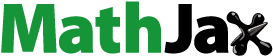
ABSTRACT
Regional clusters have become an inseparable component of modern economies. Spurred by the idea that clusters unrestrictedly encourage firm innovativeness, the cluster approach has particularly gained attention among politicians. Nevertheless, due to a lack of holistic consideration of different influencing variables, the scientific results about the effect of clusters on firm innovative performance are highly contradictive. Consequently, this paper aims to empirically investigate the conditions through which companies can gain from being located in clusters, focussing thereby particularly on moderating variables that relate to possible knowledge spillovers. Therefore, three different levels of analysis are considered separately and interactively. By analysing a unique multilevel dataset of 11.889 companies in Germany evidence is found that being located in a cluster has a positive impact on firm innovativeness. However, the results also indicate that firms benefit unequally within the cluster environment, depending on the specific firm, cluster and market/industry conditions.
1. Introduction
The geographical concentration of economic activities forms an elementary component of today´s economic reality (Brown et al. Citation2007; Nathan and Overman Citation2013). As such, regional clusters have gained considerable attention among scientists as well as policy makers (Martin and Sunley Citation2003; Sedita, Lazzeretti, and Caloffi Citation2012). Inspired by early theoretical contributions about clusters and lighthouse case studies, such as Silicon Valley, the idea that clusters promote the innovativeness and productivity has settled in the heads, particularly of policy makers. By pursuing the goal to write a similar success story for their region, policy makers at all levels of governance have implemented measures to create or support clusters (Festing, Royer, and Steffen Citation2012; Martin, Mayer, and Mayneris Citation2011; Terstriep and Lüthje Citation2018). Most European countries have therefore already realised national and regional cluster programs (European Commission Citation2016; Zenker et al. Citation2019).Footnote1
Despite the already substantial financial support of cluster activities, an invariably positive effect of clusters on firm performance is still not conclusively proven. Instead, the scientific results about the firm-specific cluster effect are indeed highly contradictive (Grashof and Fornahl Citation2021; Malmberg and Maskell Citation2002; Martin and Sunley Citation2003). Some studies have found empirical evidence for a positive performance effect (Baptista and Swann Citation1998; Bell Citation2005), while others have also emphasised rather mixed (Knoben et al. Citation2015) or even negative performance effects (Pouder and St. John Citation1996). Apart from the lack of standardised methodologies and cluster definitions, this inconsistency can mainly be explained by the missing systematic consideration of potential moderating variables. Among scientists in this field, it is actually quite prevalent to assume that all companies profit equally and in the same manner from being located in a cluster (Frenken, Cefis, and Stam Citation2013; Šarić Citation2012; Tallman et al. Citation2004). Even Michael Porter assumes that ‘a vibrant cluster can help any company in any industry compete in the most sophisticated ways, using the most advanced, relevant skills and technologies.’ (Porter Citation1998, p. 86). Nevertheless, by just having a short and superficial look at the broad field of studies dealing with firm performance differentials it becomes obvious that this idea is rather questionable, because moderating variables, such as firms internal and external resources, are indeed relevant drivers of firm performance in general (Dyer and Singh Citation1998; Van Oort et al. Citation2012; Vega-Jurado et al. Citation2008). In the cluster context, recently a comprehensive meta-analysis of the cluster literature also identified several moderating variables, such as the industry context (Grashof Citation2020a).
Frenken, Cefis, and Stam (Citation2013) therefore make a call to ‘open the black box’ (Frenken, Cefis, and Stam Citation2013, p. 23) about the conditions and mechanisms through which the firm-specific advantages of localisation economies, including for instance the benefits of specialised labour markets, specialised inputs and knowledge spillovers (e.g. Marshall Citation1920), can be realised. The aim of this paper is to respond to this call by investigating the conditions through which companies can gain from being located in clusters, focussing thereby in particular on moderating variables that relate to possible knowledge spillovers.Footnote2
While this firm-specific perspective has been widely ignored in the previous cluster research, there are some important exceptions (Lee et al., Citation2018; Šarić Citation2012; Steffen Citation2012). However, these relatively recent articles again come to contradictory empirical results (Grillitsch and Nilsson Citation2017; Hervas-Oliver et al. Citation2018). On the one hand, evidence is found that knowledge-poor firms gain the most from being located in a cluster (Rigby and Brown Citation2015; Shaver and Flyer Citation2000). But on the other hand, some studies highlight that knowledge-rich firms are the main beneficiaries (McCann and Folta Citation2011). In line with the exploratory case study of Speldekamp, Knoben, and Saka-Helmhout. (Citation2020), it is consequently reasonable to assume that additional moderating variables have to be taken into consideration in order to analyse the relationship between clusters and firm performance in a more sophisticated way.
To do so, based on a theoretical integration of the resource-based view, the relational view, the market-based view and the cluster approach, this paper investigates three different levels of analysis (micro-level, meso-level, and macro-level) by applying an OLS regression with clustered standard errors of single cross-sectional averages over time. Therefore, varying data sources are applied, ranging from firm-level to market and industry-level data. The chosen methodical approach is appropriate as it takes the hierarchical data structure, the corresponding contextuality and the year-to-year variability being ubiquitous in micro-level data into account (McNeish Citation2014; Moulton Citation1990; Rigby and Brown Citation2015). By providing an answer to the underlying research question, the paper does not only contribute to closing a still ubiquitous research gap, but also has a pragmatic meaning, because companies as well as policy makers can evaluate better under which conditions it is more likely to realise a competitive advantage in clusters or in other terms a firm-specific cluster advantage.
The remainder of this paper is structured as follows: The second section introduces the theoretical background, establishing an adequate working definition of a cluster and elaborating the corresponding hypotheses. In the third section, the applied methodical approach and data is described in detail. Thereafter, the fourth section presents the empirical results. The paper will end with concluding remarks, including limitations to this study as well as promising future research directions.
2. Theoretical foundation of the cluster and firm performance relationship
Although the term cluster is a very widespread and prevalent theme in economics, at least since the two scientific papers of Porter (Citation1990, Citation1998), there are still fundamental differences in its definition as well as understanding (Brown et al. Citation2007; Malmberg and Maskell Citation2002; Martin and Sunley Citation2003). As a consequence of the unclear definitional delimitation, the term has experienced a large proliferation and thereby has lost some of its explanatory power (Brown et al. Citation2007; Martin and Sunley Citation2003; Šarić Citation2012; Sedita, Lazzeretti, and Caloffi Citation2012). This study does, however, not intend to open a theoretical discussion about a new (conceptual) cluster definition. Instead, the following working definition of a cluster, derived through a comparative empirical approach in Grashof and Fornahl (Citation2021), is used: ‘Clusters are defined as a geographical concentration of closely interconnected horizontal, vertical and lateral actors, such as universities, from the same industry that are related to each other in terms of a common resource and knowledge base, technologies and/or product-market.’ (Grashof and Fornahl Citation2021, p. 6).
Marshall (Citation1920) was actually among the first to consider the benefits that firms can gain from being located in close proximity to firms from the same industry. He presented in this context four types of localisation externalities: access to specialised labour, access to specialised inputs, access to knowledge spillovers and access to greater demand by reducing consumer search costs (Marshall Citation1920; McCann and Folta Citation2008). As already highlighted in the previous section, the focus of this paper lies particularly on moderating variables that relate to knowledge spillovers. A view held by many economists is that geographic proximity can facilitate the transfer of knowledge in general (Jaffe, Trajtenberg, and Henderson Citation1993) and the transfer of tacit knowledge specifically because it increases the likelihood of face-to-face contacts, which is an efficient medium for the transfer of such knowledge (Daft and Lengel Citation1986). Even though not in the centre of the current scientific discussion, negative knowledge spillovers may also be the result of being located in close proximity to similar firms, in the sense that knowledge leakages are more likely to happen in an environment of reinforced knowledge exchange (Grillitsch and Nilsson Citation2017; Shaver and Flyer Citation2000). Additionally, some authors suggest that a simple reliance on local face-to-face contacts and tacit knowledge makes local networks of industry especially vulnerable to lock-in situations, which in turn enforce the inertia of companies within clusters (Boschma Citation2005; Martin and Sunley Citation2003).
While the reviewed theory is in general rather uncontroversial in its tenor about the possible (dis-)advantages of being located in a cluster, the literature is nearly silent about the concrete conditions through which those outcomes can be realised (Frenken, Cefis, and Stam Citation2013; McCann and Folta Citation2011).
2.1. Firm-level conditions (Micro-level)
This silence is particularly astonishing in the light of the resource-based view (RBV), which is regarded as one of the most widely accepted theoretical perspectives in the field of strategic management (Newbert Citation2007; Steffen Citation2012). But it has also been conceptually extended towards the regional or the cluster level (Hervas-Oliver and Albors-Garrigos Citation2007; Hervas-Oliver and Albors-Garrigos Citation2009). Its focus lies on firms’ internal resource base and how firms can utilise these resourcesFootnote3 in order to gain a competitive advantage (Barney Citation1991; Newbert Citation2007; Steffen Citation2012). The underlying assumptions of the RBV refer to the resource immobility and heterogeneity between companies. Both assumptions are necessary for the existence of different resource endowments and its persistency over time (Barney Citation1991; Newbert Citation2007). Regarding knowledge spillovers it has been argued that firms own different innovation capabilities to actually profit from these externalities (Hervas-Oliver and Albors-Garrigos Citation2009; Hervas-Oliver et al. Citation2018; McCann and Folta Citation2011). Cohen and Levinthal (Citation1990) established in this context the term absorptive capacity,Footnote4 which describes not only a firm´s ability to recognise and evaluate new information from its environment, but also its ability to process it and finally integrate it into the corresponding business innovation activities (Cohen and Levinthal Citation1990). In accordance with the core idea of the RBV, empirical evidence is found that firms with higher innovation capabilities benefit more form available knowledge spillovers. By having higher innovation capabilities, companies are better capable of accessing and integrating external knowledge (Knoben et al. Citation2015; McCann and Folta Citation2011). Although these results are quite in line with the core idea of the RBV, they neglect to some extent that strong internal innovation capabilities also implicate a higher amount of unintentional knowledge spillovers to possible competitors. These outflows of knowledge are asserted to reduce continuously a firm´s relative competitive advantage over other firms (Hervas-Oliver et al. Citation2018; Knoben et al. Citation2015; Shaver and Flyer Citation2000). Thus, the following hypothesis is proposed:
Hypothesis 1a: The strength of the innovation capabilities of a firm has an inverted U-shaped effect on firm innovative performance in clusters such that the relationship is likely to be more positive for firms with moderately strong innovation capabilities.
In this context, it has additionally been emphasised that the capacity of firms to absorb and process efficiently new knowledge requires cognitive proximity. Meaning that it is essential to own a knowledge base that is close enough to the new knowledge, so that the corresponding company can actually understand and evaluate the new knowledge in a resource efficient way (Boschma Citation2005; Nooteboom Citation2000). While a certain level of cognitive proximity is required to gain from knowledge spillovers, too much cognitive proximity might be, however, detrimental to firm innovativeness. An extensive level of cognitive proximity between two actors decreases for example the potential of learning something radically new. Furthermore, it also increases the likelihood of a lock-in situation as well as the possibility of negative knowledge spillovers (Boschma Citation2005; Fornahl, Broekel, and Boschma Citation2011). A moderate level of cognitive proximity is therefore likely to be most beneficial for firms (Boschma Citation2005; Boschma and Frenken Citation2010; Nooteboom Citation2000). While the effect of cognitive proximity has been analysed extensively on the actors’ level (e.g. Broekel and Boschma Citation2012; Fornahl, Broekel, and Boschma Citation2011), the cognitive proximity between the firm´s knowledge stock and the overall stock of knowledge within the corresponding cluster has not been investigated yet. In line with the call for further investigation of this aspect by McCann and Folta (Citation2008) as well as in accordance with Nooteboom (Citation2000), the following hypothesis is proposed:
Hypothesis 1b: The cognitive proximity between the firm´s knowledge stock and the overall stock of knowledge of the corresponding cluster has an inverted U-shaped effect on firm innovative performance in clusters such that the relationship is likely to be more positive for firms with a moderate level of cognitive proximity.
In light of the increasing significance and proliferation of inter-firm alliances, an extension of the RBV called the relational view (RV) has been developed since the late 1990s (Hervas-Oliver and Albors-Garrigos Citation2009; Lavie Citation2006; Steffen Citation2012). While the RBV has only considered those resources and capabilities that are housed within the firm, the RV focuses on inter-firm relationships and routines as valuable resources, thereby indicating that firms’ critical resources may extend beyond firm boundaries. As a consequence, for the realisation of a competitive advantage, it is not sufficient to only focus on the internal resources, but additionally it is crucial to consider relational resources (Dyer and Singh Citation1998; Lavie Citation2006). This relational dimension among economic actors has also been increasingly analysed by economic geographers as well as in the context of clusters (Giuliani Citation2007; Wu et al. Citation2010). In correspondence with the relational view, it is emphasised that the extent of strategic relationships is positively associated with firm performance (Hervas-Oliver and Albors-Garrigos Citation2009). Because maintaining a relatively high share of local connections (within the cluster) can allow firms to extract more external knowledge from their environment, which in turn makes it more likely that firms can gain from possible knowledge spillovers. Apart from the local relationships, for companies it is additionally useful to have external connections with more distant partners. Thereby, companies may acquire access to an additional knowledge base, which is different from the knowledge of local partners (Knoben et al. Citation2015; McCann and Folta Citation2011; Zaheer and George Citation2004). In this context, particularly, the engagement in collaborative R&D activities has been shown to be an important driver for the innovative performance of firms, due to the direct as well as indirect knowledge exchange (Ahuja Citation2000; Aschhoff and Schmidt Citation2008). Given the relevance of R&D collaborations, the subsidisation of joint R&D projects has also become a prominent policy tool to increase the knowledge exchange promoting the exploitation of complementary knowledge sources and thereby leading to the creation of new ideas (Fornahl, Broekel, and Boschma Citation2011; Vicente Citation2017; Weber and Rohracher Citation2012). These positive influences of the cooperative element of R&D subsidies are even more pronounced for firms located in clusters (Broekel, Fornahl, and Morrison Citation2015). Thus, the following hypothesis is proposed:
Hypothesis 1c: The number of subsidized R&D collaborations of a firm has a positive effect on firm innovative performance in clusters.
Nevertheless, as described by Dyer and Singh (Citation1998) relation-specific resources and capabilities, such as the ability to identify and evaluate potential complementarities, have to be devoted to each collaborative relationship in order to maximise the relational rents.Footnote5 The availability of these resources and capabilities is, however, limited. Consequently, the higher the share of local connections, the less relation-specific resources and capabilities can be used for establishing and/or maintaining relationships with organisations outside the cluster. The absence of outside relationships can in turn lead to lock-in situations in which companies are unresponsive to external changes (Hervas-Oliver and Albors-Garrigos Citation2009; Knoben et al. Citation2015; Uzzi Citation1997). Based on these arguments, the following hypothesis is proposed:
Hypothesis 1d: The level of local (external) connectedness has an inverted U-shaped effect on firm innovative performance in clusters such that the relationship is likely to be more positive for firms with a moderate share of local (external) connections.
Besides the level of firm connectedness, it has been highlighted that there are structural differences between the cluster core and periphery (Duranton and Kerr Citation2015). Contrary to conventional thinking, the periphery is not always the same (Eder Citation2019). As for instance indicated in Grashof, Hesse, and Fornahl (Citation2019), also within regional clusters there exist a centre and a periphery. Since knowledge spillovers are spatially limited (e.g. Rosenthal and Strange Citation2003), particularly central firms located in the cluster core have access to a large amount of local knowledge, thereby fostering their overall performance (Baldwin et al. Citation2008; Henderson Citation2003). Moreover, while the relatively high competitive pressure within the cluster core leads to higher mortality rates (e.g. De Silva and McComb Citation2012), it is reasonable to assume that this pressure also motivates firms to innovate in order to stay competitive (Harrison, Kelley, and Gant Citation1996; Porter Citation1998). Thus, the following hypothesis is proposed:
Hypothesis 1e: A firm´s central position in the cluster core has a positive effect on firm innovative performance in clusters.
2.2. Cluster-level conditions (Meso-level)
In contrast to other studies, dealing with the firm-specific cluster effect, it is claimed that in addition to this micro-level perspective the specific cluster attributes can also influence firm innovative performance within clusters.Footnote6 Likewise in the case of the differences in the resource endowments on the firm-level emphasised by the RBV (e.g. Barney Citation1991), it is therefore essential to also consider the heterogeneity on the cluster-level (Hervas-Oliver and Albors-Garrigos Citation2007). In this context, one very interesting point to look at refers to the differences in the stock of knowledge across clusters (McCann and Folta Citation2008). As highlighted at the beginning of this section, knowledge spillovers are likely to happen in all clusters, however it has been emphasised that they are likely to generate larger performance effects in clusters with a relatively high knowledge stock (Beaudry and Breschi Citation2003; Knoben et al. Citation2015; McCann and Folta Citation2008). Thus, the following hypothesis is proposed:
Hypothesis 2a: The stock of knowledge of the cluster has a positive effect on firm innovative performance in clusters.
Another relevant cluster-level attribute is the stock of alliances within the cluster (McCann and Folta Citation2008). Similar to hypothesis 1 c and in line with the core idea of the RV highlighting the importance of relational resources (e.g. Dyer and Singh Citation1998), it is expected that within alliance-rich clusters, the possibility to extract knowledge from local and external connections is enhanced. In this way, it should be much easier in an alliance-rich cluster for companies to come in contact with a larger number of different partners than in an alliance-poor cluster. Thus, the following hypothesis is proposed:
Hypothesis 2b: The stock of alliances of the cluster has a positive effect on firm innovative performance in clusters.
2.3. Market-/Industry-level conditions (Macro-level)
Alongside these two different levels of analysis, the effect of the market and industry environment on firm performance has been widely acknowledged (e.g. Zahra and Bogner Citation1999). However, in the cluster context these macro-level characteristics have so far only been considered to a limited extent (Kohlbacher et al. Citation2013), although clusters are obviously characterised by market and industry-specific differences.Footnote7 One of the most prominent theoretical streams in this context refers to the market-based view (MBV) which is predominantly influenced by the earlier work of Michael Porter (Porter Citation1980; Steffen Citation2012). In line with the MBV and building on the two scientific papers of Suarez and Lanzolla (Citation2005) and Suarez and Lanzolla (Citation2007), dealing with external influences on the first-mover advantage, it is supposed that the pace of technology evolution affects firm innovative performance in clusters (Suarez and Lanzolla Citation2005, Citation2007). The pace of technology evolution can be captured through the technology S-curve which depicts the evolution of a technology along a particular performance parameter, such as the CPU clock speed in the case of the computer industry (Cooper and Schendel Citation1976; Suarez and Lanzolla Citation2007). Under a rapid or radical technology evolution, it is likely that the current knowledge stock of firms as well as clusters becomes rather unsuitable or even obsolete. In addition, such a technological evolution will increase the market risk which in turn has again a rather negative effect as it is supposed that under a high market risk companies will exchange less knowledge as well as invest less resources and capabilities in new relationships, reducing the potential benefits from being located in close proximity to similar firms (Suarez and Lanzolla Citation2005, Citation2007). Thus, the following hypothesis is proposed:
Hypothesis 3a: The pace of technology evolution has a negative effect on firm innovative performance in clusters.
Furthermore, it is assumed that the underlying activities of research and development (R&D), namely whether basic research, applied research or experimental development are primarily conducted, can also moderate the contribution of knowledge spillovers to the realisation of a firm-specific cluster advantage. In contrast to applied research and experimental development being more commercially oriented (Czarnitzki and Thorwarth Citation2012), basic research is defined as ‘(…) experimental or theoretical work undertaken primarily to acquire new knowledge of the underlying foundation of phenomena and observable facts, without any particular application or use in view.’ (OECD Citation2002, p. 77). In other words, basic research is never some final product, but instead it refers to new knowledge that is potentially applicable in different contexts. Conducting basic research therefore provides protection against the introduction of an innovation from an unexpected (technological) direction, as companies become better capable of understanding and monitoring potential new trends as well as possible threats by competitors (Czarnitzki and Thorwarth Citation2012; Rosenberg Citation1990). Since clusters seem to be a preferable environment for radical innovations (e.g. Grashof, Hesse, and Fornahl Citation2019),Footnote8 which can lead to the formation of completely new markets and industries (e.g. Cooper and Schendel Citation1976; Henderson and Clark Citation1990; Verhoeven, Bakker, and Veugelers Citation2016), this kind of protection through basic research appears to be especially important for firms located in clusters. Besides, companies may additionally benefit from first-mover advantages, as they can gain from learning experiences as well as acquire assets, such as limited natural resources, which create entry barriers for competitors (Rosenberg Citation1990). This in turn creates a competitive advantage for subsequent applied research and development, which is supposed to be of particular importance in a highly competitive setting such as a cluster. Thus, the following hypothesis is proposed:
Hypothesis 3b: The share of basic research on the R&D activities has a positive effect on firm innovative performance in clusters.
As already highlighted at the beginning, the overall aim of this paper is to reduce the ambiguity surrounding the cluster and firm performance relationship. Therefore, the assumed effects are not just analysed separately, but also simultaneously. By taking the firm-level, cluster-level and market-/industry-level heterogeneity simultaneously into account and focussing on the interactions between these three levels of analysis, the firm performance differentials within clusters can be explained from a broader perspective.
3. Data and Methodology
In order to do so, various data sources and variables are considered for each level of analysis. Micro-level. The main database for the analysis of the innovative performance of companies within clusters is an extensive firm-level database provided by the Stifterverband. This database, which is based on a large survey taking place in a two-year rhythm, is primarily created for the use of the Federal Ministry of Education and Research in Germany. It contains innovation-related information on all identified R&D-active firms in Germany between 1995 and 2015 (Engel, Rothgang, and Eckl Citation2016; Stifterverband Citation2018). Building on this database the dependent variable for firm innovativeness can be calculated. Innovativeness is measured by the average share of the firm´s product innovations between 1997 and 2013, including incremental as well as new to the market/firm innovations, in previous 3 year sales (Delgado Citation2018; Steinberg, Procher, and Urbig Citation2017). This indicator offers two main advantages over using patents as proxy for firm innovativeness. First, the innovative output of companies that do not patent their product innovations can also be considered. Furthermore, the share of the firm´s product innovations in sales is a market-driven indicator for innovation. Consequently, unlike in the case of patents the true economic value of the corresponding innovation can be elaborated (Delgado Citation2018; Dziallas and Blind Citation2019; Kleinknecht, Van Montfort, and Brouwer Citation2002). Therefore, it is argued that the share of the firm´s product innovations in sales is for the purpose of this study more appropriate to measure firm innovativeness than patents.
As already highlighted in the previous theoretical discussion, the innovation capabilities of a firm are used as an independent variable but are measured in a twofold way. Like in previous research (e.g. Knoben et al. Citation2015; Smit, Abreu, and de Groot Citation2015), the quantitative aspect of innovation capabilities is calculated by the average share of R&D employees on the total number of employees between 1997 and 2015. In line with the indication by McCann and Folta (Citation2008) as well as Fornahl, Broekel, and Boschma (Citation2011) the qualitative aspect of innovation capabilities is additionally considered. Therefore, the cognitive proximity referring to the degree of overlap between the cluster and the firm´s knowledge base is calculated. In order to define the similarity between the cluster and the corresponding company the database from the Stifterverband is used once again. To create an average knowledge profile for each company, the internal R&D spending for each product category based on the statistical classification of products by activity in the European Economic Community is applied for the time period between 2005 and 2013. The average of all firms knowledge profiles located in one specific cluster is used to identify the knowledge profile of the corresponding cluster. To finally measure the similarity the Cosine index is estimated.
The cosine index measures the similarity between the two vectors A (firm´s knowledge profile) and B (cluster knowledge profile) for n product categories. In total, 50 different product categories are taken into consideration. The index can take a value between zero and one, where one means perfect similarity between both knowledge profiles.
For the relation and network-specific variables data about subsidised R&D collaborations from the German subsidy catalogue (‘Förderkatalog’) is utilised. The database consists of approximately more than 160.000 running or already finished R&D projects subsidised by six different ministries in the time span between 1960 and 2016 (Roesler and Broekel Citation2017). It has been already frequently used to capture (rather formal) cooperative relations in knowledge networks and it provides information at an earlier stage compared with patent data (Broekel Citation2015; Broekel and Graf Citation2012). Although this data does not provide information about all types of relations (e.g. business relations), due to the regulations agreed to when receiving a joint R&D grant, an extensive knowledge exchange is very likely, which is why this data fits to the purpose of this paper (Roesler and Broekel Citation2017). To get a preferably comprehensive picture about the firm-specific relationships, the number of subsidised R&D linkages of a firm is calculated based on the average number of links in all corresponding collaborative subsidised R&D projects between 2005 and 2014. Accordingly, two firms are linked if the participate in the same subsidised R&D project, representing a crucial channel for knowledge exchange (Fornahl, Broekel, and Boschma Citation2011). For the measurement of the level of local and extra connectedness, it is essential to make use of the cluster identification (explained in detail on the next page), which serves as the frontier between both forms of connectedness. The level of local (external) connectedness is calculated by the share of the average number of cluster internal (external) relations in the average total number of R&D linkages.
For the measurement of the firm´s geographical position within clusters, different approaches have been applied.Footnote9 In this article, the actor-based cluster index by Brenner (Citation2017), which will be described in detail in the context of the cluster identification, is applied. Besides the identification of clusters, this index offers information about the position of each actor within a cluster by considering the spatial concentration and the geographical distance on the firm-level. It can therefore also be used to determine the firm´s position within a cluster.Footnote10 High values indicate that companies are located in the centre of a cluster, whereas low values show that they are far away from clusters (Brenner Citation2017; Scholl and Brenner Citation2016).Footnote11
Meso-level. The database from the Stifterverband as well as the ‘Förderkatalog’ are also the basis for some of the variables on the meso-level. The stock of knowledge across clusters is measured by the average share of R&D employees of all firms of one specific cluster. The stock of alliances within the clusters also aggregates firm-level information. By calculating the mean of the total average R&D relationships of all firms (between 2005 and 2014) in a given cluster (based on the information from the ‘Förderkatalog’), an indicator can be derived which proxies adequately the stock of alliances within the clusters.Footnote12
Macro-level. Patents, retrieved from the database PATSTAT, are used to calculate the pace of technology evolution. In order to determine a trend and to control for possible outliers the average technological improvement (measured with the weightedFootnote13 number of patents) in three-digit NACE Rev. 2 code industries is computed for a two-year period (2012–2013). The average technological improvement is then weighted by the size of the corresponding industry, measured by the average number of employees. Despite well-discussed drawbacks, patents are widely accepted to be an adequate proxy for the technological advances in industries (Haupt, Kloyer, and Lange Citation2007; McGahan and Silverman Citation2001). For the measurement of the underlying activities of R&D the database from the Stifterverband is used, as it offers information about the share of internal R&D expenditures on basic research, applied research or experimental development. To exploit the panel structure of the database, the corresponding average share of internal R&D expenditures for the three types of activities are calculated for the time period between 2001 and 2015.
Cluster identification. As the main analysis focuses only on companies within clusters it is crucial to determine these regional clusters correctly. To identify all relevant clusters in Germany the method by Brenner (Citation2017) is applied calculating a cluster index for each single firm on the community level (‘Gemeindeebene’) based on official employment data from 2012 in three-digit NACE Rev. 2 industries. In general, the actor-based cluster identification by Brenner (Citation2017) has two main advantages over more traditional indicators, such as a regional specialisation quotient (e.g. Hervas-Oliver et al. Citation2018; Sternberg and Litzenberger Citation2004). First, it avoids the Modifiable Area Unit Problem (MAUP) because it is free of predefined borders. Consequently, in contrast to all other approaches the results of this cluster identification do not depend on the regional level that is used. As already highlighted, the corresponding cluster index can additionally be used to distinguish between the core and the periphery of a cluster. Second, it avoids a possible overvaluation of one very large company in the regional employment structure, such as VW in Wolfsburg, by considering the distance to all other firms of the same industry as a weight to the final cluster index.Footnote14 Therefore, large but geographical isolated firms are in this sense not part of a regional cluster. Besides the geographical distance, the index also considers employment in absolute terms, capturing the concentration aspect of clusters, as well as in relative terms, referring to specialisation. The used cluster index therefore corresponds adequately to the most central elements of cluster definitions (Grashof and Fornahl Citation2021).Footnote15 However, the approach requires various and rather detailed information about a set of locations (L), their geographical distances (D), the activity level at each location (vl), a set of actors (A) and their locations (la). With this information it is possible to calculate the cluster index for each actor a in the following form:
where f(d) must be a function that decreases with d. In other words, this distance decay function expresses how the relevance of activity v (location quotient and employment in absolute terms) decreases with the distance from the actor. Although there exist various alternative definitions of the distance decay function f(d), the log-logistic decay function and the radius decay function have received the most support in the literature so far (Brenner Citation2017). Following other studies (e.g. De Vries, Nijkamp, and Rietveld Citation2009; Duschl et al. Citation2015), a log-logistic decay function is finally used, being the most flexible distance decay function (Brenner Citation2017). Based on the behavioural assumptions of the literature on commuting behaviour (e.g. Johansson, Klaesson, and Olsson Citation2003) indicating that the negative travel time sensitivity is non-linear in space, the log-logistic decay function of travel time d can be expressed as follows:
where r is a parameter for the distance at which the decay function has reached a value of ½, and s defines the shape of the function. Up to now there is however little empirical knowledge about the optimal value for these two parameters (Brenner Citation2017). One crucial exception refers to Duschl et al. (Citation2015) showing that the optimal specification of these two parameters differs between industries. For the further analysis in this study, it was decided that r equals 45 minutes and s equals 7. While the former value is relatively often used within the literature (e.g. Brenner Citation2017), the latter one is inspired by the results of Duschl et al. (Citation2015), where most s values are actually at the upper bound (s = 20), but those that are not at the upper bound have a value between 5 and 10. Setting sto 7 therefore appears to be a good compromise. Although alternative specifications are not tested further, thereby leaving room for future research, the selected specifications are almost identical with the specifications tested in other recent studies, such as Brenner (Citation2017) and Ebert et al. (Citation2019), and therefore are argued to be suitable for the purpose of this study.
In addition, various control variables are included in order to account for other factors related to all three levels of analysis that might influence firm innovativeness in clusters. In line with several authors (e.g. Hervas-Oliver and Albors-Garrigos Citation2009; McCann and Folta Citation2011), firm age (years since foundation) and the company structure (dummy variable indicating whether firms are independent and do not belong to a corporate structure) are both included as firm control variables. Moreover, based on the spread of the internal R&D spending for the 50 different product categories a proxy for firm’s knowledge diversity is calculated which is assumed to positively influence firm innovativeness in clusters (Garcia-Vega Citation2006; Miller Citation2006).Footnote16 For the cluster level it is additionally controlled for cluster size, whose impact has been frequently discussed in the literature (Folta, Cooper, and Baik Citation2006; McCann and Folta Citation2011). In accordance with the most common approaches (McCann and Folta Citation2008), cluster size is measured by the average number of employees between 2008 and 2015. Furthermore, the number of research institutes within a cluster for the year 2015Footnote17 is also considered as a control variable on the cluster-level, because they play a key role in bringing different firms to cooperate as well as in promoting the generation and diffusion of knowledge within clusters (Hervas-Oliver and Albors-Garrigos Citation2007; Molina-Morales and Martınez-Fernández Citation2004; Wu et al. Citation2010). To calculate the number of research institutes within a cluster the German research directory (‘Research Explorer’) is employed, which contains information on over 25.000 university and non-university research institutes in Germany (Research Explorer Citation2018).Footnote18 Lastly, to control for research-intensive industries, which are assumed to be particularly inclined to create innovations, especially radical innovations (Tödtling, Lehner, and Trippl Citation2006), a dummy variable is included. A detailed description of all variables is listed in .
Table 1. Overview of all variables
Due to data protection regulations,Footnote19 all external data sources (e.g. relation-specific data and cluster index) could however not be directly merged with the main database provided by the Stifterverband.Footnote20 Instead as an intermediate step all external data sources had to be merged with the AMADEUSFootnote21 database offered by Bureau van Dijk (BvD). To integrate the external data with the AMADEUS database it was necessary to match the company names, as a comparable identifier is missing. As each matching algorithm has its advantages and disadvantages, four different matching algorithmsFootnote22 are applied in order to improve the overall matching quality (Raffo Citation2017; Raffo and Lhuillery Citation2009). In this context, the suggested name couples of all four matching algorithms were additionally checked manually by the author. With the unique firm identifier (BvD ID), included in the AMADEUS and Stifterverband database, the final merged data set could then be achieved. The result of this detailed procedure is a unique firm-level database which combines several data sources from different levels of analysis. It contains information about 11.889 firms in Germany from which 1.391 firms are located within a cluster. As already highlighted before, most of the variables are averaged for the years 1997 to 2015 in order to reduce a possible measurement error bias created by year-to-year variability inherent to micro-level data (Rigby and Brown Citation2015; Stern Citation2010). By forming the average for each observation i over t = 1, … T, the following equation can be derived (Cameron and Trivedi Citation2005)Footnote23
Where ,
and
.The OLS regression of the across panels´ averages equals thereby the between estimator, being particularly useful to ascertain the effect of x when x changes between companies (Cameron and Trivedi Citation2005; Gould Citation2019). By taking the hierarchical nature of the data into account, an OLS regression with cluster correction of the standard errors is applied in the main analysis ().Footnote24 Such an empirical approach is more adequate than a standard OLS regression in which the corresponding standard errors are underestimated because of the nested data structure (McNeish Citation2014; Moulton Citation1990). It was additionally tested whether a multilevel regression would be more appropriate in this context. The corresponding results of the Likelihood-ratio test indicate however, that there is no significant improvement in comparison with an OLS regression with cluster correction. Thus, it is argued that the chosen methodical approach is valid to answer the underlying research question of this paper.
In general, the analysis can be divided into three parts. First, it is investigated whether firms located within a cluster are more innovative than firms outside clusters. Second, in line with Hervas-Oliver et al. (Citation2018) as well as Hervas-Oliver and Albors-Garrigos (Citation2009) the subsample of clustered companies is analysed separately to identify the conditions under which companies can gain from the cluster environment. Third, for testing possible interaction effects and in accordance with Lee, Lee, and PENNINGS (Citation2001), the corresponding interaction terms are included one by one in order to avoid serious multicollinearity problems.
4. Results and discussion
presents a pairwise correlation matrix for all independent variables.Footnote25 In some cases the independent variables have relatively high correlations raising a potential concern of multicollinearity. In all models the corresponding Variance Inflation Factors are therefore calculated. In no case did any of the Variance Inflation Factors come close or even exceed the standard critical value of 10 (Belsley Citation1991; Myers Citation1990; Stevens Citation2002). Thus, it is concluded that multicollinearity is not a significant concern. Nevertheless, to prevent any kind of multicollinearity and thereby increasing the stability of the model estimates of the coefficients even further, in some models certain independent variables are excluded.
Table 2. Pairwise correlation matrix for the sample with cluster companies
includes the results regarding the existence of a cluster effect on firm innovativeness by using an OLS regression with robust standard errors.Footnote26 The results of model 1 and model 2 indicate that being located in a cluster asserts a highly significant positive effect on firm innovativeness. Consistent with previous empirical results (e.g. Baptista and Swann Citation1998; Bell Citation2005), it can therefore be stated that due to localisation economies firms within clusters are significantly more innovative than firms outside clusters.Footnote27
Table 3. OLS regression models with robust standard errors of single cross-section average over time for the full sample
Nevertheless, it is argued here that firms do not benefit equally and in the same manner from being located in a cluster. Consequently, tests the main formulated hypotheses regarding heterogeneous firm benefits within clusters. Model 3 illustrates the baseline model. As assumed, the coefficients for firm’s knowledge diversity, industries research-intensity and cluster size remain consistently significant positive throughout the various models. In model 4 the variables for innovation capabilities, the degree of overlap between cluster and firm knowledge base, the firm´s cluster position as well as the share of basic research are added.
Table 4. OLS regression models with clustered standard errors of single cross-section average over time for the sample with cluster companies
Table 5. OLS regression models with clustered standard errors of single cross-section average over time for interaction effects (sample with cluster companies)
4.1. Firm-level results
Regarding innovation capabilities it can be observed that, contrary to the full sample, there is a relatively strong positive but insignificant effect on innovativeness, indicating that more innovation capabilities do not lead to a significant higher innovative performance. By considering the above median of innovation capabilities, model 5 however, reveals that there is a threshold effect of innovation capabilities. Meaning that a minimum level of innovation capabilities is necessary to benefit from the cluster environment. Additionally, the results of the squared coefficient, presented in (model 8), indicate that there exists indeed a curvilinear (inverted ‘U’-shaped) effect on firm innovativeness.
In order to analyse this pattern in more detail, illustrates the corresponding quadratic prediction plot. Companies in clusters with a medium level of innovation capabilities (turning point equals a value of 0.502) present a higher effect on innovativeness than firms with very low or very high innovation capabilities. In the case of very high innovation capabilities, the effect on innovativeness can even turn into a negative one, which in accordance with Shaver and Flyer (Citation2000) can be explained with unintentional knowledge spillovers to possible competitors, which reduce a firm´s relative competitive advantage over other firms.Footnote28 Overall, the presented results are in line with very recent findings by Hervas-Oliver et al. (Citation2018) and confirm the asymmetric benefits referred to in hypothesis 1a.
Figure 1. Two-way quadratic prediction plot between innovativeness and firm´s innovation capabilities
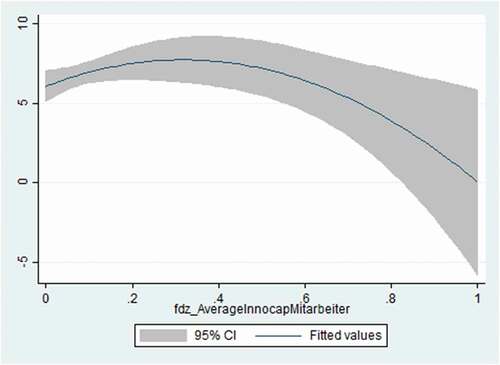
A similar pattern can be observed by analysing the more qualitative aspect of innovation capabilities, namely the degree of overlap between the firm´s and cluster´s knowledge stock. As indicated in (model 4) the effect of knowledge similarity with the cluster stock has a significant positive impact on firm innovativeness in clusters. The results of the corresponding squared coefficient, shown in (model 9), give again evidence for an inverted ‘U’-shaped effect.
The non-linear relationship between the similarity of both knowledge stocks and firm innovativeness is depicted in . Especially companies in clusters with a moderate level of cognitive proximity (turning point equals a value of 0.597) are those that profit the most. In accordance with Nooteboom (Citation2000), it can be shown that too much proximity as well as too much distance decrease the effect on innovativeness. In the former case the predicted effect on innovativeness nearly turns to be zero. Consequently, it can be resumed that the mentioned results support the proposed assumption 1b.
Figure 2. Two-way quadratic prediction plot between innovativeness and firm´s knowledge similarity with cluster stock
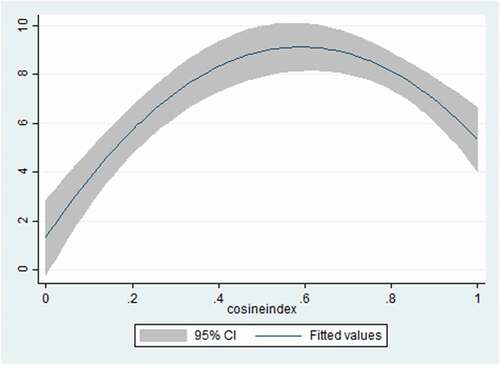
In sum, it can therefore be shown that apart from the level of innovation capabilities also the rather qualitative aspect of innovation capabilities, namely the degree of overlap between the firm´s and cluster´s knowledge stock matters for the innovative performance within clusters. Besides enriching previous scientific findings about the role of innovation capabilities in the cluster context (e.g. Hervas-Oliver et al. Citation2018), the results have also crucial implications for cluster policy. So far these policies are primarily characterised by direct R&D as well as cooperative R&D funding (Grashof Citation2020b; Zenker et al. Citation2019). However, the results of this study indicate that the concrete direction of the funding measures, referring to the question of which type of compentences is actually supported, should be considered in order to realise more targeted policy measures.
In contrast to the previous two firm-level variables, the results in (model 4) reveal that the central position within clusters alone has a positive but insignificant impact on firm innovativeness. In other words, the location in the core of the cluster does not provide by itself a competitive advantage in terms of innovative performance. The findings therefore do not confirm the assumption 1e. This can eventually be explained with the different focus of the used indicator for a firm´s central position in clusters. While other studies make use of network-specific indicators considering the concrete knowledge network within clusters (e.g. Giuliani Citation2007), the applied cluster index here primarily stresses the geographical proximity between highly concentrated and specialised companies. The relations within clusters are instead considered separately in model 6.
Prior to this, in model 5 the hypothesis 1 c concerning the influence of the number of firm linkages on innovativeness is tested. Contrary to the significant positive findings in the full sample, the results of the cluster sample show no significant effect of the number of linkages. Hypothesis 1 c has therefore to be rejected. In other words, in clusters the pure number of relationships, including cluster internal as well cluster external relationships, does not significantly foster firm innovativeness. Consequently, in model 6 the concrete impact of the share of cluster external relations is further investigated. It can be shown that the share of cluster external relations asserts a positive, however, statistically insignificant influence on firm innovative performance.Footnote29 The effect of the share of cluster internal relations is respectively negative and insignificant.Footnote30 The findings therefore do not confirm the assumption 1d.
4.2. Cluster-level and market-/industry-level results
Besides these firm-level influences, also includes results for the cluster-level as well as market-/industry-level. By investigating the influence of the share of basic research on firm innovativeness in clusters, a significant positive effect can be asserted throughout all models (3–9). Meaning that a higher share of basic research leads to a significant higher innovative performance. This seems to be a cluster-specific effect, as in the full sample it turns to be (significant) negative. Conducting basic research is therefore argued to be especially important for the innovative performance of companies in clusters, because it provides protection against the competitive setting of clusters in which possible threats by competitors in terms of new unexpected technological developments may arise (Czarnitzki and Thorwarth Citation2012; Grashof, Hesse, and Fornahl Citation2019; Rosenberg Citation1990). Hypothesis 3b can therefore be confirmed.
The seventh model of focuses on additional variables from the meso- and macro-level. The previous emphasised results for the share of basic research remain thereby unchanged. Additionally, the stock of knowledge of the cluster does not significantly influence firm innovative performance. So that the assumed generation of larger performance effects in clusters with a relatively high knowledge stock cannot be confirmed (hypothesis 2a). Nevertheless, the findings of model 7 show at the same time that the stock of alliances within clusters asserts a relatively high significant positive impact on firm innovative performance. As expected, in an alliance-rich cluster the chance to extract knowledge from local and external connections seems to be enhanced, as it is much easier to get in contact with a broad variety of partners than in an alliance-poor cluster. Hypothesis 2b can therefore be supported. Furthermore, the results of model 7 reveal that, in contrast to the full sample, the pace of technology evolution becomes insignificant. While in general asserting a significant positive impact on firm innovativeness, in clusters the pace of technology evolution seems not to have a statistically relevant stand-alone effect. Hypothesis 3a can therefore not be supported. The results of both samples however indicate that the assumption of a negative effect should be reformulated into a positive one. Meaning that companies primarily active in rather young industries, normally characterised by a fast technology evolution (Clark Citation1985; Klepper Citation1997; Neffke et al. Citation2011), are at least in the full sample more innovative than companies engaged in mature industries.
4.3. Interaction results
By investigating potential interaction termsFootnote31 in , it can be however shown that the pace of technology evolutionFootnote32 asserts statistically relevant moderation effects. The results of model 11 reveal such an effect in the context of the firm´s innovation capabilities. In industries characterised by a fast pace of technology evolution, innovation capabilities have a positive significant impact on firm innovative performance. For companies active in such industries, it is therefore especially important to own sufficiently high innovation capabilities, because they allow to access and integrate new knowledge, which is frequently changing under a rapid technology evolution.
A possible interaction effect between the pace of technology evolution and firm knowledge similarity with the cluster stock is additionally tested in model 12. Under a rapid technology evolution, it is assumed that firms need to have a larger cognitive distance towards the cluster knowledge stock in order to be more open to new external ideas, thereby preventing a possible lock-in situation. The corresponding interaction term indeed asserts a negative, however, insignificant impact on firm innovativeness.
To gain more insights about the relevance of firm-specific relationships (both the absolute number of overall relationships and the share of cluster external relations have no significant stand-alone influence), a possible interaction effect between the position within clusters and the cluster external relations is additionally analysed. The results, presented in (model 10), reveal that cluster external relations are especially important for firms located within the centre of clusters.Footnote33 In such a position, cluster external relations have a significant positive effect on firm innovativeness. A reasonable explanation for the difference between the centre and the periphery of a cluster can be found by comparing the cluster internal connectedness between both groups. Indeed, the mean of cluster internal relations is significantly higher in the centre than in the periphery of a cluster.Footnote34 The risk for a lock-in situation, preventing innovations, is therefore potentially higher in the centre than in the periphery of a cluster. Thus, for companies located in the core of a cluster it is particularly important to possess external relations. These cluster external relations provide a necessary channel for new knowledge that is different from the cluster internal knowledge.
Finally in model 13, it is investigated whether a fast pace of technology evolution moderates the relationship between firm cluster external relations and firm innovativeness. As assumed, under a fast pace of technology evolution, firm cluster external relations assert a strong significant effect on firm innovative performance. Meaning that these cluster external relations are especially crucial in industries characterised by a fast pace of technology evolution. The rapid evolution requires that firms gain access towards external knowledge sources in order to secure that their current knowledge stock does not become obsolete in the near future.
Regarding the relation-specific variables, it can therefore be resumed that the number of cluster external relationships does not significantly influence by itself firm innovative performance in clusters. Nevertheless, it becomes relevant when the position within the cluster and the pace of technology evolution are additionally considered.
Overall, evidence is found that several variables from different levels of analysis directly as well as interactively influence firm innovative performance in clusters.Footnote35 The derived results are on the one hand in line with the literature reporting a significant positive relationship between being located in a cluster and firm innovativeness (e.g. Baptista and Swann Citation1998; Bell Citation2005). On the other hand, the results also suggest that firms benefit unequally within the cluster environment. The results, however, differ in this context from similar studies (e.g. Hervas-Oliver et al. Citation2018; McCann and Folta Citation2011), because variables from three different levels of analysis are seperately as well as interactively tested, thereby enriching the current discussion about contextual conditions that contribute to firms’ heterogenous benefits within clusters. As a further robustness check of the presented results, all models have been calculated for an alternative dependent variable: radical innovativeness. In contrast to the main dependent variable, radical innovativeness is calculated only by the average share of the firm´s product innovations in previous three years sales (1997–2013), which are new to the market and/or new to the firm. The corresponding results are in line with the findings for firm innovativeness. The relation-specific variables as well as the pace of technology evolution have been additionally tested for different time periods. In both cases no relevant changes could be detected.Footnote36
5. Conclusion
Regional clusters have become an inseparable component of modern economies. However, it is still rather unclear whether companies’ innovative performance really benefits from being located in a cluster and, even more importantly, which conditions are necessary to profit particularly from the cluster environment (Festing, Royer, and Steffen Citation2012; Frenken, Cefis, and Stam Citation2013; Martin and Sunley Citation2003). By integrating theoretical perspectives from the strategic management (e.g. RBV, RV and MBV) as well as economic geography literature (e.g. cluster approach), these essential questions are answered with a specific focus on moderating variables that rather refer to the Marshallian externality of knowledge spillovers. The corresponding main empirical results for the three different levels of analysis (micro-level, meso-level, and macro-level) can be resumed as follows: (1.) Being located in a cluster increases on average firm innovative performance. (2.) However, firms gain unequally from the cluster environment. To profit the most from available knowledge spillovers firms need to have, for example, a medium level of innovation capabilities and knowledge similarity with the cluster stock. Besides, a high share of basic research and a pronounced stock of alliances within clusters are also beneficial conditions for firm innovativeness in clusters. Consequently, in light of the results for the stock of alliances within clusters, it can be argued that the concept of local buzz and global pipelines, proposed by Bathelt, Malmberg, and Maskell (Citation2004), can be extended with a cluster dimension. (3.) Nevertheless, evidence for interaction effects between the three levels of analysis can be found. Due to the significant higher number of cluster internal relations in the cluster centre, promoting a lock-in situation, it is crucial that firms located within the centre of a cluster possess sufficient cluster external relationships. Apart from the concrete location within clusters, the pace of technology evolution also has to be considered as a moderating variable. Under a rapid technology evolution, firms need to own sufficiently high innovation capabilities to gain from knowledge spillovers. They allow for access to and integration of new knowledge, which is frequently changing in an industry with rapid technology evolution. Moreover, this paper provides evidence that the rapid evolution also requires that firms have cluster external relations through which they can access external knowledge sources in order to guarantee that the current knowledge stock does not become obsolete.
However, there are some limitations to this study, which can be seen as the starting point for future research. First of all, the study does not consider the dynamic evolution across the cluster life cycle (e.g. Menzel and Fornahl Citation2010). Due to data availability the corresponding cluster index could be calculated only for the year 2012. Future research may investigate several years to capture the cluster life cycle and its possible impact on the sustainability of firm innovative performance in clusters. In this context, it is additionally suggested to make use of panel-regressions. The underlying data constraints of this studyFootnote37 have prevented the application of such approaches, instead the study applied an OLS regression with clustered standard errors to a single cross-section of variables average over time (between estimator). Historically, such an approach has, however, been criticised due to a concern that omitted variables, represented by the individual effects of the error term, may be correlated with the independent variables, leading to inconsistent results. Nevertheless, such a potential bias is also valid for other approaches.Footnote38 Moreover, it only constitutes one of several possible misspecifications of such models (Baltagi Citation2005; Hauk Jr. and Wacziarg, Citation2009; Mairesse and Sassenou Citation1991; Stern Citation2010). In view of the underlying research question concentrating more on the between-variation and the available data it is therefore stated that the selected methodical approach is suitable despite its limitations in contrast to panel-regressions (Griliches and Mairesse Citation1984; Hauk Jr. and Wacziarg, Citation2009; Kafouros Citation2008). In empirical studies using innovation surveys it is indeed quite common to rather exploit cross-sectional data (Mairesse and Mohnen Citation2010), so that it may be a promising research gap for future studies to implement panel-regressions in order to investigate properly the dynamic evolution across the cluster life cycle. Moreover, although this study has at least an implicit focus on knowledge spillovers by investigating moderating variables that are more related to the underlying notion of knowledge spillovers, similar to other studies (e.g. McCann and Folta Citation2011), the concrete localisation externalities are not directly measured here. For future research, it is therefore quite promising to further develop the measurement of the specific externalitiesFootnote39 in order to disentangle the firm-specific cluster effect into its components and thereby investigate in more detail the reasons behind the heterogeneity of firm performance within clusters. Lastly, the concrete partners and their knowledge profiles are not taken into consideration in the applied relation-specific variables. For future research it may be interesting to analyse in detail whether and under which conditions the type of partners (universities, small and medium-sized enterprises etc.) and their knowledge profiles matter for firm innovativeness in clusters.
Nevertheless, all in all it can be resumed that the derived results about the conditions necessary to profit from the cluster environment contribute to closing a still ubiquitous research gap. Although especially recent contributions (e.g. Hervas-Oliver et al. Citation2018; Lee et al., Citation2018) go in this context in the right direction by considering potential moderating variables, they do not go far enough. The findings of this study indicate that it is not sufficient to only focus on single contextual variables from one level of analysis, but instead it is necessary to pursue a unified approach where multilevel conditions and their respective interactions are considered. Additionally, the results also have a pragmatic meaning for companies as well as policy makers, because both can evaluate better under which conditions firms’ innovative performance is more likely to benefit from locating within clusters. Company managers can therefore, on the one hand, prevent expensive ill-reflected location decisions and, on the other hand, they can maximise the potential profits from being located in their corresponding cluster. From a policy perspective, the results indicate that policy makers should establish initiatives that are customised to the specific firm, cluster, and market/industry characteristics, instead of realising generic cluster policies. For instance, instead of indiscriminately fostering cooperative R&D, regardless of the existing circumstances, the results of this study draw the conclusion that particularly central firms located in the cluster core should be supported in building cluster external relations in order to avoid a possible lock-in situation. Such context-oriented cluster policy approaches would efficiently support those firms that really need help to benefit from the cluster and thereby work towards creating a cluster environment from which all companies can gain.
Acknowledgments
The author would like to thank the Wissenschaftsstatistik GmbH of the Stifterverband für die Deutsche Wissenschaft for providing access to the data (in particular Barbara Grave, Thu-Van Nguyen and Julia Angenendt for their continuous support) as well as the participants of the 4th Geography of Innovation conference 2018 in Barcelona and the research symposium 2018 in Kolding for useful comments on earlier drafts. Furthermore, the author would also like to thank Thomas Brenner for the lively exchange and support in the creation of the cluster index. Moreover, the author would like to thank the Associate Editor Andrea Morrison as well as two anonymous reviewers for their constructive feedback. Furthermore, the author gratefully acknowledges financial support from the Federal Ministry of Education and Research [grant number 03INTBF05A].
Disclosure statement
No potential conflict of interest was reported by the author(s).
Notes
1 A comprehensive overview about the financial budgets of different cluster programs in Europe is provided by Zenker et al. (Citation2019).
2 Although not directly measured (see limitations), the considered moderating variables refer more to the underlying notion of knowledge spillovers.
3 In accordance with Barney (Citation1991) resources are here defined as ‘all assets, capabilities, organizational process, firm attributes, information, knowledge, etc. controlled by a firm that enable the firm to conceive of and implement strategies that improve its efficiency and effectiveness.’ (Barney Citation1991, p. 101).
4 In accordance with Hervas-Oliver et al. (Citation2018) the terms absorptive capacity and innovation capability are used interchangeably.
5 According to Dyer and Singh (Citation1998) relational rents are defined as: ‘(…) supernormal profit jointly generated in an exchange relationship that cannot be generated by either firm in isolation and can only be created through the joint idiosyncratic contributions of the specific alliance partners.’ (Dyer and Singh Citation1998, p. 662).
6 Important exceptions are in this context Knoben et al. (Citation2015) as well as Rigby and Brown (Citation2015).
7 For example, clusters can be found in the ceramic tile and fashion industry (e.g. in north-east Italy), in the software industry (e.g. in Bangalore in India) and in the automotive industry (e.g. in south-western Germany).
8 However, it should be noted that there are also conflicting findings on the impact of clusters on the emergence of radical innovations (e.g. Hervas-Oliver et al. Citation2019), so further investigations in this new research field are needed.
9 For a good overview of the most common distance measures and functions, please see Brenner (Citation2017).
10 For a good visualisation, please review in Grashof, Hesse, and Fornahl (Citation2019).
11 As a first robustness check, for the firm´s centrality within the corresponding cluster core a dummy variable has additionally been calculated based on the above median of the cluster index (equal to a value of 2.83). The results remain thereby the same and can be provided upon request.
12 Despite the importance of R&D collaborations (e.g. Aschhoff and Schmidt Citation2008), as on the firm-level, other types of relations, such as business relations, are not considered. Due to the high relevance of R&D collaborations for the knowledge transmission, the used proxy is, however, argued to be in line with the original idea of McCann and Folta (Citation2008).
13 Weighted number of patents means in this context that the corresponding applicant share is used. For example, on a patent with two applicants, each of them get an applicant share of 0.5.
14 The applied distance decay function is thereby based on travel times, where 45 minutes represent the limit for close geographical distance (Brenner Citation2017; Scholl and Brenner Citation2016).
15 In line with the procedure of the European Cluster Observatory a value of 2 is applied as the corresponding cluster threshold, indicating whether a firm is located in a cluster or not (European Cluster Observatory Citation2018; European Commission Citation2008).
16 To also control for the knowledge diversity within clusters (see model 7 in ), the average spread of the company´s average internal R&D expenditure for the 50 different product categories within clusters is calculated.
17 Due to data constraints, the average number of research institutes within clusters could not be calculated for a longer time period.
18 For the final analysis, however, only the highest organisational level of the research institutes, e.g. universities and not their working groups, are considered.
19 For further information on this please review the terms of use available under https://www.stifterverband.org/fdz.
20 This is, however, a rather typical problem when using individual firm data (e.g. Mairesse and Mohnen Citation2010).
21 Indeed, a combination between AMADEUS and ORBIS databases is used in order to cover preferably all listed firms in Germany.
22 In more concrete terms, the Token, N-Gram, Soundex and Token Soundex algorithms were used.
23 Due to some changes in the questionnaire of the Stifterverband the dependent variable can only be averaged for the time period between 1997 and 2013. By only using the average it is argued that the results will be unaffected. As a control and a further robustness check the independent variables were also averaged for exactly the same time period as the dependent variable. The corresponding results of this robustness check are in line with the original results and can be provided upon request.:
24 The standard errors are clustered by regional cluster.
25 The corresponding descriptive statistics for all main variables can be provided upon request.
26 Since the focus of this paper is actually on the conditions through which firms can gain from being located in a cluster, only the influence of the cluster affiliation is explained here. Other selected influences from are, however, described if they differ from the results of the moderating variables presented in .
27 Following the remarks by McCann and Folta (Citation2011), it is argued that the concern that the most innovative firms choose to locate in clusters, creating a potential selection bias, cannot be justified empirically nor theoretically. This can also be confirmed by the mean and the standard deviation of firm innovativeness within clusters. The corresponding results can be provided upon request.
28 More and stronger competition, promoted through knowledge leakages, will likely reduce the share of the focal firm´s product innovations in its sales.
29 As a further sensitivity test, the effect of the degree of cluster external relations instead of the share of cluster external relations is additionally investigated. The results remain thereby however the same (positive and insignificant) and can be provided upon request.
30 To verify the results, model 6 has been replicated without the share of cluster external relations. Instead, a new dummy variable has been added, indicating whether firms own a moderate share of cluster internal (external) relations between 0.3 and 0.7. The corresponding results remain insignificant und can be provided upon request.
31 In line with the approach by Hainmueller, Mummolo, and Xu (Citation2019), the underlying LIE assumption (linear interaction effect) is tested. For all interaction terms the corresponding results of the suggested tests confirm that the LIE assumption is correct.
32 Here calculated as a dummy variable, where 1 means that the pace of technology evolution is equal or higher than the corresponding 75th quantile. The 75th quantile has been chosen, as at the very end of the distribution (from the 90th quantile upwards) the number of observations for a fast technology evolution becomes too small for a further analysis. Nevertheless, in light of other contributions using for example simply the mean as a threshold (e.g. Audretsch and Feldman Citation1996), it is argued that the 75th quantile represents a reasonable threshold for industries with a fast pace of technology evolution.
33 To simplify the interpretation of the corresponding interaction term, a dummy variable is used for the firm´s central position within the cluster core (calculated based on the above median of the cluster index; equal to a value of 2.83). However, by directly using the cluster index (discrete variable) the results remain the same and can be provided upon request.
34 Results can be provided upon request.
35 A summary of all main results can also be found in Table A1 (appendix).
36 The results of all robustness checks can be provided upon request.
37 Since the underlying survey questions of the dependent and independent variables are only inconsistently answered over the years (e.g. Mairesse and Mohnen Citation2010), a regression on a balanced panel database would result in a significant loss of observations, thereby creating a potential bias. In more concrete terms, in the underlying panel sample of this study 50% of the firms answer only four times (between 1997 and 2015) the corresponding survey questions.
38 The results of the carried out omitted variables tests, however, suggest that such a bias is not a mayor concern in this study.
39 The procedure by Rigby and Brown (Citation2015) can in this context serve as a valuable starting point, even though, following Krugman (Citation1991), they also argue that knowledge spillovers cannot adequately be measured in a direct way (e.g. through patents).
References
- Ahuja, G. 2000. “Collaboration Networks, Structural Holes, and Innovation: A Longitudinal Study.” Administrative Science Quarterly 45 (3): 425–455. doi: https://doi.org/10.2307/2667105.
- Aschhoff, B., and T. Schmidt. 2008. “Empirical Evidence on the Success of R&D Cooperation—Happy Together?” Review of Industrial Organization 33 (1): 41–62. doi: https://doi.org/10.1007/s11151-008-9179-7.
- Audretsch, D. B., and M. P. Feldman. 1996. “Innovative Clusters and the Industry Life Cycle.” Review of Industrial Organization 11 (2): 253–273. doi: https://doi.org/10.1007/BF00157670.
- Baldwin, J. R., D. Beckstead, W. M. Brown, and D. L. Rigby. 2008. “Agglomeration and the Geography of Localization Economies in Canada.” Regional Studies 42 (1): 117–132. doi: https://doi.org/10.1080/00343400701543199.
- Baltagi, B. H. 2005. Econometric Analysis of Panel Data. England: John Wiley & Sons Ltd, West Sussex.
- Baptista, R., and P. Swann. 1998. “Do Firms in clusters Innovate More?” Research Policy 27 (5): 525–540. doi: https://doi.org/10.1016/S0048-7333(98)00065-1.
- Barney, J. B. 1991. “Firm Resources and Sustained Competitive Advantage.” Journal of Management 17 (1): 99–120. doi: https://doi.org/10.1177/014920639101700108.
- Bathelt, H., A. Malmberg, and P. Maskell. 2004. “Clusters and Knowledge: Local Buzz, Global Pipelines and the Process of Knowledge Creation.”Progress in Human Geography 28 (1): 31–56. doi: https://doi.org/10.1191/0309132504ph469oa.
- Beaudry, C., and S. Breschi. 2003. “Are Firms in Clusters Really More Innovative?” Economics of Innovation and New Technology 12 (4): 325–342. doi: https://doi.org/10.1080/10438590290020197.
- Bell, G. G. 2005. “Clusters, Networks, and Firm Innovativeness.” Strategic Management Journal 26 (3): 287–295. doi: https://doi.org/10.1002/smj.448.
- Belsley, D. A. 1991. Conditioning Diagnostics: Collinearity and Weak Data in Regression. New York: Wiley.
- Boschma, R. 2005. “Proximity and Innovation: A Critical Assessment.” Regional Studies 39 (1): 61–74. doi: https://doi.org/10.1080/0034340052000320887.
- Boschma, R., and K. Frenken. 2010. “The Spatial Evolution of Innovation Networks. A Proximity Perspective.” In Handbook of Evolutionary Economic Geography, edited by R. Boschma and R. Martin, 120–138. Cheltenham: Edward Elgar.
- Brenner, T. (2017): “Identification of Clusters – An Actor-based Approach”, Working Papers on Innovation and Space, No. 02.17, Philipps University Marburg.
- Broekel, T. 2015. “The Co-evolution of Proximities – A Network Level Study.” Regional Studies 49 (6): 921–935. doi: https://doi.org/10.1080/00343404.2014.1001732.
- Broekel, T., D. Fornahl, and A. Morrison. 2015. “Another Cluster Premium: Innovation Subsidies and R&D Collaboration Networks.” Research Policy 44 (8): 1431–1444. doi: https://doi.org/10.1016/j.respol.2015.05.002.
- Broekel, T., and H. Graf. 2012. “Public Research Intensity and the Structure of German R&D Networks: A Comparison of 10 Technologies.” Economics of Innovation and New Technology 21 (4): 345–372. doi: https://doi.org/10.1080/10438599.2011.582704.
- Broekel, T., and R. Boschma. 2012. “Knowledge Networks in the Dutch Aviation Industry: The Proximity Paradox.” Journal of Economic Geography 12 (2): 409–433. doi: https://doi.org/10.1093/jeg/lbr010.
- Brown, K., J. Burgees, M. Festing, S. Royer, and C. Steffen (2007): “The Value Adding Web – A Conceptual Framework of Competitive Advantage Realisation in Clusters”, ESCP-EAP Working Paper, No. 27, ISSN: 1619-7658.
- Cameron, A. C., and P. K. Trivedi. 2005. Microeconometrics - Methods and Applications. NY, USA: Cambridge University Press.
- Clark, K. B. 1985. “The Interaction of Design Hierarchies and Market Concepts in Technological Evolution.” Research Policy 14 (5): 235–251. doi: https://doi.org/10.1016/0048-7333(85)90007-1.
- Cohen, W. M., and D. A. Levinthal.1990.“Absorptive Capacity: A New Perspective on Learning and Innovation”.Administrative Science Quarterly 351: 128–152. Special Issue: Technology. doi: https://doi.org/10.2307/2393553.
- Cooper, A. C., and D. Schendel. 1976. “Strategic Responses to Technological Threats.” Business Horizons 19 (1): 61–69. doi: https://doi.org/10.1016/0007-6813(76)90024-0.
- Czarnitzki, D., and S. Thorwarth. 2012. “Productivity Effects of Basic Research in Low-tech and High-tech Industries.” Research Policy 41 (9): 1555–1564. doi: https://doi.org/10.1016/j.respol.2012.04.009.
- Daft, R. L., and R. H. Lengel. 1986. “Organizational Information Requirements, Media Richness and Structural Design.” Management Science 32 (5): 554–571. doi: https://doi.org/10.1287/mnsc.32.5.554.
- De Silva, D. G., and R. P. McComb. 2012. “Geographic Concentration and High Tech Firm Survival.” Regional Science and Urban Economics 42 (4): 691–701. doi: https://doi.org/10.1016/j.regsciurbeco.2012.03.001.
- Delgado, M. 2018. “Firms in Context: Internal and External Drivers of Success.” In The New Oxford Handbook of Economic Geography, edited by G. L. Clark, M. P. Feldman, M. S. Gertler, and D. Wójcik, 324–346, Oxford, UK: Oxford University Press.
- Duranton, G., and W. R. Kerr (2015): “The Logic of Agglomeration, NBER Working Paper, No. 21452”, https://doi.org/10.3386/w21452.
- Duschl, M., T. Scholl, T. Brenner, D. Luxen, and F. Raschke. 2015. “Industry-specific Firm Growth and Agglomeration.” Regional Studies 49 (11): 1822–1839. doi: https://doi.org/10.1080/00343404.2013.861059.
- Dyer, J. H., and H. Singh. 1998. “The Relational View: Cooperative Strategy and Sources of Interorganizational Competitive Advantage.” The Academy of Management Review 23 (4): 660–679. doi: https://doi.org/10.5465/amr.1998.1255632.
- Dziallas, M., and K. Blind. 2019. “Innovation Indicators Throughout the Innovation Process: An Extensive Literature Analysis.” Technovation 80-81: 3–29.
- Ebert, T., J. E. Gebauer, T. Brenner, W. Bleidorn, S. D. Gosling, J. Potter, and P. J. Rentfrow (2019): “Are Regional Differences in Personality and Their Correlates Robust? Applying Spatial Analysis Techniques to Examine Regional Variation in Personality across the US and Germany”, Working Papers on Innovation and Space, No. 05.19, Philipps University Marburg.
- Eder, J. 2019. “Innovation in the Periphery: A Critical Survey and Research Agenda.” International Regional Science Review 42 (2): 119–146. doi: https://doi.org/10.1177/0160017618764279.
- Engel, D., M. Rothgang, and V. Eckl. 2016. “Systemic Aspects of R&D Policy Subsidies for R&D Collaborations and Their Effects on Private R&D.” Industry and Innovation 23 (2): 206–222. doi: https://doi.org/10.1080/13662716.2016.1146127.
- European Cluster Observatory (2018): “Methodology – Indicators”, available under http://wwwclusterobservatoryeu/indexhtml#!view=aboutobservatory;url=/about-observatory/methodology/indicators/,lastretrieved:04102018, last retrieved: 04.10.2018.
- European Commission (2008): “The Concept of Clusters and Cluster Policies and Their Role for Competitiveness and Innovation: Main Statistical Results and Lessons Learned”, Europe INNOVA/PRO INNO Europe paper N° 9.
- EuropeanCommission. 2016. Smart Guide to Cluster Policy, Guidebook Series: How to Support SME Policy from Structural Funds. Belgium: European Union doi: https://doi.org/10.2873/729624.
- Festing, M., S. Royer, and C. Steffen. 2012. “Unternehmenscluster schaffen Wettbewerbsvorteile - Eine Analyse des Uhrenclusters in Glashütte.” Zeitschrift Führung und Organisation 81 (4): 264–272.
- Folta, T. B., A. C. Cooper, and Y. Baik. 2006. “Geographic Cluster Size and Firm Performance.” Journal of Business Venturing 21 (2): 217–242. doi: https://doi.org/10.1016/j.jbusvent.2005.04.005.
- Fornahl, D., T. Broekel, and R. Boschma. 2011. “What Drives Patent Performance of German Biotech Firms? The Impact of R&D Subsidies, Knowledge Networks and Their Location.” Papers in Regional Science 90 (2): 395–418. doi: https://doi.org/10.1111/j.1435-5957.2011.00361.x.
- Frenken, K., E. Cefis, and E. Stam (2013): “Industrial Dynamics and Clusters: A Survey, Tjalling C”. Koopmans Research Institute, Discussion Paper Series nr: 13–11, Utrecht School of Economics.
- Garcia-Vega, M. 2006. “Does Technological Diversification Promote Innovation? an Empirical Analysis for European Firms.” Research Policy 35 (2): 230–246. doi: https://doi.org/10.1016/j.respol.2005.09.006.
- Giuliani, E. 2007. “The Selective Nature of Knowledge Networks in Clusters: Evidence from the Wine Industry.” Journal of Economic Geography 7 (2): 139–168. doi: https://doi.org/10.1093/jeg/lbl014.
- Gould, W. (2019): “Between Estimators”, available under: https://www.stata.com/support/faqs/statistics/between-estimator/,lastretrieved:01112018
- Grashof, N. 2020a. “Firm-specific Cluster Effects: A Meta-analysis.” Papers in Regional Science 99 (5): 1237–1260. doi: https://doi.org/10.1111/pirs.12526.
- Grashof, N. (2021): “Putting the Watering Can Away - Towards a Targeted (Problem-oriented) Cluster Policy Framework.”Research Policy50 (9): 1-23. doi: https://doi.org/10.1016/j.respol.2021.104335.
- Grashof, N., and D. Fornahl. (2021): ““To be or not to be” located in a cluster? - A descriptive meta-analysis of the firm-specific cluster effect”. Annals of Regional Science. doi: https://doi.org/10.1007/s00168-021-01057-y.
- Grashof, N., K. Hesse, and D. Fornahl. 2019. “Radical or Not? the Role of Clusters in the Emergence of Radical Innovations.” European Planning Studies 27 (10): 1904–1923. doi: https://doi.org/10.1080/09654313.2019.1631260.
- Griliches, Z., and J. Mairesse. 1984. “Productivity and R&D at the Firm Level.” In R&D, Patents and Productivity, edited by Z. Griliches, 339–374, Chicago, USA: University of Chicago Press.
- Grillitsch, M., and M. Nilsson (2017): “Knowledge Externalities and Firm Heterogeneity: Effects on High and Low Growth Firms”, Papers in Innovation Studies, Paper no. 2017/06.
- Hainmueller, J., J. Mummolo, and Y. Xu. 2019. “How Much Should We Trust Estimates from Multiplicative Interaction Models? Simple Tools to Improve Empirical Practice.” Political Analysis 27 (2): 163–192. doi: https://doi.org/10.1017/pan.2018.46.
- Harrison, B., M. R. Kelley, and J. Gant. 1996. “Innovative Firm Behavior and Local Milieu: Exploring the Intersection of Agglomeration, Firm Effects, and Technological Change.” Economic Geography 72 (3): 233–258. doi: https://doi.org/10.2307/144400.
- Hauk, J., . W. R., and R. Wacziarg. 2009. “A Monte Carlo Study of Growth Regressions.” Journal of Economic Growth 14 (2): 103–147. doi: https://doi.org/10.1007/s10887-009-9040-3.
- Haupt, R., M. Kloyer, and M. Lange. 2007. “Patent Indicators for the Technology Life Cycle Development.” Research Policy 36 (3): 387–398. doi: https://doi.org/10.1016/j.respol.2006.12.004.
- Henderson, J. V. 2003. “Marshall’s Scale Economies.” Journal of Urban Economics 53 (1): 1–28. doi: https://doi.org/10.1016/S0094-1190(02)00505-3.
- Henderson, R. M., and K. B. Clark. 1990. “Architectural Innovation: The Reconfiguration of Existing Product Technologies and the Failure of Established Firms.” Administrative Science Quarterly 35 (1): 9–30. doi: https://doi.org/10.2307/2393549.
- Hervas-Oliver, J. L., and J. Albors-Garrigos. 2007. “Do Clusters Capabilities Matter? an Empirical Application of the Resource-based View in Clusters.” Entrepreneurship & Regional Development: An International Journal 19 (2): 113–136. doi: https://doi.org/10.1080/08985620601137554.
- Hervas-Oliver, J.-L., & Albors-Garrigos, J. 2009. The role of the firm’s internal and relational capabilities in clusters: when distance and embeddedness are not enough to explain innovation, Journal of Economic Geography, Vol. 9, Issue 2, pp. 263–283
- Hervas-Oliver, J.-L., F. Sempere-Ripoll, R. R. Alvarado, and S. Estelles-Miguel. 2018. “Agglomerations and Firm Performance: Who Benefits and How Much?” Regional Studies 52 (3): 338–349. doi: https://doi.org/10.1080/00343404.2017.1297895.
- Hervas-Oliver, J.-L., F. Sempere-Ripoll, S. Estelles-Miguel, and R. Rojas-Alvarado. 2019. “Radical Vs Incremental Innovation in Marshallian Industrial Districts in the Valencian Region: What Prevails?” European Planning Studies 27 (10): 1924–1939. doi: https://doi.org/10.1080/09654313.2019.1638887.
- Hervas-Oliver, J.-L., and J. Albors-Garrigos. 2007. “The Role of the firm’s internal and relational capabilities in clusters: when distance and embeddedness are not enough to explain innovation.” Journal of Economic Geography 9 (2): 263–283. doi: https://doi.org/10.1093/jeg/lbn033.
- Jaffe, A. B., M. Trajtenberg, and R. Henderson. 1993. “Geographic Localization of Knowledge Spillovers as Evidenced by Patent Citations.” Quarterly Journal of Economics 108 (3): 577–598. doi: https://doi.org/10.2307/2118401.
- Johansson, B., J. Klaesson, and M. Olsson. 2003. “Commuters’ Non-linear Response to Time Distances.” Journal of Geographical Systems 5 (3): 315–329. doi: https://doi.org/10.1007/s10109-003-0111-2.
- Kafouros, M. I. 2008. Industrial Innovation and Firm Performance: The Impact of Scientific Knowledge on Multinational Corporations. Cheltenham, UK: Edward Elgar Publishing.
- Kleinknecht, A., K. Van Montfort, and E. Brouwer. 2002. “The Non-Trivial Choice between Innovation Indicators.” Economics of Innovation and New Technology 11 (2): 109–121. doi: https://doi.org/10.1080/10438590210899.
- Klepper, S. 1997. “Industry Life Cycles.” Industrial and Corporate Change 6 (1): 145–182. doi: https://doi.org/10.1093/icc/6.1.145.
- Knoben, J., A. T. Arikan, F. Van Oort, and O. Raspe. 2015. “Agglomeration and Firm Performance: One Firm’s Medicine Is Another Firm’s Poison.” Environment & Planning A 48 (1): 1–22.
- Kohlbacher, M., D. Weitlaner, A. Hollosi, S. Grünwald, and H.-P. Grahsl. 2013. “Innovation in Clusters: Effects of Absorptive Capacity and Environmental Moderators.” Competitiveness Review: An International Business Journal 23 (3): 199–217. doi: https://doi.org/10.1108/10595421311319807.
- Krugman, P. 1991. “Increasing Returns and Economic Geography.” Journal of Political Economy 99 (3): 483–499. doi: https://doi.org/10.1086/261763.
- Lavie, D. 2006. “The Competitive Advantage of Interconnected Firms: An Extension of the Resource-Based View.” The Academy of Management Review 31 (3): 638–658. doi: https://doi.org/10.5465/amr.2006.21318922.
- Lee, C., K. Lee, and J. M. Pennings. 2001. “Internal Capabilities, External Networks, and Performance: A Study on Technology-Based Ventures.” Strategic Management Journal 22 (6–7): 615–640. doi: https://doi.org/10.1002/smj.181.
- Lee, C.-Y. 2018. “Geographical Clustering and Firm Growth: Differential Growth Performance among Clustered Firms.” Research Policy 47 (6): 1173–1184. doi: https://doi.org/10.1016/j.respol.2018.04.002.
- Mairesse, J., and P. Mohnen. 2010. “Using Innovation Surveys for Econometric Analysis.” In Handbook of the Economics of Innovation, edited by B. H. Hall and N. Rosenberg, 1129–1155. Vol. 2, Amsterdam, Netherlands: Elsevier.
- Mairesse, J., and M. Sassenou (1991): “R&D Productivity: A Survey of Econometric Studies at the Firm Level”, NBER Working PaperNo. 3666 .
- Malmberg, A., and P. Maskell. 2002. “The Elusive Concept of Localization Economies: Towards a Knowledge-based Theory of Spatial Clustering.” Environment & Planning A 34 (3): 429–449. doi: https://doi.org/10.1068/a3457.
- Marshall, A. 1920. Principles of Economics. 8th edition ed. London: Macmillan.
- Martin, P., T. Mayer, and F. Mayneris. 2011. “Public Support to Clusters: A Firm Level Study of French “Local Productive Systems”.” Regional Science and Urban Economics 41 (2): 108–123. doi: https://doi.org/10.1016/j.regsciurbeco.2010.09.001.
- Martin, R., and P. Sunley. 2003. “Deconstructing Clusters: Chaotic Concept or Policy Panacea?” Journal of Economic Geography 3 (1): 5–35. doi:https://doi.org/10.1093/jeg/3.1.5.
- McCann, B. T., and T. B. Folta. 2008. “Location Matters: Where We Have Been and Where We Might Go in Agglomeration Research.” Journal of Management 34 (3): 532–565. doi: https://doi.org/10.1177/0149206308316057.
- McCann, B. T., and T. B. Folta. 2011. “Performance Differentials within Geographic Clusters.” Journal of Business Venturing 26 (1): 104–123. doi: https://doi.org/10.1016/j.jbusvent.2009.04.004.
- McGahan, A. M., and B. S. Silverman. 2001. “How Does Innovative Activity Change as Industries Mature?” International Journal of Industrial Organization 19 (7): 1141–1160. doi: https://doi.org/10.1016/S0167-7187(01)00067-4.
- McNeish, D. M. 2014. “Analyzing Clustered Data with OLS Regression: The Effect of a Hierarchical Data Structure.” Multiple Linear Regression Viewpoints 40 (1): 11–16.
- Menzel, M.-P., and D. Fornahl. 2010. “Cluster Life Cycles--dimensions and Rationales of Cluster Evolution.” Industrial and Corporate Change 19 (1): 205–238. doi: https://doi.org/10.1093/icc/dtp036.
- Miller, D. J. 2006. “Technological Diversity.” Related Diversification, and Firm Performance, Strategic Management Journal 27 (7): 601–619.
- Molina-Morales, F. X., and M. T. Martınez-Fernández. 2004. “How Much Difference Is There between Industrial District Firms? A Net Value Creation Approach.” Research Policy 33 (3): 473–486. doi: https://doi.org/10.1016/j.respol.2003.10.004.
- Moulton, B. R. 1990. “An Illustration of a Pitfall in Estimating the Effects of Aggregate Variables on Micro Units.” The Review of Economics and Statistics 72 (2): 334–338. doi: https://doi.org/10.2307/2109724.
- Myers, R. H. 1990. Classical and Modern Regression with Applications. 2nd edition ed. Boston: PWS-Kent. .
- Nathan, M., and H. Overman. 2013. “Agglomeration, Clusters, and Industrial Policy.” Oxford Review of Economic Policy 29 (2): 383–404. doi: https://doi.org/10.1093/oxrep/grt019.
- Neffke, F., M. Henning, R. Boschma, K.-J. Lundquist, and L.-O. Olander. 2011. “The Dynamics of Agglomeration Externalities along the Life Cycle of Industries.” Regional Studies 45 (1): 49–65. doi: https://doi.org/10.1080/00343401003596307.
- Newbert, S. L. 2007. “Empirical Research on the Resource-based View of the Firm: An Assessment and Suggestions for Future Research.” Strategic Management Journal 28 (2): 121–146. doi: https://doi.org/10.1002/smj.573.
- Nooteboom, B. 2000. Learning and Innovation in Organizations and Economies. Oxford: Oxford University Press.
- OECD. 2002. Frascati Manual: Proposed Standard Practice for Surveys on Research and Experimental Development. Paris: OECD Publications Service.
- Porter, M. E. 1980. Competitive Strategy: Techniques for Analyzing Industries and Competitors. New York: Free Press.
- Porter, M. E. 1990. The Competitive Advantage of Nations. London: Macmillan.
- Porter, M. E. 1998. “Clusters and the New Economics of Competitiveness.” Harvard Business Review 76 (6): 77–90.
- Pouder, R., and C. H. St. John. 1996. “Hot Spots and Blind Spots: Geographical Clusters of Firms and Innovation.” The Academy of Management Review 21 (4): 1192–1225. doi: https://doi.org/10.5465/amr.1996.9704071867.
- Raffo, J. 2017. MATCHIT: Stata Module to Match Two Datasets Based on Similar Text Patterns. Statistical Software Components, Boston College Department of Economics.
- Raffo, J., and S. Lhuillery. 2009. “How to Play the “Names Game”: Patent Retrieval Comparing Different Heuristics.” Research Policy 38 (10): 1617–1627. doi: https://doi.org/10.1016/j.respol.2009.08.001.
- Research Explorer (2018): “Research Explorer - the German Research Directory”, available under ,lastretrieved:10032018
- Rigby, D. L., and W. M. Brown. 2015. “Who Benefits from Agglomeration?” Regional Studies 49 (1): 28–43. doi: https://doi.org/10.1080/00343404.2012.753141.
- Roesler, C., and T. Broekel. 2017. “The Role of Universities in a network of Subsidized R&D collaboration: The Case of the Biotechnology-industry in Germany.” Review of Regional Research 37 (2): 135–160. doi: https://doi.org/10.1007/s10037-017-0118-7.
- Rosenberg, N. 1990. “Why Do Firms Do Basic Research (With Their Own Money)?” Research Policy 19 (2): 165–174. doi: https://doi.org/10.1016/0048-7333(90)90046-9.
- Rosenthal, S. S., and W. C. Strange. 2003. “Geography, Industrial Organization, and Agglomeration.” The Review of Economics and Statistics 85 (2): 377–393. doi: https://doi.org/10.1162/003465303765299882.
- Šarić, S. 2012. Competitive Advantages through Clusters - an Empirical Study with Evidence from China. Wiesbaden, Germany: Springer Fachmedien.
- Scholl, T., and T. Brenner. 2016. “Detecting Spatial Clustering Using a Firm-Level Cluster Index.” Regional Studies 50 (6): 1054–1068. doi: https://doi.org/10.1080/00343404.2014.958456.
- Sedita, S. R., L. Lazzeretti, and A. Caloffi. 2012. “The Birth and the Rise of the Cluster Concept.”Paper presented at the DRUID 2012, Copenhagen (CBS), June 19-21.
- Shaver, J. M., and F. Flyer. 2000. “Agglomeration Economies, Firm Heterogeneity, and Foreign Direct Investment in the United States.” Strategic Management Journal 21 (12): 1175–1193. doi: https://doi.org/10.1002/1097-0266(200012)21:12<1175::AID-SMJ139>3.0.CO;2-Q.
- Smit, M. J., M. A. Abreu, and H. L. de Groot. 2015. “Micro-evidence on the Determinants of Innovation in the Netherlands: The Relative Importance of Absorptive Capacity and Agglomeration Externalities.” Papers in Regional Science 94 (2): 249–272.
- Speldekamp, D., J. Knoben, and A. Saka-Helmhout. 2020. “Clusters and Firm-level Innovation: A Configurational Analysis of Agglomeration, Network and Institutional Advantages in European Aerospace.” Research Policy 49 (3). doi: https://doi.org/10.1016/j.respol.2020.103921.
- Steffen, C. (2012): “How Firms Profit from Acting in Networked Environments: Realising Competitive Advantages in Business Clusters”. A Resource-oriented Case Study Analysis of the German and Swiss Watch Industry, Schriftenreihe: Internationale Personal- und Strategieforschung.
- Steinberg, P. J., V. D. Procher, and D. Urbig. 2017. “Too Much or Too Little of R&D Offshoring: The Impact of Captive Offshoring and Contract Offshoring on Innovation Performance.” Research Policy 46 (10): 1810–1823. doi: https://doi.org/10.1016/j.respol.2017.08.008.
- Stern, D. I. 2010. “Between Estimates of the Emissions-income Elasticity.” Ecological Economics 69 (11): 2173–2182. doi: https://doi.org/10.1016/j.ecolecon.2010.06.024.
- Sternberg, R., and T. Litzenberger. 2004. “Regional Clusters in Germany--their Geography and Their Relevance for Entrepreneurial Activities.” European Planning Studies 12 (6): 767–791. doi: https://doi.org/10.1080/0965431042000251855.
- Stevens, J. P. 2002. Applied Multivariate Statistics for the Social Sciences, Hillsdale. NJ: Erlbaum.
- Stifterverband (2018): “Research Data Center Wissenschaftsstatistik”, available under https://wwwstifterverbandorg/research_data_center,lastretrieved:10032018
- Suarez, F., and G. Lanzolla. 2005. “The Half-Truth of First-Mover Advantage.” Harvard Business Review 83 (4): 121-127.
- Suarez, F. F., and G. Lanzolla. 2007. “The Role of Environmental Dynamics in Building a First Mover Advantage Theory.” Academy of Management Review 32 (2): 377–392. doi: https://doi.org/10.5465/amr.2007.24349587.
- Tallman, S., M. Jenkins, N. Henry, and S. Pinch. 2004. “Knowledge, Clusters, and Competitive Advantage.” The Academy of Management Review 29 (2): 258–271. doi: https://doi.org/10.5465/amr.2004.12736089.
- Terstriep, J., and C. Lüthje. 2018. “Innovation, Knowledge and Relations – On the Role of Clusters for Firms’ Innovativeness.” European Planning Studies 26 (11): 2167–2199. doi: https://doi.org/10.1080/09654313.2018.1530152.
- Tödtling, F., P. Lehner, and M. Trippl. 2006. “Innovation in Knowledge Intensive Industries: The Nature and Geography of Knowledge Links.” European Planning Studies 14 (8): 1035–1058. doi: https://doi.org/10.1080/09654310600852365.
- Uzzi, B. 1997. “Social Structure and Competition in Interfirm Networks: The Paradox of Embeddedness.” Administrative Science Quarterly 42 (1): 35–67. doi: https://doi.org/10.2307/2393808.
- Van Oort, F. G., M. J. Burger, J. Knoben, and O. Raspe. 2012. “Multilevel Approaches and the Firm-Agglomeration Ambiguity in Economic Growth Studies.” Journal of Economic Surveys 26 (3): 468–491. doi: https://doi.org/10.1111/j.1467-6419.2012.00723.x.
- Vega-Jurado, J., A. Gutiérrez-Gracia, I. Fernández-de-Lucio, and L. Manjarrés-Henríquez. 2008. “The Effect of External and Internal Factors on Firms’ Product Innovation.” Research Policy 37 (4): 616–632. doi: https://doi.org/10.1016/j.respol.2008.01.001.
- Verhoeven, D., J. Bakker, and R. Veugelers. 2016. “Measuring Technological Novelty with Patent-based Indicators.” Research Policy 45 (3): 707–723. doi: https://doi.org/10.1016/j.respol.2015.11.010.
- Vicente, J. 2017. “Network Failures and Policy Challenge along the Life Cycle of Clusters.” In The Life Cycle of Clusters: A Policy Perspective, edited by D. Fornahl and R. Hassink, 56–75, Cheltenham, UK: Edward Elgar Publishing.
- Weber, K. M., and H. Rohracher. 2012. “Legitimizing Research, Technology and Innovation Policies for Transformative Change: Combining Insights from Innovation Systems and Multi-level Perspective in a Comprehensive ‘Failures’ Framework.” Research Policy 41 (6): 1037–1047. doi: https://doi.org/10.1016/j.respol.2011.10.015.
- Wu, X., S. Geng, J. Li, and W. Zhang. 2010. “Shared Resources and Competitive Advantage in Clustered Firms: The Missing Link.” European Planning Studies 18 (9): 1391–1410. doi: https://doi.org/10.1080/09654313.2010.492578.
- Zaheer, A., and V. P. George. 2004. “Reach Out or Reach Within? Performance Implications of Alliances and Location in Biotechnology.” Managerial and Decision Economics 25 (67): 437–452. doi: https://doi.org/10.1002/mde.1200.
- Zahra, S. A., and W. C. Bogner. 1999. “Technology Strategy and Software New ventures’ Performance.” Journal of Business Venturing 15 (2): 135–173. doi: https://doi.org/10.1016/S0883-9026(98)00009-3.
- Zenker, A., L. Delponte, N. D. Mayán, R. Wintjes, C. Amichetti, J. Carneiro, M. Meyborg, A. Notten, E. Schnabl, and T. Stahlecker (2019): “Cluster Programmes in Europe and Beyond, European Observatory for Clusters and Industrial Change”, available under https://wwwclustercollaborationeu/eu-initiatives/european-cluster-observatory,lastretrieved:17072019
Appendix
Table A1. Summary of main results (without interaction effects)