use the 2016 EU-SILC data as the probability-based reference sample. Based on the study of GPG, we show that using propensity score weighting with post-stratification outperforms sample matching with respect to compensating for biases and improves the outcome of the Blinder–Oaxaca decomposition model in terms of the degree of similarity relative to patterns found in representative probability samples in the Netherlands.
1. Introduction
The landscape for collecting survey data has been changing rapidly in the past two decades with online web surveys becoming increasingly popular compared to standard data collections such as face-to-face surveys, mail surveys, and telephone surveys. The advantages of using web surveys have many factors. Costs for a web survey are usually lower compared to other means of survey data collection as it does not require as much resources for interviewers or printing questionnaires. The development of social media and telecommunication technologies means that web surveys can be launched quickly, distributed in various ways and easily reach a large population (Bethlehem, Citation2010). Another feature of internet-based surveys is that they provide a higher sense of anonymity for the participant than the presence of interviewers (Braunsberger et al., Citation2007) although they have been shown to be more likely to suffer problems associated with satisficing (Revilla & Ochoa, Citation2015; Stolte, Citation1994; Sue & Ritter, Citation2012). It thus allows researchers to collect data more efficiently and capture more recent memories of the respondents.
In contrast, online web surveys have serious limitations as they are not typically grounded in probabilistic methods of sampling and hence there are barriers when the aim is to analyse and provide statistical inference to a target population. One of the key issues associated with online data collection is self-selection. Self-selection occurs when data collectors are not in control of the selection process and it is largely or completely left to individuals to select themselves into the survey. Under-coverage is also a serious problem when people do not have access to the internet. Self-selection and under-coverage are determined by factors such as computer literacy, internet penetration, and interest to participate, which are rarely evenly distributed in the population. In addition, it is not possible to calculate response rates or understand the structure of the sample relative to the target population (Schleyer & Forrest, Citation2000). Consequently, while probability sampling enables statistical analyses to produce accurate and unbiased estimates of the target population, self-selection can ‘lead to biased estimates, and therefore wrong conclusions are drawn from the collected data’ (Bethlehem, Citation2010). It is thus important for social scientists to address shortcomings of an online web survey and develop innovative approaches to improve analyses and inference using such data.
One online web survey supported by the European Union’s Horizon 2020 research and innovation programme under grant agreement No 730,998 (InGRID-2 Integrating Research Infrastructure for European expertise on Inclusive Growth from data to policy) is the WageIndicator (WI) programme (www.wageindicator.org). The purpose of this funding was to make an evidence-based contribution to a European policy strategy of inclusive growth where the focus was on social in/exclusion, vulnerability-at-work and related social and labour market policies from a European comparative perspective. The WageIndicator (WI) programme was initiated in The Netherlands in 2001 as a platform for employees and employers looking for information about income. In 2020, the WI organization operated in over 80 countries worldwide. According to Wageindicator.org, the WI web survey identifies the labour force as its target population. The respondents of these multilingual web-survey are volunteers recruited through national WI websites and a wide range of websites of WI partners. The standard version of the WI survey requires approximately 10–20 minutes to complete (Tijdens et al., Citation2010) and participants are incentivized to complete the survey with the opportunity to win a cash prize. The WI web survey is managed in annual releases since 2006 and can generate large sample sizes. Apart from questions on real wage data, working conditions, and demographic characteristics, WI web surveys also cover a wide range of topics related to job and life satisfaction, work-life balance and health, which makes it a unique data source for a variety of economic and sociological studies (Smyk et al., Citation2018). The WI web survey has incubated a large volume of comparative studies in various fields of social sciences globally.
In this paper, we address the question of whether we can adjust an online web survey, such as the WageIndicator (WI) data, to allow for statistical modelling and inference to a target population. We present here approaches to adjust the online web survey using the 2016 Netherlands WI data. To adjust for selection bias in this data, it is necessary to identify a probability reference sample which can be used for sample matching or propensity score estimation and post-stratification (Elliot & Valliant, Citation2017; Lee & Valliant, Citation2009; Valliant & Dever, Citation2011; Wang et al., Citation2021; Wu, Citation2022) and for this purpose we use the 2016 European Union Statistics on Income and Living Conditions (EU-SILC) for the Netherlands. After adjustments, we will then demonstrate the use of the online web survey to answer a substantive research question related to measuring the gender pay gap (GPG) according to the variable log hourly wage. The gender pay gap (GPG) is the relative difference between average hourly wage of all women and men across a workforce. For example, if women do more less-well paid jobs compared to men, then this increases the GPG. To account for differences in characteristics of women and men in the labour market, we use the Blinder–Oaxaca decomposition method (Blinder, Citation1973; Boll & Lagemann, Citation2018; Leythienne & Ronkowski, Citation2018; Oaxaca, Citation1973) to isolate the contribution of each characteristic to the GPG.
Section 2 describes the data in more detail: the 2016 Netherlands WI web survey data and the 2016 Netherlands EU-SILC data that will be used in this analysis. Section 3 provides approaches for weighting adjustments and imputation in the nonprobability WI web survey data to account for the selection bias and Section 4 provides an evaluation and comparison of different approaches. Section 5 presents results of the application on measuring the 2016 GPG for the Netherlands. We conclude in Section 6.
2. Data
2.1. The wage indicator survey data
Existing studies have taken advantage of WI’s extensive coverage to undertake research exploring labour markets in different parts of the world. Not surprisingly, wage is arguably the most popular topic amongst these studies. Examples include comparative analysis of minimum wage representation in Asian countries (Varkkey et al., Citation2016), living wage in Europe (Fabo & Belli, Citation2017), and labour market outcomes in Sub-Saharan African countries (Tijdens et al., Citation2015). These studies are often exploratory and presented in reports produced by the WI organization and supporting institutions.
Other studies in the WI program are focused on methodological questions and whether the online survey data are suitable for rigorous academic research. When compared to national representative databases, the WI survey data were shown to be unable to represent the general population – patterns of wage distributions in the web survey and benchmark national representative surveys were largely distinct (Steinmetz et al., Citation2009). Earlier research used different correction approaches to the WI data including post-stratification weighting and inverse propensity scores to improve the representativeness of the data (Steinmetz & Tijdens, Citation2009; Steinmetz et al., Citation2009, Citation2013). In the study shown in Steinmetz et al. (Citation2009), the authors reviewed weighting methods comparing the German (Lohnspiegel) WI data (N = 21,914) with the reference sample German Socio-Economic Panel (SOEP) (N = 7,993) and the Netherlands (Loonwijzer) WI data (N = 8,015) with the reference sample OSA Labour Supply Panel (OSA Arbeidsaanbodpanel) (N = 2,019). The authors compared post-stratification weighting methods and inverse propensity score weighting methods using a series of different models. In their conclusions, the authors reveal that the impact of using balancing variables to benchmark the WI data was limited and did not make web survey data more comparable to the general population. This held for the German as well as for the Netherlands sample. With respect to the findings of propensity score weighting as a possible solution to adjust for selection bias, particularly for the attitudinal questions of the WI data, the authors found only minimal changes. Their study does find that there was some degree of accounting for selection bias when variables of interest are included in the model. In our approach presented in this paper, we use the EU-SILC data as a reference sample for the Netherlands WI data, a large cross-sectional probability based reference sample, and take into account the survey weights of the EU-SILC in the propensity score models. We also first compensate for missing data using proper imputations and then use both the inverse propensity weighting and post-stratification together in a final set of weights. Our application involves a quantitative variable of interest that investigates wage disparities, as opposed to attitudinal data, and ensures that all relevant variables in the substantive research are included in both the propensity score models and the post-stratification.
While several techniques were developed in methodological research to examine and improve the reliability of the WI data, they were still insufficiently engaged with content research on ‘real-life’ social and economic issues (Kureková et al., Citation2015). Hence, a potential direction for the future advance of studies using the WI web data is to apply innovative methodological tools to improve the design and results of content research based on this data. In this present study, we analyse the gender pay gap (GPG) using the 2016 Netherlands WI data as it has a large sample size (n = 24,267) and provides detailed variables to carry out GPG research such as individuals’ hourly wage, education, and occupation. However, like many other web surveys, the WI data includes a high percentage of missing data. We selected from the 2016 Netherlands WI data those that are employed or self-employed (n = 22,913). The minimum age in the data was 18. We also deleted outliers with very small (less than 1 Euro) or very large hourly wage (greater than 300 Euro) that did not seem feasible. This is due to the WI being an online survey with no interventions from interviewers to validate the data and therefore there is potential for erroneous responses. The final sample size was 22,643.
2.2. European Union statistics on income and living conditions (EU-SILC)
One of the main purposes of this study is to examine whether and how statistical intervention would improve the representativeness of web survey data as compared to representative surveys. We therefore use the 2016 Netherlands EU-SILC as a reference sample to the Netherlands 2016 WI data having a total sample size of 24,123 individuals over the age of 18. EU-SILC contains microdata on key socioeconomic issues such as income, labour market, and living conditions across EU member states. From this data, we selected only the employed and self-employed with a minimum of age of 18 to be consistent with the WI data. This led to a sample size of 21,556 individuals. Furthermore, we deleted cases where there were missing data on the variables of the study as shown in . There were 4% missing data on education, 12% missing data on employment status, 14% missing data on Major ISCO, and 38% missing data on wages. The final sample size was 12,096 (56.1% of the original sample).
Table 1. Descriptive results of the original (complete case) 2016 Netherlands EU-SILC, original 2016 Netherlands WI web survey data and the adjusted datasets: Weight/PMM, PMM/Weight and sample matching approaches.
In the first two columns of are the descriptive statistics for the key variables that will be used in the analysis of the GPG for the 2016 Netherlands WI data and the 2016 Netherlands EU-SILC data.
3. Methodological strategies to improve the reliability of the web-survey
As mentioned in the introduction, nonprobability online web surveys suffer from selection and coverage biases as the sampling mechanism is not under the control of the researcher and the self-selection is not random. Therefore, without statistical adjustments to the data, it is not possible to generalize to a target population, calculate estimates and confidence intervals or carry out statistical inference (Bethlehem, Citation2010). In addition, given that there is no contact with an interviewer or quality checks on the data, online web surveys may be subject to item missing data. In this section, we describe ways to adjust for the selection and coverage biases inherent in nonprobability web surveys and approaches to carry out imputations for item missing data.
There are generally two approaches to compensate for biases to allow for inference in a nonprobability sample: a model-based approach and a quasi-randomization approach that integrates the nonprobability sample with a probability reference sample. For an overview of different approaches, see Baker et al. (Citation2013), Elliot and Valliant (Citation2017), Wu (Citation2022), Kim et al. (Citation2021), Chen et al. (Citation2022) and references therein. Quasi-randomization approaches include two main techniques: sample matching and post-hoc adjustments through propensity score adjustments. Both require the use of a probability reference sample. In sample matching, a nonprobability sample is drawn with similar characteristics to a target probability-based sample and the former uses the selection probabilities of the latter to weight the final data (Kim et al., Citation2021; Liu & Valliant, Citation2023; Rivers & Bailey, Citation2009). Units are matched in the nonprobability sample to a probability reference sample based on a set of variables that explain both participation and the outcome variables so that the covariates are balanced. Then, inference is carried out using the survey weights of the probability reference sample.
Propensity score matching was first introduced by Rosenbaum and Rubin (Citation1983, Citation1984). In the case of a nonprobability web survey, propensity scores may be estimated by modelling a probability of whether individuals participate in the web survey. Since the potential respondents of a web survey can be dichotomized as two groups (those who will participate and those who will not), propensity score matching attempts to make the two groups comparable by simultaneously controlling for all variables that are thought to explain the participation and target variables (Bethlehem, Citation2010). In this approach, the nonprobability sample and the probability reference samples are stacked where the response variable is an indicator variable equal to 1 for the nonprobability sample and 0 otherwise. The probabilities of participation are estimated using a logistic regression model where explanatory variables explain both participation and key outcome variables (Chen et al., Citation2020; Lee, Citation2006; Lee & Valliant, Citation2009; Wang et al., Citation2021; Wu, Citation2022).
The predicted probabilities are then used in the calculation of pseudo-design weights for the nonprobability sample, for example taking their inverse or constructing propensity score stratification. This is followed by post-stratification where we use auxiliary variables (from the population or from the reference sample) to benchmark the pseudo-design weights and further reduce selection and coverage biases (Bethlehem, Citation2010).
Apart from the issue of representativeness, selection and coverage biases, another challenge for nonprobability web survey data is that it may have a large number of missing values in the dataset owing to the voluntary nature of the web survey. While various types of imputation strategies such as hot deck (HD) and multiple imputation (MI) are widely used in the case of probability samples, the imputation process for nonprobability samples is more complicated. Compensating for missing data in nonprobability samples needs to take into account the weight adjustments to correct for the biases.
It is worth noting that, while the methods discussed above provide valuable ways to explain and compensate for the selection mechanism that led to inclusion and missing data in the nonprobability sample, there are still relatively few studies that use large web survey data to exploit the performance of these methods in a comprehensive and comparative manner. Again, methodological advancements in the study of nonprobability web survey data will become increasingly valuable in the midst of the growing popularity of cheaper, faster, and safer online data collection methods amongst individual researchers and research organization.
3.1. Adjustment weights
We demonstrate the quasi-randomization approach to account for the selection bias in the 2016 Netherlands WI dataset where the two techniques are sample matching and post-hoc adjustments using propensity scores. Both techniques require the use of a reference sample and here we use the 2016 Netherlands EU-SILC data.
3.1.1. Sample matching
In sample matching, we first calculate a propensity score to estimate the probability of participation for the nonprobability WI dataset. The WI dataset is stacked to the EU-SILC dataset and we define if i is in the WI dataset, otherwise
. Using a logistic regression model, we estimate a propensity score of participation:
where is a vector of covariates that are common in both datasets. The covariates are: age group (18–25, 26–35, 36–45, 46–55, 56–65, 66+), sex (Males, Females), employment (Employed, Self-employed), education (Elementary, Secondary, Tertiary, Missing), occupation (Manager, Professional, Technician, Clerical, Service sales, Agricultural, Craft/trade, Operators, Elementary, Missing). Recall that the EU-SILC data did not include missing data on the covariates. Then, within strata defined by sex and age group, we found the record in the WI dataset and the record in the EU-SILC data having the closest propensity score and copied the WI log hourly wage to the EU-SILC record (we did not include those cases where WI log hourly wage was missing). It was possible to have up to 10 multiple donors from the WI dataset that were contributing their WI log hourly wage value to the EU-SILC data. For the substantive analysis on GPG, the sample weights and all covariates used were those of the EU-SILC data, but the response variable of log hourly wage is from the WI dataset.
3.1.2. Propensity score adjustment
Step 1: We first calculate a propensity score to estimate the probability of participation for the nonprobability WI dataset. The WI dataset is stacked to the EU-SILC dataset similar to the sample matching approach and we use the same covariates. To calculate the propensity score we utilise the sample design and survey weights of the EU-SILC according to the method proposed in Chen et al. (Citation2020) summarized here.
We denote the WI data as file A and the EU-SILC as file B. We define if
and
if
. The estimator for the propensity score
is
where
maximizes the log-likelihood function
Since we do not observe the whole population, Chen et al. (Citation2020) replace the second term in (1) with the Horvitz- Thompson estimator obtained from the reference sample having survey weights and with information on
, to maximize the pseudo log-likelihood function
Under a logistic regression model, the pseudo-likelihood function is
And the score equations:
Chen et al. (Citation2020) propose a Newton-Raphson procedure. Letting denote the estimate of
at the
iteration, we have
where
and setting
for the first iteration. We then define the initial weights
as the inverse of the estimated propensity scores.
We emphasize that the approach for calculating adjustment weights based on propensity scores includes some assumptions that are hard to validate (Chen et al., Citation2020, Section 2.1). These assumptions are: (1) the selection mechanism for and the target variable of interest (in this case, income) are independent given the set of covariates
, (2) all units have a non-zero propensity score, (3)
and
are independent given
and
for
The second assumption is particularly hard to validate due to the fact that individuals participating in a web survey would need access to the internet to complete the survey. Therefore, there may still be underlying biases in the WI data even after calculating the adjustment weights.
Step 2: The final step is to benchmark the WI survey to the EU-SILC data using post-stratification where the totals for the post-strata are obtained from the weighted EU-SILC data according to their final survey weights :
(see expression (2)) where h denote the post-strata. The final weight for an individual i in strata h in the WI dataset is then:
Here, the post-stratification is based on the 5 covariates that were used to estimate the propensity scores, although due to small sample sizes we used the raking method to fit two sets of margins separately: sex*age group*education and employment*occupation. The final weights were normalized to the EU-SILC sample size for use in the substantive analysis.
3.2. Imputation for item missing data
The calculation of adjustment weights based on propensity scores for the WI dataset via the EU-SILC dataset included item missing data and they were defined as separate categories for the variables education (16%) and occupation (21%). Besides these variables, there is missing data in log hourly wage (46%). Therefore, we carried out an imputation method whilst accounting for the adjustment weights to ensure that the imputation was applied on representative adjusted data. For this purpose we ran the MICE procedure with predicted mean matching (Van Buuren & Groothuis-Oudshoorn, Citation2011) in R (built-in function: mice.impute.pmm) to impute the item missing data in log hourly wage, education and occupation. The other variables in the imputation model with no missing data were sex, age group and urbanicity (Large cities, Small cities, Rural areas). To account for the correction of the selection bias we also included the adjustment weight as a covariate in the model. We denote this approach by Weight/PMM.
We also carried out a different approach assuming that the data custodian would be undertaking a single imputation approach prior to the release of the data as is the norm at statistical agencies and then calculating the adjustment weights on the imputed dataset. Therefore, we first imputed the WI dataset using a single iteration of predictive mean matching to obtain a complete dataset. We then calculated the adjustment weights with no missing data categories. We denote this approach by PMM/Weight.
A simulation study and an expanded version of the manuscript is detailed in Huang et al. (Citation2021) in the project deliverable in Lenau et al. (Citation2021). The simulation study showed that both approaches provide similar point estimates of correlations and regression coefficients however the PMM/Weight approach had less variation compared to the Weight/PMM approach as would be expected from the single imputation.
4. Descriptive statistics for original and adjusted datasets
In this section, we show the performance of the three different approaches to improve the reliability of the 2016 Netherlands WI dataset as described in Section 3 (sample matching, Weight-PMM, PMM-Weight) by comparing adjusted statistics with statistics obtained from the probability-based 2016 Netherlands EU-SILC reference dataset. show the results of descriptive statistics comparing the original and adjusted 2016 Netherlands WI dataset with the 2016 Netherlands EU-SILC data. Note that the weight variable used for sample matching is the cross-sectional survey weights provided by EU-SILC.
The key point from is that the application of weighting adjustments presents a significant improvement on the sample characteristics in the adjusted WI sample data as compared to the original WI sample data. Weighted variables in the two datasets are shown to have distribution patterns that are more comparable to the original EU-SILC sample in column 1 of . Such effect is particularly discernible in imputed variables including education and occupation, as well as on non-imputed variables including age and sex. Consequently, it is evident that the combination of PMM and weighting adjustment may improve both the representativeness and the completeness of the original WI data.
To further examine the impact of statistical adjustments on the 2016 Netherlands WI data, we present a linear regression model in where log hourly wage is the response variable for the original 2016 Netherlands WI dataset, as well as for the Weight-PMM, PMM-Weight, and sample matched datasets. This analysis demonstrates the impact of the adjustment methods on a multivariate analysis compared to the original WI dataset. The control variables in the models are age groups, gender, employment status, education, occupation, and urbanicity. The missing categories of the variables are excluded in this analysis for the original 2016 WI dataset in this regression model.
Table 2. Regression results for log hourly wage comparing Weight-PMM, PMM-Weight and sample matching approaches.
From , looking first at the results for the dataset using sample matching, we find that some variables present opposite effects as compared to the propensity-adjusted approaches and the original complete case WI dataset. This is particularly discernible in the case of employment and education. There is also a relatively larger difference in the effect sizes of professionals (in reference to managers). Overall, the PMM-Weight and Weight-PMM results are similar in terms of the patterns of the effect sizes of variables with respect to their signs and significance levels in comparison to the original complete case WI dataset and therefore appear more reliable compared to the sample matching approach.
5. Application measuring the gender pay gap
In this section, we demonstrate the improved reliability of the adjusted online nonprobability WI dataset for the substantive problem of measuring the Gender Pay Gap (GPG). The advantage of using the WI data to measure the GPG is that it has the variable log hourly wage. In contrast, the EU-SILC data has only annual income from wages and therefore is dependent on confounders such as part-time work. There are many studies of GPG using WI data and we cite one study in Van der Straaten et al. (Citation2020) who employ a series of multilevel models to explore GPG in Multi-national Enterprises (MNE) using WI survey data from over 40,000 employees in 13 countries. It is worth noting that most of the GPG studies based on the WI data are often descriptive and do not conduct more advanced multivariate analyses.
To measure the GPG, it is common to use the Blinder–Oaxaca decomposition (Blinder, Citation1973; Oaxaca, Citation1973) which is available in the STATA package (Jann, Citation2008). The method explains the difference in the means of the log hourly wage by decomposing the gender gap into that part that is due to differences in the mean values of the independent variables in the model (percent explained), and group differences in the effects (parameters) of the independent variables (percent unexplained). The method calculates the size and significance of the overall pay gap between men and women, and as mentioned also divides the gap into a part that is explained by differences in determinants of wages and a part that cannot be explained by such group differences. Moreover, since our analysis include employees and self-employed as reported by the respondents to the WI web survey, the Blinder–Oaxaca decomposition model is integrated with the Heckman’s selection model (Heckman, Citation1979) to correct for self-choice in the labour market. The model deducts the selection effects from the overall differential and then applies the standard decomposition formulas to this adjusted differential. More details are provided in Jann (Citation2008). All methods in the analyses used the adjustment weights to compensate for the selection bias in the nonprobability web survey, that is, the propensity adjustment weights for the WI dataset and the EU-SILC weights for the sample matching approach. As a benchmark for the analysis, the 2014 GPG in the Netherlands was 14.6% according to Boll and Lagemann (Citation2018) and 16.1% according to Leythienne and Ronkowski (Citation2018) of which 45–55% can be explained by personal, job, and national characteristics. Both of these studies were based on the probability-based 2014 Structure of Earnings Survey. The Netherlands GPG in 2022 was published as 14.2% (European Commission, Citation2022).
shows the results of the Blinder–Oaxaca decomposition of the difference between (natural) log hourly earnings of men and women. The upper section of exhibits the overall pay gaps between men and women under the different approaches: original WI, Weight\PMM, PMM\Weight and sample matching. In addition, the overall explained part and the unexplained part are also expressed as a percentage of the difference between log hourly earnings of men and women. The subcomponents of the explained part are displayed in the lower section of . The explanatory variables included in the analysis are age, education, occupation, and urbanicity.
Table 3. Oaxaca–Blinder decomposition of GPG for original (complete case) 2016 WI data and the adjusted data: Weight-PMM, PMM-Weight and sample matching approaches.
All approaches in suggest a pay gap between men and women in favour of men. With regard to the size of the GPG (the difference between log hourly wage of men and women), the GPG detected in the original WI data and the sample matching approach appear to be smaller than those detected in Weight/PMM and PMM/Weight approaches. The GPG is 9% in the original WI dataset and even less in the sample matching of 4% (where the difference was found to be not significant). The Weight/PMM and the PMM/Weight approaches, with the use of adjustment weights and imputation as explained in Section 3, have a GPG of 18% and 16%, respectively, and highly significant, which is approximately the expected level from other published studies of the GPG in the Netherlands (Boll & Lagemann, Citation2018; Leythienne & Ronkowski, Citation2018).
In the lower section of , the explained part of the GPG can be attributed to the difference in average characteristics of age group, education, occupation, and urbanicity between men and women workers. The overall explained part is 7% in the original complete-case WI dataset. This means that only 7% of the difference between log hourly earnings of men and women can be attributed to the difference in average characteristics (i.e. age, education, occupation, and urbanicity) between male and female workers that is in favour of men. The lower section of shows that the explained part of GPG in the original complete-case WI data is mostly driven by two explanatory factors, namely, education and occupation, which attribute 33% and 62%, respectively, to the difference between log hourly wage of men and women. The explanatory power of age and urbanicity is comparatively much weaker. The pattern in the sample matching approach using the EU-SILC weights have an overall explained part of only 3% and the effects of education and occupation are even stronger (41% and 65%, respectively) with negative effects for age and urbanicity. For the Weight-PMM and PMM-Weight approaches under the propensity adjustment and post-stratification, the overall explained part is 27% and 34%, respectively. In general, for these approaches, there are less effects from education and occupation compared to the original complete-case WI and sample matching approaches, and more of an effect from age and urbanicity. In summary, the results from the Blinder–Oaxaca decomposition model under the Weight/PMM and PMM/Weight approaches are more similar to other studies on GPG in the Netherlands, although we note that the results of the decomposition of the explained gap in are dependent on the small number of explanatory variables that we have available in the WI dataset.
6. Conclusions
In spite of the convenience and low costs of collecting data via a nonprobability online web survey, we showed that the data can suffer from selection and coverage biases in comparison to a random probability sample. This was shown based on the substantive study of estimating the 2016 Gender Pay Gap (GPG) for the Netherlands according to the nonprobability WageIndicator (WI) web survey and using the EU-SILC data as the random probability-based reference sample. We showed that we can improve the reliability of the nonprobability online data collection for carrying out general inference.
More specifically, we use the probability-based reference sample to estimate propensity scores of participation in the web survey according to the method proposed by Chen et al. (Citation2020) and then benchmark the inverse propensity scores to auxiliary totals from the reference sample to produce final weight adjustments. We showed that this approach can be used to overcome potential selection and coverage biases in the nonprobability sample. We also showed that the alternative approach of sample matching did not produce credible results for this application.
In addition, we showed two approaches for carrying out imputations of item missing data in the nonprobability sample: impute after the weighting adjustments and include the weight variable as a covariate in the imputation model; and, impute missing data within the nonprobability sample to obtain a complete dataset and then carry out the weighting adjustments. The approaches provided similar results albeit there was smaller variation in the impute/weight approach as it was typically based on a single imputation.
The final important conclusion is that we have shown clear evidence that we must implement statistical methods to improve the reliability of a nonprobability web survey before carrying out statistical analyses, otherwise we can obtain biased results. From the study of GPG, we saw that the combination of imputation and weighting adjustment techniques using propensity scores to a reference sample are able to ameliorate outcomes of the Blinder–Oaxaca decomposition model in terms of the degree of similarity relative to patterns found in representative probability samples in the Netherlands.
Future work will undertake different types of substantive analyses on other nonprobability surveys adjusted through probability-based reference samples to validate the conclusion that we can improve the reliability of these surveys for statistical modelling and inference. Other methods have been proposed in Kim et al. (Citation2021) based on mass-imputation and an Empirical Likelihood approach in Chen et al. (Citation2022).
All software codes are available on request. The calculation of propensity scores according to the Chen et al. (Citation2020) approach described in Section 3.1 is now available in an R-code package in Chrostowski et al. (Citation2023). For the imputation approach in Section 3.2, we use the MICE procedure in Van Buuren and Groothuis-Oudshoorn (Citation2011). The STATA code for the application in Section 5 of the GPG using the Blinder–Oaxaca decomposition method is available in Jann (Citation2008).
Acknowledgements
The research leading to these results has received funding from the European Union’s Horizon 2020 research and innovation programme under grant agreement No. 730998 (InGRID-2 Integrating Research Infrastructure for European expertise on Inclusive Growth from data to policy). An expanded preprint of this manuscript appears in the project deliverable in Lenau, et al. (Citation2021). The results from this study were presented at the 13th Scientific Meeting of the Classification and Data Analysis Group (CLADAG2021), Firenze, September 9–11, 2021 (see https://media.fupress.com/files/pdf/24/7254/1940).
Disclosure statement
No potential conflict of interest was reported by the author(s).
Additional information
Notes on contributors
Yinxuan Huang
Yinxuan Huang completed his PhD in the Institute of Social Change and Sociology at the University of Manchester in 2016. He was awarded the Hallsworth China Political Economy Fellowship Grant in 2016–2018, and subsequently conducted research related to cross-national surveys at City, University of London and The University of Manchester. He is currently Quantitative research manager at the British and Foreign Bible Society. His main research projects include cross-national data collection, surveys on minority groups, and studies on religion, politics, and society.
Natalie Shlomo
Natalie Shlomo is Professor of Social Statistics at the University of Manchester and publishes widely in the area of survey statistics and survey methodology. She has over 75 publications and refereed book chapters and a track record of generating external funding for her research. She is an elected member of the International Statistical Institute (ISI), a fellow of the Royal Statistical Society, a fellow of the Academy of Social Sciences and President 2023–2025 of the International Association of Survey Statisticians. Homepage: https://www.research.manchester.ac.uk/portal/natalie.shlomo.html.
References
- Baker, R. J., Brick, M., Bates, N. A., Battaglia, M., Couper, M. P., Dever, J. A., Gile, K. J., & Tourangeau, R. (2013). Report of the AAPOR task force on nonprobability sampling. Retrieved Augut 05, 2021 https://www.aapor.org/AAPOR_Main/media/MainSiteFiles/NPS_TF_Report_Final_7_revised_FNL_6_22_13.pdf.
- Bethlehem, J. (2010). Selection bias in web surveys. International Statistical Review, 78(2), 161–188. https://doi.org/10.1111/j.1751-5823.2010.00112.x
- Blinder, A. S. (1973). Wage discrimination: Reduced form and structural estimates. Journal of Human Resources, 8(4), 436–455. https://doi.org/10.2307/144855
- Boll, C., & Lagemann, A. (2018). Gender pay gap in EU countries based on SES 2014. Publications Office of the European Union. Retrieved August 14, 2023, from https://commission.europa.eu/system/files/2018-10/report-gender-pay-gap-eu-countries_october2018_en_0.pdf.
- Braunsberger, K., Wybengam, H., & Gates, R. (2007). A comparison of reliability between telephone and web-based surveys. Journal of Business Research, 60(7), 758–764. https://doi.org/10.1016/j.jbusres.2007.02.015
- Chen, Y., Li, P., Rao, J. N. K., & Wu, C. (2022). Pseudo empirical likelihood inference for non-probability survey samples. Canadian Journal of Statistics, 50(4), 1166–1185. https://doi.org/10.1002/cjs.11708
- Chen, Y., Li, P., & Wu, C. (2020). Doubly robust inference with nonprobability survey sample. Journal of the American Statistical Association, 115(532), 2011–2021. https://doi.org/10.1080/01621459.2019.1677241
- Chrostowski, L., Beresewicz, M., & Chlebicki, P. (2023). nonprobsvy – an R package for modern statistical inference methods based on non-probability surveys. Retrieved April 10, 2024, from https://cran.r-project.org/web/packages/nonprobsvy/nonprobsvy.pdf
- Elliot, M. R., & Valliant, R. (2017). Inference for nonprobability samples. Statistical Sciences, 32(2), 249–264. https://doi.org/10.1214/16-STS598
- European Commission. (2022). Factsheet: Equal pay? Time to close the gap!. European Commission. Retrieved April 14, 2023, from https://commission.europa.eu/system/files/2022-11/equal_pay_day_factsheet_2022_en_1_0.pdf
- Fabo, B., & Belli, S. S. (2017). (Un)Beliveable wages? an analysis of minimum wage policies in Europe from a living wage perspective. IZA Journal of Labour Policy, 6(4), 1–11. https://doi.org/10.1186/s40173-017-0083-3
- Heckman, J. (1979). Sample selection bias as a specification error. Econometrica, 47(1), 153–161. https://doi.org/10.2307/1912352
- Huang, Y., & Shlomo, N. (2021). Improving the reliability of web survey: an empirical evaluation of weighting adjustment, imputation, and sampling matching strategies to measure gender pay gap (pp. 65-90) in Lenau, S., Marchetti, S., Münnich, R., Pratesi, M., Salvati, N., Shlomo, N., Schirripa Spagnolo, F. and Zhang, L.-C. (2021): Methods for sampling and inference with non-probability samples. Deliverable D11.8, Leuven, InGRID-2 project 730998 – H2020.https://www.inclusivegrowth.eu/files/Output/D11.8-Methods-for-sampling-and-inference-with-non-probability-samples-updated-on-website.pdf
- Jann, B. (2008). The blinder-oaxaca decomposition for linear regression models. The Stata Journal, 8(4), 453–479. https://doi.org/10.1177/1536867X0800800401
- Kim, J. K., Park, S., Chen, Y., & Wu, C. (2021). Combining non-probability and probability survey samples through mass imputation. Journal of the Royal Statistical Society: Series A, 184(3), 941–963. https://doi.org/10.1111/rssa.12696
- Kureková, L. M., Beblavý, M., & Thum-Thysen, A. (2015). Using online vacancies and web surveys to analyse the labour market: A methodological inquiry. IZA Journal of Labour Economics, 4(18), 1–20. https://doi.org/10.1186/s40172-015-0034-4
- Lee, S. (2006). Propensity score adjustment as a weighting scheme for volunteer panel web surveys. Journal of Official Statistics, 22(2), 329–349.
- Lee, S., & Valliant, R. (2009). Estimation for volunteer panel web surveys using propensity score adjustment and calibration adjustment. Sociological Methods and Research, 37(3), 319–343. https://doi.org/10.1177/0049124108329643
- Lenau, S., Marchetti, S., Münnich, R., Pratesi, M., Salvati, N., Shlomo, N., Schirripa Spagnolo, F., & Zhang, L.-C. (2021). Methods for sampling and inference with non-probability samples (deliverable D11.8). InGRID-2 project 730998 – H2020. Retrieved August 15, 2023, from https://www.inclusivegrowth.eu/files/Output/D11.8-Methods-for-sampling-and-inference-with-non-probability-samples-updated-on-website.pdf.
- Leythienne, D., & Ronkowski, P. (2018). A decomposition of the unadjusted gender pay gap using structure of earnings survey data. Publications Office of the European Union. Retrieved April 23, 2021, from https://ec.europa.eu/eurostat/documents/3888793/8979317/KS-TC-18-003-EN-N.pdf/3a6c9295-5e66-4b79-b009-ea1604770676.
- Liu, Z., & Valliant, R. (2023). Investigating an alternative for estimation from a nonprobability sample: Matching plus calibration. Journal of Official Statistics, 39(1), 45–78. https://doi.org/10.2478/jos-2023-0003
- Oaxaca, R. (1973). Male-female wage differentials in urban labour markets. International Economic Review, 14(3), 693–709. https://doi.org/10.2307/2525981
- Revilla, M., & Ochoa, C. (2015). What are the links in a web survey among response time, quality, and auto evaluation of the efforts done? Social Science Computer Review, 33(1), 97–114. https://doi.org/10.1177/0894439314531214
- Rivers, D., & Bailey, D. (2009). Inference from matched samples in the 2008 U.S. national elections. Paper presented at the 64th Annual Conference of the American Association for Public Opinion Research, Florida.
- Rosenbaum, P. R., & Rubin, D. B. (1983). The central role of the propensity score in observational studies for causal effects. Biometrika, 70(1), 41–55. https://doi.org/10.1093/biomet/70.1.41
- Rosenbaum, P. R., & Rubin, D. B. (1984). Reducing bias in observational studies using sub-classification on the propensity score. Journal of the American Statistician, 39(1), 33–38. https://doi.org/10.1080/00031305.1985.10479383
- Schleyer, T. K., & Forrest, J. L. (2000). Methods for the design and administration of web-based surveys. Journal of the American Medical Informatics Association, 7(4), 416–425. https://doi.org/10.1136/jamia.2000.0070416
- Smyk, M., Tyrowicz, J., & Van Der Velde, L. (2018). A cautionary note on the reliability of the online survey data: the case of wage indicator. Sociological Methods and Research, 50(1), 429–464. https://doi.org/10.1177/0049124118782538
- Steinmetz, S., Raess, D., Tijdens, K., & De Pedraza, P. (2013). Measuring wages worldwide: exploring the potentials and constraints of volunteer web surveys (pp. 78-97) in Sappleton, N. (2013): Advancing research methods with new technologies. IGI Global.
- Steinmetz, S., & Tijdens, K. G. (2009). Can weighting improve the representativeness of volunteer online panels? Insights from the German wage indicator data. C&M Newsletter, 5(1), 7–11.
- Steinmetz, S., Tijdens, K. G., & Pedraza, P. (2009). Comparing different weighting procedures for volunteer web surveys. Amsterdam institute for advanced labour studies. AIAS Working Paper, 09–76. Retrieved August 6, 2021, from https://wageindicator.org/documents/publicationslist/publications-2009/0900-WP76-Steinmetz-Tijdens-Pedraza.pdf
- Stolte, J. F. (1994). The context of satisficing in vignette research. The Journal of Social Psychology, 134(6), 727–733. https://doi.org/10.1080/00224545.1994.9923007
- Sue, V. M., & Ritter, L. A. (2012). Conducting online surveys (2nd ed.). Sage Publications.
- Tijdens, K. G., Besamusca, J., & van Klaveren, M. (2015). Workers and labour market outcomes of informal jobs in formal establishments. A job-based informality index for nine sub-saharan African countries. The European Journal of Development Research, 27(5), 868–886. https://doi.org/10.1057/ejdr.2014.73
- Tijdens, K. G., Van Zijl, S., Hughie-Williams, M., Van Klaveren, M., & Steinmetz, S. (2010). Codebook and explanatory note on the wageindicator dataset: A worldwide, continuous, multilingual web-survey on work and wages with paper supplements. Amsterdam institute for advanced labour studies. AIAS working paper, 10–102. Available on: Retrieved August 6, 2021, from. https://wageindicator.org/documents/publicationslist/publications-2010/codebook-and-explanatory-note-on-the-wageindicator-dataset.pdf
- Valliant, R., & Dever, J. A. (2011). Estimating propensity adjustments for volunteer web surveys. Sociological Methods and Research, 40(1), 105–137. https://doi.org/10.1177/0049124110392533
- Van Buuren, S., & Groothuis-Oudshoorn, K. (2011). Mice: Multivariate imputation by chained equations in R. Journal of Statistical Software, 45(3), 1–67. April 10, 2024, from https://cran.r-project.org/web/packages/mice/mice.pdf
- Van der Straaten, K., Pisani, N., & Kolk, A. (2020). Unraveling the MNE wage premium. Journal of International Business Studies, 51(9), 1355–1390. https://doi.org/10.1057/s41267-019-00285-x
- Varkkey, B., Korde, R., & Singh, S. (2016). Minimum wage comparison: Asian countries – minimum wage fixing. WageIndicator Foundation. Retrieved August 6, 2021, from https://wageindicator.org/documents/publicationslist/publications-2016/varkkey-b-korde-r-singh-s-2016-minimum-wage-comparison-asian-countries
- Wang, L., Valliant, R., & Li, Y. (2021). Adjusted logistic propensity weighting methods for population inference using nonprobability volunteer-based epidemiologic cohorts. Statistics in Medicine, 40(24), 5237–5250. https://doi.org/10.1002/sim.9122
- Wu, C. (2022). Statistical inference with non-probability survey samples. Survey Methodology, 48(2), 283–311.