Abstract
Concerns have been raised in numerous countries over declining rates of active transport to school. In a UK context, the pupil-school commute is estimated to contribute around 658 kilotonnes of CO2 per year; however, tackling this issue effectively requires an improved understanding of how emissions can be modelled and mapped over a variety of scales. This paper implements a new estimation technique for the modelling of CO2 emissions linked with the school commute that integrates both transport network-level routing and geographically disaggregate vehicle emissions data. The model is then applied to a national cohort of pupils in England. Areas demonstrating the highest emissions were typically more rural and/or comprising more affluent resident populations. Emissions were also shown to increase with school year, with larger step changes between educational stages reflecting the different geography of school locations. Furthermore, where secondary school entry policies were selective or based on a religious domination, average emissions were typically higher than in non-selective schools.
Keywords:
Introduction
Internationally, the rates of travelling to school by active transport (e.g. cycling or walking) are in decline (Tudor-Locke et al. Citation2001, Schlossberg et al. Citation2006, McMillan Citation2007, Trang et al. Citation2012), and the corollary switch to less sustainable modes of travel have been linked to negative effects on the environment in terms of increased emissions (Van Ristell et al. Citation2012), increasing traffic congestion around schools (Collins and Kearns Citation2001) and health impacts related to lower physical activity levels (Faulkner et al. Citation2009, Meron et al. Citation2011) or pollutant exposure (McConnell et al. Citation2010).
In a UK context, schools account for 15% of total public sector emissions (DCFS Citation2010), which in England are estimated to be the equivalent of around 9.4 million tonnes of CO2 per year (SDC Citation2006). Seven per cent (658 kilotonnes) of this total is associated with the pupil-school commute, and as such, there are significant environmental benefits for pupils to adopt more sustainable travel behaviour.
International research on commuting to school reveals that mode choice is impacted by multiple interacting factors including actual and perceived distance to the school (McDonald Citation2007, Müller et al. Citation2008, Lang et al. Citation2011), road infrastructure (Ewing et al. Citation2004, Bejleri et al. Citation2010), urban form (McMillan Citation2007, Mitra et al. Citation2010, Panter et al. Citation2010, Cui et al. Citation2011, Broberg et al. Citation2013), ethnicity (McDonald Citation2007), socio-economic status (Wilson et al. Citation2010, Roth et al. Citation2012, Su et al. Citation2013) and lifestyle factors (Babey et al. Citation2009). It has also been argued that policies enabling school choice can lead to longer commuting distances, which has a corresponding impact on mode choice and emission levels (Marshall et al. Citation2010, Wilson et al. Citation2010, Van Ristell et al. Citation2012). The use of geographic information systems (GIS) as a framework for analysis is prevalent in many studies in this area. Examples include calibrating environmental measures related to active transport (Panter et al. Citation2010, Dalton et al. Citation2011, Wong et al. Citation2011) including accessibility and walkability (Bejleri et al. Citation2010, Giles-Corti et al. Citation2011); estimating probable routes taken (Stigell and Schantz Citation2011, Larsen et al. Citation2012) or energy expended (Cooper et al. Citation2010); and finally, assessing pollutant exposure along routes (Ashmore et al. Citation2000).
The mode of transport adopted by pupils for their commute to school has obvious implications for CO2 emissions. With a few recent exceptions (Wilson et al. Citation2007, Marshall et al. Citation2010, Van Ristell et al. Citation2012), there have been limited studies to date that estimate the emissions impact of these journeys, with only Van Ristell et al. (Citation2012) examining this at a national scale for England (albeit using a sample). In two of these studies (Marshall et al. Citation2010, Van Ristell et al. Citation2012), different types of regression analysis were used to predict mode choice given a range of influencing factors (Wilson et al. Citation2010). From these models, CO2 emissions are estimated by multiplying the distance and frequency of trips between locations by the average CO2 attributed to specific modes of transport. Commuting distance is treated differently by these studies, with Van Ristell et al. (Citation2012) adopting straight-line distance, whereas Marshall et al. (Citation2010) and Wilson et al. (Citation2007) implemented network-based distances derived using shortest or actual commuting paths. The advantage of straight-line distance is that it can be computed quickly; however, this will typically underestimate the true distance travelled, given that actual routes follow street/rail/footpath topology. However, the estimation of shortest or quickest path along a network is computationally intensive, thus creating an additional challenge for studies concerning either a very large sample or total population survey. A second consideration is what CO2 (g/km) value should be attributed to different mode choices? The use of national averages is prevalent in most existing models, however, may overestimate or underestimate geographical differences in actual emission characteristics; for example, relating to issues such as the mix of cars typically owned within different types of area.
As such, there is a challenge to create transport-linked CO2 emission estimates that better account for geography; both in the estimation of the distances travelled over multiple transport networks, and where possible, through the integration of mode-choice emission input parameters that are sensitive to geographical context. This complex task is computationally intensive, however, as illustrated in this paper, can be enabled through the application of geocomputation (Openshaw and Abrahart Citation2000). A geographically sensitive model is specified that integrates network-level (road/rail) routing with geographically disaggregate vehicle emissions data to provide estimates of pupil school commute linked CO2 emissions at an individual scale, and for a national cohort of pupils. These results are compared to those achieved using a simple model that is calibrated with straight-line distance and national average CO2 estimates. The paper concludes with an exploration of the socio-spatial structure of the geographically sensitive estimates.
A geographically sensitive model of CO2 emission estimates
An estimate of CO2 emissions attributed to a pupil (p) school commute (kp) is defined in Equation (1) where d is the distance travelled between origin i (pupil domicile unit postcode) and destination j (school unit postcode) utilising transport mode t with associated CO2 (g/km) value e. Furthermore, in the case of transport by car or light rail, these average vehicle emissions can also vary by location g. This parameter is used flexibly within the model, with location being specified as either a lower super output area (LSOA: 400–1200 households) in the case of car travel, or for light rail, the nearest network to the pupil domicile. The CO2 (g/km) estimates that are used to calibrate the model are discussed later and detailed in and . A final weighting parameter w is required to adjust an estimate where average occupancy is not already accounted for in the emission value associated with the transport mode choice. In this model, it only includes those pupils whose travel mode is by car sharing, where a weighting of 0.5 is presumed (i.e. an occupancy of two).
Table 1. CO2 emissions for different transport modes (Coley Citation2002, DEFRA Citation2011, Tranter Citation2012)
Figure 1. Average CO2 values, given in g/km, for those cars registered within lower-layer super output areas.
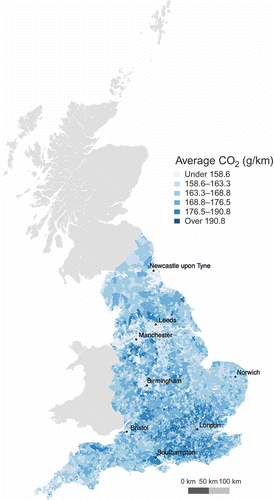
The model is empirically driven, requiring a number input of data sets. The Department for Education (DfE) supplied a national cohort data set containing individual pupil-level attributes such as age, gender, ethnicity and home postcode, with a coverage for all pupils in state-maintained schools in England, from nursery through to post-compulsory education.Footnote1 These data are collected by the DfE for policy and administrative purposes, and it is mandatory for schools to complete a return, with three separate surveys completed throughout the year, and spaced between the spring, summer and autumn terms. Data are available going back to 2002; however, between 2007 and 2012, there was also an additional requirement for schools to submit details of ‘usual’ transport mode choices adopted by pupils for their journeys to and from school. This represents the best estimate of actual mode choice, however, it should be noted that students may adopt different modes of travel at the start and end of the day (Schlossberg et al. Citation2006, Southward et al. Citation2012); however, in a UK context, travel mode to and from school has been shown to correlate strongly (van Sluijs et al. Citation2009). The DfE supplied access to the Spring 2011 data, which at the time of request was the latest available release. The total number of records in this pupil database was 7,532,205; however, from these, 158,700 were excluded as they either did not have a domicile postcode, or the postcode provided was invalid; mode choice was null, specified as a ‘border’ (attending a boarding school) or ‘other’ (an uncategorised mode choice), thus leaving a final data set of 7,373,505 observations.
The second key data set integrated into the model contains the average of CO2 (g/km) emitted by those cars that were registered to addresses within each LSOA. Averages were created for this project by the Department for Transport (DfT) as an aggregation of Driver Vehicle Licensing Agency (DVLA) data. This records an associated CO2 (g/km) emission value for those vehicles that are registered to specific addresses. These emission values are derived using a rolling road test as set out in the European legislation directive 91/441/EC and are a component of emissions approval. However, when these values are aggregated at an area level, they may underestimate true emissions averages, given that the cars registered after March 2001 are only included in these tests. For example, the average vehicle CO2 (g/km) from the DVLA data is 162.9, which differs from the Department for Environment, Food and Rural Affairs (DEFRA) national estimate of 183.2 (DEFRA Citation2011). However, without actual data on those cars driven to school, these estimates represent a plausible alternative. If the LSOA-level average car emission rates are mapped (see ), there are clear geographic variations; for example, highlighting how cars registered in the areas surrounding London and the south of Manchester have on average higher emission rates. Exploring the underlying causes of these variations lies outside the scope of this paper; however, this map does illustrate the importance of including geographically contextualised inputs into models when estimating emission levels at a disaggregate spatial scale.
The model also requires average vehicle CO2 per kilometre emission estimates for non-car transport. These are given in and are sourced from a number of publications (Coley Citation2002, DEFRA Citation2011, Tranter Citation2012). In general, these estimates are calculated as an interaction between the average emission characteristics of a type of vehicle and the typical occupancy, which is why for a single mode (e.g. light rail) there are multiple values for those schemes operating in different parts of England. The rates for vehicles were all derived from DEFRA (Citation2011) and are the official (and annually updated) figures used in government statistics concerning emissions.
An innovative feature of the model is to estimate the distances pupils travel to school along both the street and rail networks. There are numerous ways in which these network distances could be estimated, ranging from online services to various open-source or commercial software. All implementations involved the automated supply of origin destination locations and the calculation of distances over either the quickest or shortest path along the transport network. For automotive journeys, a wide variety of solutions are available to calculate routes along the road network, and those tested included the application programming interfaces (API) for Google MapsFootnote2 and Bing Maps,Footnote3 alongside the open-source routing library RoutinoFootnote4 that utilises OpenStreetMapFootnote5 data. The API for both Google Maps and Bing Maps enabled a query to be sent to their respective online servers, a route calculated and returned in a standardised format. Routino is an open-source software that can be compiled for both Linux (http://www.linux.org) or OS X (http://www.apple.com/uk/osx/) and installed locally. A query can be sent to the library using the terminal, and will return a result that calculates a route over OpenStreetMap street network data. Other options considered, but not tested, included various online routing services that also integrated with OpenStreetMap data such as OpenRouteServiceFootnote6 or YOURS.Footnote7 Given the non-commercial nature of these services, the impact on their servers and bandwidth of calculating such large volumes of routes would have been significant; and as such, these services were not tested. Both the Google and Bing APIs were subject to search limits. For Bing, this was 125,000 sessions or 500,000 transactions within a 12-month period (developers), and for Google, 2500 per 24-hour period. However, in addition to these limits, both services appeared subject to adaptive firewall technology, thus preventing multiple queries in succession. The calls made were within the bounds of the daily usage limits, however, at various random intervals, would fail to return a result. The reasons for these failures were not clear, and indeed results could be returned if the failed origin destination queries were re-run at a later stage. A further limitation to the Google service was a requirement in their terms of use that any results derived from routing queries have to be displayed on a Google map after the trip calculation. Thus, although the distance could be extracted, it was also a necessity to display the returned route on a map, which has large performance implications, considerably slowing down batch calculations given the rendering time of maps would comprise an unnecessary processing overhead. Overall, both Google and Bing services provided a reasonable solution for calculating routes, however, daily query limits, in addition to adaptive firewall issues limited their utility when calculating routes in large volumes. Furthermore, from a methodological perspective, these services are closed source, and there is little information on those algorithms utilised or metadata about the network over which the routes are calculated. An alternate solution evaluated was the open-source Routino libraries that can run under Linux or OS X. The Routino libraries (version 2.2) were complied from source under OS X Lion (http://www.apple.com/uk/osx/), and an extract of the Great Britain OpenStreetMap data was imported. The default configuration of the Routino library was implemented with the exception of enabling pedestrian access to ‘trunk roads’ (larger, often dual carriageways). This corrected problems where pedestrian access had not always been explicitly labelled in the underlying OpenStreetMap data, and because of this, the routing algorithm would occasionally calculate implausible and very long routes to avoid the ‘trunk roads’. Solutions were compared by examining a set of 500 randomly selected origin–destination pairs from the pupil database. There was a strong positive linear relationship between the distance estimates with Pearson correlation coefficients ranging between 0.98 and 0.99 (P < 0.001). As such, the routing solutions are reasonably interchangeable; however, Routino was implemented in the model as this offered the lowest computational processing time given that the calculations were completed locally, and furthermore, unlike the two online systems is open source, thus enabling greater transparency of the results.
For non-road journeys (rail, underground, light rail), appropriate online routing applications were not available, as although it is currently possible for services to supply quickest travel times, actual track distances are not provided. In the case of the Google Maps API, some railway routes have started to be added (at least visually); however, these are not uniformly available. As such, a custom solution was developed by importing rail network data into a PostgreSQLFootnote8 database with the PostGISFootnote9 (spatial enabling) and pgRoutingFootnote10 (routing enabling) extensions. Railway network and station location data were extracted from national mapping agency open data (Ordnance Survey: Meridian 2Footnote11); and for light rail and the London Tube, these were exported from OpenStreetMap. The topologies of these data were extensively checked to ensure that routes could be correctly calculated after they were imported into the database. For pupils travelling on non-road transport, a nearest neighbour calculation was used to return the closest station to both the pupil domicile, and additionally the school attended, with routes being calculated between these two station locations. Rail has reasonably universal coverage; however, for both tube and light rail, these modes of transport are only found within certain areas. As such, an error check was required to examine the plausibility of the assignment of both pupils and schools to a nearest station (see Equation (2)). For each distance (D) between a pupil (p) domicile and their nearest (x) station, a Tukey outlier filter (Tukey Citation1977) was calculated using the quartile (Q) and inter-quartile range (IQR) specific to each network (n).
An outlier check is necessary given that a light rail, tube or rail mode choice could have been specified in error, which would result in pupils being assigned to a station at an unrealistic distance from their domicile. The same calculation was implemented for schools, thus supplementing p in the above equation with s for school. Those distance scores returned as outliers were removed from the analysis, as were those pupil journeys that returned a valid result but were equidistant between two networks. For these cases, there was no way of determining which network was used, and was an issue specific to London, where two light rail networks are present within reasonably close proximity, however, are not physically connected. These procedures were not implemented for the railway network given that station coverage is national, and as such, based on a nearest neighbour assignment, it would be difficult to pick out those matches that were in error. However, the distances calculated from erroneous origin destination pairs would be detectable as outliers (i.e. travelling implausible distances), which is discussed later in the context of all transport mode choices. Out of 16,048 light rail and tube journeys, 1621 were identified as outliers (∼10%). Finally, a further error check compared the distance to the nearest station with the distance to the school attended. If the straight-line distance to the school was shorter than the distance to the station, then the mode was presumed to be in error, and as such, these pupils were also excluded from the analysis. For all non-road journeys, this removed another 1914 records.
When implementing distance calculations, either the shortest or quickest journeys were estimated. For road network calculations, these were implemented as the quickest routes, with access and speed restrictions appropriate to the mode of transport. For example, a car would not be able to access pedestrian footpaths running through a park. Although bus routes are beginning to become available for some operators in England, coverage is currently limited. Furthermore, dedicated school bus routes are not known. As such, these distances were also calculated as road optimised quickest paths. For rail-based calculations, the shortest path was calculated, optimising routes based on the track length.
After the model input data were assembled into a PostgreSQL database and routing solutions were created for both rail and road journeys, these were coupled using the statistical programming language R (http://www.r-project.org). A pseudo-code implementation of the model is detailed below:
For each pupil, identify the mode choice
Implement a rail- or road-based distance calculation appropriate to the mode
Store the distance travelled
Check that the pupil distances returned are plausible, and extract outliers or detected errors
For each valid pupil commute
Retrieve an appropriate geographically sensitive CO2 (g/km) emissions estimate depending on location and mode choice
Calculate CO2 (g/km) for each pupil trip
If necessary, weight CO2 (g/km) to account for a car share arrangement
Store emissions estimate
At stage 1(a) in the above pseudo-code, distances are returned using either road or rail network distances. All rail routes were returned, however, the Routino-based road network calculations could not return a distance for 24,319 routes despite having valid co-ordinates specified for both origins and destinations. These errors occur because the algorithm could not find a proximal node within the OpenStreetMap network data and can occur where the network coverage is lacking.
After calculating appropriate network distances, an error test was implemented to check the plausibility of the results. There are a number of reasons why errors may occur, including
that the domicile postcode is valid but specified in error
that the school postcode may be an error (although unlikely given the administrative importance of these data being correct)
that there has been a geocoding error
that the routing calculation had returned an implausible result (possible for road calculations that are dependent on underlying OpenStreetMap (OSM) data quality)
A modified Tukey outlier filter (Tukey Citation1977) was again implemented to examine each pupil commute (Kp) against the quartile (Q) and inter-quartile range (IQR) for all distances disaggregated by the combination of year group (a), local authority (g) and mode type (m). This was necessary to account for geographic differences in transport infrastructure, differences in those distances typically travelled using different types of transport, and finally, to control for how travel behaviour changes between age groups. This was implemented using Equation (3):
This analysis resulted in a final database of 6,912,226 records being returned for input into the CO2 estimation model. The distances were then multiplied by an appropriate CO2, given in g/km, for the allocated mode choice, and if necessary, weighted, as described earlier in this section, and then stored back in the database.
The validity of the model can be explored through comparison with official national estimates, which as described at the start of this paper are 658,000 tonnes of CO2 per year for the UK. If the emission value for each pupil is multiplied by 5 (days of the week) and then by 38 (weeks of schooling), these scores give a summed value for England of 618,602 tonnes CO2 per year. These are reasonably in line with UK statistics, given that they exclude Northern Ireland, Wales and Scotland; and although there are no methodological details of how the official UK estimates were calculated, these would most probably utilise Euclidian distances, which as discussed earlier, would systematically underestimate the real distances travelled. It should also be noted that the estimates calculated in this paper exclude those pupils in non-state schools and those records identified as outliers. Further validation of the model was implemented by comparing the results of the geographically sensitive model with an alternate simple model that supplemented transport network distance with straight-line distance, and swapped the location specific vehicle CO2 emission values (previously given in and ) for national averages (DEFRA Citation2011). A map illustrating the differences between the CO2 emissions estimated by the geographically sensitive and the simple models are shown in The choropleth units relate to local authority boundaries, which are an administrative geography for England. Positive scores within these zones indicate that the simple model is overestimating emissions relative to the geographically sensitive model, with negative results illustrating the inverse pattern. The top ten local authorities with the highest overestimated and underestimated values shown in . These patterns demonstrate a degree of systematic error, with the simple model more prevalently exhibiting underestimated values for urban areas and the opposite pattern observed for rural local authorities. The implication of these differences reinforces a recommendation for models to better integrate local geography, and is arguably acutely important if the results are to be presented at sub-national scales.
Table 2. The top 10 overestimation and underestimation of CO2 values between the geographically sensitive and simple model by local authority
The geography of CO2 emissions linked to the school commute
The model presented in the previous section created an estimated CO2 emission value for each valid pupil-school commute. In this following section, these estimates are explored by various factors including school characteristics and year groupings, pupil locations, and a geodemographic classification that provides a summary of multiple characteristics about the areas in which the pupils are domiciled. These descriptive analyses illustrate key characteristics of the English national geography of CO2 emissions linked to the school commute.
The national geography of CO2 emissions was explored by calculating the pupil average daily CO2 emission values within local authority administrative areas (see ).
The local authorities with the highest values attributed to the school commute were Sevenoaks with estimated average daily emissions of 1184 g of CO2; Canterbury 1115 g; Tonbridge and Malling 1110 g, East Lindsey 1086 g and South Holland 969 g. Unpicking this geography is complex given the national coverage, however, in general, those areas with higher average emissions appeared to be in lower density and more rural areas. This geography can be explored further by profiling the model results by a geodemographic classification linked to each pupil postcode. The importance of contextual effects on children’s school travel mode choice has been explored elsewhere (Sidharthan et al. Citation2011). Although some have criticised their use (Goss Citation2003), there is a diverse and international legacy of successful application of geodemographics in the exploration of urban socio-spatial structure; with utility demonstrated across a wide variety of public sector domains (Singleton and Spielman Citation2013). The ACORN geodemographic classification from CACI Ltd (http://www.caci.co.uk/) was made available for this analysis and comprised three hierarchically nested levels of 5 categories, 17 groups and 56 types. Each of the clusters within the typology is named and described to give end-users an impression of those salient characterisers of the classified areas. The average daily CO2 (g) associated with the pupil-school commutes are presented by the ACORN typology in .
Table 3. Average CO2 (g) emitted during the pupil-school commute disaggregated by the ACORN geodemographic typology
A number of interesting patterns are highlighted. Firstly, reinforcing those previous observations from , some of those geodemographic types with the highest emission values are typically found within more rural areas, for example, ‘Villages with wealthy commuters’, ‘Farming communities’ and ‘Large families and houses in rural areas’. Secondly, the most affluent types typically have higher emission values (e.g. those within the category ‘Wealthy achievers’). The inverse of these patterns emerges in those types that pertain to areas where residential populations have more constrained finances and are typically found within urban areas (e.g. within the categories ‘Moderate means’ and ‘Hard-pressed’). Drivers of these patterns will likely relate to both the characteristics of the built environment in the places where the geo-demographic clusters are typically found, and also the behavioural choices of their typical resident populations. For example, affluent populations may more prevalently choose to drive over other forms of transport; or, those cars purchased by people living within more rural areas may bias vehicles with larger engines that are more suitable to variable terrain. Exploring the underlying motivations for such choices lies outside the scope of this study; however, to illustrate the influence that location and transport mode choice has on CO2 emissions between geodemographic types, a scatter plot of commuting distance and the percentage of pupils adopting active transport is shown in
The points plotted in are ACORN types, coloured by their nested category and are scaled by the average CO2 (g) emitted, with smaller points equating to lower emissions. As might be expected, there is a strong negative linear relationship between the distance travelled to school and the adoption of active transport, with pupils resident in areas classified by those types travelling further, typically contributing more CO2 on average per commute. The disaggregation of this distribution by geo-demographic categories is quite striking, illustrating again that those types containing more affluent populations are on average contributing higher levels of CO2 emissions per daily commute, travelling further, and with lower rates of active transport.
Preferences for active transport will not be homogenous across school years given the variable geographic distribution of schools at various educational stages, and additionally, growing independence of pupils over time. presents a disaggregation by school year for the average CO2 (g) emitted during the school commute. The coloured bars represent different educational stages from nursery (years N1, N2; aged 4–5), through reception (year R; aged 5), and then into primary school (years 1–6/7; aged 6–11/12), secondary school (years 6/7–11; aged 11/12–16) and finally, post-compulsory education (years 12–13; aged 16–18). Average impact grows as school year progresses with step changes occurring as pupils transfer from nursery school to primary school, primary school to secondary school, and finally, into post-compulsory education. The step changes relate to the different distribution of schools and/or travel options; for example, primary schools are typically smaller than secondary schools and have a more defuse geographic coverage. The gradual increase in emissions between years is also interesting; more so given that this pattern follows for primary, secondary, and post-compulsory education. The distances travelled to school demonstrated the same gradual increase, which is perhaps not that unexpected given their use in the estimation of the CO2 emitted; however, these are also matched by an overall gradual decline in active transport (see ). One issue with these observations is that the patterns observed are not those of a single cohort of students over the duration of their education, rather those pupils within schools during a single academic year; and as such, it is problematic inferring too strongly about the changing behaviour of individuals over time.
Figure 5. (a) Average CO2 emissions by school year (b) percentage of pupils travelling using active transport by school year.
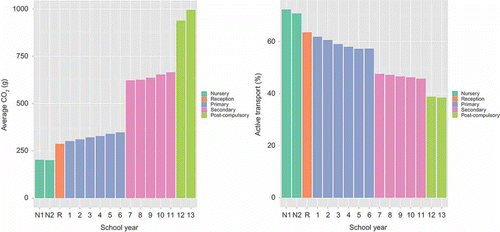
The availability and preferences for different types of school will also have an interacting effect on overall emission rates; and as such, the final attribute explored in this paper considers secondary school admissions criteria. Only secondary schools are considered in this section given that there are a greater diversity of admissions criteria, and that these will typically have greater impact on distances pupils travel to school given their more defuse geographic coverage. Within England, there are a number of different systems that govern admissions to secondary schools. These differ regionally, and broadly can be divided into selective (admissions based on a test) and comprehensive (admissions without a test). In those areas with only selective admissions, a further category exists (‘Modern’) which is applied to those schools where pupils would attend if they failed to attain a place at a selective school. Furthermore, some schools also have a requirement (variably enforced) that pupils should be from a specific religious denomination; providing a secondary and sometimes overlapping constraint on choice.
Parents choose which secondary school to send their children; however, the allocation of pupils into schools is bound by the availability of places. Higher performing schools will typically have increased demand, often leading to over subscription, where more applications are received than available places. Although various criteria are used to allocate pupils to available places in non-selective schools, a commonly used differentiating factor is straight-line distance between a pupil domicile and the school (Singleton et al. Citation2011). As such, those pupils who are more proximal to the school, on average, will share a greater chance of admission, all other criteria being equal. For selective schools, these distance criteria will not typically be applicable, and where the performance of the school is high, this may attract pupils from more disparate locations. For non-selective schools, religious denomination can often usurp distance-based entrance criteria, and again, has the effect of increasing the average distances that pupils will travel to these schools. The impact of school policy on commuting-linked emissions and the distances pupils travel to school are summarised in . This shows that pupils attending religious schools are on average travelling further to school, with lower rates of active transport and higher average CO2 in g/km travelled. A similar, but more extreme pattern is exhibited for those attending selective schools, and additionally, again, with a very low rate of active transport. These data illustrate that school admission criteria impact strongly on emissions and support previous work in this area (Van Ristell et al. Citation2012) that argue for more sustainable ‘neighbourhood schools’, where pupils are assigned to a nearest (or zoned) school, thus minimising travel and increasing the prevalence for active transport.
Table 4. Emission characteristics disaggregated by secondary school selection criteria and presence or absence of religious denomination
Conclusions
This paper has explored the geography of CO2 emissions linked with the pupil-school commute in England. A method of estimation is presented that accounts for local geography through the inclusion of transport network distances and vehicle emission characteristics that are geographically disaggregate. This inclusion represents an incremental improvement on established methods that typically utilise straight-line distances and national average vehicle emission values. A second innovative feature of the model is that the calculations have been scaled for a near-total population of school pupils, thus creating CO2 emission estimates for around 7 million individual commutes over the transport network. Previous work in this area has relied on samples (Van Ristell et al. Citation2012) or small focused case studies (Wilson et al. Citation2007, Marshall et al. Citation2010), and as such, this work represents the first time a model of such disaggregate scale and extensive coverage has been implemented in the context of pupil-school commuting. When comparing results to alternate estimates generated using straight-line distance and national average vehicle emission values, it was found that these simplified models had a tendency to underestimate emissions relative to a geographically sensitive model for a number of more urban local authorities. This likely relates to a composite of factors, however, an obvious impact would be the added complexity of the urban street network (density and directionality of traffic), which would impact road travel distances.
The results of the geographically sensitive model were mapped and then profiled by a series of categorical descriptors to elicit the types of areas in which the pupils were domiciled, alongside the characteristics of those schools attended. This analysis supported findings from the wider literature that has illustrated links between socio-economic status, the built environment and travel demand/mode choice (Ewing and Cervero Citation2001, Handy et al. Citation2005, Brownstone and Golob Citation2009). Higher average emissions per pupil were more prevalently found in lower density areas and/or with more affluent populations. The importance of geographic context has been shown elsewhere to influence pupil mode choices (Sidharthan et al. Citation2011), and as such, there may also be a degree of self-reinforcement within or between proximal zones that have populations predisposed to adopting less sustainable methods of commuting to school. From a policy perspective, this may make ‘neighbourhood’ an appropriate scale at which to encourage more sustainable behaviour.
Average emissions increased with each year of academic progression, with larger step changes occurring between the different educational stages (e.g. primary to secondary). The type of school that parents choose for their children was also shown to have an impact on emissions. Pupils attending schools where entrance was governed by selective admission policies (based on a test) tended to exhibit a higher propensity for travelling longer distances, with a correspondingly lower rate of active transport and increased average emissions. Similar patterns were also observed for those pupils attending schools where religious denomination formed a component of the entrance criteria. It is argued that this relates to an absence of entrance criteria linked to proximity measures, which is a common metric used in non-selective schools where demand outstrips supply. From a policy perspective, this supports those arguments made elsewhere in the literature (Van Ristell et al. Citation2012) that significant potential benefits for reducing CO2 emissions could be attained through policy that encourages schools with localised catchments.
The model presented in this paper has illustrated a method of improving geographical sensitivity in transport-linked emissions models; however, it has also highlighted some areas for extension. For example, actual bus routes could be integrated when national coverage of these data becomes available. This would also enable multi-mode routing to be considered, which may be especially important in areas such as London where a more integrated public transport infrastructure exists. Further model refinements could also be envisaged to better account for the physical characteristics of road routes, for example, by measuring terrain, assessing the probable number of stops at lights and accounting for other network characteristics such as speed limits. These data could be used to weight CO2 estimates, as they would have an impact on car emissions. In addition to these methodological enhancements, a number of substantive directions for future research emerged from the empirical analysis. Firstly, to explore how emission patterns changed between 2007 and 2012, corresponding to when the national mode choice data were collected; and secondly, to explore how emissions and mode choices change between educational stages through the linking of individual pupil records over time.
This paper has described and then implemented an alternate way in which CO2 emissions linked with the pupil-school commute can be estimated for a national cohort of pupils; building on previous work by encapsulating the topological structure of the transport network and geographic differences between average vehicle emission characteristics. The substantive findings are supportive of previous studies in this area, indicating that built structure, affluence and school admission policies all have impact on overall CO2 emissions.
Acknowledgements
The author thanks Dr Daryl Lloyd at the Department for Transport for supplying the CO2 estimate data and answering numerous queries about their calculation! The author also thanks the anonymous reviewers for their useful comments and suggestions. This work was funded by a 2012 Royal Geographical Society Small Grant and an Economic and Social Research Council research grant (ES/K007459/1).
Notes
1. 1. DfE school census data: http://www.education.gov.uk/rsgateway/schoolcensus.shtml
4. 4. http://www.routino.org
9. 9. http://postgis.net/
10. 10. http://pgrouting.org/
References
- Ashmore, M., et al., 2000. Effects of traffic management and transport mode on the exposure of schoolchildren to carbon monoxide. Environmental Monitoring and Assessment, 65 (1–2), 49–57.
- Babey, S.H., et al., 2009. Sociodemographic, family, and environmental factors associated with active commuting to school among US adolescents. Journal of Public Health Policy, 30, S203–S220.
- Bejleri, I., et al., 2010. Using GIS to analyze the role of barriers and facilitators to walking in children’s travel to school. Urban Design International, 16 (1), 51–62.
- Broberg, A., Salminen, S., and Kyttä, M., 2013. Physical environmental characteristics promoting independent and active transport to children’s meaningful places. Applied Geography, 38, 43–52.
- Brownstone, D. and Golob, T.F., 2009. The impact of residential density on vehicle usage and energy consumption. Journal of Urban Economics, 65 (1), 91–98.
- Coley, D.A., 2002. Emission factors for human activity. Energy Policy, 30 (1), 3–5.
- Collins, D.C. and Kearns, R.A., 2001. The safe journeys of an enterprising school: negotiating landscapes of opportunity and risk. Health & Place, 7 (4), 293–306.
- Cooper, A.R., et al., 2010. Mapping the walk to school using accelerometry combined with a global positioning system. American Journal of Preventive Medicine, 38 (2), 178–183.
- Cui, Z., Bauman, A., and Dibley, M.J., 2011. Temporal trends and correlates of passive commuting to and from school in children from 9 provinces in China. Preventive Medicine, 52 (6), 423–427.
- Dalton, M.A., et al., 2011. Built environment predictors of active travel to school among rural adolescents. American Journal of Preventive Medicine, 40 (3), 312–319.
- DCFS, 2010. Road to zero carbon. London: Department for Children, Schools and Families. No. DCSF–00111–2010
- DEFRA, 2011. 2011 guidelines to Defra/DECC’s GHG conversion factors for company reporting: methodology paper for emission Factors. London: Department for Environment, Food and Rural Affairs.
- Ewing, R. and Cervero, R., 2001. Travel and the built environment: a synthesis. Transportation Research Record, 1780 (1), 87–114.
- Ewing, R., Schroeer, W., and Greene, W., 2004. School location and student travel analysis of factors affecting mode choice. Transportation Research Record, 1895 (1), 55–63.
- Faulkner, G.E.J., et al., 2009. Active school transport, physical activity levels and body weight of children and youth: a systematic review. Preventive Medicine, 48 (1), 3–8.
- Giles-Corti, B., et al., 2011. School site and the potential to walk to school: the impact of street connectivity and traffic exposure in school neighborhoods. Health & Place, 17 (2), 545–550.
- Goss, J., 2003. The instrumental rationatity of geodemographic systems. In: D. Clarke, ed. The consumption reader. London: Routledge, 211–215.
- Handy, S., Cao, X., and Mokhtarian, P., 2005. Correlation or causality between the built environment and travel behavior? Evidence from Northern California. Transportation Research Part D: Transport and Environment, 10 (6), 427–444.
- Lang, D., Collins, D., and Kearns, R., 2011. Understanding modal choice for the trip to school. Journal of Transport Geography, 19 (4), 509–514.
- Larsen, K., Gilliland, J., and Hess, P.M., 2012. Route-based analysis to capture the environmental influences on a child’s mode of travel between home and school. Annals of the Association of American Geographers, 102 (6), 1348–1365.
- Marshall, J.D., et al., 2010. Vehicle emissions during children’s school commuting: impacts of education policy. Environmental Science & Technology, 44 (5), 1537–1543.
- McConnell, R., et al., 2010. Asthma and school commuting time. Journal of Occupational and Environmental Medicine, 52 (8), 827–828.
- McDonald, N.C., 2007. Children’s mode choice for the school trip: the role of distance and school location in walking to school. Transportation, 35 (1), 23–35.
- McMillan, T.E., 2007. The relative influence of urban form on a child’s travel mode to school. Transportation Research Part A: Policy and Practice, 41 (1), 69–79.
- Meron, D., et al., 2011. Changes in active travel of school children from 2004 to 2010 in New South Wales, Australia. Preventive Medicine, 53 (6), 408–410.
- Mitra, R., Buliung, R.N., and Roorda, M.J., 2010. Built environment and school travel mode choice in Toronto, Canada. Transportation Research Record: Journal of the Transportation Research Board, 2156 (1), 150–159.
- Müller, S., Tscharaktschiew, S., and Haase, K., 2008. Travel-to-school mode choice modelling and patterns of school choice in urban areas. Journal of Transport Geography, 16 (5), 342–357.
- Openshaw, S. and Abrahart, R.J., 2000. GeoComputation. London: Taylor & Francis.
- Panter, J.R., et al., 2010. Neighborhood, route, and school environments and children’s active commuting. American Journal of Preventive Medicine, 38 (3), 268–278.
- Roth, M.A., Millett, C.J., and Mindell, J.S., 2012. The contribution of active travel (walking and cycling) in children to overall physical activity levels: a national cross sectional study. Preventive Medicine, 54 (2), 134–139.
- Schlossberg, M., et al., 2006. School trips: effects of urban form and distance on travel mode. Journal of the American Planning Association, 72 (3), 337–346.
- SDC, 2006. School carbon footprinting. London: Sustainable Development Commission.
- Sidharthan, R., et al., 2011. Model for children’s school travel mode choice. Transportation Research Record: Journal of the Transportation Research Board, 2213, 78–86.
- Singleton, A.D., et al., 2011. Estimating secondary school catchment areas and the spatial equity of access. Computers, Environment and Urban Systems, 35 (3), 241–249.
- Singleton, A.D. and Spielman, S.E., 2013. The past, present and future of geodemographic research in the United States and United Kingdom. Professional Geographer, Forthcoming.
- Southward, E.F., et al., 2012. Contribution of the school journey to daily physical activity in children aged 11–12 years. American Journal of Preventive Medicine, 43 (2), 201–204.
- Stigell, E. and Schantz, P., 2011. Methods for determining route distances in active commuting – their validity and reproducibility. Journal of Transport Geography, 19 (4), 563–574.
- Su, J.G., et al., 2013. Factors influencing whether children walk to school. Health & Place, 22, 153–161.
- Trang, N.H.H.D., Hong, T.K., and Dibley, M.J., 2012. Active commuting to school among adolescents in Ho Chi Minh City, Vietnam. American Journal of Preventive Medicine, 42 (2), 120–128.
- Tranter, M., 2012. Light rail and tram statistics: England 2011/12. London: Department for Transport.
- Tudor-Locke, C., Ainsworth, B.E., and Popkin, B.M., 2001. Active commuting to school: an overlooked source of childrens physical activity?. Sports Medicine, 31 (5), 309–313.
- Tukey, J.W., 1977. Exploratory data analysis. Reading, MA: Addison-Wesley.
- Van Ristell, J., et al., 2012. Quantifying the transport-related impacts of parental school choice in England. Transportation, 40 (1), 69–90.
- Van Sluijs, E.M.F., et al., 2009. The contribution of active travel to children’s physical activity levels: cross-sectional results from the ALSPAC study. Preventive Medicine, 48 (6), 519–524.
- Wilson, E.J., et al., 2010. By foot, bus or car: children’s school travel and school choice policy. Environment and Planning A, 42 (9), 2168–2185.
- Wilson, E.J., Wilson, R., and Krizek, K.J., 2007. The implications of school choice on travel behavior and environmental emissions. Transportation Research Part D: Transport and Environment, 12 (7), 506–518.
- Wong, B.Y.-M., Faulkner, G., and Buliung, R., 2011. GIS measured environmental correlates of active school transport: a systematic review of 14 studies. International Journal of Behavioral Nutrition and Physical Activity, 8 (1), 39.