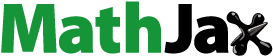
ABSTRACT
Instead of comparing open and closed triads as static phenomena, this study examines how closure dynamics among inventors impact the extent to which inventors generate high-quality inventions at the triad level. Combining literature on small group synergy, social networks, and recombinant innovation, we propose that initial open triads of collaborating inventors that turn into a closed triad generate higher quality inventions than triads that maintain open. We also examine how the connectedness of the triad moderates the relationship between triad closure and the generation of triadic high-quality inventions. Using a matched sample of open and closed triads from 1987 to 2008, we find that over time, open triads that turn into closed ones generate higher-quality inventions than triads that remain open. Moreover, the triad’s degree of connectedness weakens the triadic closure’s positive impact on inventive performance. We discuss the implications for the study of innovation, network triads, and collective synergy.
1. Introduction
A core area of research on inventor networks concerns the impact on innovation generation (H. Singh et al. Citation2016). This literature emphasises the importance of networks because the innovation process is inherently social, where inventors engage in tasks that focus on collaborative problem-solving by providing each other with knowledge, support, and referrals (Fleming and Sorenson Citation2004; J. Singh and Fleming Citation2010; H. Singh et al. Citation2016). Within this area of investigation, Burt’s structural hole concept (Burt Citation2004), in particular, has been highly influential as it highlighted the benefits of open triads for ideas and novelty for individual brokers. Individuals occupying network brokerage positions tend to create novel innovations due to access to diverse and new knowledge and control benefits (Burt Citation2004; Fleming, Mingo, and Chen Citation2007). However, while structural brokerage positions promote novel recombination of existing ideas, a brokerage position simultaneously hampers the implementation of these combinations and utilisation by others (Burt Citation2004; Fleming, Mingo, and Chen Citation2007; Obstfeld Citation2005).
Prior work on brokerage has improved our understanding of how triadic structural properties influence innovation-related outcomes (Kauppila, Bizzi, and Obstfeld Citation2018; Obstfeld Citation2005; Soda, Tortoriello, and Iorio Citation2018). Interestingly, studies on the relationship between triadic structures and invention outcomes primarily focus on individual performance.Footnote1 An example is the study by Fleming, Mingo, and Chen (Citation2007), who examine the influence of brokered versus cohesive, collaborative social structures on an individual’s creativity. Furthermore, Gómez‐Solórzano, Tortoriello, and Soda (Citation2019) study, within the R&D function of a large European pharmaceutical company, how embedded triadic relationships of instrumental (i.e. knowledge-sharing) and non-instrumental (i.e. friendship) ties among inventors affect their ability to generate innovations. More recently, Llopis and D’Este (Citation2022) investigate the effects of balanced and unbalanced open triads on individual innovativeness.
Focusing on individual outcomes has greatly improved our understanding of how the social environment influences individual innovative performance. However, it draws attention away from the empirical and organisational fact that knowledge production and innovation often result from a combined effort of social actors whose resources synthesise novel outcomes at a collective level. Collaborative knowledge production is becoming the standard in many creative and innovation-based sectors (Wuchty, Jones, and Uzzi Citation2007). Invention follows this same development, fuelled by the increasingly high cumulative inventor knowledge needed to excel in a domain (Aggarwal, Hsu, and Wu Citation2020). With inventors becoming narrower but deeper in their knowledge domains, a group-based patent production structure may compensate for specialisation at the individual level (Orsatti, Quatraro, and Pezzoni Citation2020). It is, therefore, essential to shift from the individual to the group level. Our study focuses on the triadic level as the basic social unit, when investigating networks and relationships (Simmel Citation1950; Tortoriello and Krackhardt Citation2010).
Combining a dynamic view of triadic structure and outcomes (Spiro, Acton, and Butts Citation2013) with the recombinant view on innovation (Schumpeter Citation1934) and literature on small groups (Larson Citation2010), we answer our research question: To what extent do open collaboration triads among inventors, that over time turn into closed triads, generate higher quality inventions at the triadic level compared to triads that remain open? Furthermore, we explore the question of how the connectedness of the inventors’ triad influences the relationship between dynamic triadic structure and invention outcomes. We test our hypotheses on a large-scale longitudinal dataset comprising a matched set of 41,698 inventor triads in the U.S. biotechnology industry from 1987 to 2008. Findings reveal that open triads that close over time and connect previously unconnected alters positively influence invention quality at the triadic level. However, we find that the connectedness of the triad negatively moderates the positive relationship between triadic structure and triadic innovation outcomes.
Our study makes three contributions to the existing network and innovation literature. First, informed by the observation that innovation is increasingly a collective effort, this study shifts the theoretical and empirical attention from the individual to outcomes at the triadic level. In this way, we increase our knowledge of network dynamics and its outcome effects at this fundamental network unit and shift our attention to a collective outcome highly relevant to organisational practice. Second, we challenge the conventional wisdom in the social network literature that open triads, in most instances, perform better. We combine theoretical insights from network and innovation studies with insights from the teams and small groups literature, more specifically from the literature on group synergy (Kurtzberg and Amabile Citation2001) and collective intelligence (Woolley, Aggarwal, and Malone Citation2015) in one model. Furthermore, we theoretically argue and empirically show that closed triads are conducive to triadic innovation performance. The small group synergy literature’s theoretical precision helps network scholars delineate why specific tasks conducted in closed triads are more productive. Combining these relatively detached bodies of literature is a main theoretical contribution that leads to a deeper understanding of the relationship between network and collective outcome. Third, we contribute to brokerage theory on how the broader co-invention context in which the triad is located moderates the ability of the inventor triad to benefit in terms of inventive performance. In their review of the brokerage literature, Kwon et al. (Citation2020) conclude that prior research on brokerage as behaviour has paid predominant attention to the broker. Other actors inside and outside the triad are usually neglected, with only a few exceptions (e.g. Zhelyazkov Citation2018). Kwon et al. (Citation2020) consider this a major future research challenge. We answer this call by studying the connectedness of alters of the triad in the context of invention quality. In this way, a network structural element external to the triad is regarded as impactful for the collective outcomes of the invention process.
The rest of the paper is organised as follows. The following section reviews the literature on triadic performance and its relation with triadic network structure. Section three develops the theoretical mechanisms and sets up the hypotheses we seek to test. Section four introduces the treatment-control research design and discusses the development of the biotechnology sample, variable construction, and estimation method. Sections 4 and 5 present the results and robustness checks. Sections 6 and 7 discuss the implications of our findings and broader contribution to the literature while also identifying avenues for future research.
2. Theoretical background
2.1. Triadic performance
We build upon the common notion in innovation studies, which asserts that invention is the outcome of knowledge recombination (Fleming and Sorenson Citation2004; Schumpeter Citation1934). To arrive at new combinations of existing and novel knowledge components, inventors usually engage in creative, problem-solving, and search processes in which they explore and apply solutions to problems that they encounter themselves (Arthur Citation2007) or are identified by their superiors at the corporate research and development (R&D) lab (Toh and Polidoro Citation2013). Prior research has shown that the recombinant innovation process is often a social or relational process where inventors engage in tasks that focus on collaborative or interactive problem-solving by providing each other with (novel) knowledge, support, referrals, and cognitive search maps (Fleming and Sorenson Citation2004; A. Hargadon and Sutton Citation1997; H. Singh et al. Citation2016).
The literature on teams and group outcomes has shown that depending on the nature of the task and under certain conditions, groups outperform individuals or vice versa. Idea generation is a good example. The literature (e.g. Osborn Citation1953) initially maintained that individuals outperformed small groups. For instance, so-called production blocking causes poor group performance because group members must wait for each other to share ideas, severely hindering individual idea generation (Diehl and Stroebe Citation1987). Later research (Dugosh et al. Citation2000) showed that conditionally on cognitive stimulation, groups generated more ideas than individuals did. Cognitive stimulation regards activities designed to enhance cognitive functioning, including memory, attention, and other mental processes. This leads to an important question: For which tasks and under which conditions do small groups, like triads, perform better than individuals, and which mechanisms are at work in these cases?
Put differently, when do (small) collectives show synergy or collective intelligence? Larson (Citation2010, 4) states that ‘a group is said to exhibit synergy when it is able to accomplish collectively something that could not reasonable have been achieved by any simple combination of individual member efforts’. A related concept stressing group processes instead of outcomes is collective intelligence. Teams or groups behave collectively intelligently when their collective behaviours during the collaboration period are in line with the requirements of the (cognitive) tasks the team is assigned to and the (changing) environment (Janssens, Meslec, and Leenders Citation2022). Both concepts share a focus on interaction among individuals. Interaction refers to group members’ behaviours conducted together with or performed in the presence of other group members. It concerns (non)verbal face-to-face behaviours and behaviours conducted remotely or asynchronously, which also implies mutual adjustments (see Larson Citation2010, 5–6). The literature distinguishes weak and strong synergy (Larson Citation2010). Weak synergy is commonly defined as the performance of a group that is higher than the performance of a typical group member working individually. Strong synergy refers to the situation in which the group performs higher than the best-performing group member. This distinction is relevant to the discussion on the mechanisms supporting the relationship between triadic closure and invention quality.
Invention generation by inventors includes several tasks. The most important ones are generating creative ideas, solving problems, sharing and integrating knowledge, and learning (such as acquiring, retaining, and retrieving knowledge; Arthur Citation2007; Savino, Messeni Petruzzelli, and Albino Citation2017). The literature on synergy and collective intelligence in small groups identifies three main tasks in which groups outperform individuals: (1) idea generation, (2) problem-solving, and (3) learning. Small groups tend to outperform individuals when it comes to idea generation conditional on the use of cognitive stimulation (Nijstad, Stroebe, and Lodewijkx Citation2002). Social interaction is essential here as it compels people to the ideas of others.
Furthermore, groups outperform individuals if problems to solve are complex (Jones Citation2009) and outcomes are demonstrable, meaning people can make inferences about correct problem solutions (Laughlin et al. Citation2006). Because solving complex problems requires higher intellectual effort, small groups can benefit from collective and complementary knowledge, social support, and a division of labour among group members.
In the context of learning, small groups tend to learn better and more than individuals alone. The literature lists several cognitive and social mechanisms (Nokes-Malach, Richey, and Gadgil Citation2015): cross-cueing, increasing working memory resources, joint management of attention, and using multiple perspectives. Important conditions for this group learning to emerge are that groups have a history of collaboration and work on similar tasks.
2.2. Triadic structure as a driver of triadic performance
The previous sections argued that a shift from the individual to the small group (triadic) level is needed. Furthermore, mechanisms and conditions were discussed when and why specific tasks performed by small groups, like triads, outperform individuals.
In this study, we particularly focus on the relationships among inventors as indicated by co-invention patterns (Moreira, Markus, and Laursen Citation2018; Nerkar and Paruchuri Citation2005) in triadic network structures. Both anecdotal evidence and large-scale quantitative studies have shown that such patent co-invention ties involve intensive interaction and actual knowledge transfer between inventors (Carnabuci and Operti Citation2013; Fleming, Mingo, and Chen Citation2007; J. Singh Citation2005). These ties are likely to be strong ties, meaning they may even facilitate the transfer of tacit knowledge, widening the scope for idea generation, problem-solving, knowledge recombination, and learning. As indicated, the literature shows a widespread consensus that interpersonal (i.e. between employees) relationships, such as the collaboration ties among inventors that we study here, shape the recombinant invention potential of individuals (Baer et al. Citation2015; Phelps, Heidl, and Wadhwa Citation2012; J. Singh and Fleming Citation2010). However, the question of which triadic structure (open versus closed) is especially beneficial for triadic performance remains unanswered. The next sections develop a theoretical perspective on this question.
3. Hypotheses
3.1. Triadic closure and invention quality
We examine the situation where an open triad closes over time and theoretically argue why this triadic structural dynamic benefits the quality of triadic invention. To structure our arguments, we use the core tasks to develop an invention (i.e. idea generation, problem-solving, and learning).
3.1.1. Idea generation
The primary goal of this task is to create new concepts, approaches, or possibilities. It focuses on the expansive thinking phase, aiming to generate a wide scope of ideas without immediately evaluating their feasibility or practicality. The initial open triad has the collective ability to produce a high number of ideas due to the diversity of inputs and the inflow of information channelled through the missing connection. We draw, therefore, upon the brokerage literature to understand an open triad’s information and control benefits (Burt Citation1992, Citation2004; Grosser, Obstfeld et al. Citation2018). Open triads give one of the participating actors an improved vision, with timely and proprietary access to information and knowledge. In the open triad, different social domains connect, which offer access to more diverse information, knowledge, experiences, and thought worlds (Burt Citation2004; A. Hargadon and Sutton Citation1997). Combining such unique resources provides an opportunity structure for the open triad to generate synergies of diverse ideas and perspectives that build upon the recombination of knowledge from the (three) inventors (Fleming, Mingo, and Chen Citation2007).
Nevertheless, the inventors in the open triad face a selection problem. After all, generating many ideas is one step, but selecting high-quality ones is the next step. The unconnected inventors’ different interests, perspectives, and languages will limit the triad’s ability to circulate ideas and knowledge efficiently. Idea circulation and communication among previously unconnected individuals generate an alignment of interests, perspectives, and languages (Obstfeld Citation2005). A welcome side effect of such connecting behaviour is that it motivates all three inventors to attend to usefulness when considering each other’s ideas and perspectives (Grant and Berry Citation2011), resulting in synergetic effects. This synergy offers the now-closed triad a situation in which there is a high-quality reciprocated exchange of ideas, which fosters the selection of high-quality ideas resulting in a higher invention quality. Empirical research produced mixed results, however. For instance, Williams and Sternberg (Citation1988) found that the quality of ideas was a positive function of small group interaction, whereas Rietzschel, Nijstad, and Stroebe (Citation2006) did not report a synergetic effect, although those groups tend to generate high-quality ideas more efficiently, that is, a lower number of ideas, but the same number of high-quality ideas. At best, there are some indications of weak synergy effects.
3.1.2. Problem-solving
Although idea generation and problem-solving are related, they are different processes. The former aims at producing a large number of ideas, which can later be filtered and refined. The latter follows a structured approach such as defining the problem, analysing possible causes, generating possible solutions, evaluating and selecting the best solution, and implementing it (Wang and Chiew Citation2010). McGrath (Citation1984) categorises them as generative talks with no correct solutions and intellective tasks with a correct solution respectively.
Open triads can promote innovativeness as diverse perspectives from external sources are introduced into the problem-solving process. This diversity can lead to creative and novel solutions (Reagans and McEvily Citation2003). The asymmetry in relationships within open triads allows for a broader range of ideas, potentially leading to a more comprehensive exploration of possible solutions and outcomes. The problem-solving outcomes in open triads follow a longer path length between actors, potentially resulting in delays or misunderstandings and therefore hindering optimal knowledge and information circulation. Once triads close and as compared to triads that stay open, more efficient problem-solving processes emerge due to direct communication and coordinated efforts. Solutions can be implemented more quickly with minimal communication barriers. The close-knit nature of closed triads results in consistent and cohesive outcomes, as inventors are closely connected and share a similar understanding of the problem and solution. Consequently, higher levels of coordination and integration generate higher invention quality (Amon, Vrzakova, and D’Mello Citation2019). In terms of synergy strength, there is ample evidence of strong synergy in group problem-solving performance. In sum, idea generation and problem-solving are related but activities with different foci. Both activities connect triadic closure and invention quality in comparable ways, but due to strong synergy, the mechanism is stronger for problem-solving activities.
3.1.3. Learning
Open triads introduce a broader range of perspectives into learning in the triad, as external ideas can enter the triad through an intermediary (A. B. Hargadon Citation2002). This diversity can enrich discussions and enhance the learning experience. The asymmetry in ties within open triads may stimulate innovative thinking and problem-solving within the group, contributing to a more creative learning environment. Learning in open triads may face challenges related to indirect communication and coordination. Information may need to pass through intermediaries, potentially leading to circulation delays and negatively influencing learning processes. Once a triad closes, coordinated learning efforts often emerge, fostering a synchronised understanding of the resources among triad members. Closed triadic structures facilitate efficient knowledge transfer and circulation among its members due to direct connections (Chai, Yap, and Wang Citation2011). Information can flow quickly through the established network, leading to a rapid acquisition of a shared understanding.
Furthermore, individuals within a closed triad likely accumulate trust and cooperative norms (Coleman Citation1988). This creates a safe environment where inventors are less worried about colleague inventors, particularly the initial broker, stealing ideas from them (Fleming, Mingo, and Chen Citation2007; Soda, Tortoriello, and Iorio Citation2018). Additionally, trust facilitates the acceptance of a new idea or divergent thinking (Burt and Merluzzi Citation2016). Due to the generated mutual ownership of newly developed knowledge combinations, the inventors engaged in the closed triad are likelier to adopt, champion, and diffuse ideas from the involved inventors (Fleming, Mingo, and Chen Citation2007). In turn, the increased trust among the inventors in the triad facilitates ongoing communication and effective transfer of complex knowledge, which is particularly relevant in R&D labs that rely on support and referrals to relevant bodies of knowledge (Reagans and McEvily Citation2003).
In sum, we assert that triadic closure improves the initial brokerage benefits of the open triad and counters the potential lack of coordinated action and communication from which open triads may suffer. The superior ability of the closed triad to mobilise, circulate, and coordinate diverse knowledge resources improves her future invention output quality at the triad level. Thus, we posit:
Hypothesis 1:
Open inventor triads that turn into closed triads over time generate higher triadic quality inventions than those that remain open.
3.2. The moderating effect of triad connectedness
Our first hypothesis considered the relationship between triad closure and the quality of inventions generated at the triad level. Additionally, we argue that the level of connectedness of the members of the triad influences this relationship. Connectedness refers to whether the inventors in a triad are connected to a few or many other alter inventors (i.e. via direct ties) and whether the alters are connected to highly connected alters of alters (i.e. indirect ties) (Bonacich Citation1987). We maintain that the extent to which a triad is connected to highly connected alters influences the relationship between triadic closure and invention quality. To develop a theoretical argument for this moderation effect, we build on Ahuja’s (Citation2000) distinction between direct and indirect ties and their function for invention. The number of direct ties of a triad brings three benefits for invention and its quality: knowledge sharing, complementarity, and scale (Ahuja Citation2000, 429). Indirect ties, however, perform different functions for invention generation. First, indirect ties can serve as an information-collection mechanism (Salman and Saives Citation2005). Through indirect ties, inventors can receive information on the success or failure of simultaneous research efforts (Breschi and Lenzi Citation2017), and technological dead ends or promising technological trajectories can be detected early. Second, indirect network ties can serve as screening devices (Devarakonda, Pavićević, and Reuer Citation2022), where additional partners inventors have can serve as an information filter, absorbing, sifting, and classifying new technical developments in a manner that goes beyond its information-processing capabilities.
Higher levels of triadic connectedness increase the amount of knowledge and information available to the triad. Triads connected to highly connected contacts have access to and receive a wider variety of diverse knowledge and information. Building on cognitive load theory (Sweller Citation2011), which is related to the bounded rationality concept (Simon Citation1991), we argue that the relationship between triadic closure and invention quality is negatively moderated by the connectedness of the members of a triad.
Cognitive load theory aims to understand how the cognitive load produced by tasks can hinder the actors’ ability to process new information and create long-term memories (Kirschner et al. Citation2018). Cognitive load typically increases when higher demands are imposed on an actor, making the task of processing information overly complex. The more direct and indirect ties an actor has, the more information is received. These large amounts of information lead to high levels of cognitive load, a situation often labelled as information overload (Edmunds and Morris Citation2000). Today, a broad consensus is that a heavy information load harms social actors’ performance.
When the supply of information is substantially higher than the information-processing capacity of the triad, difficulties emerge in identifying and dealing with (ir)relevant information. This especially occurs if the members of a triad have a higher level of connectedness. In this situation, more and more diverse information is flowing to the members of the triad via a larger number of indirect ties. Furthermore, research has shown that there is a negative relationship between path distance and tie strength (Burt, Opper, and Holm Citation2022), which as a consequence implies that the quality of the information is on average lower.
Although the information processing capacity of a closed triad is higher than that of a triad that stays open, the limits of the former can be reached, too. Information overload is the result (Jackson and Farzaneh Citation2012). In sum, higher levels of connectedness can lead to information overload due to too much diverse and difficult-to-understand inflow of information, which is more severe for triads that stay open compared to triads that close. As a result, invention quality suffers.
Therefore, our second hypothesis is as follows:
Hypothesis 2:
The relationship between triadic closure and triadic invention quality is negatively moderated by the inventor triad’s connectedness
4. Data and methods
Previous studies on triadic closure have predominantly used survey-based data-gathering methods in a single firm (Kauppila, Bizzi, and Obstfeld Citation2018; Quintane and Carnabuci Citation2016; Soda, Tortoriello, and Iorio Citation2018). This paper uses a different data collection approach to capture actual triadic closure over time. We rely instead on a large-scale and longitudinal dataset covering many firms and their inventors to accommodate the empirical demands. The primary data source is patent data, which allows us to observe both the collaborative behaviour of inventors over time and the quality of their inventions. For several reasons, we chose the biotechnology industry as our research setting. First, the biotechnology industry is one of the most innovative and knowledge-intensive fields (Tzabbar Citation2009) and is well-known for its distributed knowledge (Powell, Koput, and Smithdoerr Citation1996). Although not all biotechnology-related inventions are patentable, patents are widely used in this sector to measure knowledge and technological invention (Fritsch, Titze, and Piontek Citation2020; Lanjouw and Schankerman Citation2004). Second, teamwork is common in the biotech sector, and this industry is therefore often studied in the context of inventor collaboration (Nerkar and Paruchuri Citation2005; Paruchuri Citation2010; Tzabbar and Vestal Citation2015). Hence, collaboration among biotech R&D scientists leading to co-invented patents provides a rich setting for observing the evolution of collaboration networks and testing the relationship between triadic closure and invention quality.
4.1. Research design
We explain the research design here and describe how we created the sample in the Sample section below. The unit of analysis in our study is the triad level. Specifically, we regard the three inventors in the open or closed triad as one combined group and use the combined invention measures averaged over three inventors. We aim to create a sample of open triad relations that subsequently become closed triad relations (treatment group) and a comparable sample of open triad relations that did not become closed triads (control group). This design identifies the treatment effect of the treatment (Zhang and Tong Citation2021). Our study allows us to test whether inventors in the open triad experience the predicted change in their invention quality when they are involved in a triadic closure process. By adopting the triad level analysis and including a control sample of open triads that stay open over time, we can control for changes in the invention quality of three inventors due to the triadic closure. However, this design cannot tell whether triadic closure increases invention quality under all conditions or is a preferred mode for organising invention compared with other arrangements. Though less ideal than randomised controlled experiments, this research design represents a more feasible approach to address selection bias, a common issue for scholars working with observational data.
We further implement Coarsened exact matching (CEM) from the full treatment and control samples to develop a set of treatment and control triads that are more balanced on pretreatment covariates (Blackwell et al. Citation2009; Younge, Tong, and Fleming Citation2015). By balancing the pretreatment covariates, the matched treatment and control inventor triads are assumed to share similar characteristics of key covariates, further reducing endogeneity concerns.
4.2. Sample
Our primary data source is the DISCERN (Duke Innovation & Scientific Enterprises Research Network) dataset, complemented by two other data sources, the Harvard Patent Network Dataverse and WRDS Compustat database. DISCERN data matched the publicly listed U.S. headquartered firms with the assignees of patents from the United States Patent and Trademark Office (USPTO) from 1980 to 2015. DISCERN data accounts for firms’ name and ownership changes, thus improving the accuracy and scope of matches to patents, ownership structure, and dynamic reassignments of GVKEY codes to companies. Harvard Patent Data offers the cleaned and disambiguated inventor names, and the WRDS Compustat database includes firm attributes. Our sample consists of U.S. biotechnology utility patents granted between 1987 and 2008. We chose the period 1987–2008 because the biotechnology sector did not exist until 1973 when recombinant DNA was discovered, and serious collaboration and innovation started in the late 1980s. In addition, as we used the patent application year rather than the grant year to construct the inventor network, we need sufficient time lag between the application of patents to the grant of patents. Hence, as we only cleaned and disambiguated inventor names till 2010, we ended the observation in the year 2008. There are advantages and disadvantages to using patent data. For example, as not all inventions are patentable, using patent data to capture collaborative relations may result in neglecting other forms of collaborative relations (Fritsch, Titze, and Piontek Citation2020). Nevertheless, prior research has established that co-patenting can be utilised to represent collaboration and actual knowledge transfer between inventors during the innovation process (Carnabuci and Operti Citation2013; Fleming, Mingo, and Chen Citation2007; J. Singh Citation2005).
Our data construction process went as follows: First, we identified biotechnology patents by obtaining biotech firms and their patents. Specifically, we identified all publicly traded U.S. biotech firms alive between 1987 to 2008 using Standard Industrial Classification (SIC) Codes (2833, 2834, 2835, 2836, 5122)Footnote2 in the Compustat database (George et al. Citation2001; Rzakhanov Citation2004). There are around 2000 public biotech firms in the chosen period. Second, we searched these biotech firms’ GVKEY in the DISCERN dataset to obtain their USPTO patents. DISCERN data provides dynamic reassignments of GVKEY codes to companies and their patents. Dynamic reassignment means that, for instance, if a sample firm merges with another firm, the patents of the merged firm are included in the stock of patents linked to the Compustat recode from that point onward, but not before. DISCERN data also matched the patent data to Compustat companies and their related subsidiaries. This step produces approximately 578 biotechnology firms and 96,419 patents produced by these firms. Third, we searched these patents using their publication number in the Harvard Dataverse to obtain the cleaned and disambiguated inventor names associated with these patents (Li et al. Citation2014). Finally, these steps gave us 66,037 unique U.S. biotechnology patents by 48,960 biotech inventors.
We relied on the patent data to construct the inventors’ co-patenting network. When inventors are listed as inventors in a biotechnology patent, we assume that these inventors participate in biotechnology-related invention projects. Evidence from empirical studies (J. Singh Citation2005) and interviews with inventors and patent attorneys (Carnabuci and Operti Citation2013; Fleming, Mingo, and Chen Citation2007) indicate that co-invention or co-patenting reflects actual collaboration and that co-inventors provide information exchange and infrastructural support during and after the actual collaboration. A collaboration tie exists when two inventors work together on a project, which is operationalised in our study as two inventors listed as co-inventors on a patent (Fleming, Mingo, and Chen Citation2007; Lissoni, Llerena, and Sanditov Citation2013). We used patent application year rather than patent grant year as the former more accurately reflects inventor collaboration time (Guler and Nerkar Citation2012). We also assumed that collaborative relations last several years, even after the actual collaborative innovation, and followed previous studies in creating an inventor collaboration network with a three-year moving window (Fleming, Mingo, and Chen Citation2007). Specifically, we used patent applications within each three-year window to construct the collaboration network, and the collaboration network serves as input to analyse inventors’ triadic closure and predict invention quality. Similar archival approaches have used five-year windows, but we found no substantive differences in window size and chose the smaller size to maximise observations.
illustrates the construction of the inventor collaboration network, which follows the standard practice in the literature on inventor collaboration (Fleming, Mingo, and Chen Citation2007). Each inventor is linked to a patent that they have co-invented. As shown in , as inventors A, B, and C are listed as co-inventors on patent i, they have collaborative ties with each other in the inventor network. Thus, each three-year period leads to one whole network in which inventors of one single patent form a fully linked clique, and inventors of multiple patents are linked to several other cliques. This approach leads to the collaboration network. Then, we utilised the igraph package in R to obtain structural measures of the inventor collaboration networks.
The inventor collaboration network is further analysed to obtain a set of inventor triads. We obtain open triads by identifying inventor brokers. Inventor brokers collaborate with two alters while there is no direct tie between the alters. As shown in , inventor C has collaborated with B on patent i and has collaborated with D on patent j. As B and D have not collaborated on any patents, C is a broker between B and D forming an open triad relation. Then, we compared the open triad in the prior three-year period with the following three-year period to see the extent to which the open triad becomes closed or remains open. For example, we examined to what extent the alter inventors in an open triad relationship in the period 1987–1989 would collaborate in a closed triad manner in 1988–1990, as well as how this predicts the forward citation of the focal inventor triad in 1990 (‘invention quality’). As shown in , if inventors B and D are listed as co-inventors on a patent in the next period, the open triad relation becomes a closed triad. Hence, our subset sample involves inventors who are involved in open triad relations in the network. Additionally, these inventors should have been patented in the year t to measure invention quality.
The final sample contains fewer cases of open triads that turn into closed triads than those that remain open. Specifically, our full sample consists of 20,849 open triad cases that become closed triads, and 319,810 cases that remain open triads. At this time, we implement CEM to create a matched treatment sample and a control sample. We followed prior research and matched on a subset of key covariates strongly affecting inventor innovation quality. The pretreatment covariates are triad-level inventor characteristics representing multiple attributes of all three inventors in a triad relation. Previous studies used attributes such as an inventor’s city, assignee, patent technology classes, citations, and past performance to match inventors (Fleming, Mingo, and Chen Citation2007). An inventor’s past patent performance, knowledge diversity, and tenure are some of the matching criteria most related to an inventor’s innovation capacity (Lee Citation2010). Therefore, we selected triad-level past performance, knowledge diversity, and tenure as pretreatment covariates. We measure past performance by counting the number of patents in a three-year rolling window. Knowledge diversity is computed using the Herfindahl index of knowledge diversification. Finally, tenure is computed as the average number of years since the first patent is applied by the inventors. The CEM process allows us to compare similar, initially open triads that turn into a closed triad with triads that remain open (as shown in ). The triads in the control group (1) have generated approximately the same number of patents in the previous three years, (2) exhibit approximately the same extent of knowledge diversity in their previous patenting, and (3) have similar tenure years as triads in the control group. The summary of the matched and control sample is in . By merging the treated and control groups, we obtained a dataset of 41,698 cases.
Table 1. Descriptive analysis of treated and control group.
4.3 Measures
4.3.1. Dependent variable
Our theory aims to predict the quality of the patented inventions generated by the inventor triad (Invention quality). Note that we consider the sum of generated patents, as all three inventors may jointly or individually benefit from the collaborative structural context in which they work. At least one of the inventors is involved in the patents considered. We utilise the inventive quality of the patent as a proxy for the inventive performance of patents generated by the triad. Previous work suggests that forward citation count is an appropriate indicator of the significance and quality of inventions and can be used to measure the (future) invention quality of inventors (Fleming Citation2001; Jaffe, Trajtenberg, and Henderson Citation1993; Lee Citation2010; H. Singh et al. Citation2016). Thus, the key dependent variable in our study is the future quality of the patents invented by the triad, measured as the citation-weighted patent count invented by the three inventors involved in the triad relation in the observation year (Hall, Jaffe, and Trajtenberg Citation2001; Katila Citation2000). In our study, the citation-weighted patent count is measured by adding citations to the patent counts, i.e. citations that the focal patent receives in subsequent patents (Katila Citation2000). These patents include the patents individually or collectively filed by the three inventors. For example, if an inventor has one patent and it received 10 citations, then this patent is counted as 11 patents. In this way, our measurement can reflect the quality of the invention. To address the truncation problem of the citation-weighted patent count, we only include citations in the following three years after the grant year of the patent. Self-citations are excluded from this data. We also used patent citations up to now and adjusted the citation-weighted patent count by the group size as an alternative measure of invention quality.
4.3.2. Independent variables
Our main independent variable compares open triads that remain open with open triads that turn into a closed triad, which we obtain through the matching process. As a result, our independent variable is a binary variable with 1 representing triads that become closed triads (closed triad), and 0 representing triads that remain open.
The second independent variable in our model is the connectedness of the inventor triad (connectedness). To measure to what extent the three inventors are linked to more or less central actors in the network, we used Bonacich’s (Citation1972) eigenvector centrality measure to represent the alters’ degree of connectedness. We chose eigenvector centrality measure as it captures the extent to which an inventor is directly or indirectly connected to more central inventors in the network, representing the information volume inventors receive. Highly connected inventors mean more direct ties and their direct ties are connected to other highly connected actors in the network (i.e. indirect ties). We think that eigenvector centrality reflects the level of connectedness taking into account the inventor triad’s first, second, and third levels of collaborators and is a proper measurement to measure the connectedness of the inventor triad. Bonacich’s eigenvector centrality measure (Bonacich Citation1987) quantifies the extent actors are connected to other central actors. In general, vertices with high eigenvector centralities are those connected to many other vertices, which are, in turn, connected to many others (and so on). For a given graph G with V vertices, let A=(a_(v,t)) be the adjacency matrix and the relative eigenvector centrality of vertex v can be defined as:
Where M(v) is a set of neighbours of v and λ is the largest eigenvalue of the adjacency matrix. It is rewritten as:
We relied on the biotechnology industry’s overall inventor network to calculate each inventor’s eigenvector centrality. Then, we summed up the eigenvector measures of the three inventors involved in the triad relation and divided them by 3 to obtain the average value.
4.3.3. Control variables
We controlled for other network features that might influence triad invention quality. Previous studies have pointed to the importance of individual brokerage behaviour for inventor performance (Fleming, Mingo, and Chen Citation2007). By connecting otherwise disconnected actors, inventors are in an advantageous position to control and access heterogeneous knowledge (Ahuja Citation2000; Burt Citation2004), which might influence triad-level invention quality. To address this possible effect on triad invention quality, we controlled for the average of the three inventors’ ego-network brokerage measure (brokerage) based on Burt’s measure of constraints subtracted from 1. The formula of Burt’s constraint measure (Burt Citation2004) is:
Where C_i is the network constraint of inventor i, and p_ij is the proportion of time and energy that inventor i invested in inventor j compared to all the network ties that have formed (p_iq and p_qj are defined analogously). The proportional tie strengths p_ij (p_iq and p_qj analogously) are defined as:
a_ij is an element of adjacency matrix A. Lower values on this measure imply that inventors occupy less constrained positions, thereby brokering more extensively in the network. For isolated vertices, the constraint is undefined. We transformed the network constraint by subtracting it from 1 so that higher values indicate higher brokerage levels (Lee Citation2010). We controlled for inventor brokerage in the collaboration network constructed for the previous three years. The average of the three inventors’ ego-network density (density) is also included as a triad control variable. The network density for an ego inventor is given by the proportion of links between the ego’s alters divided by the number of links that could theoretically exist between them. Prior research on inventor networks has shown that ego network density decreases creativity but increases future use of inventions (Fleming, Mingo, and Chen Citation2007). Besides, we also controlled for the average degree centrality of the three inventors involved in the triad (centrality). Note that network variables at the triad level are obtained by summing up each of the measures for the three inventors involved in the triad and dividing by three.
We also included several other triad-level controls. Past performance (past performance) controls for the patent stock of the three inventors before the observation year to account for triad-level inventive capability differences. In our study, we used patent counts of the inventors in the open triad relation accumulated in the previous three-year period to measure past performance. This includes patents invented by each of the three inventors and co-patented by two or three inventors. We did not use patent count to t-1 as this measure is often skewed. We checked the regression results using both measures, which do not differ. Knowledge diversity (diversity) controls for a triad’s knowledge breadth. Previous studies found that heterogeneous knowledge contributes to inventive impact, independent of network position. Triads with a broad knowledge base may find unique or new combinations of technologies that lead to higher invention quality (Fleming Citation2001). We used the Herfindahl index of knowledge diversification (Garcia-Vega Citation2006) derived from the Herfindahl-Hirschman Index (HHI) to proxy for a triad’s heterogeneous knowledge. The Herfindahl index of knowledge diversification can be expressed as follows:
where Pi denotes the proportion of patents invented by three inventors in the triad relation in the previous three years in patent class i. These patents include patents invented by the inventor triad and co-patented by two or three of the inventors. For example, if inventors A, B, and C in the open triad relation have two patents and three co-patented patents, we merged these five patents and measure their distribution over different IPC classes. The index equals zero when the three inventors patent only in a single knowledge domain, and it is close to one when they patent in a broad knowledge domain. Tenure (tenure) controls for inventor experience. Previous studies show that inventors’ experience might influence their invention output (Fleming, Mingo, and Chen Citation2007). Tenure is measured by the year difference between the earliest years that the inventor has patented and the observation years and averaged over the three inventors. The tie between brokers and alters may appear several times on different triads. To accommodate this effect, we included the control variable of tie strength, which captures the sum of the number of times the broker and alters collaborated during the three-year period before the observation year. For example, in triad B-C-D, if B-C tie appeared twice on triad relations, and C-D appeared once on triad relations during the three-time window before the observation year, then the measure of tie strength is 3. We also control for whether the inventor triad is formed with inventors from the same firm or different firms using the control variable of a cross-firm triad. The dummy variable of the cross-firm triad is 1 when the inventors of the triad relation are from different firms, and 0 when the inventors are all from the same firm.
Although our level of analysis is triad-level, we also included firm-level attributes to control for firm effects. Observations across triads are not independent because inventors of the same firm are related. To account for this concern, we included firm-level past innovative performance (firm past performance), geographic distribution of firm inventors (geographic distribution), firm alliance number (alliance), and firm turnover (turnover) as control variables. If the triad involves inventors from different firms, we take the average of the firm-level measures. A firm’s past innovative performance is related to triad-level invention quality, as inventors in a highly innovative firm may have a higher probability of participating in close collaboration and producing high-quality inventions. Firm past invention performance is measured using a firm’s accumulated patent count up to the observation year. Firm inventor geographic distribution captures the extent to which the firm sources knowledge from different geographic locations (Tóth et al. Citation2021), which is also related to the firm’s potential to produce high-quality inventions (Lahiri Citation2010). The geographic distribution of inventors of a firm is obtained using the Herfindahl index of geographic distribution which is derived from the Herfindahl-Hirschman Index (HHI) (Garcia-Vega Citation2006). The Herfindahl index of geographic distribution can be expressed as follows:
where Pi denotes the proportion of patents invented by a firm in the previous three years in city i. For patents associated with more than one city, we view the first city appearing on the patent as the location it is invented. We also controlled for the number of alliances announced by the firm in the previous five years. We obtained the alliance data from the SDC Platinum and matched the alliance data with the sample firms using their cusips. According to the previous research, inventor turnover of a firm provides a channel for new knowledge, thus may influence a firm’s innovation process. To accommodate this effect, we controlled for a firm’s turnover. Firm turnover is measured by the total number of inter-firm inventor mobility events (new recruits and inventors leaving the firm) that happened during the previous five years. Based on the previous research, we tracked the patents to identify instances where a researcher was listed as an inventor in patents granted to two different firms (Corredoira and Rosenkopf Citation2010; Wagner and Goossen Citation2018). We excluded instances where two firms applied for the same patent since it is assumed that these patents derive from the research cooperation between these two firms. Then, we counted the inventor turnover events that occurred in the five-year window preceding the year of observation for each firm to obtain the measure of turnover. We also measured the firm-level network size, i.e. the number of inventors of the firm to capture the network size the triad is embedded in. The measure of firm network size is highly correlated with the measure of firm past performance. Therefore, we did not include this measure in the model. Triad- and firm-level fixed effects make our analyses a firm- and triad-level fixed-effect model. The results now represent the change in the dependent variable with the changes in the level of independent variables for triads and firms with similar characteristics. Finally, year dummies were included to control for time-fixed effects. The description of the variables is presented in .
Table 2. Measurement of variables.
4.4. Estimation method
The dependent variable in our study is the citation-weighted patent count of the triad. We included the natural logarithm of the measure in the regression model and used an OLS (Ordinary Least Squares) model two-way clustered across triad and firm. This model can control for the triad and firm-level fixed effects. We logged the variables of past performance, firm past performance, and centrality before including them in the model. In the results, heteroskedasticity-robust standard errors clustered at triad and firm levels are reported.
5. Results
provides the descriptive statistics including means, standard deviations, minimum values, maximum values, and VIF values for all the variables. Our sample included 41,698 cases. As a diagnostic test, we performed variance inflation factors (VIF) for each variable. The maximum VIF is below the generally accepted threshold of 10 (O’Brien Citation2007). shows the correlation between variables. Multicollinearity is not an issue.
Table 3. Descriptive statistics of variables.
Table 4. Correlation among the variables.
displays the main regression results of our estimations. In Model 1, control variables are included. As shown in Model 1, triad-level density, past performance, tie strength, cross-firm triad, and firm-level inventor geographic dispersion are positively related to triad invention quality. Triad-level centrality and technological diversity have negative effects on triad invention quality. In Model 2, the main independent variable, closed triad, is added. Model 2 shows a positive effect (β = 0.071, p-value <0.05) of a triad becoming closed on triad invention quality. The coefficients of other controls remained similar. In terms of marginal effect, the coefficient implies that for a one-unit change in closed triads, the difference in the logs of expected counts of the innovation quality is 0.071, given the other predictor variables in the model are held constant. Analogous results were obtained when we measured the citation-weighted patent counts using forward citations up to now, adjusted by group size.
Table 5. Regression results of OLS models with invention quality (3-year citation-weighted patent count) as DV.
In Model 3, the connectedness of the triad is added. As shown, connectedness is negatively related to the triad invention quality. Model 4 includes the interaction term of closed triad and connectedness. We compute the marginal effects and visualise the moderation effect. Overall, the marginal effect of the interaction between closed triad and connectedness is negative and statistically significant, supporting our moderation hypothesis. A summary is provided in and the accompanying graphs.
Table 6. Marginal effects of closed triad.
shows the average marginal effect and margins of closed triad on invention quality. The table reads as follows. The average marginal effect of closed triad is 1.72. It means that on average, the case of a dynamic closed triad effect, compared to the case of open triad, increases the invention quality by 1.72. The margins also show that the margins of open triad are 7.85, while the margins of closed triad are 9.58. shows the comparison of margins of open triad and closed triad.
compares the margins of open and closed triads at different levels of connectedness. Connectedness takes the value of one standard deviation below the mean (−0.05) to one standard deviation above the mean (0.08). The figure shows that as connectedness increases, the level of invention quality decreases, indicating that connectedness negatively moderates the main effect.
Figure 4. The predictive margins of closed triad on triad invention quality at different levels of connectedness.
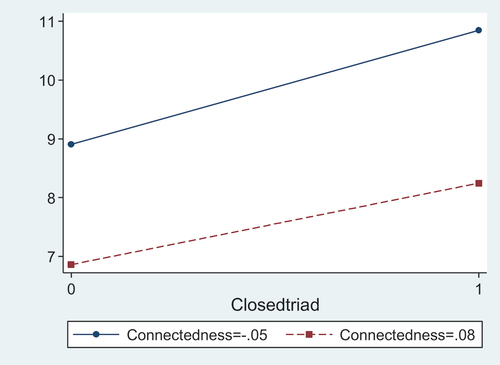
In , we plot the conditional effect of closed triad at each observed value of connectedness using the R interplot package (Solt and Hu Citation2015). The solid line represents coefficients of closed triad on invention quality for all values of connectedness estimated from the data and the model. The shaded region represents the 95% confidence intervals of these estimates. This plot reveals that closed triad’s estimated marginal effects on invention quality are positive and statistically significant for those with connectedness values up to 0.3. They are not distinguishable from zero for those with higher connectedness values. In our sample, about 90% of connectedness values lie between 0 ~ 0.2. It means that at most of the observed values of connectedness, closed triad has a positive effect on invention quality. Also, as connectedness increases, the coefficient of closed triad on invention quality decreases and when the connectedness reaches a high level, closed triad may even have a negative effect on invention quality. Thus, connectedness has a negative moderation effect on the relationship between closed triad and invention quality.
6. Robustness check
We utilised an alternative measure of the dependent variable in the robustness check. When measuring the citation-weighted patent count, instead of using patents that the three inventors independently or jointly invented, we utilised patents jointly invented by the three inventors as our dependent variable. The regression results are displayed in . The results show similar effects of the independent variables.
Table 7. Regression results of OLS models with invention quality (measured with patents jointly applied by triad inventors) as DV.
7. Discussion and conclusion
In this study, we specifically examined to what extent and why triadic closure is related to invention quality at the triadic level. We built on the literature on brokering behaviour, the recombinant view on innovation, and small group synergy to propose those open triads that turn into a closed triad – connecting previously unconnected inventor alters – generate higher-quality inventions compared to open triads that maintain separated alters. We asserted that triadic closure over time benefits triad outcomes by initially providing proprietary access to diverse and non-redundant knowledge resources and deep knowledge exchange to generate new ideas and subsequently mobilising and coordinating the resources of their connections to implement and diffuse inventions. We also examined the extent to which the connectedness of the triad influences the relationship between triad closure dynamics and the generation of high-quality inventions.
Our study has important theoretical implications. We challenge existing work on brokerage and brokering by taking a collective triadic perspective. For instance, Soda, Tortoriello, and Iorio (Citation2018) find that employees who combine a brokerage position with a tertius gaudens orientation perform better. In another (ethnographic) study, Kellogg (Citation2014) shows that brokerage practices – maintaining disconnected alters – benefit healthcare professionals by making them indispensable as managers of information. While brokerage-gaudens pairing might be helpful in certain professions, as they benefit the individual broker with unique information and control benefits, we find the opposite in the context of innovative performance at the triad level. One possible way to explain this difference is the importance of recombining knowledge and information, which is the nature of the tasks conducted in the innovation project (Patrucco et al. Citation2022). If, for example, the invention is characterised by a high level of interdependence between its components (complex) and asks for, for example, intensive problem solving, information search, and learning, triadic closure is preferred over an open triad, as the former allows for tighter control, higher cohesion levels, and the production of synergy. Another reason for our divergent findings is that we study state changes of triadic networks and relate this to triad-level outcomes, whereas the vast majority of brokerage studies focused on individual (broker) or firm-level outcomes. This supports the notion that at different levels of analysis, different mechanisms produce distinct outcomes. We also challenge the idea that open triads are mere catalysts that ‘alter the interaction among other actors and are minimally affected by the interaction’ (Stovel and Shaw Citation2012, 146). Instead, our study shows that open triads that unite initially unconnected alters enjoy tangible gains – in terms of superior inventive performance.
We also contribute to the network and innovation literature (Fleming, Mingo, and Chen Citation2007) by infusing our understanding of triads and innovation with insights from the literature on small group synergy (Kurtzberg and Amabile Citation2001) and collective intelligence (Woolley, Aggarwal, and Malone Citation2015). A few studies have examined triads in the context of innovation (e.g. Soda et al. Citation2021), but the theoretical precision of the small group synergy literature supports more fine-grained theoretical notions of why certain tasks are more productive in open triads that close over time versus triads that remain open. In particular, we highlight processes key to invention quality, that is, idea generation, problem-solving, and learning processes, and argue that initial open triads that close over time benefit weak and strong synergy effects among inventors. Combining the small groups literature with triadic network literature provides a deeper understanding of networks and collective outcomes.
This study also adds to existing work on networks that takes an alter-centric approach (Grosser, Venkataramani, and Labianca Citation2017; Kleinbaum, Jordan, and Audia Citation2015; Lin Citation20022). The prior literature on networks in the context of invention has primarily focused on ego network structure and less on the alters’ individual characteristics and network ties. With our findings, we join an ongoing conversation on how external indirect ties influence the innovation performance of a social actor. For instance, Grosser, Venkataramani, and Labianca (Citation2017) show how the innovative behaviour and network density of the alter influence the innovation behaviour of an ego. In another study, H. Singh et al. (Citation2016) studied the value of the knowledge obtained from direct versus indirect contacts (through the alters). We provide evidence of the importance of the connectedness of the triad. Despite triadic closure, the triad’s well-connectedness might overload the processing capability of the triad in such a way that it decreases its ability to generate high-quality inventions.
Our triad-level study contributes to the literature on networks and innovation (Allen and Cohen Citation1969; Baer et al. Citation2015; Obstfeld Citation2005; Perry-Smith and Mannucci Citation2017; Reagans and McEvily Citation2003). Two existing studies are pertinent to assess our contribution in the context of inventor brokerage. In a cross-industry study, Fleming, Mingo, and Chen (Citation2007) illustrate how inventors that span structural holes in collaboration networks generate new combinations of knowledge, while inventors located in cohesive sub-networks are more likely to generate useful inventions. We extend this line of reasoning by showing how inventor triads close over time, combining the benefits of brokerage and closure at the triadic level in a dynamic fashion. Our findings nuance the results of Lee (Citation2010), who found that the brokerage-performance relationship disappears when including fixed effects. In our estimations of a matched sample of open triads that close versus remain open, we demonstrate how triadic closure is superior to remaining open. Our focus on inventor triads complements existing work on networks of R&D personnel and their individual invention capabilities (Carnabuci and Diószegi Citation2015; Fleming, Mingo, and Chen Citation2007; Nerkar and Paruchuri Citation2005; Tortoriello Citation2015).
8. Limitations and future research
This study is not without limitations. For instance, we cannot identify who exactly is responsible for establishing connections between alters. We can only proxy this as a state change of a triad, which implies connecting behaviour by at least one of the inventors in the triad. The existing research on brokering behaviour places a disproportionate focus on the broker’s role in uniting behaviour (Halevy, Halali, and Zlatev Citation2018; Zhelyazkov Citation2018). However, we do not know whether the broker promotes collaboration among alter inventors or whether alternative channels, such as alters’ strategic orientation or behaviour, facilitate closure. After all, having a shared intermediary provides both alters with detailed information about that common partner’s availability, suitability, and reliability (Obstfeld Citation2005) and might trigger strategic ‘tertius iungens’ behaviour. There also are indications that collaboration history in the form of prior ties in the past is an important antecedent of triadic closure (Ter Wal Citation2014). Furthermore, Soda et al. (Citation2021) show that knowledge differences among the broker and alters encourage triadic closure. These different determinants of triadic closure might result in different processes (e.g. trust building, knowledge sharing) impacting levels of synergy and collective outcomes.
Our study also suffers from using co-invention data, which is common in patent-based studies (e.g. Fleming, Mingo, and Chen Citation2007). Co-invention data can only proxy for the collaborative behaviour of inventors. Moreover, to identify the co-patenting behaviour of inventors, they need to patent regularly, thus, we zoom in on a subset of productive inventors.
Building on cognitive (over) load theory, we hypothesised and empirically found a negative moderation effect of triad connectedness. However, one can further develop a complementary interpretation of this effect, which is different from our information processing argument. As we mentioned, triad connectedness might also proxy for access to relatively mature knowledge and is subject to and limited by a firm’s dominant logic. Thus, the negative moderation effect could result from the type of knowledge the well-connected triad has access to. For example, Dong and Yang (Citation2016) argue that such actors, due to their many ties, predominantly have access to what they call mature knowledge. This more mature knowledge is well-established and often less valuable for recombinant efforts aiming to identify new technological opportunities. A related argument is developed and tested by van de Kaa (Citation2018), who maintains that well-connected actors can exert more influence on other actors in the network and can spread information to other actors more easily. Through this influence, these actors can force standards (van de Kaa Citation2018) or dominant designs (Brem, Nylund, and Schuster Citation2016) on less influential network actors, inhibiting their innovation quality because these standards and designs build on more established knowledge. Our study does not, however, allow us to disentangle the underlying reasons.
We identify three promising areas for future research. Future research could examine how the strength of tie configuration in triads impacts innovation outcomes. Moon, Di Benedetto, and Kim (Citation2022) study shows, for example, that a closed triad with one strong tie between the focal actor and an alter, weak ties between the two alters, and a weak tie between the focal unit and the second alter, outperforms a closed triad with only strong ties between alters. How do innovation outcomes pan out if we take the strength of ties configuration of open triads into the equation? To what extent would open and closed triads that are composed of different configurations of uniplex and multiplex ties impact invention quality and other innovation outcomes (Ferriani, Fonti, and Corrado Citation2013)? Second, we invite scholars to study the so-called network oscillation in which individuals might embed themselves in closed groups during some period, followed by periods of connecting across social domains (Burt and Merluzzi Citation2016). We acknowledge Burt and Merluzzi’s (Citation2016) call for future research on the mechanisms that drive such network oscillation. For instance, in the context of innovation, individuals or even triads that oscillate between dense and sparse networks might influence their ability to engage in exploitative versus exploratory innovation behaviour (March Citation1991; Paruchuri and Awate Citation2017). Finally, our study raises questions regarding the processes, behaviour, and outcomes of inventor triad collaboration. For instance, the brokering behaviour of ego brokers and their roles may shape alters’ innovation outcomes. Generating symmetric networks might influence the broker’s ability to generate new combinations and marshal resources and may also help or harm the alters. As we study overall triad outcomes, this is an interesting avenue for future research, connecting individual- and triad-level literature.
In conclusion, this study demonstrates the importance of a dynamic view of triad closure when investigating how collaboration networks among inventors impact R&D-based invention. We encourage future research to continue to study how triads and their behaviour influence invention outcomes.
Acknowledgements
The authors gratefully acknowledge the feedback of participants of SWG 7 on networks at the European Group for Organizational Studies (EGOS) Colloquium and the Danish Research Unit on Industrial Dynamics (DRUID) Conference. The paper benefitted greatly from the valuable guidance from three anonymous reviewers and the I&I handling editor, Holmer Kok. We also like to thank Nicoleta Meslec from Tilburg University for helping us to navigate the small group literature. All errors remain our own.
Disclosure statement
No potential conflict of interest was reported by the author(s).
Notes
1 Only one exception exists: Soda et al. (Citation2021) show in a supplementary analysis in the appendix of their study that inventor triads that remain open positively influence’s the ability of the triad to explore new knowledge areas.
2 SIC 2833 (medicinal chemicals), SIC 2834 (pharmaceutical preparations), SIC 2835 (in vitro/in vivo diagnostics).
SIC 2836 (biological products), SIC 5122 (drugs and proprietary)
References
- Aggarwal, V. A., D. H. Hsu, and A. Wu. 2020. “Organizing Knowledge Production Teams within Firms for Innovation.” Strategy Science 5 (1): 1–16. https://doi.org/10.1287/stsc.2019.0095.
- Ahuja, G. 2000. “Collaboration Networks, Structural Holes, and Innovation: A Longitudinal Study.” Administrative Science Quarterly 45 (3): 425–455. https://doi.org/10.2307/2667105.
- Allen, T. J., and S. K. Cohen. 1969. “Information Flow in Research and Development Laboratories.” Administrative Science Quarterly 14 (1): 12–19. https://doi.org/10.2307/2391357.
- Amon, M. J., H. Vrzakova, and S. K. D’Mello. 2019. “Beyond Dyadic Coordination: Multimodal Behavioral Irregularity in Triads Predicts Facets of Collaborative Problem Solving.” Cognitive Science 43 (10): e12787. https://doi.org/10.1111/cogs.12787.
- Arthur, W. B. 2007. “The Structure of Invention.” Research Policy 36 (2): 274–287. https://doi.org/10.1016/j.respol.2006.11.005.
- Baer, M., K. Evans, G. R. Oldham, and A. Boasso. 2015. “The Social Network Side of Individual Innovation: A Meta-Analysis and Path-Analytic Integration.” Organizational Psychology Review 5 (3): 191–223. https://doi.org/10.1177/2041386614564105.
- Blackwell, M., I. Stefano, K. Gary, and P. Giuseppe. 2009. “CEM: Coarsened Exact Matching in Stata.” The Stata Journal: Promoting Communications on Statistics & Stata 9 (4): 524–546. https://doi.org/10.1177/1536867X0900900402.
- Bonacich, P. 1972. “Factoring and Weighting Approaches to Status Scores and Clique Identification.” Journal of Mathematical Sociology 2 (1): 113–120. https://doi.org/10.1080/0022250X.1972.9989806.
- Bonacich, P. 1987. “Power and Centrality: A Family of Measures.” The American Journal of Sociology 92 (5): 1170–1182. https://doi.org/10.1086/228631.
- Brem, A., P. A. Nylund, and G. Schuster. 2016. “Innovation and de Facto Standardization: The Influence of Dominant Design on Innovative Performance, Radical Innovation, and Process Innovation.” Technovation 50–51:79–88. https://doi.org/10.1016/j.technovation.2015.11.002.
- Breschi, S., and C. Lenzi. 2017. “The Role of External Linkages and Gatekeepers for the Renewal and Expansion of US Cities’ Knowledge Base, 1990–2004.” In Evolutionary Economic Geography (pp. 78–93). Routledge.
- Burt, R. S. 1992. Structure Holes. Cambridge, MA: Harvard University Press.
- Burt, R. S. 2004. “Structural Holes and Good Ideas.” The American Journal of Sociology 110 (2): 349–399. https://doi.org/10.1086/421787.
- Burt, R. S., and J. Merluzzi. 2016. “Network Oscillation.” Academy of Management Discoveries 2 (4): 368–391. https://doi.org/10.5465/amd.2015.0108.
- Burt, R. S., S. Opper, and H. J. Holm. 2022. “Cooperation Beyond the Network.” Organization Science 33 (2): 495–517. https://doi.org/10.1287/orsc.2021.1460.
- Carnabuci, G., and B. Diószegi. 2015. “Social Networks, Cognitive Style, and Innovative Performance: A Contingency Perspective.” Academy of Management Journal 58 (3): 881–905. https://doi.org/10.5465/amj.2013.1042.
- Carnabuci, G., and E. Operti. 2013. “Where Do Firms’ Recombinant Capabilities Come From? Intraorganizational Networks, Knowledge, and Firms’ Ability to Innovate Through Technological Recombination.” Strategic Management Journal 34 (13): 1591–1613. https://doi.org/10.1002/smj.2084.
- Chai, K. H., C. M. Yap, and X. Wang. 2011. “Network closure’s Impact on firms’ Competitive Advantage: The Mediating Roles of Knowledge Processes.” Journal of Engineering and Technology Management 28 (1–2): 2–22. https://doi.org/10.1016/j.jengtecman.2010.12.001.
- Coleman, J. S. 1988. “Social Capital in the Creation of Human Capital.” The American Journal of Sociology 94:95–121. https://doi.org/10.1086/228943.
- Corredoira, R., and L. Rosenkopf. 2010. “Should Auld Acquaintance Be Forgot? The Reverse Transfer of Knowledge Through Mobility Ties.” Strategic Management Journal 31 (2): 159–181. https://doi.org/10.1002/smj.803.
- Devarakonda, R., S. Pavićević, and J. J. Reuer. 2022. “Microfoundations of R&D Alliance Formation: The Interplay of Scientist Mobility and the Cooperative Context of Collaboration.” Strategy Science 7 (1): 56–70. https://doi.org/10.1287/stsc.2021.0127.
- Diehl, M., and W. Stroebe. 1987. “Productivity Loss in Brainstorming Groups: Toward the Solution of a Riddle.” Journal of Personality & Social Psychology 53 (3): 497–509. https://doi.org/10.1037/0022-3514.53.3.497.
- Dong, J. Q., and C. H. Yang. 2016. “Being Central is a Double-Edged Sword: Knowledge Network Centrality and New Product Development in U.S. Pharmaceutical Industry.” Technological Forecasting & Social Change 113:379–385. https://doi.org/10.1016/j.techfore.2016.07.011.
- Dugosh, K. L., P. B. Paulus, E. J. Roland, and H. C. Yang. 2000. “Cognitive Stimulation in Brainstorming.” Journal of Personality & Social Psychology 79 (5): 722. https://doi.org/10.1037/0022-3514.79.5.722.
- Edmunds, A., and A. Morris. 2000. “The Problem of Information Overload in Business Organisations: A Review of the Literature.” International Journal of Information Management 20 (1): 17–28. https://doi.org/10.1016/S0268-4012(99)00051-1.
- Ferriani, S., F. Fonti, and R. Corrado. 2013. “The Social and Economic Bases of Network Multiplexity: Exploring the Emergence of Multiplex Ties.” Strategic Organization 11 (1): 7–34. https://doi.org/10.1177/1476127012461576.
- Fleming, L. 2001. “Recombinant Uncertainty in Technological Search.” Management Science 47 (1): 117–132. https://doi.org/10.1287/mnsc.47.1.117.10671.
- Fleming, L., S. Mingo, and D. Chen. 2007. “Collaborative Brokerage, Generative Creativity, and Creative Success.” Administrative Science Quarterly 52 (3): 443–475. https://doi.org/10.2189/asqu.52.3.443.
- Fleming, L., and O. Sorenson. 2004. “Science As a Map in Technological Search.” Strategic Management Journal 25 (8–9): 909–928. https://doi.org/10.1002/smj.384.
- Fritsch, M., M. Titze, and M. Piontek. 2020. “Identifying Cooperation for Innovation―A Comparison of Data Sources.” Industry & Innovation 27 (6): 630–659. https://doi.org/10.1080/13662716.2019.1650253.
- Garcia-Vega, M. 2006. “Does Technological Diversification Promote Innovation?: An Empirical Analysis for European Firms.” Research Policy 35 (2): 230–246. https://doi.org/10.1016/j.respol.2005.09.006.
- George, G., S. A. Zahra, K. K. Wheatley, and R. Khan. 2001. “The Effects of Alliance Portfolio Characteristics and Absorptive Capacity on Performance: A Study of Biotechnology Firms.” Journal of High Technology Management Research 12 (2): 205–226. https://doi.org/10.1016/S1047-8310(01)00037-2.
- Gómez‐Solórzano, M., M. Tortoriello, and G. Soda. 2019. “Instrumental and Affective Ties within the Laboratory: The Impact of Informal Cliques on Innovative Productivity.” Strategic Management Journal 40 (10): 1593–1609. https://doi.org/10.1002/smj.3045.
- Grant, A. M., and J. W. Berry. 2011. “The Necessity of Others Is the Mother of Invention: Intrinsic and Prosocial Motivations, Perspective Taking, and Creativity.” Academy of Management Journal 54 (1): 73–96. https://doi.org/10.5465/amj.2011.59215085.
- Grosser, T. J., D. Obstfeld, G. Labianca, and S. P. Borgatti. 2018. “Measuring Mediation and Separation Brokerage Orientations: A Further Step Toward Studying the Social Network Brokerage Process.” Academy of Management Discoveries 5 (2): 114–136. https://doi.org/10.5465/amd.2017.0110.
- Grosser, T. J., V. Venkataramani, and G. Labianca. 2017. “An Alter-Centric Perspective on Employee Innovation: The Importance of Alters’ Creative Self-Efficacy and Network Structure.” Journal of Applied Psychology 102 (9): 1360–1374. https://doi.org/10.1037/apl0000220.
- Guler, I., and A. Nerkar. 2012. “The Impact of Global and Local Cohesion on Innovation in the Pharmaceutical Industry.” Strategic Management Journal 33 (5): 535–549. https://doi.org/10.1002/smj.957.
- Halevy, N., E. Halali, and J. J. Zlatev. 2018. “Brokerage and Brokering: An Integrative Review and Organizing Framework for Third Party Influence.” The Academy of Management Annals 13 (1): 215–239. https://doi.org/10.5465/annals.2017.0024.
- Hall, B. H., A. Jaffe, and M. Trajtenberg. 2001. “The NBER Patent Citation Data File: Lessons, Insights and Methodological Tools.” NBER Working Paper 8498. Cambridge, MA: National Bureau of Economic Research.
- Hargadon, A. B. 2002. “Brokering Knowledge: Linking Learning and Innovation.” Research in Organizational Behavior 24:41–85. https://doi.org/10.1016/S0191-3085(02)24003-4.
- Hargadon, A., and R. I. Sutton. 1997. “Technology Brokering and Innovation in a Product Development Firm.” Administrative Science Quarterly 42 (4): 716–749. https://doi.org/10.2307/2393655.
- Jackson, T. W., and P. Farzaneh. 2012. “Theory-Based Model of Factors Affecting Information Overload.” International Journal of Information Management 32 (6): 523–532. https://doi.org/10.1016/j.ijinfomgt.2012.04.006.
- Jaffe, A. B., M. Trajtenberg, and R. Henderson. 1993. “Geographic Localization of Knowledge Spillovers As Evidenced by Patent Citations.” Quarterly Journal of Economics 108 (3): 577–598. https://doi.org/10.2307/2118401.
- Janssens, M., N. Meslec, and R. T. A. Leenders. 2022. “Collective Intelligence in Teams: Contextualizing Collective Intelligent Behavior Over Time.” Frontiers in Psychology 13:989572. https://doi.org/10.3389/fpsyg.2022.989572.
- Jones, B. F. 2009. “The Burden of Knowledge and the ‘Death of the Renaissance Man’: Is Innovation Getting Harder?” “Review of Economic Studies 76 (1): 283–317. https://doi.org/10.1111/j.1467-937X.2008.00531.x.
- Katila, R. 2000. “Using Patent Data to Measure Innovation Performance.” International Journal of Business Performance Management 2 (1/2/3): 180–193. https://doi.org/10.1504/IJBPM.2000.000072.
- Kauppila, O. P., L. Bizzi, and D. Obstfeld. 2018. “Connecting and Creating: Tertius Iungens, Individual Creativity, and Strategic Decision Processes.” Strategic Management Journal 39 (3): 697–719. https://doi.org/10.1002/smj.2728.
- Kellogg, K. C. 2014. “Brokerage Professions and Implementing Reform in an Age of Experts.” American Sociological Review 79 (5): 912–941. https://doi.org/10.1177/0003122414544734.
- Kirschner, P. A., J. Sweller, F. Kirschner, and R. J. Zambrano. 2018. “From Cognitive Load Theory to Collaborative Cognitive Load Theory.” International Journal of Computer-Supported Collaborative Learning 13 (2): 213–233. https://doi.org/10.1007/s11412-018-9277-y.
- Kleinbaum, A. M., A. H. Jordan, and P. G. Audia. 2015. “An Altercentric Perspective on the Origins of Brokerage in Social Networks: How Perceived Empathy Moderates the Self-Monitoring Effect.” Organization Science 26 (4): 1226–1242. https://doi.org/10.1287/orsc.2014.0961.
- Kurtzberg, T. R., and T. M. Amabile. 2001. “From Guilford to Creative Synergy: Opening the Black Box of Team-Level Creativity.” Creativity Research Journal 13 (3–4): 285–294. https://doi.org/10.1207/S15326934CRJ1334_06.
- Kwon, S. W., E. Rondi, D. Z. Levin, A. D. Massis, and B. Dan. 2020. “Network Brokerage: An Integrative Review and Future Research Agenda.” Journal of Management 46 (6): 1092–1120. https://doi.org/10.1177/0149206320914694.
- Lahiri, N. 2010. “Geographic Distribution of R&D Activity: How Does it Affect Innovation Quality?.” Academy of Management Journal 53 (5): 1194–1209.
- Lanjouw, J. O., and M. Schankerman. 2004. “Patent Quality and Research Productivity: Measuring Innovation with Multiple Indicators.” The Economic Journal 114 (495): 441–465. https://doi.org/10.1111/j.1468-0297.2004.00216.x.
- Larson, J. 2010. In Search of Synergy in Small Group Performance. New York: Psychology Press.
- Laughlin, P. R., E. C. Hatch, J. S. Silver, and L. Boh. 2006. “Groups Perform Better Than the Best Individuals on Letters-To-Numbers Problems: Effects of Group Size.” Journal of Personality & Social Psychology 90 (4): 644. https://doi.org/10.1037/0022-3514.90.4.644.
- Lee, J. J. 2010. “Heterogeneity, Brokerage, and Innovative Performance: Endogenous Formation of Collaborative Inventor Networks.” Organization Science 21 (4): 804–822. https://doi.org/10.1287/orsc.1090.0488.
- Li, G. C., R. Lai, A. D’Amour, D. M. Doolin, Y. Sun, V. I. Torvik, and L. Fleming. 2014. “Disambiguation and Co-Authorship Networks of the US Patent Inventor Database (1975–2010).” Research Policy 43 (6): 941–955.
- Lin, N. 2002. Social Capital: A Theory of Social Structure and Action. Vol. 19. Cambridge University Press.
- Lissoni, F., P. Llerena, and B. Sanditov. 2013. “Small Worlds in Networks of Inventors and the Role of Academics: An Analysis of France.” Industry & Innovation 20 (3): 195–220. https://doi.org/10.1080/13662716.2013.791128.
- Llopis, O., and P. D’Este. 2022. “Brokerage That Works: Balanced Triads and the Brokerage Roles That Matter for Innovation.” Journal of Product Innovation Management 39 (4): 492–514. https://doi.org/10.1111/jpim.12618.
- March, J. G. 1991. “Exploration and Exploitation in Organizational Learning.” Organization Science 2 (1): 71–87. https://doi.org/10.1287/orsc.2.1.71.
- McGrath, J. 1984. Groups: Interaction and Performance. Englewood Cliffs, NJ: Prentice Hall.
- Moon, H., A. Di Benedetto, and S. K. Kim. 2022. “The Effect of Network Tie Position on a Firm’s Innovation Performance.” Journal of Business Research 144:821–829. https://doi.org/10.1016/j.jbusres.2022.02.035.
- Moreira, S., A. Markus, and K. Laursen. 2018. “Knowledge Diversity and Coordination: The Effect of Intrafirm Inventor Task Networks on Absorption Speed.” Strategic Management Journal 39 (9): 2517–2546. https://doi.org/10.1002/smj.2914.
- Nerkar, A., and S. Paruchuri. 2005. “Evolution of R&D Capabilities: The Role of Knowledge Networks within a Firm.” Management Science 51 (5): 771–785. https://doi.org/10.1287/mnsc.1040.0354.
- Nijstad, B. A., W. Stroebe, and H. F. Lodewijkx. 2002. “Cognitive Stimulation and Interference in Groups: Exposure Effects in an Idea Generation Task.” Journal of Experimental Social Psychology 38 (6): 535–544.
- Nokes-Malach, T. J., J. E. Richey, and S. Gadgil. 2015. “When Is it Better to Learn Together? Insights from Research on Collaborative Learning.” Educational Psychology Review 27 (4): 645–656. https://doi.org/10.1007/s10648-015-9312-8.
- O’Brien, R. M. 2007. “A Caution Regarding Rules of Thumb for Variance Inflation Factors.” Quality & Quantity 41 (5): 673–690. https://doi.org/10.1007/s11135-006-9018-6.
- Obstfeld, D. 2005. “Social Networks, the Tertius Iungens Orientation, and Involvement in Innovation.” Administrative Science Quarterly 50 (1): 100–130. https://doi.org/10.2189/asqu.2005.50.1.100.
- Orsatti, G., F. Quatraro, and M. Pezzoni. 2020. “The Antecedents of Green Technologies: The Role of Team-Level Recombinant Capabilities.” Research Policy 49 (3): 103919. https://doi.org/10.1016/j.respol.2019.103919.
- Osborn, A. 1953. Applied Imagination: Principles and Procedures of Creative Thinking. New York: Scribners.
- Paruchuri, S. 2010. “Intraorganizational Networks, Interorganizational Networks, and the Impact of Central Inventors: A Longitudinal Study of Pharmaceutical Firms.” Organization Science 21 (1): 63–80. https://doi.org/10.1287/orsc.1080.0414.
- Paruchuri, S., and S. Awate. 2017. “Organizational Knowledge Networks and Local Search: The Role of Intra-Organizational Inventor Networks.” Strategic Management Journal 38 (3): 657–675. https://doi.org/10.1002/smj.2516.
- Patrucco, A., C. M. Harland, D. Luzzini, and F. Frattini. 2022. “Managing Triadic Supplier Relationships in Collaborative Innovation Projects: A Relational View Perspective.” Supply Chain Management: An International Journal 27 (7): 108–127. https://doi.org/10.1108/SCM-05-2021-0220.
- Perry-Smith, J. E., and P. V. Mannucci. 2017. “From Creativity to Innovation: The Social Network Drivers of the Four Phases of the Idea Journey.” Academy of Management Review 42 (1): 53–79. https://doi.org/10.5465/amr.2014.0462.
- Phelps, C., R. Heidl, and A. Wadhwa. 2012. “Knowledge, Networks, and Knowledge Networks: A Review and Research Agenda.” Journal of Management 38 (4): 1115–1166. https://doi.org/10.1177/0149206311432640.
- Powell, W. W., K. W. Koput, and L. Smithdoerr. 1996. “Interorganizational Collaboration and the Locus of Innovation: Networks of Learning in Biotechnology.” Administrative Science Quarterly 41 (1): 116–145. https://doi.org/10.2307/2393988.
- Quintane, E., and G. Carnabuci. 2016. “How Do Brokers Broker? Tertius Gaudens, Tertius Iungens, and the Temporality of Structural Holes.” Organization Science 27 (6): 1343–1360. https://doi.org/10.1287/orsc.2016.1091.
- Reagans, R., and B. McEvily. 2003. “Network Structure and Knowledge Transfer: The Effects of Cohesion and Range.” Administrative Science Quarterly 48 (2): 240–267+355–356. https://doi.org/10.2307/3556658.
- Rietzschel, E. F., B. A. Nijstad, and W. Stroebe. 2006. “Productivity Is Not Enough: A Comparison of Interactive and Nominal Brainstorming Groups on Idea Generation and Selection.” Journal of Experimental Social Psychology 42 (2): 244–251.
- Rzakhanov, Z. 2004. “Innovation, Product Development and Market Value: Evidence from the Biotechnology Industry Innovation in Biotechnology Industry.” Economics of Innovation & New Technology 13 (8): 747–760. https://doi.org/10.1080/1043859042000226211.
- Salman, N., and A. L. Saives. 2005. “Indirect Networks: An Intangible Resource for Biotechnology Innovation.” R & D Management 35 (2): 203–215. https://doi.org/10.1111/j.1467-9310.2005.00383.x.
- Savino, T., A. Messeni Petruzzelli, and V. Albino. 2017. “Search and Recombination Process to Innovate: A Review of the Empirical Evidence and a Research Agenda.” International Journal of Management Reviews 19 (1): 54–75. https://doi.org/10.1111/ijmr.12081.
- Schumpeter, J. A. 1934. Theorie Der Wirtschaftlichen Entwicklung. Berlin: Duncker & Humblot.
- Simmel, G. 1950. The Sociology of Georg Simmel. New York: Free Press.
- Simon, H. A. 1991. “Bounded Rationality and Organizational Learning.” Organization Science 2 (1): 125–134. https://doi.org/10.1287/orsc.2.1.125.
- Singh, H., D. Kryscynski, X. Li, and R. Gopal. 2016. “Pipes, Pools, and Filters: How Collaboration Networks Affect Innovative Performance.” Strategic Management Journal 37 (8): 1649–1666. https://doi.org/10.1002/smj.2419.
- Singh, J. 2005. “Collaborative Networks As Determinants of Knowledge Diffusion Patterns.” Management Science 51 (5): 756–770. https://doi.org/10.1287/mnsc.1040.0349.
- Singh, J., and L. Fleming. 2010. “Lone Inventors As Sources of Breakthroughs: Myth or Reality?” Management Science 56 (1): 41–56. https://doi.org/10.1287/mnsc.1090.1072.
- Soda, G., M. Tortoriello, and A. Iorio. 2018. “Harvesting Value from Brokerage: Individual Strategic Orientation, Structural Holes, and Performance.” Academy of Management Journal 61 (3): 896–918. https://doi.org/10.5465/amj.2016.0123.
- Soda, G., A. Zaheer, X. Sun, and W. Cui. 2021. “Brokerage Evolution in Innovation Contexts: Formal Structure, Network Neighborhoods and Knowledge.” Research Policy 50 (10): 104343. https://doi.org/10.1016/j.respol.2021.104343.
- Solt, F., and Y. Hu. 2015. “Dotwhisker: Dot-And-Whisker Plots of Regression Results.” Available at the Comprehensive R Archive Network (CRAN).
- Spiro, E. S., R. M. Acton, and C. T. Butts. 2013. “Extended Structures of Mediation: Re-Examining Brokerage in Dynamic Networks.” Social Networks 35 (1): 130–143. https://doi.org/10.1016/j.socnet.2013.02.001.
- Stovel, K., and L. Shaw. 2012. “Brokerage.” Annual Review of Sociology 38 (1): 139–158. https://doi.org/10.1146/annurev-soc-081309-150054.
- Sweller, J. 2011. “Chapter Two - Cognitive Load Theory.” In Psychology of Learning and Motivation, edited by P. M. Jose and H. R. Brian, 37–76. Vol. 55. Academic Press.
- Ter Wal, A. L. J. 2014. “The Dynamics of the Inventor Network in German Biotechnology: Geographic Proximity versus Triadic Closure.” Journal of Economic Geography 14 (3): 589–620. https://doi.org/10.1093/jeg/lbs063.
- Toh, P. K., and F. Polidoro. 2013. “A Competition-Based Explanation of Collaborative Invention within the Firm.” Strategic Management Journal 34 (10): 1186–1208. https://doi.org/10.1002/smj.2059.
- Tortoriello, M. 2015. “The Social Underpinnings of Absorptive Capacity: The Moderating Effects of Structural Holes on Innovation Generation Based on External Knowledge.” Strategic Management Journal 36 (4): 586–597. https://doi.org/10.1002/smj.2228.
- Tortoriello, M., and D. Krackhardt. 2010. “Activating Cross-Boundary Knowledge: The Role of Simmelian Ties in the Generation of Innovations.” Academy of Management Journal 53 (1): 167–181. https://doi.org/10.5465/amj.2010.48037420.
- Tóth, G., S. Juhász, Z. Elekes, and B. Lengyel. 2021. “Repeated Collaboration of Inventors Across European Regions.” European Planning Studies 29 (12): 2252–2272. https://doi.org/10.1080/09654313.2021.1914555.
- Tzabbar, D. 2009. “When Does Scientist Recruitment Affect Technological Repositioning?” Academy of Management Journal 52 (5): 873–896. https://doi.org/10.5465/amj.2009.44632853.
- Tzabbar, D., and A. Vestal. 2015. “Bridging the Social Chasm in Geographically Distributed R&D Teams: The Moderating Effects of Relational Strength and Status Asymmetry on the Novelty of Team Innovation.” Organization Science 26 (3): 811–829. https://doi.org/10.1287/orsc.2015.0969.
- van de Kaa, G. 2018. “Who’s Pulling the Strings? The Influence of Network Structure on Standard Dominance.” R&D Management 48 (4): 438–446. https://doi.org/10.1111/radm.12295.
- Wagner, S., and M. C. Goossen. 2018. “Knowing Me, Knowing You: Inventor Mobility and the Formation of Technology-Oriented Alliances.” Academy of Management Journal 61 (6): 2026–2052. https://doi.org/10.5465/amj.2016.0818.
- Wang, Y., and V. Chiew. 2010. “On the Cognitive Process of Human Problem Solving.” Cognitive Systems Research 11 (1): 81–92. https://doi.org/10.1016/j.cogsys.2008.08.003.
- Williams, W., and R. Sternberg. 1988. “Group Intelligence: Why Some Groups Are Better Than Others.” Intelligence 12 (4): 351–377. https://doi.org/10.1016/0160-2896(88)90002-5.
- Woolley, A. W., I. Aggarwal, and T. W. Malone. 2015. “Collective Intelligence and Group Performance.” Current Directions in Psychological Science 24 (6): 420–424. https://doi.org/10.1177/0963721415599543.
- Wuchty, S., B. F. Jones, and B. Uzzi. 2007. “The Increasing Dominance of Teams in the Production of Knowledge.” Science 316 (5827): 1036–1039. https://doi.org/10.1126/science.1136099.
- Younge, K. A., T. W. Tong, and L. Fleming. 2015. “How Anticipated Employee Mobility Affects Acquisition Likelihood: Evidence from a Natural Experiment: Anticipated Employee Mobility and Acquisition Likelihood.” Strategic Management Journal 36 (5): 686–708. https://doi.org/10.1002/smj.2237.
- Zhang, Y., and T. W. Tong. 2021. “How Vertical Integration Affects Firm Innovation: Quasi-Experimental Evidence.” Organization Science 32 (2): 455–479. https://doi.org/10.1287/orsc.2020.1396.
- Zhelyazkov, P. I. 2018. “Interactions and Interests: Collaboration Outcomes, Competitive Concerns, and the Limits to Triadic Closure.” Administrative Science Quarterly 63 (1): 210–247. https://doi.org/10.1177/0001839217703935.