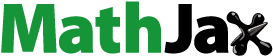
ABSTRACT
This paper examines the evolution of niches in creative industries, specifically the music industry. We conceptualise niche evolution as a manifestation of Schumpeterian disruption, and the music scene as a representation of a creative niche. Through mixed methods, we analyse niche evolution in collaboration networks over sixty years. We show that niche evolution exhibits recombination and reinforcement of new ideas, and propose that niche emergence and evolution in the music industry can be categorised as following three different pathways: seed fragmentation networks with early recombination and intermediate-stage reinforcement, often resulting from break-ups of highly influential bands; creative horizontal networks with intermediate-stage recombination and reinforcement, consisting of tightly knit communities with delayed commercial breakthroughs; and artist experimentation networks with late recombination and reinforcement, consisting of small niches of sub-genre innovations. This paper opens up new research directions for niche evolution, which can advance understanding of knowledge-intensive innovation ecosystems in other sectors.
1. Introduction
This paper examines the emergence and subsequent evolution of niches in creative industries, specifically the global music industry. Creative industries are composed of various niches that have a defined identity, audience and expertise, that often combine creative and economic forces. We propose that creative niches require a critical threshold of interactions in order to form. Rather than measuring relative ‘success’ or ‘performance’ of niches, we consider that niches may be defined as the interactions of collaborating agents. By regarding these interactions in the form of a network, we demonstrate how niches emerge and evolve via the combination or recombination of existing ideas and concepts, which are subsequently reinforced through the participation of new entrants; a pattern that has been inferred in previous research (Hermans et al. Citation2013) but not explicitly or empirically shown. Put another way, we examine the mechanisms within a collaboration network that lead to the generation and continuation of variety within a system leading to niche creation. Given this premise, we ask whether different patterns of niche emergence and growth are due to the underlying nature of its inherent properties; which may include resources, means of production, initial conditions, subsequent events and genre.
The study of niche emergence is not new, and prior studies have addressed this area from a number of scientific standpoints (Frenken, Saviotti, and Trommetter Citation1999; Oliver, Marwell, and Teixeira Citation1985; Gould Citation1993; Kim and Bearman Citation1997; Crossley Citation2009). These streams of prior research are unified in that they argue that a certain critical mass is required for niches to come into existence. Furthermore, music niches in particular have been shown to establish themselves via the formation of interaction networks among a few key social actors with a defined geographical area (Crossley Citation2009). The intrinsic value offered by creative industries is subjective, often non-financial in nature. We thus view and analyse creative industries via a scope of experimentation, collaboration and production alone, through the lens of the artist who has intimate knowledge of her market creative space. Creative industries may be linked to the social process of the generation of variety (Potts Citation2009). Therefore, we have chosen to focus on variety generation. Although we recognise that market selection is important, this is not studied here, as niches in creative industries require interactions of collaborating agents who are in the process of such experimentation. Hence, we recognise variety generation as the key evolutionary process inherent in such industries, and that new niches are expressions of novel variety. In this paper, we focus upon variety generation as captured by later-stage processes of niche emergence.
This paper uses the music industry as the subject of analysis, a creative industry where artistry and originality are important for not only financial success, but also brand status and insidership among participating parties (Bridson et al. Citation2017). The music industry exhibits a high degree of collaboration between different actors, and it is high-collaboration creativity which is the main focus of this paper. Furthermore, compared to other creative industries (e.g. the video game industry), this collaboration is a highly experimental type of collaboration, an experimentation potentially exacerbated as a result of recent industry shifts to streaming services where it has become difficult to secure pecuniary value. It is an industry that enjoys fluid labour mobility, lower costs and fewer (but not absent) institutional barriers such as intellectual property rights. The multi-role (guitar, vocals, sound design, etc.) aspect to music production further highlights the industry’s collaborative nature. Here, we focus on network nodes that have a purely creative aspect in terms of collaboration.Footnote1 We also take the perspective that the network patterns inherent to this sector may be applied to creative industries in general, as well as a wider Schumpeterian framework that includes entrepreneurs, firms, and non-market agents in a knowledge-intensive innovation ecosystem (Gifford, McKelvey, and Saemundsson Citation2021). These models may be applicable to other sectors. We choose the music industry thanks to its relatively experimental nature, which we believe better represents knowledge networks in a creative industry.
Given that we are aware of the salient mechanisms behind such interaction networks, we address the literature’s research gaps in the following ways. Firstly, by identifying and mapping creative knowledge networks and providing greater, measurable insight into those of creative industries, and in doing so, gaining a deeper understanding of its collaborative dynamics. Secondly, we gain further awareness on not only the mechanisms and properties behind niche emergence, but also broaden our understanding on what conditions influence their evolutionary trajectory. Finally, we separate the market from the purely creative search process in order to observe variety generation in a purer sense.
Evolutionary systems are one of change, and our conceptualisation is that new knowledge in the form of innovation is the basis of that change. We thus map collaboration networks that capture the flow of ideas via linkages between musical artists and groups. We draw upon the MusicBrainz.org database, which contains information on 1.1 million entries for artists, groups, producers and any individual relevant to the production and performance of a musical release. By using a cleaned version of this database, we are able to derive a network that describes collaboration across musical groups and performers globally over 60 years. This collaboration network is compiled every year from 1960 to 2019. Given this assembled network we identify niches by distinguishing sections of the network where nodes exhibit a pattern of belonging to tightly-knit groups. We then ‘wind back time’ to analyse the evolutionary properties of these niches, in particular their recombinant attributes as well as their reinforcing behaviour, in order to address our research questions.
This paper is structured as follows. In the next section, we develop our conceptualisation based upon a theoretical overview of niche creation, variety generation, knowledge networks and system transitions; and we describe these concepts within the context of evolutionary systems and creative industries. Section 3 introduces the data and describes the topology of the knowledge network used in this study, as well as the assumptions behind its nodes and linkages. Section 4 gives an overview and order of the methods used in this paper; including those that pertain to niche identification, recombination and reinforcement; along with a description of the qualitative analysis. The results are given in Section 5, followed by a final section concluding with a discussion of these results and the implications of our findings.
2. Theoretical background
Creative industries are widely seen as challenging to assess in terms of performance outputs. This presents an interesting opportunity to study the generation of variety within such industries, where there is a commercial component of value in the product or service offered and where variety generation occurs unbounded over time. A common way to frame a creative industry is one in which substantial artistic or creative elements are present in the product or service offering (Caves Citation2000). We consider the music industry to be one such industry, and draw upon several aspects of the literature on creative industries, specifically entrepreneurship in creative industries, in order to frame our investigation. The entrepreneurial character of the music industry is visible in the way in which many of the artists creating music are in effect small business owners marketing their own brand, relying heavily on self-management and network capability (Bridgstock Citation2013). Of course, also existing within this sector are the ‘titans’, or so-called ‘superstars’ (Giles Citation2006): the high-profile bands and artists that have built a massive business often spanning multiple countries or continents, whose tours and music sales generate millions. Here, we forgo analysis of pecuniary or financial returns in creative industries to look more closely at how linkages between artists impact niche emergence and evolution.
We thus draw on recent literature within an evolutionary tradition investigating the phenomena surrounding knowledge-intensive entrepreneurship (Malerba and McKelvey Citation2020). This literature combines Schumpeterian perspectives with those of innovation systems, organisational ecology and evolutionary economics to analyse a subset of entrepreneurial activity that is based on novel scientific, technological, business or creative knowledge which benefits the economy at large, and has high potential to transform society for the better. This stream focuses on how new types of knowledge are used and applied by firms for competitive gains. This literature is useful since creative industries figure prominently within its boundaries, and some studies have been carried out with the aim of analysing the knowledge-intensity inherent in creative industries, as well as how the use of new knowledge in these industries leads to commercial and societal gain (Lassen, McKelvey, and Ljungberg Citation2018; Gustafsson Citation2019). Research of Knowledge-Intensive Enterprises (KIEs) has a strong emphasis on the specific knowledge of entrepreneurs. We therefore consider band members as the equivalent to a collection of entrepreneurs or a founding team, and that the knowledge they create, use and apply, and how they combine this knowledge with others artists results in their artistic output in the form of musical releases. Using this Schumpeterian and system-based perspective, we view artists and bands as part of a wider system, where new knowledge, through networks, gives rise to new niches over time.
Hence, by combining these theoretical insights, we aim to study the mechanisms within a network that lead to niche creation and subsequent evolution. Below, we first review the literature on the conceptualisation and creation of the niche. We then turn to the idea of knowledge networks and outline how niches may be identified and measured by capturing the knowledge linkages that connect creative entities. Knowledge networks are then used to describe the system transition of niche emergence via the recombination and reinforcement of new ideas. Finally, we discuss variety generation in a more general context applicable to creative industries or knowledge-intensive sectors that exhibit evolutionary change.
2.1. Niche creation and variety generation
2.1.1. The concept of the niche
The notion of ‘niche’ in the social sciences can take on different meanings, interpretations and implications, which vary with theoretical approach.Footnote2 Geroski (Citation2001) provides an overview of these interpretations, which are roughly divided into two main camps; that of organisational ecology, and of economics. For organisational ecologists (Hannan and Freeman Citation1977), a ‘niche’ is a collection of resources that sustains a population (a population, in-turn, is a collection of organisations that have a common reliance on a set of resources). ‘Niche width’ is defined as the breadth of resources needed to sustain a population, and ‘niche overlap’ is where two populations compete for a similar set of resources. Thus, organisational ecologists consider populations as having their own niche, and are mostly concerned with width and overlap with other niches. This perspective is frequently shared by management scholars, as they often distinguish a firm’s market from other, competing firms.
For economists (Winter Citation1990; Boone and Van Witteloostuijn Citation1995), on the other hand, niches equate to markets, which are platforms that host a number of firms, who in-turn may or may not compete. Conversely, a firm may pursue multiple markets. Thus, the number and size of firms is endogenous, operating on an exogenous market. However, if we were to place this perspective within the prism of organisational ecology, the separation between endogeneity (i.e. population) and exogeneity (i.e. niche) becomes blurry. Furthermore, within economics, markets tend to be defined by demand, and thus identify market boundaries by estimating cross-elasticities (Geroski Citation2001). This presents another duality with the organisational ecology framework as market participants may be both producers as well as consumers. Thus, one would need to consider the supply-side as well as the demand-side when considering the definition of niche that satisfies both perspectives.
This paper brings together these two perspectives by taking a more evolutionary angle. We view agents (in our case, artists) as ‘seekers’ on a fitness landscape (Wright Citation1932; Kauffman and Levin Citation1987), an evaluation of the fitness function that has been applied in a variety of fields including the social sciences. This ‘rugged’ landscape, with peaks and valleys, measures the amenity, quality, merit or distinction of the musical output of artists, who occupy the fitness landscape in a perpetual search process for higher peaks. Artists have imperfect information of the fitness landscape, they are ‘short-sighted’, with better information of their local surroundings (facilitated by their own intimate knowledge of their musical speciality and creative space). Peaks on the fitness landscape represent an appreciation of what works and what does not. They may represent a dominant design or a saturation of endowment in this respect. The formation of a niche is not the realisation of a landscape ‘peak’. Rather, it is the outcome of exploration itself. A niche is a result of a collaborative search process, culminating in a critical mass, a realisation of what works. This realisation is never final but in flux, as variety is continuously introduced by other artists adding to a design with their own creative input.
We thus view collaboration in the creation of music as a search process. The artefact or outcome of the search process is a recording, deemed by its creators and associated actors (e.g. producers), as having sufficient value or quality, and thus eligible for release. This eligibility represents a point of accession on the fitness landscape. A release, which is the culmination of past collaborations and influences, is the representation of the fitness landscape. Thus, sales are not the type of market demand we are interested in.Footnote3 Here, we are more concerned with the collaborations involved when moving into a new creative arena. Artists, despite having imperfect information of the fitness landscape, have better knowledge of the fitness landscape compared to those analysing it. We do not have information of the fitness landscape, but we can see the realisation of that fitness landscape, as ‘found’ and explored by artists. Artist collaboration gives way to resulting knowledge linkages that are an aggregation of experimental actions. We study the behaviour of this experimentation, with the view that agents do so while exploring their fitness landscapes.
We thus take an abstract view that niches originate from new ideas, which in-turn are recombinations of existing ideas. A successful new idea provides the basis of a niche growing around it, and can thus be viewed as the ‘seed of a niche’. Innovations within a niche are those that are accepted by a market (Savino, Messeni, and Albino Citation2017). The search process occurs at the artist-level, who explore existing ideas and recombinations (Arthur Citation2007; Fleming Citation2001; Simon Citation1978). Innovators then harness their knowledge and skills to find the best possible new combination (Nelson and Winter Citation1982). In our model, this initial search space is the past collaborations of bands and artists; and the knowledge and experiences gained from such collaborations give rise to a cognitive map of ideas which the artist may exploit. This is not simply a passive historical consequence. Highly creative artists may actively seek new collaborations to enhance her knowledge and skills (Simon Citation1983, Citation1996), which is a necessity in order to contribute to the field (Cohen and Levinthal Citation1990). Higher levels of creative contribution (which may be viewed as a radical innovation) require increasingly disparate knowledge sets to combine and recombine; which also has an effect of pushing the artist away from constrained and tired methods, perspectives and ideas (Campbell Citation1960; Fleming Citation2002). These disparate knowledge sets arise out of social, cognitive and geographic dimensions (Torre and Gilly Citation1999).
Thus, the explorative and collaborative actions of a set of artists is the niche. They create their market while also seeking dimensions of existing ones. This dynamic, experimental view of niche addresses the demand- and supply-side duality while also incorporating aspects of Schumpeterian disruption of existing ideas and processes (Debruyne and Reibstein Citation2005), as well as integrating the idea that a niche may represent a complementary market for existing niches (Singh and Prasad Citation2014). This idea of niche emergence is also allied with the various standpoints identified by Coles, Piterou, and Sentić (Citation2018), including the economics of science and policy (Frenken, Saviotti, and Trommetter Citation1999) as well as collective action (Oliver, Marwell, and Teixeira Citation1985; Gould Citation1993; Kim and Bearman Citation1997; Crossley Citation2009), which are united in their view that some degree of critical mass is required for a niche to be created. Given the nature of idea exchange, a geographic aspect may be involved (Foray Citation2014; Komninos et al. Citation2014).
The idea of niche creation is easily relatable in creative industries, in which artistry and creativity are often crucial for not only economic success, but maintaining brand status and insidership within a group of like-minded firms, entrepreneurs, contractors or even customers (Bridson et al. Citation2017). The phenomenon has been conceptualised in a variety of ways, including ‘art worlds’ (Becker Citation1976), artistic fields (Bourdieu Citation1993) and the idea of the sceneFootnote4 (Straw Citation1991). While different niches may be inherently local, trans-local or even virtual (i.e. entirely web-based) (Bennett Citation2004), we incorporate all of these perspectives into our approach to the niche concept.
2.1.2. Variety generation
Our conceptualisation of niche is complimentary to the idea of variety generation. An established literature in evolutionary economics seeks to address the processes that underly dynamism and change in an economic system, with a special emphasis on a range of innovations being introduced (i.e. variety), as well as the processes by which these out-compete alternative solutions to economic problems, i.e. selection (Witt Citation1992; Metcalfe Citation1994; Hodgson Citation1996). We take inspiration from Frenken, Saviotti, and Trommetter (Citation1999), who discussed the intersection between the concept of variety in industrial activity with that of biology-based niche theory. Their paper explored four high-tech industries, and how and in what form niche theory could be applied, and their results indicated that changes in variety over time relate to the effects of a given innovation on increasing the range of services derived from a technology. This implies that when more complementary activities or services are necessary for the performance of an innovative product or service offering, variety generation seems to increase within that industry. We argue that the music industry is, at least in terms of the creativity and musical productivity of its artists, driven by such complementary activities in that there is constant cross-pollination of musical ideas across bands and artist networks, leading to continuous variety generation.
We thus draw upon this evolutionary framework in several ways. First, we view variety generation, an outcome of experimentation, as our key evolutionary process of interest rather than selection. The emergence of niche in a creative business area such as the music industry provides a fruitful opportunity to study the conditions needed for variation to occur and proliferate throughout a system. As the intrinsic value of creative offerings within music and art is subjective, there will nearly always be room for offshoots of style, technique, and form within art and music. This allows us to explore a creative industry in its ‘raw’ form, without the hindrance of institutional (e.g. patentsFootnote5) or organisational (e.g. labour mobility) barriers. Second, we investigate the degree to which variety is a product of network interactions, in-turn resulting in new musical niches characterised by combinations of genre and geography. We argue that continuous variety generation is highly likely in music niches, giving rise to further spin-off niches. Third, we explore the idea that certain evolutionary properties of a network are conductive to generating the critical mass required for these niches to emerge, spread, and become self-sustaining, mirroring the stream of literature on dominant design (Utterback and Abernathy Citation1975) and the industry lifecycle (Klepper Citation2002).
2.2. Knowledge networks
In our view, knowledge – and new knowledge in the form of innovation – is the basis of change within an evolutionary system (Metcalfe Citation2002). Given the above foci upon the dynamics of interactions needed for niche evolution, how the music scene relates to the concept of the niche and to idea recombination and reinforcement, we have chosen to study niches by focusing on knowledge networks.
Some streams of research have shown that knowledge networks exhibit a strong geographical characteristic, as the returns from such networks tend to display a powerful geographic constraint. Indeed, when one discusses creative niches, there is a strong local trait that defines them from other niches in the same genre. Maggioni and Uberti (Citation2011) identify two streams of literature on knowledge networks and how they relate to geography. The first of these, ‘putting networks into geography’, argues that the greater returns (innovation, creative output, etc.) in certain regions are due to the intentional (Breschi and Lissoni Citation2003; Møen Citation2005) and unintentional (Maggioni, Nosvelli, and Uberti Citation2007; Audretsch and Feldman Citation1996; Gersbach and Schmutzler Citation1999; Döring and Schnellenbach Citation2006) flows of knowledge that happen to exist within those regions. The second stream is ‘putting geography into networks’, which uses a gravity model approach to infer that closer geographic proximity results in a higher probability of linkages. This is closely associated with the literature on geographic proximity in industrial clusters (e.g. Boschma (Citation2005)) as well as that of homophily (e.g. McPherson, Smith-Lovin, and Cook (Citation2001)). Therefore, as knowledge is difficult to quantify, we may still map the flow of knowledge in the form of a network.
As previously reviewed, new knowledge arises from existing knowledge (Nelson and Winter Citation1982). Existing research on knowledge networks have focused on the diffusion and adoption of information and innovation (Becker Citation1970), the effect of social networks on individual creativity (Burt Citation2004; Perry-Smith Citation2006; Obstfeld Citation2005), and the production and diffusion of knowledge (Azoulay, Zivin, and Wang Citation2010; Jackson Citation2008). Rake, D’este, and McKelvey (Citation2021) explore knowledge networks in complex fields of science, by studying knowledge networks as self-organising systems, where homophily in research fields and heterophily in publications together promote the formation of ties to knowledge translators.
This paper does not adhere to either of the above streams but seeks to view both as one feedback loop of knowledge network formation and geographic constraints in order to capture and identify a music niche. Geography may act as a container, binding together agents with similar interests, specialisations, and talents. The size of such a container, however, may vary, with some occurring on a small, regional scale to large, global-scale dynamics. A network forms as a result, which in-turn reinforces the returns unique to a given geographic region, relating to previous literature on music scenes and its links to economic geography (Florida, Mellander, and Stolarick Citation2010). Rather than investigating geographic scale per se, we recognise the relevance of different local and global scales and its factor in determining the size and shape of knowledge networks.
3. Data and topology
3.1. The MusicBrainz database
The MusicBrainz project is a collaborative music database that collects information about performing artists, their performances and recorded works; and the relationships between these performing artists. The project is run by the MusicBrainz foundation, which is a non-profit organisation with the aim of keeping the database free and open source. The database is released to the public domain and continuously updated by a community of contributors, who also serve to approve new additions and edits (MusicBrainz Citation2019). As of September 2019, the database contains over 1.1 million entries for artists, groups, producers and any individual relevant to the production and performance of a musical release.
To arrive at the dataset used in this paper, we first separate musical groups (such as bands and collaborative ensembles) from individuals (who may or may not be part of a group). We disregard classical orchestras, choirs and tribute bands for the purpose of this paper, as such groups don’t reflect the same collaborative and creative nature of original music production. This leaves us with a dataset of 364,651 groups and 816,276 individuals.Footnote6 By definition, musical groups are made up of two or more individuals, and hence these two subsets of data overlap. Additionally, we arrive at a further subset of data regarding solo artists. We regard an artist as a solo artist if he or she has released a recorded work with no other individual.
3.2. Network topology and variables
Every network can be defined as having two components; nodes (or vertices) and links (or edges). In social network analysis, nodes tend to represent agents; which may be individuals, firms or organisations. The links therefore represent the relationships between those agents; and these can have a cognitive, social, or relational significance. In our case, nodes represent performers (whether an individual artist or a whole group), and links represent the creative and collaborative relationships between such actors.
Our network is based on two simple rules. First, if an individual performed in two or more groups, whether presently or historically, we signify this by establishing a link between those groups. Secondly, if a solo artist has also performed as part of a group (presently or historically), a link is established between the solo artist and the group. Thus, a node in the networkFootnote7 can represent an individual or group of individuals. These two relationships may be visualisedFootnote8 in .
Consider nodes G1 and G2 in , which denote two musical groups (i.e. bands). A link exists between G1 and G2 due to both bands sharing a common band member. Now, consider the occurrence where a band member leaves G2 to embark on a solo project. This individual, now regarded as a solo-artist, is depicted as A1, who establishes a link with G2. If A1 then joined another band, G3, this would result in not only a linkage between G3 and A1 but also G3 and G2 (due to these two bands sharing an artist). This results in a triad between A1, G2 and G3, an important structure that will be discussed later.
We may apply these network rules to a snapshot of the data to illustrate the network in an applied and illustrative manner. Footnote9 depicts the ego network of the Canadian post-rock band Godspeed You! Black Emperor. An ego network implies a focal node, with each other node described in relation to that focal node. Thus, the focal node is depicted in blue in . Nodes separated by one link (i.e. an order of one) from the focal node are depicted in green. Orange nodes have an order of two, and pale yellow nodes an order of three,Footnote10 only a few of which are labelled. The size of each node is a function of its degree, or the number of links adjacent (i.e. connected) to a given node.
The bands depicted in are predominantly post-rock bands from Montreal, Canada. This is a highly experimental musical genre whose main focus is upon texture and timbre, and draws upon many different genres and streams for new ideas and inspiration. A given musical track tends to be rather long, often in excess of 20 minutes, and is thus often compared to classical overtures in terms of structure and composition. Bands tend to be large and diverse, with multiple members simultaneously members of other bands with a different creative focus. reflects this, as the focal node has a large number of immediate neighbours (that is, first-order) that are also highly interconnected. This pattern continues for the second- and third-order nodes. It may already be observed that the geographic quality of the network begins to fall away at the city-level after the second order, with Broken Social Scene originating from Toronto, and Nive Nielsen & The Deer Children from Greenland. However, the bulk of this network is largely geographically intact at the country-level.
Our dataset contains variables specific to a given performer. The first of these is the year in which the group or solo artist became active. For groups, this year is explicitly recorded in the MusicBrainz database. For solo artists, we assign the starting year to the year of first release.Footnote11 All nodes in the network have an assigned founding year, and a network for any given year will contain all artists with a founding year
. Of note, we do not address a performer’s end year. This is due to the ambiguity of ‘year of exit’ in the music industry (artists may go on a hiatus, reunite after a break-up, etc.), as well as the nature of the network’s links. An ‘exited’ artist, in our view, may still provide creative influence upon future artists and collaborations, even if the original artist is no longer active. Thus, networks are cumulative year-on-year, with the network in year
effectively a subset of the network in year
. Founding year ranges from 1817 to 2019, with a mean of 1997 and a median of 2000.
A second node attribute is that of genre. The MusicBrainz database contains a set of tags pertaining to this characteristic, with additional data scraped from Spotify.Footnote12 A given performer may display multiple genres, and this is to be expected.Footnote13 We include all genres for a given performer for our analysis. This results in a potential 23 different genres for a given performer, although the vast majority (who do have a stated genre) display 5 or 6. A third node attribute is that of geography. Like genre, not all performers have a geographic attribute listed. Those who do, however, have only one, and this can take on varying levels of precision ranging from country (e.g. the United States), to larger administrative units (e.g. New York State), cities (New York City), or even a subregion within a city (e.g. Queens). Descriptive overviews for geography and genre (applicable to the final sample) may be found in respectively in the appendix.
The genre and geography attributes assist our work in identifying niches, but we do not rely on them. Niches are fundamentally identified using network analysis techniques, with genre and geography attributes acting as a means to refine our results. This process is discussed in the next section.
4. Method
4.1. Analytical design
We propose an order of analysis that begins with a full network in the year 2019 (the final year of observation) and then ‘wind back the clock’ to explore patterns of path dependence and initial conditions. We use the network’s giant component in 2019, which is the largest separate network that has reached a certain level of dynamic stability (Bollobás Citation2001; Kauffman Citation1996). Focussing on the network’s giant component has become a standard procedure in network analysis, and a necessary one as comparing the properties of separate networks is problematic (Bagley Citation2019a,Citationb; Borgatti Citation2006; Gulati, Sytch, and Tatarynowicz Citation2012; Davis, Yoo, and Baker Citation2003; Uzzi and Spiro Citation2005). The order of analysis (which does not follow the successive order of niche evolution), along with the measures used, is summarised in , and visualised in .
Using the network in 2019, we begin our analysis by identifying established scenes, which are subnetworks of nodes with common attributes. Then, by using networks of proceeding years, we go back in time to measure the nature of the beginnings of that subnetwork to identify a niche’s initial conditions. shows an example of the process of niche emergence and these initial conditions. Here, the recombination of ideas occurs first with a single performer. Later, new entrants build around that performer. Thus recombination occurs first, and reinforcement second.
shows just one example however, and other sequences are possible. A niche may, for instance, start off as a collection of tightly connected performers who then bridge into other niches, catalysing the start of its own niches’ growth. It is these contrasting sequences of conditions that we will explore in this paper, and how they relate to genre-specific and geographic properties of a given niche.
4.1.1. Post-emergence
The first step of analysis is that of the identification of niches that have already come into being. This is performed on the entire network on the final year of observation (i.e. 2019). Our aim here is to identify distinct, structural clustering on a local scale. To do this, we use the clustering coefficient, first introduced by Watts and Strogatz (Citation1998). The clustering coefficientFootnote14 of node is the proportion of
’s neighbours (i.e. nodes it is directly connected to) that are also connected to each other. In other words, it measures the degree to which a node’s neighbours belong to a clique. Formally, the clustering coefficient
may be expressedFootnote15 as:
where is the neighbourhood of node
,
is the number of nodes in that neighbourhood,
are the links between them, represented by
(where node
and
are nodes other that
). Thus, the maximum number of links in a neighbourhood may be expressed as
, which would result in a complete, perfectly connected neighbourhood of nodes. The clustering coefficient is thus expressed as a proportion, and varies between 0 and 1. A visualisation of the clustering coefficient may be seen in 9 in the appendix.
The clustering coefficient has a number of advantages in that it is a relatively simple measure to calculate (it’s measured on the node level, disregarding the entire network), and thus its less demanding in terms of computing power. It does pose a disadvantage in that it can ‘punish’ nodes located on the periphery of a clique (and thus less likely to have neighbours connected with each other). Indeed, other measures such as subgraph centrality (Estrada and Rodríguez-Velázquez Citation2005) or the label propagation algorithm (Raghavan, Albert, and Kumara Citation2007) may also be used to measure cliques. However, as we are concerned with the mere identification of niches (and therefore cliques) and less concerned with mapping the entire niche, while also considering the demands on computing power, the clustering coefficient is an ideal measure for our purposes.
Upon measuring the clustering coefficient for the entirety of the network in 2019, we then apply a series of thresholds to isolate cliques. The specific thresholds are chosen depending on the statistical distribution of the network. A clique is then considered a niche by applying a series of simple rules relating to specificity in genre and geography. These rules may be summarised as:
At least one genre level (supergenre or subgenre) is identified. That is, the mode of listed genres for artists in the clique.
At least one geographical level identified. That is, the mode of listed locations for artists in the clique.
For supergenres and geographical attributes on the country level, a variation ratio of less than 0.1.
This method, while stringent, may of course disregard some niches due to the incompleteness of genre and geography variables in the dataset. However, it is liberal to the extent that it does not exclude niches that may see a small variance in these variables. For example, an artist may be strongly connected to the Japanese Pop scene but located in San Francisco.
4.1.2. Recombination
Upon identification of our niches, we then seek out the set of initial conditions required for niche emergence. A niche’s recombinant properties take the form of a ‘seed’ that exhibits a certain level of differentiation from current varieties and niches, which later provides the basis of a new niche. The ‘seed’ displays a combinatorial aspect. In other words, a ‘seed’ may be a performer that finds itself situated and connected with two or more disparate incumbent niches, which infers an influence of those two or more niches to form a novel musical form.
This may be illustrated in in the appendix. Here, node may be seen as a ‘bridge-maker’ between three separate cliques of nodes. These cliques we may define as existing niches. Note that it would not be accurate to describe node
as a member of any clique specifically. Node
does, however, enjoy connectivity with (and therefore influence from) all three niches. It is from node
where a future niche may emerge. We measure node
’ s position in the network with betweenness centrality, a measure originally proposed by Freeman (Citation1977). The betweenness centrality of node i may formally express as:
where is the shortest path between nodes
and
and
is the number of those paths that pass through node
. Betweenness centrality is often normalisedFootnote16 between 0 and 1, which makes different networks comparable (and which we do here). In a nutshell, Equationequation (2)
(2)
(2) describes to what extent node
acts as an intermediary, i.e. if a path exists between nodes
and
, to what extent does node
play a role in that path? Nodes that exhibit high betweenness centrality are not necessarily part of an existing clique. They do, however, display a high level of reach (via short paths of links) with existing cliques, compared to other nodes. The scale on denotes betweenness centrality. It may be seen here that node
exhibits the highest betweenness centrality in the network as it acts as a strong intermediary of all three cliques. Thus, when ‘winding back the clock’ of an existing niche, it is anticipated that one would eventually arrive at one node with a relatively high betweenness centrality score. There is no specific ‘threshold’ for this score, but as a rule of thumb it is expected to be high relative to the node’s network neighbourhood.
4.1.3. Reinforcement
New ideas provide the basis for niche emergence and subsequent evolution, which in-turn relies on a number of conditions inherent to the participating agents. Hermans et al. (Citation2013) provide an outline of these conditions in a network analysis framework. These conditions are a) the convergence of shared visions; b) learning and experimentation; and c) the formation of social networks.Footnote17 Niches may qualitatively differ due to the underlying nature and characteristics of the participating artists, the features of the genre, as well as the role of central actors in some niches and self-organisation in others. Niches may arise organically on a local level to spread outward later, or may be part of a larger global movement that arises in unison. This in-turn may have an effect on measured network properties.
Thus, the reinforcement of new ideas lays down the foundation of niche emergence; and is associated with the convergence of shared visions, learning and experimentation, and the formation of social networks. Network density describes the level of connectivity of nodes within a given network. The measure, which ranges between 0 and 1, is simply a ratio of the number of links to the number of potential links. A network with every node connected to every other node would thus have a density equal to one. Hence, density is a global (rather than a nodal) measure. Formally we may express density () as:
Hermans et al. (Citation2013) use density to measure the convergence of shared visions, learning and experimentation, as well as, of course, the formation of social networks. Higher collaboration between nodes is marked by an increase in network density, and new nodes in an emerging niche are associated with a proportional increase in links, especially if it is the result of incumbent band members embarking on new projects. It should be noted that for the formation of social networks, density may reflect linkages seen beyond those that are formally mapped in our model. A link between two artists or groups may be the culmination of networking with intermediaries that bring groups together such as producers, agents or any actor embedded within the niche.
5. Results
5.1. Identified niches
We begin with a network of 71,687 nodes and 108,656 links, assembled according to our topology rules. Isolated nodes, that is, nodes with no links, are removed from analysis. The resulting network constitutes the relationship of artists and groups in September 2019. Using a clustering coefficient () of 1/3, we identify an initial 124 niches. This is a relatively low threshold, and results in a skewed size distribution with the largest niche comprising 5006 nodes. This large niche, on closer examination, was comprised of a large patchwork of multiple music genres and geographic locales. This size distribution is not surprising, as our network displays a power law with respect to nodes and links. To strive for a sample where niches are no larger than 100 nodes but no smaller than 20, we reapply the process on the largest niche by increasing the threshold of
to 1/2. We repeat this process (with a
of 2/3) on the largest component of the last round.Footnote18 After applying the geography and genre rules we arrive at a sample of 47 niches, summarised in in the appendix.
5.2. Betweenness and density
For any given node, betweenness () may change with time as a niche’s network evolves. Thus we observe the first non-zero (or non-null) value of each node’s
from the year they enter existence. The goal is to identify early bridge-makers rather than those that may establish linkages with other niches (either new or existing) later into its own niche’s evolutionary trajectory. Upon casual scrutiny, we observe that
is zero for most nodes, with the exception of a few noticeable anomalies. As hypothesised, it is these anomalies that are of interest as these exceptional nodes act as the recombinant ‘seed’. Furthermore, we note that these anomalies appear early in niche development for many niches, but later in others. There is an observed pattern of three separate clusters or groupings of niches, which we further confirm using a k-means clustering analysis (Steinhaus Citation1957; MacQueen Citation1967). This employs a heuristic algorithm that partitions observations into k clusters where each observation occupies the cluster with the nearest mean. The plot of the k-means clustering analysis may be seen in .
plots the first observed non-zero betweenness for nodes in all nichesFootnote19 against time . The smaller symbols represent these plotted betweenness scores, while the larger symbols their within-group averages. We choose 3 clusters based on observed patterns discerned from the initial plots,Footnote20 and find that there is a clear separation between these groups. Niches in group 1 (in red triangles in ) display the earliest (and, on average, smallest) spikes in betweenness amongst its nodes, which conforms to our basic hypothesis that niches begin in such a manner. Groups 2 and 3 (labelled in black circles and green crosses in respectively) show later (and, on average, larger) spikes in
, which may indicate a different set of niche network dynamics, which in-turn may be influenced by genre-specific characteristics. We turn to network density to further discern these network dynamics. shows the density for each niche’s network, over time, split between the three groups identified in the k-means analysis.
The different coloured lines (black and grey) in are to discern between two different patterns in density. The grey lines denote niches that begin small yet connected. A niche may, for example, begin with two nodes connected to each other, giving the network a density . Over time density collapses as new nodes join the network, without a compensating number of linkages to all other nodes. Thus, we separate these niches away from our analysis in discerning the different patterns between the three groups. The lines in black show a different dynamic. Niches begin unconnected, or with an isolated node. Over time the niche network becomes progressively more connected and density increases. Of note, density increases before falling and maintaining at a stable level. Furthermore, this peak changes depending on the group of niches considered.
The three identified patterns of niche formation serve to further our discussion of the dynamics of creative industries, with a specific focus on the music industry, given our dataset. It must be stressed, however, that these three patterns are not inferred to be universal, but serve to guide our evolutionary discourse. The identified patterns are open to further research and discussion. In the case of this paper, these groupings serve to aid our understanding of creative niches on the qualitative level.
5.3. Qualitative analysis
Our qualitative analysis considers the three groups that transpired from the quantitative analysis, and explores the bands and artists present in each within the context of the revealed niche. This process involved an initial exploration of each identified niche, by the gathering and analysis of secondary data available via the Internet concerning the artists, bands, and music scenes contained within each niche. This was carried out by returning to the CitationMusicbrainz database for further analysis; the use of Wikipedia pages of bands in a variety of languagesFootnote21; utilising other archival ‘completionist’ or collector-run sites for music genres such as Metal-Archives Citation2019for metal bands or Progarchives Citation2019for progressive rock bands; general Google searches for album and artist pages; and Spotify for further analysis of band profiles.
We then compared these qualitative findings with the quantitative results in order to arrive at a deeper level of analysis. To gain a more intricate detail of inter-niche dynamics, we then sampled one niche per group in order to illustrate the general group properties. This was a largely purposive sampling strategy, based on the availability of secondary (Internet-based) data about the bands and artists in the niche; an assessment of the representativeness of the niches for the overall tendencies of the group as revealed by the cluster analysis. Finally, the authors’ own familiarity with the niche in question was relied upon. Below, we discuss each major group that was revealed by the cluster analysis, and explore an example for each niche group.
5.3.1. Type 1 – Seed fragmentation network (early recombination, intermediate-stage reinforcement)
This group of niches may be characterised by wide geographic dispersion. Within some of these identified niches, collaborations centred around record labels dominate the network (mainly those focused on electronic music). Furthermore, some niches resulted from the breaking up of a larger (and popular) band that was considered innovative or otherwise influential in style, producing high levels of betweenness centrality early on. Thus, we may characterise such niche networks as one of seed fragmentation, i.e, an initial, influential seed breaking up to form a niche from its remnants. In many of these cases, the new projects spawned are genre-defining and more experimental within a given genre. This can mean a more successful band acted as a bridge-maker in the niche’s early years (UK Psychedelic Rock, Melbourne New Wave, and Argentinian Progressive Rock, to name a few). In electronic music genres, it is apparent that the network expanded quite rapidly in many cases, but slowed down after initial bursts of collaboration. Overall, early betweenness and early high density can be accounted for by the early commercial success of central artists, and an organised marketing push through music producers and record labels, or a more successful band breaking up and creating spinoff projects and solo artists. We also observe here that there may be differing mechanisms of variety generation and continuation for instrument-based versus electronic-based niches. Artists and bands in niches within Type 1 are listed in in the appendix. These are niches with nodes that exhibit early high scores for betweenness centrality, accompanied by increases in niche network density (with peaks at ).
5.3.1.1. Illustration: UK Psychedelic and Acid Rock
This niche, which may be visualised in 6, captures fairly spread out collaborations centred around London, UK, and Dublin, Ireland; as well as other areas of the United Kingdom (Bath, Dolton, Surrey) and New York/Canada. The major ‘event’ in this niche was the break-up of noteworthy London-based hard rock pioneers Cream. This iconic ‘power trio’ consisted of Eric Clapton, Jack Bruce, and Ginger Baker. With an extremely successful run of albums in the late 1960s, Cream’s break-up spawned a wealth of collaborations, and cross-pollinations of different UK bands as well as some spinoffs in North America, most notably Mountain, featuring Leslie West on guitar and Corky Laing on drums. Mountain was discovered by, and their debut was produced by, Cream’s former producer Felix Pappalardi. The niche captures bands that were active around the time of Cream and even before in the mid-1960s, including a string of collaborations that led up to the formation of Tears for Fears in the 1980s, a pop rock band that achieved wide commercial success. Additionally, several bands formed out of Cream’s ashes, such as Blind Faith, which featured Steve Winwood and Ric Grech (of Traffic), and Jack Bruce’s work with Gary Moore (of Irish band Thin Lizzy and its precursor Skid Row), leading to an impressive network of musicians collaborating off and on for several decades after the psychedelic rock heyday of the 1960s and 70s. Moreover, ventures into the jazz genre transpired via bands and projects by Ginger Baker and Jack Bruce of Cream origin, along with drummer Charlie Watts of the Rolling Stones. Overall this niche captures more of a collaborative web than genre creation, although bands like Cream and Mountain were certainly influential in the evolution of rock and heavy metal over the following decades. This niche shows that the break-up of a widely influential and culturally significant band spawns multiple new projects, activities and creative partnerships that may last decades.
Figure 6. An early recombination, intermediate-stage reinforcement (group 1) niche: UK Psychedelic and Acid Rock.
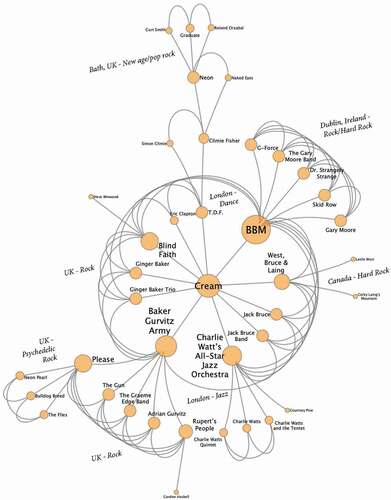
While Cream itself was an incredibly influential band in the rock genre, and their influence was certainly variety generating, this niche captures more of ‘what happened after’ their break-up. While many artists became quite popular (Eric Clapton arguably became more famous than Cream), new genre creation is difficult to track. What we can find is an evolution of musical styles through interaction of individual artists and group formation. Psychedelic rock in the late 1960s led to Cream, whose members came from fairly well-known rock/blues ensembles at the time (Clapton, like many other famous British guitarists of his era, started in John Mayall’s Bluesbreakers). Cream took rock to new places, combining the early 60s rock sound, late 60s psychedelia, and their own band of riff-focused proto-heavy metal/hard rock. Their break-up set the stage for multiple ‘spinoff’ bands, either through sound engineers involved with Cream (i.e. Mountain), or the genre explorations of the members themselves (Baker and Bruce experimented with Jazz fusion, rock, and other styles, while Clapton collaborated with Dance Pop projects together with Simon Climie in T.D.F., who later worked with Curt Smith and Roland Orzabal of Tears for Fears). In this case, the Cream artists experienced widespread success that put them on the musical map, and thus following the break-up, they became well known and sought after musical partners for a variety of artists, building several new bands in the process.
Graphically, the betweenness of the niche has two main arcs, one during the 1970s following Cream’s break-up, and again in the 1980s and onward when the bands with Tears for Fears origins, Neon and Graduate, took off, as well as the later collaborations of the Cream-spinoffs. The density function ranges from moderate to low in the post-1990s. Much of this could be due to the age and thus declining activity of the artists and their collaborators. The size function is a steady concave curve, flattening out around 2003–2004 ( in the appendix).
5.3.2. Type 2 – Creative horizontal network (intermediate-stage recombination and reinforcement
These network types display longer term density building, and later betweenness than Type 1, though Types 1 and 2 are more closely related compared to Type 3. Within this group, we observed delayed, prolonged, or resurgent (via band reformation) commercial breakthroughs and collaboration spikes. In many of the Type 2 niches, we observe a resurgent process of reunions, reformation, and related activities. In others, we observe highly collaborative side projects of members of more recognised bands that gradually established themselves as a network over the medium term. We may thus describe these niches as creative horizontal networks, i.e. those that exhibit a somewhat constant mutual collaborative exchange of ideas without a defined central actor. There are tendencies for the niches in Type 2 to be more akin to what in the literature have been described as ‘scenes’ (Straw Citation1991) in that it becomes more of a long term, cultural collaborative space. These niches are more driven collectively by multiple artists striving for success as well as artistic fulfilment, resulting in longer time periods before maximum density is reached. A list of these intermediate-stage recombination and reinforcement niches may be seen in the appendix in .
5.3.2.1. Illustration: Chicago Skate and Pop Punk
The Chicago Skate and Pop Punk niche captures musical traditions spanning from the late 80s and early 90s to the present day. The niche’s network may be seen in . It accounts mainly for developments in the Chicagoland music scene, spilling over into nearby states Indiana (Lafayette), and more distant states such as California (Berkeley and the Bay Area, along with San Diego) and Wyoming (Laramie), North Carolina (Chapel Hill), Pennsylvania (Philadelphia) and Massachusetts (Boston), among others. The core of the niche can be traced back to the bands Screeching Weasel, formed in 1986, the Smoking Popes, formed in 1991, and Slapstick, formed in 1993 in Chicago. The former broke up and reformed several times since their inception, resulting in a wide and varied cast of supporting artists surrounding the core members. The latter was not in itself a widely popular band, but the bands that it spawned thereafter became mainstays of the Chicago punk and indie rock scene (often called the Slapstick family tree). This included bands like the Lawrence Arms and Alkaline Trio. Over time the scene became more diverse, with more hardcore, metalcore, and experimental post-rock/post-punk artists emerging out of the scene. This arguably culminated in the popular appeal of bands like Teenage Bottlerocket, and the even more popular Rise Against and Fall Out Boy, which rose from more metal and hardcore-inspired roots to become an extremely popular pop-punk band across the US and beyond. The niche also captures the West Coast scene that arguably began with ska-punk trailblazers Operation Ivy, whose spinoff band Rancid became widely influential. Side bands of the Operation Ivy members crossed over with Chicago artists, further linking together the two geographic areas of stylistically similar music. This link with Rancid also brings in Southern California pop-punk mainstays like Blink 182 and its surrounding side projects, thanks to collaborations between Rancid and Operation Ivy member Tim Armstrong, and Blink 182 drummer Travis Barker.
Figure 7. An intermediate-stage recombination and reinforcement (group 2) niche: Chicago skate and pop punk.
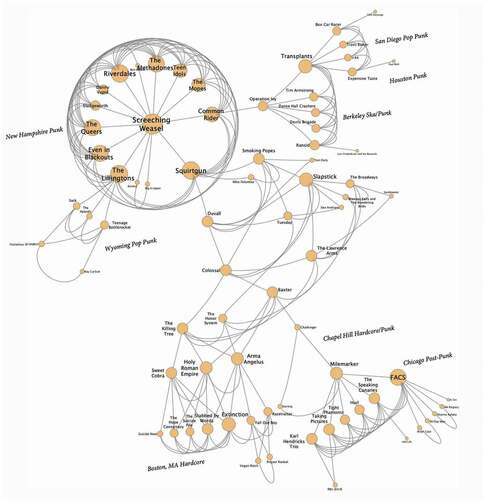
Overall, one can see that the niche began with quite low levels of betweenness centrality, spiking upwards around the years 2000–2010 (the popular appeal of Rise Against, Rancid, and later, Fall Out Boy, plus a resurgence of popularity of the core bands like Screeching Weasel (who reformed 1996–2001) and the Smoking Popes (who reformed in 2005)). The density of the niche was low in the 80s, then jumped substantially in the 90s with the rise to fame of the core bands, along with the materialisation of spinoffs as core bands broke up and reformed during the period. The density function likens to that of the logarithmic distribution with a large hump early on, followed by a long tailed decline over time, whereas the size function shows an S-shaped curve (, in the appendix).
In this example, we can trace variety generation through the continual and gradual stylistic evolution from early punk to pop-punk (1990s), from pop-punk to hardcore, crossover, and metalcore (2000s), and a resurgence of popularity of pop punk in the late 2000s and early 2010s.
5.3.3. Type 3 – Artistic experimentation network (late recombination and reinforcement)
This network type includes, to a large extent, niches that have stayed small, but often contributed to innovations within a given genre. Most of these niches focus around one to three individual artists, whose collaborations over a longer time period (for as much as 20 years) lead to maximum density and high betweenness at a later stage. The scene figureheads are often innovators within a style, but do not achieve widespread commercial breakthroughs. These are network types that exhibit a high degree of artistic experimentation. Examples include Detroit Electro (Belleville Three), Los Angeles Doom Metal (Scott Weinrich), and Japanese Noise Music (Acid Mothers Temple/Kawabata Makoto). Side projects spinning off from prominent individuals, rather than bands, are more common. A list of these niches may be found in (appendix).
5.3.3.1. Illustration: Detroit Electro
The Detroit Electro () niche spans from the early 80s until the present day. The main distinguishing factor of this niche is that it captures the creation of the electronic music subgenre Detroit Electro. This has arguably revolved around the musical releases and collaborations of several key figures in Detroit (and surrounding metropolitan and suburban areas). These are the so-called Belleville Three (Juan Atkins, Derrick May, and Kevin Saunderson), and the duo that (allegedly) formed the group Drexciya; Gerald Donald and James Stinson. The Belleville Three attended high school (in Belleville, Michigan) together, became fast friends, and started writing and producing music. Donald and Stinson have been enigmatically related to the Detroit scene, working often under aliases and distancing themselves from public limelight. The Detroit electro style was a combination, or re-interpretation, of trends in electronic music in the early 80s (including Chicago House) combined with European-based electronic music like Kraftwerk, with more industrial factory-inspired noise constituting major elements of the beats, with a detached yet dance-infused rhythm. Indeed, a few actors of this scene often collaborated on projects with German-based musicians and producers, and often adopted German aliases or German-language project/band names (Drexciya’s Gerald Donald recorded under the name Heinrich Mueller multiple times, in bands such as Der Zyklus, Zwischenwelt, and Gedankenexperiment). While mostly active during the 1980s and 1990s, the genre has crossed over into the modern music era, with artists like Santonio Echols collaborating with Kevin Saunderson as Reese & Santonio. Aktins, May and Saunderson now tour as the Belleville Three, a moniker given to them by the British press, but now fully adopted as a band name.
Graphically, the niche starts with a low level of betweenness centrality, with a jump in the mid-90s and a steady decline again towards 2010. The density measure falls in a manner that approximates a smoothed out tail not unlike the higher values of a log distribution. The size function is more a steady rise with a slight jump around the late 1990s than an S-curve (, in the appendix).
5.4. Niche network types – final remarks
Viewing all three network types’ formation over time, we find that differences stem from mechanisms with respect to idea recombination and reinforcement. Type 1 (seed fragmentation network) niches display early recombination and intermediate stage reinforcement. This can be characterised as the initial foundation of an influential central band, upon whose break-up or extended inactivity is followed by cross-pollination and rapid variety generation either within one scene, or spanning others as well. In this group, we find that early ‘bridge-makers’ in instrument-based scenes create a ‘buzz’ of high early betweenness measures. Here we suggest that while the overall pattern remains the same, there are likely different mechanisms driving the change, which in-turn depend on the underlying musical architecture captured in this niche. The most obvious mechanism in play can be characterised as more traditional, instrument-based production as compared to more electronic, studio production. On the one hand, influential instrument-based bands splitting up may lead to heavy periods of cross-genre pollination, though largely through individual artists and groups experimenting with established genres or fusions of genres across different music scenes (i.e. jazz fusion in the UK Psychedelic Rock example). On the other hand, electronically driven niches tend to exhibit more of a ‘label push’ phenomenon, where artists, producers, and other recording industry actors establish network ties leading to new, at times innovative, projects. Hence, under this scenario, the subsequent variety generation adhering to this niche may be considered as the result of either inherent experimentation or label-push.
Type 2 (creative horizontal network) niches display intermediate-stage recombination and reinforcement. This represents a more long-term and collaborative space than Type 1, with many artists and bands sharing roles within the scene in functions that fall outside performance (i.e. production, art stage design, and marketing activities). shows that this type of niche spreads geographically far, and linking into multiple music scenes of very similar genres. While relatively successful bands do emerge, they tend to do so quite late, as a result of an organic build-up of cross-collaborations within the niche. However, the timespan recombination and reinforcement of the niche is, on average, not as long as seen in Type 3 niches.
Finally, Type 3 (artistic experimentation network) niches represent late recombination and reinforcement. This type also constitutes a long-term collaborative space with characteristics of a bottom-up music scene (Crossley Citation2009), but often is more innovative or path breaking within a given stylistic genre. In our example, Detroit Electro, there is one conjoined music scene (Detroit electro with some German collaborations). This type of niche is very scaled down, and intensely collaborative between a few different artists over an extended period. The evolution can be seen as very gradual and drawn-out over many years, around a small core group of artists. Nonetheless, Type 3 shows artist-centric tendencies for experimentation that trace network elements of niche proliferation, and both geographic and stylistic crossovers.
6. Discussion and conclusion
This paper, by identifying and mapping measurable creative knowledge networks, has examined the emergence and evolution of niches in creative industries, specifically the music industry. We have explored niche dynamics through manifestations of creative collaboration, as captured by linkages between artists on recorded music releases using a large database over sixty years. In doing so, and by isolating the creative search process from the market, we have broadened our understanding of what conditions have influenced their evolutionary trajectory. We have shown that niche evolution exhibits both the recombination and reinforcement of new ideas, and specified three different pathways in the music industry, through mixed methods.
Based on the above empirical analysis, we propose that these three ideal types represent three different patterns of niche emergence and growth. We have identified such new combinations which may be driven either by top-down or bottom-up processes. As such we propose they are a manifestation of the underlying nature of the niche’s inherent properties, be that in terms of the means of production, initial conditions, genre, or subsequent structural events.
Specifically, top-down processes are visible in Type 1 (seed fragmentation network), with spinoffs emerging from successful bands and/or label and producer-led collaborations. In contrast, we identified bottom-up collaboration over longer time periods, either in terms of a wide stylistic and geographic distribution is seen in Type 2 (creative horizontal network), or a more localised and individual-centric dynamic in Type 3 (artistic experimentation network). Hence, our proposition is that the mechanism of variety generation is largely dependent on niche type.
Viewing all three network types’ formation over time, we find that differences stem from mechanisms with respect to idea recombination and reinforcement. Type 1 (seed fragmentation network) niches display early recombination and intermediate stage reinforcement. In this group, we find that early ‘bridge-makers’ in instrument-based scenes create a ‘buzz’ of high early betweenness measures. Here we suggest that while the overall pattern remains the same, there are likely different mechanisms driving the change which in-turn depend on the underlying musical architecture captured in this niche. The most obvious mechanism in play can be characterised as more traditional, instrument-based production as compared to more electronic, studio production.
Type 2 (creative horizontal network) niches display intermediate-stage recombination and reinforcement. This represents a more long-term and collaborative space than Type 1, with many artists and bands sharing roles within the scene in functions that fall outside performance (i.e. production, art stage design, and marketing activities). shows that this type of niche spreads geographically far, linking into multiple music scenes of very similar genre.
Finally, Type 3 (artistic experimentation network) niches represent late recombination and reinforcement. This type also constitutes a long-term collaborative space with characteristics of a bottom-up music scene (Crossley Citation2009), albeit more innovative or path breaking within a given stylistic genre. In our example, Detroit Electro, there is really one conjoined music scene (Detroit electro with some German collaborations). This type of niche is very scaled down, and intensely collaborative between a few different artists over an extended period.
We observe empirically that certain bands or artists dominate, or have central role, in the emergence and the evolution of niche. Those bands or artists may do one or all of the following: i.) they may set either a stylistic precedent to be followed, or expanded upon; ii.) they may act as an initial gathering of like-minded seekers whobriefly explore the landscape together before going their separate ways (but not without vastly influencing the landscape around them); iii.) they may reunite or rejoin one another on different musical ventures; iv.), they may build a closely knit community where they experiment with thematic variations of their style, never settling on any dominant design, and all the while influencing those around them. We find all these cases within our sampled niches: whether in the classic/psychedelic rock and proto-metal of the UK (centred around Cream), the pop-punk of Chicago (e.g. Slapstick, Screeching Weasel); or Detroit Electro (the Belleville Three). During the experimentation process, they act under uncertainty or short-sightedness, their work creates paths for further variety generation within a niche, in turn spurring on that niche’s evolution.
We relate the implications of our research to theories on knowledge-intensive entrepreneurship. In the first group, we find that different genre instrumentation (instrumental versus electronic music) exhibits slightly different tendencies in terms of drivers of niche evolution. From a KIE perspective, we argue that niches that are dominated by electronic music might be driven more by business knowledge of labels and producers, whereas niches with traditional instrumentation at their musical core might rely more on creative knowledge of artists. Moreover, we find that in our latter two niche types, artists and bands display an entrepreneurial character that is commonly attributed to creative industries, and exhibit characteristics commonly captured in studies of KIE (Lassen, McKelvey, and Ljungberg Citation2018), where ventures combine different varieties of competition-enhancing knowledge as they strive for both commercial and societal (or social) gains simultaneously. In any case, artists and bands utilise creative knowledge (e.g. stylistic and musical knowledge and skills) as well as business knowledge (many are more than just musicians), to build and expand their musical brand and/or identity. We propose that these knowledge varieties underlie the recombination and reinforcement patterns we identify in our niches and groups.
Given our above analysis, we find that after the emergence of a niche, niche evolution is a system transition that involves the recombination and reinforcement of ideas. While our technique has limitations in isolating the initial ‘spark’ of niche formation, in that we are unable to observe artists’ motivations in their musical direction outside of what they release commercially, we see a continuation of variety generation within the boundaries of the identified niches we analysed within the given time frame. In the example of the Detroit Electro niche, qualitative analysis reveals that its core artists were clearly inspired by a number of different sounds (i.e. early German electronic music and Chicago house music), but collaborations with these original artists are not forthcoming within their network or patterns observable in their released music. Hence, while we acknowledge that inspiration without collaboration is then a likely explanation for the formation of new genres of music, we argue that latter stage niche evolution is interesting in that multiple instances of new niche formation and proliferation may occur through the original niches’ network evolution, something that we do observe. The initial ‘spark’ or emergence of a niche may be endogenous or exogenous to a given niche’s network. Our findings suggest that this perhaps mainly occurs exogenously, but following this, a process of continuous variety generation proliferates.
We propose several avenues for further research. First, the idea of top-down as opposed to bottom-up niche emergence and evolution might constitute a mechanism that creative industries, specifically music industries, share with wider classifications of manufacturing and service firms, namely spinoff dynamics leading to new networks and collaborations. This in-turn leads to more economic experimentation and variety generation. This idea should be explored further.
Second, we find that electronic-based music niches such as EDM, House, Techno, and others, exhibit different properties from more instrument-based scenes (rock, jazz, etc.) in terms of how variety is generated within a collaborative network. We recommend further exploration of the similarities and differences between these two types of music performance and production.
Third, while we argue that networks are a means for musical creativity as well as business stability for many bands and artists, we do not assess economic success, or the more intangible cultural acceptance of a given niche, and how this in-turn affects niche emergence and evolution. Moreover, collaboration through networks may constitute a means of survival for many bands and artists, especially in more bottom-up driven niches like those found in Types 2 and 3. This is in-line with research findings regarding specialised knowledge usage and the presence of driving artistic elements in creative industries (Caves Citation2000), and should be explored further.
Fourth, novel network measures and tools, including computationally intensive algorithms for identifying communities and therefore niches could be applied for large networks in subsequent studies. Ultimately, we anticipate that the findings in this paper may be applied to other innovation-intensive sectors, using the theoretical underpinnings pertaining to creative industries applied to the knowledge-intensive firm. The contrasting evolutionary paths of niche emergence within the music industry are an avenue for further study in both innovation and entrepreneurship studies.
Lastly, the methods and findings in this paper can potentially be used to address how knowledge networks play a role in the clustering patterns of creative industries as a whole, a research gap highlighted by Gong and Hassink (Citation2017). The three network patterns identified in our groups display parallels to other creative sectors, drawing upon similar power dynamics. These include patterns of local specialisation arising from university spinoffs, e.g. the Shanghai architecture design cluster (Cang and Wang Citation2008), and the New York fashion cluster (Wu Citation2005), where new ventures spawned from a central, knowledge-rich institution. Alternatively, corporate spinoffs such as those in the global fashion industry (Wenting Citation2008) and the global video game industry (De Vaan, Boschma, and Frenken Citation2013) exhibit localisation, but without as much reliance on a central, knowledge-intensive entity. Furthermore, the role of formal institutions, such as those in the Leipzig new media cluster (Bathelt Citation2004) may also serve as a non-nodal controller of network dynamics. Finally, the role of informal institutions and ‘communities of practices’ ma give rise to geographically dispersed sectors such as ICT (Darchen Citation2016).
Disclosure statement
No potential conflict of interest was reported by the author(s).
Additional information
Funding
Notes
1 With these propositions in mind, we acknowledge that other actors play a role (e.g. managers, producers, album cover artists, etc.). However, this paper considers a knowledge network purely in the creative dimension.
2 We do note that the concept of niche has been applied in recent literature on innovation, particularly the transition to sustainability in an evolutionary context, i.e. Strategic Niche Management (Schot and Geels Citation2008). However, we view niche creation here as creativity-driven and less as a necessary demand-driven function of transforming society towards sustainable development.
3 While sales are important in many respects, artists will often continue to release work that they deem to have artistic merit or value even if it does not generate vast financial returns. We argue that many musician-entrepreneurs may make decisions of this nature based on what they can afford to lose (Shackle Citation1966) when it comes to releasing recorded music via a record label or on-line platform.
4 While not an exact equivalent, a scene may be characterised as a cultural space within which a wide range of musical practices coexist with one another, including personal interaction and various processes of differentiation, leading to cross-fertilisation in a variety of ways that may in-turn spawn new scenes (Straw Citation1991). While scenes in art and music have been combined with social network analysis to explore sociological issues such as cultural and social movements (Straw Citation1991), their productive capacity for collective action (Crossley Citation2008, Citation2009; Bottero and Crossley Citation2011), along with how systemic properties of such networks may lead to increased financial and artistic performance (Uzzi and Spiro Citation2005), there has been little application of music scene networks to evolutionary theory and its relation to creative industries.
5 With, of course, the exception of direct intellectual property rights on music releases. What we are referring to here is the freedom to emulate style and sound.
6 Note that individuals can also include graphic artists, DJs and anyone that does not have a direct connection to a piece of music.
7 This may be described as a one-mode network, i.e. one that exhibits one set of nodes with their linkages mapped on a single dimension. Thus, although this network may be defined as an ‘affiliation network’ (Davis, Gardner, and Gardner Citation1941), this paper places an emphasis on performances.
8 Note that the colour version of all figures may be found in the on-line version of this manuscript.
9 Composed with a Compound Spring Embedder (CoSE) Algorithm.
10 Note that for illustrative reasons no further orders are depicted here, and hence some linkages are hidden.
11 This is due to individuals’ year of birth being recorded in the database, rather than the year they were first active as a solo performer. With groups, this same variable is interpreted as founding year.
12 These tags were then organised into a number of defined genre categories, and under two hierarchical levels, the definition of which was taken from the Musicmap project (Crauwels Citation2019). It should be acknowledged that this stated hierarchy is in no way absolute, as in reality several subgenres may overlap between more than one supergenre.
13 For example, the band Massive Attack lists electronic, trip hop, downtempo, ambient and dub as genres.
14 That is, the local clustering coefficient.
15 For an undirected graph.
16 That is, .
17 These conditions are broadly in-line with the three commonly cited in the Strategic Niche Management (SNM) literature. Kemp, Schot, and Hoogma (Citation1998) and Schot, Hoogma, and Elzen (Citation1994) provide a theoretical foundation of this process.
18 We choose these specific thresholds due to the observed distribution of in 2019. There is a notable spike in
at 1/3, 1/2 and 2/3. This is not surprising, given the nature of
with respect to node and link ratios (factors of either 2 or 3).
19 Note that what appears to be zero is actually very close to zero.
20 Empirically, the decision to choose three clusters was based on identifying the ‘elbow point’ when plotting the number of clusters against the total within clusters sum of squares.
21 Predominantly, German, Spanish, Swedish, Danish as well as English; as English language pages do not always provide sufficient details for artists outside the English-speaking world.
References
- Arthur, B. 2007. “The Structure of Invention.” Research Policy 36 (2): 274–287. doi:https://doi.org/10.1016/j.respol.2006.11.005.
- Audretsch, D. B., and M. P. Feldman. 1996. “R&D Spillovers and the Geography of Innovation and Production.” American Economic Review 86 (3): 630–640.
- Azoulay, P., J. S. G. Zivin, and J. L. Wang. 2010. “Superstar Extinction.” Quarterly Journal of Economics 125 (2): 549–589. doi:https://doi.org/10.1162/qjec.2010.125.2.549.
- Bagley, M. J. O. 2019a. “Networks, Geography and the Survival of the Firm.” Journal of Evolutionary Economics 29 (4): 1173–1209. doi:https://doi.org/10.1007/s00191-019-00616-z.
- Bagley, M. J. O. 2019b. “Small Worlds, Inheritance Networks and Industrial Clusters.” Industry and Innovation 26 (7): 741–768. doi:https://doi.org/10.1080/13662716.2018.1539650.
- Bathelt, H. 2004. “Toward a Multidimensional Conception of Clusters: The Case of the Leipzig Media Industry, Germany.” In Cultural Industries and the Production of Culture, edited by D. Power and A. J. Scott, 147–168. London: Routledge.
- Becker, H. S. 1976. “Art Worlds and Social Types.” American Behavioral Scientist 19 (6): 703–718. doi:https://doi.org/10.1177/000276427601900603.
- Becker, M. H. 1970. “Sociometric Location and Innovativeness: Reformulation and Extension of the Diffusion Model.” American Sociological Review 35 (2): 267–282. doi:https://doi.org/10.2307/2093205.
- Bennett, A. 2004. “Consolidating the Music Scenes Perspective.” Poetics 32 (3–4): 223–234. doi:https://doi.org/10.1016/j.poetic.2004.05.004.
- Bollobás, B. 2001. “Random Graphs.” Cambridge Studies in Advanced Mathematics, Vol. 73 vols. 2nd ed. Cambridge: Cambridge University Press.
- Boone, C., and A. Van Witteloostuijn. 1995. “Industrial Organization and Organizational Ecology: The Potentials for Cross-fertilization.” Organization Studies 16 (2): 265–298. doi:https://doi.org/10.1177/017084069501600204.
- Borgatti, S. 2006. “Identifying Sets of Key Players in a Social Network.” Computational and Mathematical Organization Theory 12 (1): 21–34. doi:https://doi.org/10.1007/s10588-006-7084-x.
- Boschma, R. A. 2005. “Proximity and Innovation: A Critical Assessment.” Regional Studies 39 (1): 61–74. doi:https://doi.org/10.1080/0034340052000320887.
- Bottero, W., and N. Crossley. 2011. “Worlds, Fields and Networks: Becker, Bourdieu and the Structures of Social Relations.” Cultural Sociology 5 (1): 99–119. doi:https://doi.org/10.1177/1749975510389726.
- Bourdieu, P. F. 1993. The Field of Cultural Production: Essays on Art and Literature. New York: Columbia University Press.
- Breschi, S., and F. Lissoni. 2003. “Mobility and Social Networks: Local Knowledge Spillovers Revisited.” CESPRI Working Paper No. 142.
- Bridgstock, R. 2013. “Not a Dirty Word: Arts Entrepreneurship and Higher Education.” Arts and Humanities in Higher Education 12 (2–3): 122–137. doi:https://doi.org/10.1177/1474022212465725.
- Bridson, K., J. Evans, R. Varman, M. Volkov, and S. McDonald. 2017. “Questioning Worth: Selling Out in the Music Industry.” European Journal of Marketing 51 (9/10): 1650–1668. doi:https://doi.org/10.1108/EJM-06-2015-0391.
- Burt, R. S. 2004. “Structural Holes and Good Ideas.” American Journal of Sociology 110 (2): 349–399. doi:https://doi.org/10.1086/421787.
- Campbell, D. 1960. “Blind Variation and Selective Retention in Creative Thought as in Other Knowledge Processes.” Psychological Review 67 (6): 380–400. doi:https://doi.org/10.1037/h0040373.
- Cang, P., and S. Wang. 2008. “Studies on Mechanism of Industrial Clusters around University Based on Ground Theory – A Case Study of the Industrial Clusters around Tongji University.” Tongji University Journal Social Science Section. 19 (2): 115–124. (in Chinese).
- Caves, R. E. 2000. Creative Industries: Contracts between Art and Commerce. Cambridge, MA: Harvard University Press.
- Cohen, W., and D. Levinthal. 1990. “Absorptive Capacity: A New Perspective on Learning and Innovation.” Administrative Science Quarterly 35 (1): 128–152. doi:https://doi.org/10.2307/2393553.
- Coles, A.-M., A. Piterou, and A. Sentić. 2018. “Is Small Really Beautiful? A Review of the Concept of Niches in Innovation.” Technology Analysis & Strategic Management 30 (8): 895–908. doi:https://doi.org/10.1080/09537325.2017.1408907.
- Crauwels, K. 2019. “Music Map.” Accessed October 13. https://musicmap.info
- Crossley, N. 2008. “Pretty Connected: The Social Network of the Early UK Punk Movement.” Theory, Culture & Society 25 (6): 89–116. doi:https://doi.org/10.1177/0263276408095546.
- Crossley, N. 2009. “The Man Whose Web Expanded: Network Dynamics in Manchester’s Post/punk Music Scene 1976–1980.” Poetics 37 (1): 24–49. doi:https://doi.org/10.1016/j.poetic.2008.10.002.
- Darchen, S. 2016. “’Clusters’ or ‘Communities’? Analysing the Spatial Agglomeration of Video Game Companies in Australia.” Urban Geography 37 (2): 202–222. doi:https://doi.org/10.1080/02723638.2015.1067981.
- Davis, A., B. B. Gardner, and M. R. Gardner. 1941. Deep South: A Social Anthropological Study of Caste and Class. Chicago: University of Chicago Press.
- Davis, G. F., M. Yoo, and W. Baker. 2003. “The Small World of the American Corporate Elite, 1982–2001.” Strategic Organization 1 (3): 301–326. doi:https://doi.org/10.1177/14761270030013002.
- De Vaan, M., R. Boschma, and K. Frenken. 2013. “Clustering and Firm Performance in Project-based Industries: The Case of the Global Video Game Industry, 1972–2007.” Journal of Economic Geography 13 (6): 965–991. doi:https://doi.org/10.1093/jeg/lbs038.
- Debruyne, M., and D. J. Reibstein. 2005. “Competitor See Competitor Do - Incumbent Entry in New Market Niches.” Marketing Science 24 (1): 55–66. doi:https://doi.org/10.1287/mksc.1040.0064.
- Döring, T., and J. Schnellenbach. 2006. “What Do We Know about Geographical Knowledge Spillovers and Regional Growth?” Regional Studies 40 (3): 375–395. doi:https://doi.org/10.1080/00343400600632739.
- Estrada, E., and J. A. Rodríguez-Velázquez. 2005. “Subgraph Centrality in Complex Networks.” Physical Review E 71 (5): 056103. doi:https://doi.org/10.1103/PhysRevE.71.056103.
- Fleming, L. 2001. “Recombinant Uncertainty in Technological Search.” Management Science 47 (1): 117–132. doi:https://doi.org/10.1287/mnsc.47.1.117.10671.
- Fleming, L. 2002. “Finding the Organizational Sources of Technological Breakthroughs: The Story of Hewlett Packard’s Thermal Inkjet.” Industrial and Corporate Change 11 (5): 1059–1084. doi:https://doi.org/10.1093/icc/11.5.1059.
- Florida, R., C. Mellander, and K. Stolarick. 2010. “Music Scenes to Music Clusters: The Economic Geography of Music in the US, 1970–2000.” Environment & Planning A 42 (4): 785–804. doi:https://doi.org/10.1068/a4253.
- Foray, D. 2014. “From Smart Specialisation to Smart Specialisation Policy.” European Journal of Innovation Management 17 (4): 492–507. doi:https://doi.org/10.1108/EJIM-09-2014-0096.
- Freeman, L. C. 1977. “A Set of Measures of Centrality Based on Betweenness.” Sociometry 40 (1): 35–41. doi:https://doi.org/10.2307/3033543.
- Frenken, K., P. P. Saviotti, and M. Trommetter. 1999. “Variety and Niche Creation in Aircraft, Helicopters, Motorcycles and Microcomputers.” Research Policy 28 (5): 469–488. doi:https://doi.org/10.1016/S0048-7333(99)00008-6.
- Geroski, P. A. 2001. “Exploring the Niche Overlaps between Organizational Ecology and Industrial Economics.” Industrial and Corporate Change 10 (2): 507–540. doi:https://doi.org/10.1093/icc/10.2.507.
- Gersbach, H., and A. Schmutzler. 1999. “External Spillovers, Internal Spillovers and the Geography of Production and Innovation.” Regional Science and Urban Economics 29 (6): 679–696. doi:https://doi.org/10.1016/S0166-0462(99)00026-5.
- Gifford, E., M. McKelvey, and R. Saemundsson. 2021. “The Evolution of Knowledge-intensive Innovation Ecosystems: Co-evolving Entrepreneurial Activity and Innovation Policy in the West Swedish Maritime System.” Industry and Innovation 28 (5): 651–676. doi:https://doi.org/10.1080/13662716.2020.1856047.
- Giles, D. E. 2006. “Superstardom in the US Popular Music Industry Revisited.” Economics Letters 92 (1): 68–74. doi:https://doi.org/10.1016/j.econlet.2006.01.022.
- Gong, H., and R. Hassink. 2017. “Exploring the Clustering of Creative Industries.” European Planning Studies 25 (4): 583–600. doi:https://doi.org/10.1080/09654313.2017.1289154.
- Gould, R. V. 1993. “Collective Action and Network Structure.” American Sociological Review 58 (2): 182–196. doi:https://doi.org/10.2307/2095965.
- Gulati, R., M. Sytch, and A. Tatarynowicz. 2012. “The Rise and Fall of Small Worlds: Exploring the Dynamics of Social Structure.” Organization Science 23 (2): 449–471. doi:https://doi.org/10.1287/orsc.1100.0592.
- Gustafsson, E. 2019. “Fashioning a Knowledge Intensive Entrepreneur?” PhD diss., University of Gothenburg.
- Hannan, M., and J. Freeman. 1977. “The Population Ecology of Organizations.” American Journal of Sociology 82 (5): 929–964. doi:https://doi.org/10.1086/226424.
- Hermans, F., D. van Apeldoorn, M. Stuiver, and K. Kok. 2013. “Niches and Networks: Explaining Network Evolution through Niche Formation Processes.” Research Policy 42 (3): 613–623. doi:https://doi.org/10.1016/j.respol.2012.10.004.
- Hodgson, G. M. 1996. Economics and Evolution: Bringing Life Back into Economics. Ann Arbor: University of Michigan Press.
- Jackson, M. O. 2008. Social and Economic Networks. Princeton: Princeton University Press.
- Kauffman, S., and S. Levin. 1987. “Towards a General Theory of Adaptive Walks on Rugged Landscapes.” Journal of Theoretical Biology 128 (1): 11–45. doi:https://doi.org/10.1016/S0022-5193(87)80029-2.
- Kauffman, S. 1996. At Home in the Universe. Oxford: Oxford University Press.
- Kemp, R., J. Schot, and R. Hoogma. 1998. “Regime Shifts to Sustainability through Processes of Niche Formation: The Approach of Strategic Niche Management.” Technology Analysis & Strategic Management 10 (2): 175–198. doi:https://doi.org/10.1080/09537329808524310.
- Kim, H., and P. S. Bearman. 1997. “The Structure and Dynamics of Movement Participation.” American Sociological Review 62 (1): 70–93. doi:https://doi.org/10.2307/2657453.
- Klepper, S. 2002. “The Capabilities of New Firms and the Evolution of the U.S. Automobile Industry.” Industrial and Corporate Change 11 (4): 645–666. doi:https://doi.org/10.1093/icc/11.4.645.
- Komninos, N., B. Musyck, A. I. Reid, and M. Landabaso. 2014. “Smart Specialisation Strategies in South Europe during Crisis.” European Journal of Innovation Management 17 (4): 448–471. doi:https://doi.org/10.1108/EJIM-11-2013-0118.
- Lassen, A. H., M. McKelvey, and D. Ljungberg. 2018. “Knowledge-intensive Entrepreneurship in Manufacturing and Creative Industries: Same, Same, but Different.” Creativity and Innovation Management 27 (3): 284–294. doi:https://doi.org/10.1111/caim.12292.
- MacQueen, J. B. 1967. “Some Methods for Classification and Analysis of Multivariate Observations.” Proceedings of the fifth Berkeley symposium on mathematical statistics and probability, 1, 281–297. Oakland, CA, USA.
- Maggioni, M. A., M. Nosvelli, and T. E. Uberti. 2007. “Space versus Networks in the Geography of Innovation: A European Analysis.” Papers in Regional Science 86 (3): 471–493. doi:https://doi.org/10.1111/j.1435-5957.2007.00130.x.
- Maggioni, M. A., and T. E. Uberti. 2011. “Networks and Geography in the Economics of Knowledge Flows.” Quality & Quantity 45 (5): 1065–1066. doi:https://doi.org/10.1007/s11135-011-9491-4.
- Malerba, F., and M. McKelvey. 2020. “Knowledge-intensive Innovative Entrepreneurship Integrating Schumpeter, Evolutionary Economics, and Innovation Systems.” Small Business Economics 54 (2): 503–522. doi:https://doi.org/10.1007/s11187-018-0060-2.
- McPherson, M., L. Smith-Lovin, and J. M. Cook. 2001. “Birds of a Feather: Homophily in Social Networks.” Annual Review of Sociology 27 (1): 415–444. doi:https://doi.org/10.1146/annurev.soc.27.1.415.
- Metal-Archives. 2019. “Encyclopaedia Metallum: The Metal Archives.” Accessed 20 September 2019. https://www.metal-archives.com
- Metcalfe, J. S. 1994. “Evolutionary Economics and Technology Policy.” The Economic Journal 104 (425): 931–944. doi:https://doi.org/10.2307/2234988.
- Metcalfe, J. S. 2002. “Knowledge of Growth and the Growth of Knowledge.” Journal of Evolutionary Economics 12 (1–2): 3–15. doi:https://doi.org/10.1007/s00191-002-0107-y.
- Møen, J. 2005. “Is Mobility of Technical Personnel a Source of R&D Spillovers?” Journal of Labor Economics 23 (1): 81–114. doi:https://doi.org/10.1086/425434.
- MusicBrainz. 2019. “The MusicBrainz Database.” Accessed 10 September 2019. http://www.musicbrainz.org
- Nelson, R., and S. Winter. 1982. An Evolutionary Theory of Economic Change. Cambridge, MA: Harvard University Press.
- Obstfeld, D. 2005. “Social Networks, the Tertius Iungens Orientation, and Involvement in Innovation.” Administrative Science !uarterly 50 (1): 100–130. doi:https://doi.org/10.2189/asqu.2005.50.1.100.
- Oliver, P., G. Marwell, and R. Teixeira. 1985. “A Theory of the Critical Mass. I. Interdependence, Group Heterogeneity, and the Production of Collective Action.” American Journal of Sociology 91 (3): 522–556. doi:https://doi.org/10.1086/228313.
- Perry-Smith, J. E. 2006. “Social yet Creative: The Role of Social Relationships in Facilitating Individual Creativity.” Academy of Management Journal 49 (1): 85–101. doi:https://doi.org/10.5465/amj.2006.20785503.
- Potts, J. 2009. “Why Creative Industries Matter to Economic Evolution.” Economics of Innovation and New Technology 18 (7): 663–673. doi:https://doi.org/10.1080/10438590802564592.
- Progarchives. 2019. “The Progarchives Database.” Accessed 19 September 2019. http://www.progarchives.com
- Raghavan, U. N., R. Albert, and S. Kumara. 2007. “Near Linear Time Algorithm to Detect Community Structures in Large-scale Networks.” Physical Review E 76 (3): 036106. doi:https://doi.org/10.1103/PhysRevE.76.036106.
- Rake, B., P. D’este, and M. McKelvey. 2021. “Exploring Network Dynamics in Complex Fields of Science: The Formation of Ties to Knowledge Translators.” Journal of Evolutionary Economics 31 5 1433–1464 https://doi.org/https://doi.org/10.1007/s00191-020-00716-1 .
- Savino, T., P. A. Messeni, and V. Albino. 2017. “Search and Recombination Process to Innovate: A Review of the Empirical Evidence and A Research Agenda.” International Journal of Management Reviews 19 (1): 54–75. doi:https://doi.org/10.1111/ijmr.12081.
- Schot, J., and F. W. Geels. 2008. “Strategic Niche Management and Sustainable Innovation Journeys: Theory, Findings, Research Agenda, and Policy.” Technology Analysis & Strategic Management 20 (5): 537–554. doi:https://doi.org/10.1080/09537320802292651.
- Schot, J., R. Hoogma, and B. Elzen. 1994. “Strategies for Shifting Technological Systems: The Case of the Automobile System.” Futures 26 (10): 1060–1076. doi:https://doi.org/10.1016/0016-3287(94)90073-6.
- Shackle, G. L. S. 1966. “Policy, Poetry and Success.” The Economic Journal 76 (304): 755–767. doi:https://doi.org/10.2307/2229081.
- Simon, H. A. 1983. “Discovery, Invention, and Development: Human Creative Thinking.” Proceedings of the National Academy of Sciences of the United States of America Washington, D.C., USA 80 ( 14): 4569–4571.
- Simon, H. A. 1978. “Rationality as Process and as Product of Thought.” American Economic Review 68 (2): 1–16.
- Simon, H. A. 1996. The Sciences of the Artificial. Cambridge, MA: MIT Press.
- Singh, G., and A. Prasad. 2014. “Innovation and Entrepreneurial Activities of SMEs in Fiji.” International Journal of Entrepreneurship and Small Business 22 (2): 251–265. doi:https://doi.org/10.1504/IJESB.2014.062504.
- Steinhaus, H. 1957. “Sur la division des corps matériels en parties.” Bulletin L’Académie Polonaise des Science 4 (12): 801–804.
- Straw, W. 1991. “Systems of Articulation, Logics of Change: Communities and Scenes in Popular Music.” Cultural Studies 5 (3): 368–388. doi:https://doi.org/10.1080/09502389100490311.
- Torre, A., and J. P. Gilly. 1999. “On the Analytical Dimension of Proximity Dynamics.” Regional Studies 34 (2): 169–180.
- Utterback, J. M., and W. J. Abernathy. 1975. “A Dynamic Model of Process and Product Innovation.” Omega 3 (6): 639–656. doi:https://doi.org/10.1016/0305-0483(75)90068-7.
- Uzzi, B., and J. Spiro. 2005. “Collaboration and Creativity: The Small World Problem.” American Journal of Sociology 111 (2): 447–504. doi:https://doi.org/10.1086/432782.
- Watts, D. J., and S. H. Strogatz. 1998. “Collective Dynamics of “Small-world” Networks.” Nature 393 (6684): 440–442. doi:https://doi.org/10.1038/30918.
- Wenting, R. 2008. “Spinoff Dynamics and the Spatial Formation of the Fashion Design Industry, 1858–2005.” Journal of Economic Geography 8 (5): 593–614. doi:https://doi.org/10.1093/jeg/lbn030.
- Winter, S. G. 1990. “Survival, Selection, and Inheritance in Evolutionary Theories of Organization.” In Organizational Evolution: New Directions, edited by J. Singh, 269–297. London: Sage.
- Witt, U. 1992. “Evolutionary Concepts in Economics.” Eastern Economic Journal 18 (4): 405–419.
- Wright, S. 1932. “The Roles of Mutation, Inbreeding, Crossbreeding, and Selection in Evolution.” Proceedings of the Sixth International Congress on Genetics. The Hague, The Netherlands 1 ( 8): 355–366.
- Wu, W. 2005. Dynamic Cities and Creative Clusters. Washington, DC: World Bank.
Table 1.
Order of analysis
Table 2. Overview: Geography
Table 3. Overview: Genre
Table 4. Identified niches
Table 5. Early recombination, intermediate-stage reinforcement (group 1) niches
Table 6. Intermediate-stage recombination and reinforcement (group 2) niches
Table 7. Late recombination and reinforcement (group 3) niches