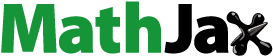
ABSTRACT
For some time now, the research focusing on Knowledge Intensive Business Services (KIBS) has been very active. Observing that knowledge as a production factor is only becoming more and more pronounced, this focus is well-grounded. It is therefore important to examine how these knowledge-hubs gain and propagate their knowledge. We hypothesize that KIBS (as many other sectors) benefit from intra-industry knowledge spillovers facilitated by geographical concentration. Our focus is the innovative capacity of KIBS, which we measure through trademarks registered by KIBS firms. While there may be several mechanisms facilitating knowledge spillovers, we can identify local intra-sectoral labor mobility as one. Accessibility measures are used to assess the geographical attenuation of the spillover effects. Results show that the distance decay of spillovers is fast. Only local concentrations of KIBS seem to be of importance. Over longer distances, we instead observe negative consequences for trademarking, indicating possible spatial competition effects.
1. Introduction
In this study we explore how the agglomeration of KIBS firms in Swedish municipalities is related to its innovative capacity. The aim is to contribute to the extensive but still growing literature on the ‘black box’ of agglomeration economies, often referred to as knowledge spillovers. While there are many ways as to how knowledge may spread over geographical space, we focus on and model empirically the importance of intra-sectoral local labor mobility between KIBS firms as one potential mechanism. The idea is that individuals who change employment bring with them the knowledge they have accumulated in their previous KIBS workplaces, which may then be diffused across the workers in the new job.
To our knowledge, there are only a handful of studies that focus on the importance of intra-industry spillovers for innovation in this sector (Shearmur and Doloreux Citation2012). Yet, these few studies do not elucidate the potential mechanisms at work. Moreover, we measure the innovation performance of KIBS using trademarking activity. Trademarking as a proxy for innovation is not yet widely in use in the literature. We argue that it is appropriate to use for the KIBS sector due to its characteristics.
Observing the spatial concentration of chairmakers in Buckinghamshire, Marshall (Citation1890) was among the first to argue about the potential importance of intra-industry knowledge spillovers for the concentrated sector. While Marshall (Citation1890) was exclusively focusing on manufacturing industries, Krugman (Citation1991) argues that most agglomerations are rather seen in the service sector. Along these lines, a dynamic subsector of services, namely knowledge intensive business services (KIBS), are found to be highly geographically concentrated (Muller and Doloreux Citation2009). KIBS include consultancy firms that offer advanced and knowledge-intense services, R&D firms and IT firms and are often viewed as the ‘high-tech’ branch of the service sector (Nählinder Citation2010). Since their main task is to provide other organizations with knowledge, they are highly dependent on face-to-face contacts with their clients, and therefore concentrated in the larger and denser areas. With knowledge as the most important factor of production, KIBS has been among the fastest growing sectors in the past decades (Miles Citation2005). As economies are shifting toward the ‘knowledge economy’, their importance is becoming more recognized. It is therefore important to study how these knowledge agents themselves gain their knowledge, which at a later stage benefits the economy at large.
Even if agglomeration economies attenuate sharply over space (Rosenthal and Strange Citation2003), the sensitivity to distance depends on the specific sector and mechanism under study. Thus, interactions among KIBS firms which might be beneficial for innovation, are still possible across municipalities. To take this into account in our analysis, we use accessibility measures. These are based on distance-decay functions that integrate and allow for potential knowledge spillovers that may take place across municipality borders and possibly longer distances. These measures can be separated into local, regional and extra-regional accessibility, to enable the capturing of potential interactions between locations that are geographically far away or relatively close to each other (Johansson, Klaesson, and Olsson Citation2002).
In addition to Marshall’s perspective, there are two other sources of knowledge spillovers controlled for in the analysis. Jacobs (Citation1969) argues that inter-industry knowledge spillovers are the main vehicle of growth. Porter (Citation1990) considers the importance of concentration of similar actors, but also claims that it is the intensity of local competition which creates higher incentives for innovation. As firms want to create a competitive advantage on the market, they are more inclined to innovate. In our analysis, we also control for regional-specific variables such as the employment rate, the education level, and regional size.
To test how the agglomeration of KIBS relates to the innovative performance of the sector, we use yearly data between 2002 and 2010 on Sweden’s 290 municipalities. Empirical results from Tobit estimations are in line with the underlying economic theory. They show that local proximity matters and KIBS benefit from co-location in terms of innovation output as measured by trademarks. Regional accessibility displays a statistically insignificant relation and extra-regional accessibility a negative one. This may be an indicator of the fact that KIBS are dependent on face-to-face contacts which are distance sensitive and the negative sign of extra-regional accessibility can be interpreted as a spatial competition effect. Building on these results, we test whether intra-sectoral (between KIBS firms) and intra-municipal labor mobility function as a mechanism for the importance of proximity between KIBS firms. Results show that this is indeed the case and that higher levels of labor mobility are indeed connected to higher innovation capacity. In addition, being in a denser region in terms of population (and economic activity in general), a region with high employment rate, and high levels of human capital also increases the innovation output for KIBS. Competition externalities show an insignificant relationship, while diversity a negative one, which we interpret as the result of correlations between the independent variables. In the present study these factors are treated as controls and our main result is that the spatial concentration and resulting intra-sectoral knowledge spillovers foster innovation as proxied by the volume of trademarks.
The rest of the paper is organized as follows. Section 2 introduces the concept of economies of agglomeration and knowledge spillovers. Then we introduce inter-firm and intra-industry labor mobility as a vehicle for knowledge spillovers. Section 3 elaborates on the KIBS sector and introduces the use of trademarks as a proxy for innovation. Section 4 introduces a conceptual model laying the groundwork for the empirical model and estimations. Section 5 presents the data used and the specification of the empirical model. In section 6 results are presented along with an analysis and interpretation. Section 7 concludes.
2. Agglomeration and knowledge spillover
In this section we start by discussing the importance of agglomeration economies for knowledge spillovers in general. We then move on to the location decisions of KIBS firms, why we find them agglomerated in larger markets as well as why we believe they benefit from intra-sectoral knowledge spillovers. In section 2.2 we discuss the importance of labor mobility as a channel for the diffusion of knowledge.
2.1. Agglomeration economies
As repeated in many places the geographical distribution of economic activities is clustered (agglomerated). This is a clear sign that the location of one individual plant is influenced by the location of others. Despite living in a globalized economy, with declining costs of transport, communication, and information exchange, the importance of agglomeration forces that rely on geographical proximity keep increasing (Groot, Poot, and Smit Citation2009; Torre and Rallet Citation2005). What are then the benefits that influence location choices and shape location patterns? New economic geography (NEG) theoretically underpin several reasons as to why local agglomeration occurs, including reduced transportation costs, specialization and diversification economies, knowledge spillovers and innovation externalities (Fujita, Krugman, and Venables Citation1999). In the past years, these questions have received an increased interest from regional scientists and economists (Glaeser et al. Citation1992; Rosenthal and Strange Citation2003, Citation2004). Even though research efforts have only picked up momentum rather recently, the theories behind the phenomena have always been part of the foundations to economics and economic geography.
The ideas about the sources and effects of geographic concentration go back already to Marshall (Citation1890), who argued that the localization of industries creates a more efficient labor market and reduces transportation costs. He then proposed three broad origins of agglomeration economies: (i) input sharing, (ii) labor market pooling, and (iii) knowledge spillovers, known to the literature as the Marshallian externalities.Footnote1 Of interest to us, are the importance of knowledge spillovers which refers to the flows of knowledge between workers located in some proximity to each other. The idea is that as local concentrations of firms belonging to similar industries facilitate the flow of knowledge across these firms, which later on lead to more innovation and higher growth rates. Glaeser et al. (Citation1992) were the first to combine Marshall’s ideas with Arrow (Citation1962) and Romer (Citation1986) to create what is known in the literature as the Marshall-Arrow-Romer (MAR) externalities. Arrow put forward the importance of learning by doing, while Romer introduced the endogenous growth theory which considers human capital and innovation as crucial for economic growth. MAR externalities imply that concentrations of similar industries in a region enable more knowledge spillovers since the transmission of knowledge becomes easier. Geographical proximity does not only facilitate face-to-face contacts and non-market interactions (Glaeser and Scheinkman Citation2000), but also increases the inter-firm mobility rates of skilled labor (Fallick, Fleischman, and Rebitzer Citation2006).
Besides the MAR externalities, there are two more theories regarding the sources of knowledge spillovers, diversity and competition. Jacobs (Citation1969) reasons that the most important mechanism for economic growth are inter-industry (across industries) knowledge spillovers rather than intra-industry ones. A variety of industries trigger innovation and economic growth and a more diverse economy give rise to so-called Jacobs’ externalities. Porter (Citation1990) supports the importance of intra-industrial concentration, but states however that it is competition that gives the most incentives to exchange knowledge and innovate. Empirical research is still not undivided on whether it is concentration, diversity, or competition which matters most for growth and innovation (Groot, Poot, and Smit Citation2009, Citation2016; Beaudry and Schiffauerova Citation2009).
Earlier literature supports the idea that geographical proximity facilitates interactions, knowledge exchange and face-to-face contacts, thus leading to higher productivity and innovation capacity (Jaffe Citation1989; Audretsch and Feldman Citation1996). However, the sheer existence of geographical proximity does not by itself generate cooperation or even knowledge spillovers. For knowledge spillovers to take place in an effective way, there is a high need of the cognitive proximity; i.e. similarity in how firms and individuals understand and interpret information. Actors need cognitive proximity to be able to communicate and understand each other effectively; i.e. they need to share a common vocabulary and framework (Boschma Citation2005).Footnote2
While Marshallian externalities were initially based on manufacturing industries, there is no reason for them to not be applied on the service sectors, especially for KIBS where no significant decline of its concentration has been observed in the last years with the development of the information and communication technologies (Chadwick, Glasson, and Smith Citation2008). Since KIBS primarily provide services to other client firms, the need for proximity to suppliers and clients creates high demand for interaction and face-to-face contacts. This is a key determinant for KIBS locational decisions (Isaksen Citation2004). Also, KIBS firms need access to high-quality communication infrastructure as well as access to national and international markets (Chadwick, Glasson, and Smith Citation2008; Simmie and Strambach Citation2006). These requirements are sensitive to distance. For instance, Antonietti and Cainelli (Citation2008) emphasize the role of agglomeration externalities for the decisions of firms to outsource KIBS. Consequently, it is not surprising to observer high degrees of agglomeration of KIBS in large markets (Andersson and Hellerstedt Citation2009). The relative absence of this sector in smaller regions can either be explained by the fact that the types of firms and sectors that are located there do not have enough resources exert a demand for KIBS services, or because the types of KIBS services that they do demand can be delivered over longer distances (Doloreux, Freel, and Shearmur Citation2010).
Literature therefore argues that KIBS are highly dependent on the location of their clients, and thus found in larger markets. What we then argue is that a by-product of these location decisions is the co-location of KIBS firms together. We assume that KIBS should also benefit from intra-industry spillovers facilitated by geographical concentration and not only from contacts with their clients. While there can be several channels through which this knowledge ‘…in the air’ diffuses across the firms, we discuss and test the importance of intra-sectoral (within KIBS) flows of labor which are facilitated by co-location.
2.2. Labor mobility and knowledge spillovers
Several researchers have recognized the role of inter-firm mobility as a key channel through which knowledge spreads across firms, industries, and space (Saxenian Citation1994; Almeida and Kogut Citation1999). This idea has become more pronounced in a knowledge-intensive economy where workers cannot leave everything behind as they change employment. They can bring with them the experience and knowledge that they have accumulated in their previous workplace (Eriksson and Lindgren Citation2008). Along these lines, the mobility of skilled labor is often treated as a source of innovation capability (Hoisl Citation2007; Storz, Riboldazzi, and John Citation2015).Footnote3 However, for learning to happen, some level of cognitive proximity among different actors is required (Nooteboom Citation2000). Thus, Boschma, Eriksson, and Lindgren (Citation2009) argue that for labor mobility to be a channel of knowledge spillovers, cognitive proximity among workers is required. Thus, they discuss the importance of the relatedness of skills between employees. Hence, we argue that since KIBS employees have high cognitive proximity to each other, due to their related industry experience in the sector, the significance of intra-sectoral mobility of labor across KIBS firms for innovation should be high.
Since it is costly to move, inter-firm labor mobility has a rather local dimension where most job switches happen within the region. Andersson and Thulin (Citation2013) find for instance that employment density spurs inter-firm mobility, and this relation is even stronger for non-routine professions (Larsson Citation2016). Therefore, as tacit knowledge follows the individuals and their mobility patterns (Gertler Citation2003), it is not surprising that literature has treated knowledge spillovers to be highly localized and attenuate sharply with distance (Andersson, Klaesson, and Larsson Citation2016).
3. Knowledge intensive business services and their role in the economy
In this section we start with discuss the importance of KIBS firms for the economy as well as their innovation capabilities. We then move on to a discussion to why trademarks would fit as a measure of innovation for such firms.
KIBS consist of firms whose business it is to offer expert services to other firms, as well as to private and public organizations (Miles Citation2005). A similar but more detailed definition is provided by Miles et al. (Citation1995) who argue that all KIBS firms fulfill the following three conditions: (i) KIBS’ typical employees are educated engineers, scientists or other experts, (ii) KIBS offer knowledge intensive input to other organizations, and (iii) KIBS’ clients are other businesses in both private and public sectors. Even if there still is no standardized definition,Footnote4 consensus is often found when identifying industries that belong to KIBS. Most papers use the following classification: ‘computer and related activities’ (72), ‘research and development’ (73) and ‘other business activities’ (74) in the NACE industrial classification of year 2002 (Miles Citation2005; Muller and Doloreux Citation2009; Gotsch and Hipp Citation2011). Strambach (Citation2008) classifies KIBS instead into economic services, marketing/advertising, technical/IT, and R&D services. Following this definition, Jacobs et al. (Citation2016) include ‘economic services’ (64) in their definition but ignores the last group in the previous classification. Andersson and Hellerstedt (Citation2009) test their results on different definitions of KIBS and show that no matter the classification, their conclusions stay the same. Thus, while we recognize the need for a more standardized definition, we have no reason to believe that these variations in industrial codes will change empirical results in any significant way given the research question at hand.
In the literature, firms within the KIBS sector are regarded as serving a special function that is central to the functioning and productivity of other firms. These service firms have come to the forefront when discussing the so-called knowledge economy. KIBS firms maintain and create a local knowledge infrastructure that plays an important role in regional development and the functioning of local innovation systems. In their important function, they have the power to impact the overall competitiveness and productivity of firms in the area. A high number of KIBS workplaces in a municipality make that area more attractive for other firms (Isaksen Citation2004; Nählinder Citation2010). Hence, the municipality may undergo a self-reinforcing cumulative process by attracting other firms to locate there and enhancing its growth even more (Myrdal Citation1957). Therefore, a large regional KIBS presence does not only promote regional economic growth (Moyart Citation2005), but also higher productivity growth (Zhang Citation2016).
Corrocher and Cusmano (Citation2014) argue that KIBS are a key feature in regional innovation capacity, and regions which show lower innovation rates are characterized by a lower number of KIBS firms. Economies benefit from KIBS innovation through the following two sources. First, KIBS help other firms to be/become innovative. E.g. this includes assisting in their clients’ organizational restructuring or helping them develop and use new technologies (Doloreux, Freel, and Shearmur Citation2010). Muller and Zenker (Citation2001) find for instance that small manufacturing firms that collaborate with KIBS were more innovative than their competitors that did not. Similarly, 76 percent of all enterprises that collaborated with KIBS were innovative. Second, besides acting as enablers, KIBS also innovate themselves (Miles Citation2000). These activities can be labeled as scientific, technological organizational and commercial (Doloreux and Shearmur Citation2012). For instance, in Sweden, 81% of all KIBS firms have presented at least one type of innovation to the market during a two-year period (Nählinder Citation2005). According to Tether and Hipp (Citation2000), innovation in KIBS is shaped by a number of features that are different compared to the manufacturing sector: they rely more on human capital; production and consumption are a simultaneous process; their products are intangible; and they are strongly related to customers. The fact that production and consumption happen simultaneously makes it difficult to differentiate between product and process innovation within KIBS. Schricke, Zenker, and Stahlecker (Citation2012) argue that innovation in KIBS can come in terms of new products and technologies such as the customization of different software, in terms of new processes such as new methods of delivering the services or in terms of new organizational types of marketing processes.
3.1. Trademarks as a proxy of innovation for KIBS
Many KIBS firms experience difficulties when they need to protect knowledge and innovations from being imitated as their innovations cannot be protected by patents since not many of them include a technical breakthrough (Gallouj and Weinstein Citation1997). However, all service innovations can in fact be protected by trademarks (Gotsch and Hipp Citation2011). A trademark is a sign (or design or an expression) protected by intellectual property rights that make goods or services of one enterprise distinguishable from others (World Intellectual Property Organization Citation2015). Trademarks are low-cost, requires little processing time and do not require a technical breakthrough to be granted (Mendonça, Pereira, and Godinho Citation2004). One of the first papers that empirically tests whether trademarks may function as an appropriate measure of innovation is one of Schmoch (Citation2003) in Germany. He argues that trademarks satisfy all the preconditions needed to be sound innovation indicators: high correlation with innovation, high number of applications, data availability, disaggregation into sectors and time and comparison between countries. From this we conclude that trademarks are reasonably good indicators of innovation, especially in the KIBS sector. Mendonça, Pereira, and Godinho (Citation2004) argue that although novelty is not a requirement when attaining a trademark, it is just a minor percentage of the applications that do not entail some originality. From an economic point of view firms do not have incentives to pay fees to register and renew something that does not have any distinguishable advantages from other products or services in the market. The authors test this claim empirically in Portugal by analyzing ‘Community Innovation Survey’ (CIS) data and a survey conducted by themselves with a representative number of firms, and results show that trademarks do indeed act as a partial output indicator of innovations. Gotsch and Hipp (Citation2011) use two independent studies to explore the use and relevance of trademarks for services in Germany by using data from the German CIS and a survey with 278 participating firms. They conclude that trademarks are significantly connected to innovation and they seem to be a good indicator of innovation. Flikkema, De Man, and Castaldi (Citation2014) study the relation of newly registered trademarks in Belgium, Netherlands, and Luxemburg by analyzing 660 new Benelux trademarks filed by SMEs. Even if the authors are aware of the relatively small sample that does not cover all firms, they find that 60% of the trademarks refer to innovative activity.
As for the case of studies covering Sweden, the only study that analyses trademark applications as an innovation indicator is Malmberg (Citation2005). He compares the number of trademarks and the number of new products in firms. He finds that the relationship between trademark registration and innovation depends on the sector, unfortunately he does not cover KIBS in his discussion. Results from CIS 3, shown in , also support the use of trademarks as measures of innovation since most (KIBS) that use trademarks are innovative ones.
Table 1. CIS 3 results regarding the use of trademarks from enterprises in Sweden.
The Community Innovation Survey of 2006 also shows that innovative KIBS firms apply for more trademarks compared to the non-innovative ones (Schricke, Zenker, and Stahlecker Citation2012). The information above reassures us about the value of the use of trademarks as innovation indicators. Even if trademarks might not be a perfect measure of innovation, there are both theoretical and empirical justifications to why we are confident that they make a suitable indicator of innovation for the KIBS sector.
4. Regional interdependencies influencing innovation by KIBS firms
In the present section we will outline a simple but useful model than serve the purpose of summarizing the most important features determining KIBS trademarking output in a region.
Referring to below we start with the fundamental properties of a region. Size determines many of the regional features. A larger region in terms of its economy allows for more agglomeration benefits to manifest themselves. Also, in a larger region, markets have the potential to work in a better and more efficient way. Part of this comes from the fact that size allows for the existence of more specialized producers and consumers. This means that both supply and demand will be more diversified. A large enough total demand can accommodate the demand for specialized (niche) products and services, indicating that suppliers of these products and services will be able to sell a large enough amount to cover fixed costs and at least break even. All production entails some amount of fixed costs and hence need a certain volume of sales. This means that the economy of a larger region will be more diversified.
A large and economically diverse regional economy is able to host a larger and higher skilled workforce. Together with sectoral diversity there will also be more diversity in the labor market in terms of demand for many different skills and occupations. Also, because a larger region hosting a bigger labor market with more, and more diverse, demand and supply of labor will work better (superior matching) employment rates will be higher. A higher employment rate, in turn, attracts labor looking for jobs to the region, increasing the size of the region. The number of KIBS firms will of course be higher in a large region compared to a smaller one. Further, it is likely that a home market effect is present so that the number of KIBS firms increases faster than regional size itself as we move up the size hierarchy of regions.
More skilled workers (in all sectors, but also KIBS) will support more labor mobility, as high skilled workers are more mobile compared to other workers. Also, a high employment rate will support labor mobility. The reason is that workers may be more willing to try new jobs if the employment rate is relatively high. It means that the worker takes a lower risk since if the new match turns out to be unsatisfactory the ability to get yet another job is high.
In turn, labor mobility function as a channel of information and knowledge between firms. So, we expect a higher labor mobility will result in more knowledge spillovers between KIBS firms. In a next step, we conclude that more knowledge may lead to more innovation of different sorts. Some of this innovation output will translate into trademarks. Following the above reasoning, we have established a logical chain of interrelated phenomena that together influence trademarking activity by firms in the KIBS sector.
To the outline of interrelations sketched out we will add two more channels depicted in . If the number of KIBS firms increases with larger regions there is a possibility that this increase is steeper than actual employment within the KIBS sector. This may be the case if there are more possibilities for specialization and exploitation of niche markets in a larger region. In any case, it holds true that the more firms that are present in the region the higher will be the competition in the sector. More firms mean more (potential) competitors. In a highly competitive environment, firms will benefit from being able to convince customers that they are unique in some respect. One way to achieve this is through trademarking. A trademark signals that a firm is unique and not easily exchangeable for some other firm. In the economics literature this kind of market situation is called monopolistic competition, where firms have some market power enabling them to sell their product at a mark-up over marginal cost. For our purposes we concentrate on the facts that we have established a link between the number of firms and trademarking activity.
Yet another important factor may influence trademarking activity. There may be some competition across regional borders. Even though KIBS services are generally very distance sensitive it is not in all cases necessary that markets or competition stop at regional borders. Especially, this may be the situation if firms (either KIBS firms or customers firms or both) are located close to those borders. In this case, there may be some regional level competition, especially this may be detrimental for a smaller region neighboring a larger one.
Based on the conceptual model presented here which is built upon the theories and literature discussed above we construct our empirical model, which is found in the next section.
5. Data, variables, and empirical model
In this section we present data sources variables used and our empirical setup.
5.1. Data and variables
To test the influence of agglomeration on KIBS trademarking, we use yearly data from 2002 to 2010 for Sweden’s 290 municipalities, maintained by Statistics Sweden and the Swedish Trademark Database. Changes in the classification of economic activities in the European community, NACE Rev. 2 industry codes, make it complicated (or impossible) to include more years in the panel not only because the codes SNI02 and SNI07 do not match anymore, but also because they have merged and split several industries.Footnote5 Consequently, our study period ends in 2010.
Trademarks are categorized in classes, according to the NICE classification system that distinguishes between 34 classes of manufactured goods and 11 classes of services. Unfortunately, they are not comparable with NACE industry codes. Hence, we made our own interpretation regarding which of these classes were the closest to the definition of KIBS. These codes are presented in .
Table 2. Detailed description of the trademarks’ classification.
Accessibility is used as a measure of geographical proximity to a mass of KIBS firms and employment. One way to understand accessibility is as the ‘potential of opportunities for interaction’. High accessibility can be understood as a situation where a high potential for interaction and spatial externalities are at hand. It is a measure for reaching an opportunity and it decays with travel time distance. To take into account potential dependencies between different locations, accessibility is classified into three ‘zones’: within the municipality (local), between municipalities belonging to one local labor market region, sometimes called a commuting region (regional) and the rest of the municipalities in all the other regions (extra-regional) in the country (Johansson, Klaesson, and Olsson Citation2002; Johansson and Klaesson Citation2007, Citation2011). Andersson and Klaesson (Citation2009) calculate the three accessibility measures as follows:
Where ,
, and
respectively show local, regional and extra-regional accessibility to KIBS workplaces for municipality r. R denotes the set of municipalities in a functional economic labor market region and W represents the set of all municipalities in the country.
represents the time-distance in minutes between two different municipalities, r and k. λ’s are parameters of time sensitivity. They determine the effect on the accessibility measure of changed or different time-distances.
An important thing we must consider is that we are interested in investigating the relationship between the clustering of KIBS firms and innovative output as measured by KIBS firms trademarking activity. To do that we need to isolate that effect from the one of regional size. The problem encountered in the data is the very high correlations between population density and the accessibility measures. This is just a reflection of the fact that KIBS firms are generally found in larger (and denser) municipalities. This is, of course, expected given the discussion above concerning the characteristics of KIBS firms. As regional size is a very important control variable and cannot be excluded from the regression, the solution that we decided to implement was to follow the approach of Elton and Gruber (Citation1991). They argue that the linear relationship between two variables can be removed through orthogonalizing them with respect to each other.Footnote6 For this paper, orthogonalization is achieved by running a separate pooled OLS regression between each accessibility measure on regional density. What is then introduced in the empirical model, are not the actual measures of accessibility themselves, but the residuals from each of the regressions. Hence, it can be argued that the relation of accessibility on innovation in the empirical model, is the relation over and above region size.
The variable of competition in municipality r, sector k, time t is based on the measure presented in Glaeser et al. (Citation1992).
The numerator is the number of KIBS establishments in a municipality divided by the amount of KIBS employment in the municipality. The denominator of Equationequation 4(4)
(4) denotes the same relation but in Sweden rather than in the municipality. A large number of firms in the municipality relative to the number of workers, compared to Sweden as a whole, signifies a highly competitive situation.
There are several mechanisms that might enable the spillover of knowledge between KIBS firms. The one we examine is the importance of labor mobility within the KIBS sector inside the municipality in a year. Inter-firm mobility is facilitated by the density of similar workplaces, something also shown in the positive correlation between the local accessibility variable and intra-sectoral intra-municipal labor mobility rates. We measure labor mobility between KIBS firms within a municipality using the following formula:
Switchestr is defined as the sum of all labor flows between KIBS firms k in a municipality r during time period t. This is normalized using the size of total KIBS employment in the municipality. Empktr measures employment in KIBS firms k in a municipality r in time period t. In the estimations below, we allow for a one-year lag in the mobility rates to account for the time lag between the timing of movement and its effect. presents the full list of variables used in the empirical estimations.
Table 3. Variables included in the analysis.
5.2. Empirical model
We examine how the agglomeration of KIBS firms relate to the innovation output of KIBS firms. The idea behind what is driving this innovation output is that a relatively high accessibility to other similar establishments renders the interactions in terms of different (knowledge) spillovers within the sector easier and with less frictions. The flow of ideas should facilitate the creation of new knowledge and innovation, which we proxy using trademark applications. At the same time, the opposite may also be true. Locating close to each other does not necessarily imply that collaboration or knowledge spillovers take place and if there is ‘too much’ proximity, negative effects can arise (Boschma Citation2005). E.g. if many KIBS firms are concentrated in a region, each of them has a small market share. This might depress their incentives to innovate, as the profits they would get from innovation are lower than those that would have obtained if there were fewer firms in the market. Hence, they might innovate less.
When testing the relationship between agglomeration and innovation in KIBS, it is important to consider the nature of the dependent variable we use. Since it is in a count form, and following a Poisson distribution, models such as negative binomial regressions are suggested. The negative binomial model is derived from a Poisson-gamma mixture distribution and is estimated using maximum likelihood methods (Hilbe Citation2011). However, approximately 31 percent of the trademark data consists of zeros. This fact creates some problems when using count-data models. A solution is to utilize a Tobit model (Autant-Bernard and James Citation2011). For panel data, a fixed effects Tobit model is not recommended due to the inconsistency of parameters. Thus, following Czarnitzki and Toole (Citation2011), we present both results from a pooled Tobit model and the random effects (RE) Tobit model. We cannot say whether the RE model is superior to the pooled one since the random effects model requires strict exogeneity in terms of the error term being uncorrelated with covariates over the time period. The model we use for the estimation is displayed in Equationequation (6)(6)
(6) . Municipalities are denoted by r and time by t.
gives the descriptive statistics of the variables used for analyzing the innovation effects of agglomeration on KIBS firms. Large differences between the values of the mean and the median of the accessibility variables as well as the density variable, mean that the distributions are skewed. To remedy that they are log-transformed. After the transformation, their mean and median are closer to each other, indicating more normal distributions.
Table 4. Descriptive statistics (all the variables in their level form).
in the appendix presents the bivariate correlations between the variables in the form used in the empirical model. The low correlation values imply no serious multicollinearity. We have also tested for it in the estimations using variance inflation factors (VIFs). Note that the accessibility variables presented are orthogonalized with density, consequently the 0 values. Two regressors that show high correlations are the local accessibility to KIBS workplaces and the measure of diversity. This result is not surprising and theoretically supported. Higher diversity levels usually go together with a larger market. Both variables measure very important regional characteristics for KIBS firms’ locational decisions. Likewise, if more KIBS firms locate in a municipality, they contribute to making it more attractive. Hence, several other firms from a diverse set of sectors will be drawn to the area and thereby boosting diversity.
6. Estimation results, empirical findings and analysis
The following section presents the results from running our model using different techniques. In we show the marginal effects of our Tobit estimations. As discussed above, we show random effect specifications along with a pooled version of the Tobit model. In the appendix, shows the same model but estimated using random effects and pooled negative binomial regressions. These are provided for the sake of checking the robustness and stability of our results.
Table 5. Tobit regression results. Relating trademarking activity by KIBS firms to explanatory variables.
In we enter the variables sequentially over four steps. The aim is to detect (in)stability of the estimates when including different subsets of the full specification. The order of inclusion is guided by the theoretical considerations introduced earlier in the paper. Also, we are guided by the bivariate correlations of all variables that are presented in the appendix.
We start with the inclusion of the accessibility variables only. This is to establish the influence of the spatial distribution of the KIBS sector itself within the local municipality, the functional region and places outside the functional region. The local presence and accessibility of KIBS is clearly positive and significant across all specifications. Result give that KIBS firms located outside the municipality but within the region do not influence trademarking. Interestingly KIBS firms located outside the region exert a negative influence pointing at some kind of competition effect. This lends support to the Marshallian view on agglomeration; if similar workplaces are located close to each other, knowledge spillovers boost their innovative power, through the knowledge that is ‘…in the air’. This implies that knowledge spillovers in the KIBS sector are highly localized where limited spillovers occur across municipality borders.
Next, we add five more variables characterizing the municipality. These are measures of competition within the KIBS sector, diversity in terms of the number of different types of economic activities that are present, education level, employment rate, and population density. Results for the competition measure say that it does not appear to be important if the local KIBS sector is made up of relatively many firms for innovative activity in terms of trademarking. Diversity is insignificant in the RE specification but statistically significant in the pooled one. While it might seem surprising, we believe this is due to the high correlation between diversity and the local accessibility variable.Footnote7 The education level is robustly related to trademarking activity. The same is true for the employment level. These both measures can be said to indicate the ‘prosperity’ and economic well-being of the municipality.
Next, we introduce our main variable of interest by itself. This is labor mobility which here is working as a proxy for ‘potential’ knowledge spillovers. The variable is positively related to trademarking. It is significant at the five percent level in the RE Tobit estimations but at the one percent level in the pooled version. This result is in line with previous research commented on earlier in this paper. The idea is that individuals who have been previously employed in KIBS industries can use this sector-specific knowledge they have accumulated into the new firm they move on to. As their experience is combined with the one of the existing employees in the firm, new knowledge is created and perhaps transformed into an innovation.
Lastly, we include all variables and estimate the full model. Going directly to the variable labor mobility we conclude that results are pretty much the same, so the inclusion of all the other (control) variables does not interfere much with the outcome. The is one major change that transpires though. In the pooled model the size of the effects drops considerably while the significance level stays unchanged. As for the other variables, they are very stable in the full model compared to the models with less included variables. The only exception to the rule is the diversity variable that turns significant and negative. This is an unexpected result that we interpret to be the result of correlations between the independent variables.
Thus, we argue that while KIBS’ main preference is to locate close to their customers, a by-effect of that is the co-location among each other. The proximity of these KIBS firms may make the spillover of knowledge through non-market interactions and face-to-face contacts easier, but we are unable to test that hypothesis empirically. What we can identify and argue for is the importance of local accessibility to KIBS firms for facilitating intra-sectoral mobility of labor. Labor mobility larger in denser areas. Thus, if local accessibility to KIBS would be low, the odds of job switches within the region would not be as extensive. Therefore, the flow of knowledge would not be that fast or frictionless.
6.1. Robustness checks
To further test the stability and robustness of our results, we split the municipalities into two groups. Based on the labor mobility rates we classify the municipalities as those having rates above the mean (high mobility) and below the mean (low mobility). Similarly, we also split them into two groups based on density; those with high-density and low density. RE and pooled Tobit estimations for these results are presented in :
Table 6. Tobit regression results. Robustness checks. high/low mobility; high/low density.
The local accessibility measure is consistently statistically significant in all the estimations. This is not surprising per sig since the variable is the effect of accessibility in the main empirical results should not be influenced by regional size due to the orthogonalization of the variables. What this robustness also shows is that there are always positive spillovers ‘in the air’, no matter how big KIBS is in the region. When the splits are made, regional accessibility displays negative and significant results in two cases in the pooled Tobit estimations (high mobility and low density), compared to no statistical significance throughout specifications in . Even here, the results can be explained through spatial competition arguments. Extra-regional accessibility is also only significant in two of the specifications but keeps its negative sign.
However, what is interesting is that local labor mobility within KIBS is only significant in high-mobility and high-density municipalities. Statistical significance is not found for regions which show lower rates of mobility and are smaller in size. What this may indicate is that labor mobility does matter for the trademarking activity of KIBS. Yet, for the relation to gain significance, a relatively high churn across employees needs to happen in the municipality. Lower rates of mobility in the region do not create enough spillovers for the creation of new ideas which would increase the level of KIBS trademarking in the region.
Regarding the other control variables, the results shown in are in general consistent with the main Tobit specifications () and the negative binomial ones () indicating robustness of the results. Thus, it can be concluded that the main conclusions of this paper are stable to alternative specifications. Intra-sectoral knowledge spillovers are important for the innovation performance of KIBS and labor mobility is one of the potentially many mechanisms allowing for the diffusion of this knowledge. However, the mobility of labor needs to reach some level of churn to show significant results.
7. Concluding remarks
The key role of knowledge intensive business services (KIBS) in economic growth has in the past years received a lot of attention in the literature, due to the increasing importance of knowledge as a production input. KIBS include consultancies, R&D firms, and IT firms, and their main function is to provide their clients with knowledge. Thus, they are dependent on face-to-face interactions with their clients, and they are often found to be highly concentrated in larger markets. At the same time, KIBS show high rates of innovation themselves.
What we argue in this paper, is that while the main preference of KIBS firms is to locate close to their customers, a side-effect is co-location among each other. Thus, we explore how the agglomeration of KIBS firms in Swedish municipalities is related to its innovative capacity. The importance of intra-sectoral spillovers conceptually dates to Marshall (Citation1890) who noted their significance in the growth of agglomerated sectors. Moreover, we use accessibility measures to allow for potential knowledge spillovers that may take place across municipality borders and possibly longer distances. To measure innovation, we use trademarks applications by the KIBS firms in a municipality.
While there are many mechanisms in play through which knowledge can spread across KIBS firms, we test for the importance local intra-sectoral mobility of labor, which is facilitated by geographical concentration. Many KIBS firms in the municipality facilitate job switching. As skilled individuals switch employment between KIBS they bring their skills with them, which is later on diffused to other workers in the firms and new knowledge is created.
Using yearly data between 2002 and 2010, Tobit estimations show that local accessibility to KIBS matters for the innovative performance of the sector, arguing for the importance of intra-sectoral spillovers. However, regional accessibility shows a statistically insignificant relation and extra-regional accessibility a negative one. We argue that this might be a spatial competition effect. Following these results, we test if local intra-sectoral mobility is a mechanism which allows for the flow of knowledge. Results confirm this hypothesis, but when robustness checks were provided labor mobility shows a significant relation after it reaches a certain threshold. Too low local labor mobility is not linked to higher innovation. Moreover, being in a denser region, a region with a high employment rate, and high levels of human capital also increases the innovation output for KIBS.
Disclosure statement
No potential conflict of interest was reported by the authors.
Notes
1 Duranton and Puga (Citation2004) reclassify Marshall’s sources of agglomeration and distinguishing between three types of micro-foundations: sharing, matching and learning.
2 Boschma (Citation2005) does also argue that too much proximity is harmful for innovation performance due to the lock-in effect. Hence, there should exist a balance between local and non-local relations.
3 Yet, there are also arguments discussing that extensive labor mobility can be negative since firms might not find it worthy to invest in the training and education of new workers (Combes and Duranton Citation2006).
4 See for example Hertog (Citation2000), Bettencourt et al. (Citation2002) etc.
5 Swedish SNI codes follow NACE codes. SNI02 covers our study period 2002–2010.
6 The same approach has also been applied by, among others, Öner (Citation2017).
7 When we run the same estimates without the local accessibility variable, diversity shows a positive and significant sign, meaning that the high correlation between those two variables creates problems for the true relation between diversity and innovation. Results for those specifications are available upon request.
References
- Almeida, P., and B. Kogut. 1999. “Localization of Knowledge and the Mobility of Engineers in Regional Networks.” Management Science 45 (7): 905–917. doi:10.1287/mnsc.45.7.905.
- Andersson, M., and J. Klaesson. 2009. “Regional Interaction and Economic Diversity: Exploring the Role of Geographically Overlapping Markets for a Municipality’s Diversity in Retail and Durables.” In Innovation, Agglomeration and Regional Competition, edited by C. Karlsson, B. Johansson, and R. Stough, 19–37. Cheltenham: Edward Elgar.
- Andersson, M., J. Klaesson, and J. P. Larsson. 2016. “How Local are Spatial Density Externalities? Neighbourhood Effects in Agglomeration Economies.” Regional Studies 50 (6): 1082–1095. doi:10.1080/00343404.2014.968119.
- Andersson, M., and K. Hellerstedt. 2009. “Location Attributes and Start‐Ups in Knowledge‐Intensive Business Services.” Industry and Innovation 16 (1): 103–121.
- Andersson, M., and P. Thulin. 2013. “Does Spatial Employment Density Spur Inter-Firm Job Switching?” The Annals of Regional Science 51 (1): 245–272. doi:10.1007/s00168-012-0544-y.
- Antonietti, R., and G. Cainelli. 2008. “Spatial Agglomeration, Technology and Outsourcing of Knowledge-Intensive Business Services: Empirical Insights from Italy.” International Journal of Services Technology and Management 10 (2–4): 273–298. doi:10.1504/IJSTM.2008.022123.
- Arrow, K. J. 1962. “The Economic Implications of Learning by Doing.” The Review of Economic Studies 29 (3): 155–173. doi:10.2307/2295952.
- Audretsch, D. B., and M. P. Feldman. 1996. “R&D Spillovers and the Geography of Innovation and Production.” The American Economic Review 86 (3): 630–640.
- Autant-Bernard, C., and L. James. 2011. “Quantifying Knowledge Spillovers Using Spatial Econometric Models.” Journal of Regional Science 51 (3): 471–496. doi:10.1111/j.1467-9787.2010.00705.x.
- Beaudry, C., and A. Schiffauerova. 2009. “Who’s Right, Marshall or Jacobs? the Localization versus Urbanization Debate.” Research Policy 38 (2): 318–337. doi:10.1016/j.respol.2008.11.010.
- Bettencourt, L. A., A. L. Ostrom, S. W. Brown, and R. I. Roundtree. 2002. “Client Co-Production in Knowledge-Intensive Business Services.” California Management Review 44 (4): 100–128. doi:10.2307/41166145.
- Boschma, R. 2005. “Proximity and Innovation: A Critical Assessment.” Regional Studies 39 (1): 61–74. doi:10.1080/0034340052000320887.
- Boschma, R., R. Eriksson, and U. Lindgren. 2009. “How Does Labour Mobility Affect the Performance of Plants? the Importance of Relatedness and Geographical Proximity.” Journal of Economic Geography 9 (2): 169–190. doi:10.1093/jeg/lbn041.
- Chadwick, A., J. Glasson, and H. L. Smith. 2008. “Employment Growth in Knowledge-Intensive Business Services in Great Britain during the 1990s – Variations at the Regional and Sub-Regional Level.” Local Economy 23 (1): 6–18. doi:10.1080/02690940801917384.
- Combes, P.-P., and G. Duranton. 2006. “Labour Pooling, Labour Poaching, and Spatial Clustering.” Regional Science and Urban Economics 36 (1): 1–28. doi:10.1016/j.regsciurbeco.2005.06.003.
- Corrocher, N., and L. Cusmano. 2014. “The ‘KIBS Engine’ of Regional Innovation Systems: Empirical Evidence from European Regions.” Regional Studies 48 (7): 1212–1226. doi:10.1080/00343404.2012.731045.
- Czarnitzki, D., and A. A. Toole. 2011. “Patent Protection, Market Uncertainty, and R&D Investment.” The Review of Economics and Statistics 93 (1): 147–159. doi:10.1162/REST_a_00069.
- Doloreux, D., M. S. Freel, and R. G. Shearmur. 2010. “Introduction.” In Knowledge-Intensive Business Services: Geography and Innovation, edited by D. Doloreux, M. S. Freel, and R. G. Shearmur, 1–19, Farnham, UK: Ashgate Publishing.
- Doloreux, D., and R. Shearmur. 2012. “Collaboration, Information and the Geography of Innovation in Knowledge Intensive Business Services.” Journal of Economic Geography 12 (1): 79–105. doi:10.1093/jeg/lbr003.
- Duranton, G., and D. Puga. 2004. “Micro-foundations of Urban Agglomeration Economies.” In Handbook of Regional and Urban Economics, edited by V. Henderson and F. Thisse (eds.), Vol. 4, 2063–2117. North-Holland, Amsterdam.
- Elton, E. J., and M. J. Gruber. 1991. “Differential Information and Timing Ability.” Journal of Banking & Finance 15 (1): 117–131. doi:10.1016/0378-4266(91)90041-J.
- Eriksson, R., and U. Lindgren. 2008. “Localized Mobility Clusters: Impacts of Labour Market Externalities on Firm Performance.” Journal of Economic Geography 9 (1): 33–53. doi:10.1093/jeg/lbn025.
- Fallick, B., C. A. Fleischman, and J. B. Rebitzer. 2006. “Job-Hopping in Silicon Valley: Some Evidence Concerning the Microfoundations of a High-Technology Cluster.” Review of Economics and Statistics 88 (3): 472–481. doi:10.1162/rest.88.3.472.
- Flikkema, M., A.-P. De Man, and C. Castaldi. 2014. “Are Trademark Counts a Valid Indicator of Innovation? Results of an In-Depth Study of New Benelux Trademarks Filed by SMEs.” Industry and Innovation 21 (4): 310–331. doi:10.1080/13662716.2014.934547.
- Fujita, M., P. Krugman, and A. Venables. 1999. The Spatial Economy: Cities, Regions, and International Trade. Cambridge: Mit Press.
- Gallouj, F., and O. Weinstein. 1997. “Innovation in Services.” Research Policy 26 (4–5): 537–556. doi:10.1016/S0048-7333(97)00030-9.
- Gertler, M. S. 2003. “Tacit Knowledge and the Economic Geography of Context, or the Undefinable Tacitness of Being (There).” Journal of Economic Geography 3 (1): 75–99. doi:10.1093/jeg/3.1.75.
- Glaeser, E. L., H. D. Kallal, J. A. Scheinkman, and A. Shleifer. 1992. “Growth in Cities.” Journal of Political Economy 1126–1152. doi:10.1086/261856.
- Glaeser, E. L. and J. Scheinkman. 2000. Non-Market Interactions. National Bureau of Economic Research.
- Gotsch, M., and C. Hipp. 2011. “Measurement of Innovation Activities in the Knowledge-Intensive Services Industry: A Trademark Approach.” The Service Industries Journal 32 (13): 2167–2184. doi:10.1080/02642069.2011.574275.
- Groot, H. L. F., J. Poot, and M. J. Smit. 2009. “Agglomeration Externalities, Innovation and Regional Growth: Theoretical Perspectives and Meta-Analysis.” In Handbook of Regional Growth and Development Theories, edited by R. Capello and P. Nijkamp, 256–281, Cheltenham, UK & Northampton, MA, USA: Edward Elgar Publishing.
- Groot, H. L. F., J. Poot, and M. J. Smit. 2016. “Which Agglomeration Externalities Matter Most and Why?” Journal of Economic Surveys 30 (4): 756–782. doi:10.1111/joes.12112.
- Hertog, P. D. 2000. “Knowledge-Intensive Business Services as Co-Producers of Innovation.” International Journal of Innovation Management 04 (04): 491–528. doi:10.1142/S136391960000024X.
- Hilbe, J. 2011. Negative Binomial Regression. New York, NY: Cambridge University Press.
- Hoisl, K. 2007. “Tracing Mobile inventors—The Causality between Inventor Mobility and Inventor Productivity.” Research Policy 36 (5): 619–636. doi:10.1016/j.respol.2007.01.009.
- Isaksen, A. 2004. “Knowledge-Based Clusters and Urban Location: The Clustering of Software Consultancy in Oslo.” Urban Studies 41 (5–6): 1157–1174. doi:10.1080/00420980410001675797.
- Jacobs, J. 1969. The Economy of Cities. New York: Random House.
- Jacobs, W., T. van Rietbergen, O. Atzema, L. van Grunsven, and V. D. Frank. 2016. “The Impact of Multinational Enterprises (Mnes) on Knowledge-Intensive Business Services (KIBS) Start-Ups: Empirical Evidence from the Dutch Randstad.” Regional Studies 50 (4): 728–743. doi:10.1080/00343404.2014.932905.
- Jaffe, A. B. 1989. “Real Effects of Academic Research.” The American Economic Review 79 (5): 957–970.
- Johansson, B., and J. Klaesson. 2007. “Infrastructure, Labour Market Accessibility and Economic Development.” In The Management and Measurement of Infrastructure, Performance, Efficiency and Innovation, edited by C. Karlsson, B. Johannsson, and K. Kobayashi, 69-98. Edward Elgar.
- Johansson, B., and J. Klaesson. 2011. “Agglomeration Dynamics of Business Services.” The Annals of Regional Science 47 (2): 373–391. doi:10.1007/s00168-010-0377-5.
- Johansson, B., J. Klaesson, and M. Olsson. 2002. “Time Distances and Labor Market Integration.” Papers in Regional Science 81 (3): 305–327. doi:10.1007/s101100200000.
- Krugman, P. 1991. Geography and Trade. Cambridge, MA: MIT Press.
- Larsson, J. P. 2016. “Non-Routine Activities and the Within-City Geography of Jobs.” Urban Studies 54 (8): 1808–1833. doi:10.1177/0042098016643266.
- Malmberg, C. 2005. “Trademarks Statistics as Innovation indicator?-A Micro Study.” Working Paper, Centre for Innovation, Research and Competence in the Learning Economy (CIRCLE) (2005/17).
- Marshall, A. 1890. Principles of Economics. Vol. 1. London: MacMillan.
- Mendonça, S., T. S. Pereira, and M. M. Godinho. 2004. “Trademarks as an Indicator of Innovation and Industrial Change.” Research Policy 33 (9): 1385–1404. doi:10.1016/j.respol.2004.09.005.
- Miles, I. 2000. “Services Innovation: Coming of Age in the Knowledge-Based Economy.” International Journal of Innovation Management 04 (04): 371–389.
- Miles, I. 2005. “Knowledge Intensive Business Services: Prospects and Policies.” Foresight 7 (6): 39–63. doi:10.1108/14636680510630939.
- Miles, I., N. Kastrinos, K. Flanagan, R. Bilderbeek, P. Den Hertog, W. Huntink, and M. Bouman. 1995. Knowledge-Intensive Business Services. EIMS Publication, 15.
- Moyart, L. 2005. “The Role of Producer Services in Regional Development: What Opportunities for Medium-Sized Cities in Belgium?” The Service Industries Journal 25 (2): 213–228. doi:10.1080/0264206042000305420.
- Muller, E., and A. Zenker. 2001. “Business Services as Actors of Knowledge Transformation: The Role of KIBS in Regional and National Innovation Systems.” Research Policy 30 (9): 1501–1516. doi:10.1016/S0048-7333(01)00164-0.
- Muller, E., and D. Doloreux. 2009. “What We Should Know about Knowledge-Intensive Business Services.” Technology in Society 31 (1): 64–72. doi:10.1016/j.techsoc.2008.10.001.
- Myrdal, G. 1957. Economic Theory and Under-Developed Regions. London: Duckworth.
- Nählinder, J. 2005. “Innovation and employment in services: the case of knowledge intensive business services in Sweden.” Dissertation Thesis, Linköping University.
- Nählinder, J. 2010. “Knowledge Intensive Business Services as Gazelles: Implication of Size on Innovation.” In Entrepreneurship and Regional Development: Local Processes and Global Patterns, edited by C. Karlsson, B. Johansson, and R. R. Stough, 172–195. Cheltenham, UK: Edward Elgar.
- Nooteboom, B. 2000. Learning and Innovation in Organizations and Economies. Oxford: Oxford University Press.
- Öner, Ö. 2017. “Retail City: The Relationship between Place Attractiveness and Accessibility to Shops.” Spatial Economic Analysis 12 (1): 72–91. doi:10.1080/17421772.2017.1265663.
- Porter, M. E. 1990. The Competitive Advantage of Nations. New York: Free Press.
- Romer, P. M. 1986. “Increasing Returns and Long-Run Growth.” Journal of Political Economy 94 (5): 1002–1037. doi:10.1086/261420.
- Rosenthal, S. S., and W. C. Strange. 2004. “Evidence on the Nature and Sources of Agglomeration Economies.” In Handbook of Regional and Urban Economics, edited by J. Vernon Henderson and T. Jacques-François, 2119–2171. North-Holland, Amsterdam: Elsevier.
- Rosenthal, S. S., and W. C. Strange. 2003. “Geography, Industrial Organization, and Agglomeration.” Review of Economics and Statistics 85 (2): 377–393. doi:10.1162/003465303765299882.
- Saxenian, A. 1994. Regional Advantage. Cambridge, MA: Harvard University Press.
- Schmoch, U. 2003. “Service Marks as Novel Innovation Indicator.” Research Evaluation 12 (2): 149–156. doi:10.3152/147154403781776708.
- Schricke, E., A. Zenker, and T. Stahlecker. 2012. Knowledge-Intensive (Business) Services in Europe Belgium: European Commission.
- Shearmur, R., and D. Doloreux. 2012. “Is There a Connection between Geographic Clustering and KIBS Innovation?” In Exploring Knowledge-Intensive Business Services: Knowledge Management Strategies, edited by E. D. Maria, R. Grandinetti, and B. D. Bernardo, 193–213. London: Palgrave Macmillan UK.
- Simmie, J., and S. Strambach. 2006. “The Contribution of KIBS to Innovation in Cities: An Evolutionary and Institutional Perspective.” Journal of Knowledge Management 10 (5): 26–40. doi:10.1108/13673270610691152.
- Storz, C., F. Riboldazzi, and M. John. 2015. “Mobility and Innovation: A Cross-Country Comparison in the Video Games Industry.” Research Policy 44 (1): 121–137. doi:10.1016/j.respol.2014.07.015.
- Strambach, S. 2008. “Knowledge-Intensive Business Services (KIBS) as Drivers of Multilevel Knowledge Dynamics.” International Journal of Services Technology and Management 10 (2–4): 152–174. doi:10.1504/IJSTM.2008.022117.
- Tether, B. S., and C. Hipp. 2000. “Competition and Innovation Amongst Knowledge Intensive and Other Service Firms : Evidence from Germany.” In Knowledge and Innovation in the New Service Economy, edited by B. Andersen, 49-67. Cheltenham, Northampton: Edward Elgar.
- Torre, A., and A. Rallet. 2005. “Proximity and Localization.” Regional Studies 39 (1): 47–59. doi:10.1080/0034340052000320842.
- World Intellectual Property Organization. 2015. “What is a trademark.” Accessed November 2015. http://www.wipo.int/trademarks/en/
- Zhang, C. 2016. “Agglomeration of Knowledge Intensive Business Services and Urban Productivity.” Papers in Regional Science 95 (4): 801–818. doi:10.1111/pirs.v95.4.
Appendix
Table A1. Bivariate correlation between all variables.
Table A2. Neg. Bin. Regression results. Relating trademarking activity by KIBS firms to explanatory variables.