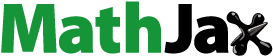
Abstract
During the COVID-19 pandemic, people had two important but imperfect teachers of risk. First, they learned about incidence, mortality rates, and reproduction numbers from reports and graphs. Second, they learned about the risk of infection through their own experience and the experience of others. Personal experience has been found to serve as an input for risk judgments in numerous contexts, including climate change. Here we examine how it shapes risk judgments within the dynamics of a pandemic, drawing on data collected from more than 15,000 German citizens between March 2020 and May 2022. People with personal experience of having contracted the virus consistently rated the risk of infection higher than those without such experience. The influence of others’ experience increased with their social distance to the respondent. Media coverage also influenced risk judgments but to a lesser extent. We discuss the implications of these patterns for risk communication.
Introduction
It is difficult to anticipate how the public will perceive the risk of hazardous events such as virus outbreaks, terrorist attacks, and the short- and long-term consequences of climate change. Several determinants of public risk judgments have been identified, including perceived properties of the risk itself (e.g. novelty, voluntariness of exposure, and dread potential; Slovic Citation1987), attributes and values of the perceiving individual and collective (e.g. trust in government and science, individualism, and fairness; Douglas Citation2013; Douglas and Wildavsky Citation1983), and the dynamics of the information environment (e.g. social amplification of risk in the transmission of information; Kasperson et al. Citation1988; Moussaïd, Brighton, and Gaissmaier Citation2015). A factor that has recently received increasing attention is the extent to which an individual has had personal experience of a risk, be it a macroeconomic shock such as the Great Depression (Malmendier and Nagel Citation2011) or a period of inflation (Malmendier and Nagel Citation2016); natural hazards such as floods, droughts, or earthquakes; (Wachinger et al. Citation2013); or living through a pandemic such as COVID-19 (Dryhurst et al. Citation2020). In the context of climate change, personal experience has been shown to have motivational and psychological relevance for a range of outcomes, including risk judgments and responses to risks (Akerlof et al. Citation2013; Demski et al. Citation2017; Myers et al. Citation2013; Reser, Bradley, and Ellul Citation2014; Van der Linden Citation2014). For instance, Viscusi and Zeckhauser (Citation2015) observed that relative to indirect experiences (i.e. the experience of peers/others), direct experiences have a stronger and more consistent effect on different measures of environmental risk beliefs. They also found that people with direct experiences are more likely to take precautionary measures. Relatedly, in an international survey of 24 countries, personal experience of global warming predicted endorsement of specific mitigation actions (Broomell, Budescu, and Por Citation2015).
Personal experience clearly matters. It is one of the main ways that individuals learn about risk and can be a powerful—though by no means perfect—teacher of risk (Hertwig and Wulff Citation2022). Experience can be understood as the process and result of living through events (Hertwig, Hogarth, and Lejarraga Citation2018; March Citation2010). It is multidimensional and includes cognitive (e.g. information), subjective (e.g. [un]pleasantness), and physiological (e.g. pain or pleasure) aspects; sometimes it has predominantly informational value and sometimes informational and material effects co-occur (Sutton and Barto Citation1998). Experience can be used to evaluate past actions and guide future ones (March Citation2010). Experiencing an option as positive increases the probability of that option being chosen in the future; the opposite holds for negative experiences (Denrell and March Citation2001). Although undergoing an experience may require effort, learning from experience may also be relatively effortless and immediate for the individual (e.g. avoiding a specific food after food poisoning). This is likely to hold for risk experiences that are imposed by the environment (e.g. experiencing the health consequences of record temperatures during a heat wave) or acquired through voluntary exposure (e.g. fracturing your shinbone while skiing). Experience has, in any case, special authority, both to the person living through it and to others who are direct observers or to whom that experience is communicated (Kubin et al. Citation2021).
In this article, we focus on the role of personal experience for risk judgments during the COVID-19 pandemic. Examining how personal experience shapes risk judgments during a global health crisis such as a pandemic is especially informative because disease transmission often follows nonlinear spatio-temporal dynamics (Stone, Olinky, and Huppert Citation2007), causing, for instance, seasonal fluctuations in incidence, epidemic peaks, and intermittent drops to a minimum level. plots the incidence of COVID-19 infections in Germany between March 2020 (the onset of the pandemic in Germany) and April 2022, a period of about two years. Cases fluctuated seasonally, with peaks being highly variable (e.g. compare the peaks in April 2020, 2021, and 2022). The baseline likelihood of people having had personal experience of the COVID-19 virus therefore varied greatly across the period of observation.
Figure 1. Panel A: COVID-19 incidence in Germany across a two-year time period, starting in March 2020 (the onset of the pandemic in Germany). Vertical dashed lines mark key events: lockdowns, beginning of vaccination rollout, and vaccination progress. The numbers from 1 to 17 indicate the survey waves. Panel B: Average risk judgments in each of the 17 survey waves, plotted separately for ‘direct experience’ and ‘no experience’ of a COVID-19 infection. Error bars represent bootstrapped 95% CIs.

How does experience affect people’s judgment of the risk of getting infected with such a dynamic, recurrent disease? A study across 10 countries focused on the brief period between March and April 2020. Findings showed that the direct experience of COVID-19 was indeed a significant predictor of risk judgments: ‘Specifically, people who have had direct personal experience with the virus perceive more risk compared to those who have not had direct experience’ (Dryhurst et al. Citation2020; p. 998). We build on this finding of ‘experiential amplification’ of risk by addressing three main research questions. All three concern the judgment of the risk of contracting COVID-19 and consider the alignment (or misalignment) of the judged risk (likelihood) of an infection with the actual temporal dynamics of the pandemic, and the role of personal experience in this (dis-)association.Footnote1 Our first research question takes experiential amplification of risk (Dryhurst et al. Citation2020) as the starting point. Does having been infected with COVID-19—in other words, having direct experience of COVID-19 infection—consistently amplify the judged risk of an infection relative to no experience across the highly dynamic course of the pandemic from March 2020 to May 2022 (see )?
Our second research question concerns the propagation of risk within a person’s close social circles. Even if someone has not been infected themself, a family member, friend, or colleague may have had the virus. The experience of family members is relatively tangible, immediate, and observable. Does such proxy family experience have the same potential effect of amplification on risk judgment, in magnitude and direction, as direct experience? Or does it have a lesser effect—potentially due to greater psychological distance?Footnote2 We also investigated how the experience of socially more distant circles (e.g. friends, colleagues, and neighbors) influenced individual risk judgment. We refer to the experience of this more distal group as network experience.
Our third research question concerns the role of communication about the COVID-19 infection risk. According to sociologist and scholar of systems theory Niklas Luhmann, hazardous events have no social impact unless they are the subject of communication (Luhmann Citation2004, 63). That is, physical, chemical, or biological facts—even human deaths—only generate social resonance through communication. Communication about COVID-19 was extensive, especially in the early stages of the pandemic; the World Health Organization even coined the term ‘infodemic’ to describe the onslaught of information and false information.Footnote3 Do dynamic changes in the intensity of such communication amplify (Renn et al. Citation1992) or attenuate people’s risk judgments, potentially causing a disconnect between the public’s risk judgment and objective disease incidence? We tracked COVID-19 media mentions (in offline and online newspapers) and online searches for information on COVID-19 in relation to respondents’ perceived risk judgments. Additionally we evaluate the sentiment of articles published in two large online media outlets (Bild.de and Spiegel.de) and ask whether valence of communication changes over time.
Methods
Procedure
We drew on data collected in the COMPASS (Corona-Online-Meinungs-Panel-Survey-Spezial) survey to examine the impact of personal experience on judgments of risk by German citizens. The COMPASS series was launched and financed by the commercial polling institute infratest dimap (https://www.infratest-dimap.de) in March 2020, we had limited control over the timing and content of the survey waves. Consequently, questions of particular interest (e.g. fear of a life-threatening case of COVID-19) were not consistently included in all waves; we return to this issue below.
Participants were recruited to the COMPASS panel from the German loyalty program PAYBACK, which has about 25 million members. Participation was voluntary. Our data derive from the 17 survey waves run between 17 March 2020 and 19 April 2022. Survey waves 1 to 15 lasted between 34 and 36 days each; wave 16 lasted 9 days and wave 17 lasted 6 days. Participants also responded to several questions not related to our analysis that are omitted here.
Data
Incidence
COVID-19 incidence in Germany was accessed through the COVID-19 Datenhub (https://npgeo-corona-npgeo-de.hub.arcgis.com). This website is associated with the Robert Koch Institute (RKI, http://www.rki.de), the German government’s central scientific institute in the field of biomedicine. It is one of the most important centers for safeguarding public health in Germany, and is responsible for identifying, surveying, and preventing disease, especially infectious diseases.
Risk judgments
In the COMPASS survey, risk judgments were measured by the following question: ‘What is your estimate of the risk that you or members of your family will become infected with the coronavirus in the future?’ Responses were given on a 11-point Likert scale with the end points ‘very small’ (=0) and ‘very large’ (=10). (The original German wording can be found in the Appendix, p. 17). This question was included in each of the 17 waves; we were therefore able to analyze the data across the full observation period.
Infection status
In waves 1–4, direct and indirect experience of infection was probed by the following question: ‘In many countries, including Germany, people have been infected with the coronavirus. Have you or anyone close to you had a confirmed infection, a suspected infection, or no indication of infection?’ From wave 5 onwards, the question was worded: ‘Have you or anyone close to you been diagnosed with a COVID-19 infection since the outbreak of the corona pandemic?’. Infection status was determined separately for six levels of social proximity (e.g. ‘I was diagnosed’ to ‘In my neighborhood someone was diagnosed’) that were then recoded into the four distance levels: ‘direct experience’, ‘family experience’, ‘network experience’ and ‘no experience’ (see Appendix, p. 17, for the original wording)
Media mentions, sentiment and google trends analyses
We used LexisNexis (www.lexisnexis.com), an information and data provider specialized in news and business sources worldwide, to gauge the communication landscape on COVID-19 in Germany over the observation period. A keyword search for ‘COVID*’ & ‘Corona*’ with a geographical restriction to Germany was performed in six daily and one weekly print news outlets (Der Spiegel, Kölner Stadt-Anzeiger, Kölnische Rundschau, Rheinische Post, Stuttgarter Zeitung, Südwest Presse) and two online-only outlets (Bild.de, Spiegel.de) between 1 January 2020 and 1 May 2022. The print newspapers represent seven of the 10 most widely read newspapers in Germany. Bild.de and Spiegel.de are German online news sources numbers one and three, respectively, in terms of visits. Our search resulted in 27,869 articles featuring the search terms. We divided the number of occurrences of the search term per article by the length (word count) of the article, resulting in a length-normalized measure of occurrence.
To better understand how COVID-19 might have an effect not only on the number of articles but also on the content we ran a sentiment analysis (determining whether a text uses more positive or negative emotion words) on the two online news outlets (for better comparison) in our dataset: Bild.de and Spiegel.de. From these 1863 articles mentioned Covid* and/or Corona*. We tokenized the text into 1,9 M single words. On this dataset we ran a standard dictionary search using the SentiWS database, which contains, in version v1.8b, ratings for 16,406 positive (maximum positive = +1) and 16,328 negative (maximum negative = −1) word forms including inflections (Remus, Quasthoff, and Heyer Citation2010). For each month of our survey window, we then averaged the positive and negative sentiments, separately for Bild.de and Spiegel.de resulting in an valence index of more positive or negative usage of emotionally laden words. The results of this analysis can be found in .
Communicative acts are not limited to the traditional media. Users of search engines such as Google also communicate through their exploratory behaviors. We therefore additionally conducted a Google Trends (https://trends.google.com/) analysis for the keywords ‘Corona*’ and ‘Covid’Footnote4 in Germany during the observation period. This analysis resulted in a normalized measure of search frequency for the search terms.
Vaccination rates in Germany
Vaccination rates were imported from the webpage of the Robert Koch Institute (https://www.rki.de; ‘Digitales Impfquoten Monitoring’). We calculated the cumulative number of vaccinations in Germany (aggregated across first, second, and third shot) for the observation period.
Fear
To provide insights into how fear about COVID-19 compared with fear of other risks, the COMPASS survey asked respondents to rate fear of 12 risks in various life domains: cancer, heart attack, stroke; climate change; COVID-19; damage to home through natural disasters; economic hardship; extreme weather events; financial hardship through natural disasters; flying; lack of digitalization; political extremism; tax hikes due to COVID-19; traffic accidents (see Appendix, p. 17, for the original wording, English translation, and response options). These data were obtained only once, in survey wave 15 (between 9 September 2021 and 13 October 2021).
Participants
Table A1 in the Appendix shows the demographic characteristics of the panel. Overall, 15,015 unique participants (51.2% female) completed the questionnaires. Average age was M = 48.6 years (SD = 17.1) in women and M = 49.5 years (SD = 16.9) in men. This difference was statistically significant t(14,995) = 3.21, p = .001, d = .05; however, in terms of Cohen’s d, the effect size was negligible. Although the gender age difference in the general German age distribution is in the opposite direction, with the female population being older (average age of 46.0 years for women and 43.4 years for men in 2021 (Bundesinstitut für Bevölkerungsforschung Citation2023), it is not likely, given the sample size and the wide distribution in terms of age groups and education (see Table A1), that the present results were substantially affected).
Of the more than 15,000 participants in all waves the range of participants in each wave was relatively stable between waves 1 to 16 (Range = 9234 to 11,200) with the maximum in the first wave. Table A2 in the Appendix lists these participation rates in detail. Only wave 16 and 17 had a substantial decline in participation, due to financial restrictions in inviting participants to the panel. Many participants were in more than one wave, column ‘Percent participants in wave x and wave 1′ indicates the percentage of participants who were in a given wave and also participated in the first wave, the range of these percentages is between 86% and 89%. There was also a stable core of 1107 participants who answered all 17 waves.
Results
Dynamics of incidence and risk judgments
plots the incidence (i.e. the number of new infections over the past seven days per 100,000 inhabitants) of COVID-19 infections across the 17 survey waves (indicated by grey blocks). To the extent that incidence is a proxy for the risk of contracting COVID-19, the temporal dynamics of the objective risk varied greatly over the observation period: Whereas the average incidence was as low as four cases in 100,000 in the first three survey waves (march, April, and May 2020), it rose to 253 in 100,000 in March 2022, thus increasing by a factor of 63.
Across the 17 survey waves, respondents were repeatedly asked to judge their risk of contracting COVID-19. plots the average risk judgments. Although the judgments track some features of the incidence distribution in (e.g. the decline in summer 2020), it is clear that the average subjective risk judgment does not closely follow the enormous fluctuations in incidence. This suggests that people’s risk judgments do not simply reflect the objective incidence, but that they are also shaped by other factors.
Does direct experience of COVID-19 infection influence risk judgments?
One potential factor is direct experience of COVID-19 infection, that is, having contracted the virus oneself. plots risk judgments as a function of whether respondents had direct experience or no experience of COVID-19. Notwithstanding the dynamic course of the pandemic, the average risk judgment was—consistently across all waves—notably higher for respondents with direct experience (M = 5.3, SD = 2.6) than for those with no experience (M = 3.8, SD = 2.5). The effect size of this difference was d = 0.61.
To further examine this difference, we calculated a mixed-effects model, predicting risk judgment on the basis of direct experience or no experience of an infection. We added random effects for survey wave (nested in year and month of the pandemic) and participant (nested in age group). We calculated a basic model and a full model controlling for gender, age group, media mentions, Google searches, COVID-19 incidence, and aggregated vaccination numbers in Germany. The relevant variables were standardized and centered before being entered in the models. The results of the basic and full model are reported in Table A3 in the Appendix. Both models resulted in a significant effect of experience (direct vs. no experience) on risk judgment (full model: ), indicating lower risk judgments in the no experience group than in the direct experience group.
Does indirect experience of COVID-19 infection influence risk judgments?
Relative to the power of personal experience, how does the proxy experience of others impact people’s risk judgments? To find out, we distinguished between four categories of experience with increasing social distance to the target person: direct experience: the individual personally experienced infection; family experience: at least one family member experienced infection; network experience: someone in the target person’s social network of friends, colleagues, and neighbors experienced infection, and no experience: none of the above.
plots risk judgments as a function of these four categories. A systematic pattern emerged. The further distant from the individual a COVID-19 infection was, the smaller the judged risk of contracting the infection. In the aggregate, this regularity can be observed in decreasing average risk ratings across the four categories. The direct experience and no experience groups gave the highest and lowest risk judgments, as reported above, with family experience () and network experience (
) sandwiched in between. We used a mixed-effects model to further examine the effect of distance on experience, predicting risk judgment on the basis of the four experience categories. We again built a basic model and a full model with the same control variables. The full model revealed significant decreases in risk judgments between direct and family experience (
–
), between direct and network experience (
–
), and between direct and no experience (
– −0.33], (see Table A4 in the Appendix).
Figure 2. Average risk judgments in each of the 17 survey waves, plotted separately for (in)direct experience and no experience of COVID-19 infection. Error bars represent bootstrapped 95% CIs.

We modeled time in the basic and full model in two ways: 1) by adding wave as a predictor and 2) by nesting wave in the Year + Month of the pandemic as a random effect. Both treatments in both models did result in a small negative effect ( –
) indicating a reduction in risk judgments over time. Among many other external variables changing during this period, one central variable to our observation had large changes across the 17 waves - the proportion of respondents with experience of an infection. plots the proportion of participants with each category of experience of COVID-19 across the survey waves. Three phases can be identified: Phase 1, survey wave 1 to 5, with a relatively stable distribution of more than two-thirds of the sample having no experience of COVID-19. Phase 2, survey waves 6 to 15, with a steady increase in direct experiences of infection. Finally, phase 3, survey waves 16 and 17, with a sharp increase in the proportion of direct experience of infection (to 21% on average). The proportion of respondents with no experience of COVID-19 decreased accordingly, from, on average, 66% to 46% to 17% across the three phases.
To summarize the findings on the effects of experience, direct experience of infection notably increased risk judgments across all survey waves. These results corroborate the findings of Dryhurst et al. (Citation2020) and show that the amplification effect of direct experience persisted throughout the two-year time window. Furthermore, we found that others’ experience also increased risk judgments, with this effect being most pronounced for family members. We now turn to our third research question, investigating the impact of media mentions and Google searches on risk judgments.
Is the volume and sentiment of communication about COVID-19 related to risk judgments?
According to Luhmann (Citation2004), hazardous events have no social resonance unless they are communicated. Against this background, we investigated the extent to which media coverage of COVID-19 correlated with risk judgments. plots how often COVID-19 was mentioned in major German print and online media outlets across the 17 survey waves (length-normalized measure). Users of search engines such as Google also communicate through their exploratory behaviors. We therefore additionally tracked the frequency of Google searches for information on COVID-19. plots the results of the Google Trends analysis.
Figure 4. Panel A: Media mentions of ‘COVID*’ and ‘Corona*’ in major German print and online media outlets. Panel B: Sentiment analysis for positive and negative words found in Bild.de and Spiegel.de. Panel C: Google searches for ‘Corona*’ in Germany.

Both trends are almost reversed images of the incidence trend shown in . Not surprisingly, communication about the pandemic (in terms of media reporting and Google searches) was most pronounced during the early stages of the pandemic and then dropped, with substantial fluctuations, to a much lower level in May 2022. A mixed-effects model predicting risk judgments by changes in communicative trends, and with participant (nested in age group) as a random effect, yielded significant effects for both media mentions –
and Google searches
–
. This indicates that higher risk judgments were positively associated with the frequency of media mentions and Google searches (see Table A5 in the Appendix for details). Nevertheless, during waves 16 and 17, when COVID-19 incidences were highest, media mentions and Google searches dropped to their lowest levels and apparently uncoupled from people’s risk judgments.
We have only considered the volume of articles to this point. In , we add an additional dimension - the valence of these publications. Exemplary we use data from the two available online-media: Bild.de and Spiegel.de. Bild.de is widely recognized as a ‘Boulevard’ medium, topping national usage numbers in Germany every year; Spiegel.de, in contrast, is recognized as a quality source for journalism. During most of the observational period valence of the articles is negative for both sources (with the exception of June 2020 and December 2020 for Bild.de). Only more recently valence switched to positive, in December 2021 for Bild.de, followed by Spiegel.de in April 2022. An opposite, correlative, trend can be observed, where higher media attention, earlier in the pandemic, generally is accompanied by a more negative sentiment in these outlets. This changes later in the pandemic when media attention (and to that end Google searches) generally decrease and the sentiment of reporting trends more toward a positive sentiment. It is important to note that this observation is purely correlational and we do not want to speculate about a causal mechanism here.
Our analysis up to this point uses an important structural factor only as a co-variate: age. In what follows we first want to focus on the question whether age plays a moderating role for risk judgments and then turn to the analysis of fear in different life domains.
Does age moderate risk judgments?
In the early days of COVID-19, the mortality risk was especially high for vulnerable groups. There is scientific consensus that the most vulnerable population group was the elderly (Daoust Citation2020). Accordingly, many governments targeted older adults in their virus containment strategies, and introduced drastic public health measures including social isolation and lockdowns. Furthermore, older adults were prioritized once vaccines were available (Shahid et al. Citation2020). How did this heightened concern for their wellbeing affect the risk judgments of older adults? Across the 17 survey waves, we found that the two oldest age groups (66–75 years and 76–85 years) had the lowest perceived risk judgments, whereas the youngest age group (18–25 years) had the highest (see ).
This finding was substantiated by a multilevel analysis predicting risk judgments by age groups (using the youngest participants, aged 18–25 years, as the reference category). We found no significant difference between the youngest and the adjacent age groups up to 55 years. In contrast, the three older age groups had significantly lower risk judgments: –
for 56–65 years,
–
for 66–75 years, and
–
for 76–85 years (see Appendix A4 for details, note that we will focus on the four-way split here. Results for the age groups, no effect up to 55, a significant effect for 56 and older, were similar using the two-way split analysis reported above). We return to this somewhat puzzling finding in the Discussion.
How does fear of COVID-19 compare to other fears?
Our final analysis concerns how the fear of COVID-19 compares with other fears (e.g. fear of flying, extreme weather events, and political extremism). plots these average fear ratings (on a 11-point scale), split into experience versus no experience and ordered from lowest to highest overall, average rating. Flying had the lowest average fear rating () in comparison COVID-19 the third lowest (M = 4.5, SD = 2.6) of the 12 evaluated fears. The average rating for other health fears (cancer, heart attack, stroke) was more than one scale point larger, at an average of M = 5.7 (SD = 2.7). At the time this question was asked (in wave 15, i.e. September–October 2021), participants seemed to be most worried about (in increasing order) Germany lagging behind on digitalization, climate change, political extremism, and tax hikes due to COVID-19 (all with
. A differential effect of experience (i.e. a difference between direct experience versus no experience) was only found (in simple effects t-tests) in three domains: COVID-19, climate change and political extremism with a maximum of .5 differences on the rating scale in these domains. At this late point in time, in the pandemic, more current topics than COVID-19 (e.g. the formation of the Last Generation movement in Germany 2021) became apparently more prominent in the public eye.
Discussion
The world is currently in a state of polycrisis, defined as the simultaneous occurrence of several catastrophic events (Heading and Zahidi Citation2023). These include the COVID-19 pandemic, the war in Ukraine, the energy crisis, the cost-of-living crisis, and the climate crisis. Faced with a world in polycrisis, how citizens perceive various risks is becoming increasingly important, particularly as it informs their willingness to take action. One factor driving risk judgments has received increasing attention in recent years: personal experience. The COVID-19 pandemic can serve as a ‘model risk’ to investigate how personal experience changes people’s risk judgments. Like climate change, a pandemic is a process that unfolds in time—but at a much faster pace. Drawing on data from a panel survey with 17 waves from March 2020 to May 2022, we examined for a western society how direct and indirect experience of infection with COVID-19 affected the judgment of risk (of infection) relative to the impact of objective incidence and collective measures of communication about the hazard.
Our results can be summarized in four key findings about the link between personal experience of COVID-19 and risk judgments. First, there was a marked difference in risk judgments between people with no experience and those with direct experience. The latter deemed the risk of infection consistently and notably higher. This result is in line with the findings of Dryhurst et al. (Citation2020), who found that direct experience of the virus was an important predictor of risk perception in the early months of the pandemic (and, for instance, the most important predictor of risk perception in the United States). We refer to the effect of direct experience as experiential amplification of risk. Second, we found a ‘gradient’ in the power of experience: The closer the respondent was to the person who was the source of experience, the stronger the impact on their risk judgment. For instance, a family member being infected had a stronger psychological impact than a friend or a colleague being infected. Third, we found a positive association between communication on the virus and risk judgments: When COVID-19 media mentions (in online and traditional newspapers) and Google searches for COVID-19-related information were high, the risk of infection was generally judged higher. This correlation vanished as media coverage and Google searches decreased over time. Finally, risk judgments (of contracting COVID-19) were consistently lower in older than in younger age groups.
Let us briefly discuss the effect of age. As shows, the two most vulnerable age groups, those aged 66 to 85 years, consistently gauged the risk of infection lowest—much lower than, for instance, those aged 18 to 25 years. There are at least two possible interpretations of this somewhat puzzling result. One is that the older age cohorts were not systematically more responsive to the threat of infection than younger cohorts, and that, if anything, they were less responsive. Such an interpretation would echo the observation of Daoust (Citation2020) that elderly people (age > 70 years, across 27 countries) were not more disciplined in terms of compliance with preventive measures (compared to people in their 50’s and 60’s). However, a worldwide assessment of adherence behavior from March through December 2020 in 124 countries found that the elderly (age > 60 years) adhered more initially and maintained greater relative adherence over time (Petherick et al. Citation2021). This is consistent with a second interpretation of the findings presented in : Because older and more vulnerable risk groups adhered more strictly to precautionary measures (e.g. staying at home, wearing a face mask), their perceived risk of infection was lower. Indeed, as an additional analysis (see Figure A1 in the Appendix) of fear of a life-threatening disease showed, older age cohorts were not unduly optimistic (Weinstein Citation1982) about their susceptibility to a severe (life-threatening) case of corona. Throughout 2020 and in early 2021, the older age groups’ assessments of their risk of life-threatening disease were consistently much higher than those of the younger age groups. This only changed when vaccines became widely available in summer 2021.Footnote5
What are the policy implications of our results? Our findings converge with others that show (in)direct experience to be important for risk judgments. In further interpreting the role of direct experience in the pandemic, however, some important issues should be kept in mind. First, we do not know whether the risk judgments of people with (in)direct experience are better calibrated than those of people without. Second, policy makers should not focus solely on people with direct experience of infection; not having been infected over hundreds of days in all likelihood has strong informational value for the person. By the same token, as Hertwig and Wulff (Citation2022) pointed out, most people living in the red zone of Mount Vesuvius, Europe’s ticking time bomb, have never experienced it erupting—the last eruption was in March 1944. Many all-clear experiences are also a source of risk beliefs and may affect individuals’ risk judgments. Third, if the risk judgments of people with direct experience of an infection do prove to be better calibrated, then it raises the question of how the risk judgments of those who lack this experience can be improved. One approach could be to enrich standard risk information, that is, numerical and graphical descriptions, with what Kaufmann, Weber, and Haisley (Citation2013), in the context of investment decisions, have called experience sampling. Kaufmann, Weber, and Haisley (Citation2013) designed a simple risk tool that enabled participants to experience the distribution of a risky financial product. Rather than providing clients with standard risk information (e.g. fact sheets on historical returns), they had clients interactively sample possible outcomes, that is, possible returns on an investment. Each sampled outcome contributes to the return distribution, which is displayed at the end of the sampling process. Kaufmann, Weber, and Haisley (Citation2013) observed differences between experience and description on various dimensions. For instance, ‘experience’ investors allocated a larger percentage of their endowment to the risky fund than did ‘description’ investors; they were more accurate in their understanding of the options’ expected return and, importantly, in the estimated probability of a loss. Enlisting the methodology of experience sampling, Wegwarth et al. (Citation2023) found that vaccine-hesitant adults who engaged with an interactive experience simulator were more likely to show positive change in COVID-19 vaccination intention and benefit-to-harm assessment than those presented with the same information in a conventional text-based format.
To conclude, the COVID-19 pandemic offers a unique opportunity to examine how changing personal experience shapes changing risk judgments across the highly volatile spatio-temporal dynamics of a recurrent disease. We found that direct experience of COVID-19 infection increased risk judgments. In addition, the impact of others’ experience gradually declined as a function of social distance. Personal experience has also been shown to matter for risk assessments and willingness to take action in the context of other risks, such as climate change. One resulting task for future risk communication is to go beyond one-size-fits all approaches and to tailor communication to people’s changing levels of experience.
Author note
While we were working on the revision of this manuscript, Gert G. Wagner passed away. Without Gert’s scientific curiosity and his unrivaled ability to find interesting and informative data sets, this study would never have been carried out.
Acknowledgments
The authors would like to thank Infratest dimap, Nico A. Siegel and Christian Spinner for providing us with the microdata analyzed here. We are grateful to the flamboyant Susannah Goss for editing the manuscript.
Disclosure statement
No potential conflict of interest was reported by the author(s).
Notes
1 There are, of course, other key dimensions of COVID-19 risk judgments, such as fear of a life-threatening case of COVID-19. We also shed some light on this dimension of risk judgment (see Discussion and Figure A1 in the Appendix), but because of decisions on the COMPASS questionnaire (see Procedure for details) over which we had no control, the data are incomplete. Fortunately, we do have complete data for judgments of the risk of contracting COVID-19 across the full two-year period (see Method for details).
2 Psychological distance is a construct referring to the extent to which an object or event is removed from the self. It can be brought about via temporal, spatial, and social distances (Trope and Liberman Citation2010).
3 ‘An infodemic is too much information including false or misleading information in digital and physical environments during a disease outbreak. It causes confusion and risk-taking behaviors that can harm health. It also leads to mistrust in health authorities and undermines the public health response. An infodemic can intensify or lengthen outbreaks when people are unsure about what they need to do to protect their health and the health of people around them’ (Definition retrieved from https://www.who.int/health-topics/infodemic).
4 Note that the relative searches for ‘Covid’ were comparably small. We therefore use this variable for the modeling but do not plot the respective data in .
5 Data on fear of a life-threatening case of COVID-19 were collected in 13 of the 17 waves.
References
- Akerlof, K., E. W. Maibach, D. Fitzgerald, A. Y. Cedeno, and A. Neuman. 2013. “Do People “Personally Experience” Global Warming, and If so How, and Does It Matter?” Global Environmental Change 23 (1): 81–91. https://doi.org/10.1016/j.gloenvcha.2012.07.006.
- Broomell, S. B., D. V. Budescu, and H.-H. Por. 2015. “Personal Experience with Climate Change Predicts Intentions to Act.” Global Environmental Change 32: 67–73. https://doi.org/10.1016/j.gloenvcha.2015.03.001.
- Bundesinstitut für Bevölkerungsforschung. 2023. Durchschnittsalter der Bevölkerung in Deutschland [Average age of the population of Germany]. https://www.bib.bund.de/Permalink.html?cms_permaid=1217910.
- Daoust, J.-F. 2020. “Elderly People and Responses to COVID-19 in 27 Countries.” PLoS One 15 (7): E 0235590. https://doi.org/10.1371/journal.pone.0235590.
- Demski, C., S. Capstick, N. Pidgeon, R. G. Sposato, and A. Spence. 2017. “Experience of Extreme Weather Affects Climate Change Mitigation and Adaptation Responses.” Climatic Change 140 (2): 149–164. https://doi.org/10.1007/s10584-016-1837-4.
- Denrell, J., and J. G. March. 2001. “Adaptation as Information Restriction: The Hot Stove Effect.” Organization Science 12 (5): 523–538. https://doi.org/10.1287/orsc.12.5.523.10092.
- Douglas, M. 2013. Risk and Blame. New York, NY: Routledge.
- Douglas, M., and A. Wildavsky. 1983. Risk and Culture: An Essay on the Selection of Technological and Environmental Dangers. Berkley, CA: University of California Press.
- Dryhurst, S., C. R. Schneider, J. Kerr, A. L. J. Freeman, G. Recchia, A. M. van der Bles, D. Spiegelhalter, and S. van der Linden. 2020. “Risk Perceptions of COVID-19 around the World.” Journal of Risk Research 23 (7-8): 994–1006. https://doi.org/10.1080/13669877.2020.1758193.
- Heading, S., and S. Zahidi. 2023. Global Risks Report 2023. Geneva, Switzerland: World Economic Forum.
- Hertwig, R., R. M. Hogarth, and T. Lejarraga. 2018. “Experience and Description: Exploring Two Paths to Knowledge.” Current Directions in Psychological Science 27 (2): 123–128. https://doi.org/10.1177/0963721417740645.
- Hertwig, R., and D. U. Wulff. 2022. “A Description-Experience Framework of the Psychology of Risk.” Perspectives on Psychological Science: A Journal of the Association for Psychological Science 17 (3): 631–651. https://doi.org/10.1177/17456916211026896.
- Kasperson, R. E., O. Renn, P. Slovic, H. S. Brown, J. Emel, R. Goble, J. X. Kasperson, and S. Ratick. 1988. “The Social Amplification of Risk: A Conceptual Framework.” Risk Analysis 8 (2): 177–187. https://doi.org/10.1111/j.1539-6924.1988.tb01168.x.
- Kaufmann, C., M. Weber, and E. Haisley. 2013. “The Role of Experience Sampling and Graphical Displays on One’s Investment Risk Appetite.” Management Science 59 (2): 323–340. https://doi.org/10.1287/mnsc.1120.1607.
- Kubin, E., C. Puryear, C. Schein, and K. Gray. 2021. “Personal Experiences Bridge Moral and Political Divides Better Than Facts.” Proceedings of the National Academy of Sciences 118 (6): E 2008389118. https://doi.org/10.1073/pnas.2008389118.
- Luhmann, N. 2004. Ökologische Kommunikation [Ecological Communication]. 4th ed. Wiesbaden, Germany: Springer.
- Malmendier, U., and S. Nagel. 2011. “Depression Babies: Do Macroeconomic Experiences Affect Risk Taking?” The Quarterly Journal of Economics 126 (1): 373–416. https://doi.org/10.1093/qje/qjq004.
- Malmendier, U., and S. Nagel. 2016. “Learning from Inflation Experiences.” The Quarterly Journal of Economics 131 (1): 53–87. https://doi.org/10.1093/qje/qjv037.
- March, J. G. 2010. The Ambiguities of Experience. Ithaca, NY: Cornell University Press.
- Moussaïd, M., H. Brighton, and W. Gaissmaier. 2015. “The Amplification of Risk in Experimental Diffusion Chains.” Proceedings of the National Academy of Sciences of the United States of America 112 (18): 5631–5636. https://doi.org/10.1073/pnas.1421883112.
- Myers, T. A., E. W. Maibach, C. Roser-Renouf, K. Akerlof, and A. A. Leiserowitz. 2013. “The Relationship between Personal Experience and Belief in the Reality of Global Warming.” Nature Climate Change 3 (4): 343–347. https://doi.org/10.1038/nclimate1754.
- Petherick, A., R. Goldszmidt, E. B. Andrade, R. Furst, T. Hale, A. Pott, and A. Wood. 2021. “A Worldwide Assessment of Changes in Adherence to COVID-19 Protective Behaviours and Hypothesized Pandemic Fatigue.” Nature Human Behaviour 5 (9): 1145–1160. https://doi.org/10.1038/s41562-021-01181-x.
- Remus, R., U. Quasthoff, and G. Heyer. 2010. “SentiWS - A Publicly Available German-Language Resource for Sentiment Analysis.” In Proceedings of the Seventh International Conference on Language Resources and Evaluation (LREC’10), edited by N. Calzolari, K. Choukri, B. Maegaard, J. Mariani, J. Odijk, S. Piperidis, M. Rosner, and D. Tapias,1168–1171. Valletta, Malta: European Language Resources Association (ELRA).
- Renn, O., W. J. Burns, J. X. Kasperson, R. E. Kasperson, and P. Slovic. 1992. “The Social Amplification of Risk: Theoretical Foundations and Empirical Applications.” Journal of Social Issues 48 (4): 137–160. https://doi.org/10.1111/j.1540-4560.1992.tb01949.x.
- Reser, J. P., G. L. Bradley, and M. C. Ellul. 2014. “Encountering Climate Change: ‘Seeing’ Is More than ‘Believing.” WIREs Climate Change 5 (4): 521–537. https://doi.org/10.1002/wcc.286.
- Shahid, Z., R. Kalayanamitra, B. McClafferty, D. Kepko, D. Ramgobin, R. Patel, C. S. Aggarwal, et al. 2020. “COVID-19 and Older Adults: What We Know.” Journal of the American Geriatrics Society 68 (5): 926–929. https://doi.org/10.1111/jgs.16472.
- Slovic, P. 1987. “Perception of Risk.” Science 236 (4799): 280–285. https://doi.org/10.1126/science.3563507.
- Stone, L., R. Olinky, and A. Huppert. 2007. “Seasonal Dynamics of Recurrent Epidemics.” Nature 446 (7135): 533–536. https://doi.org/10.1038/nature05638.
- Sutton, R. S., and A. G. Barto. 1998. Reinforcement Learning: An Introduction. Adaptive Computation and Machine Learning. Cambridge, MA: MIT Press.
- Trope, Y., and N. Liberman. 2010. “Construal-Level Theory of Psychological Distance.” Psychological Review 117 (2): 440–463. https://doi.org/10.1037/a0018963.
- Van der Linden, S. 2014. “On the Relationship between Personal Experience, Affect and Risk Perception: The Case of Climate Change.” European Journal of Social Psychology 44 (5): 430–440. https://doi.org/10.1002/ejsp.2008.
- Viscusi, W. K., and R. J. Zeckhauser. 2015. “The Relative Weights of Direct and Indirect Experiences in the Formation of Environmental Risk Beliefs.” Risk Analysis 35 (2): 318–331. https://doi.org/10.1111/risa.12271.
- Wachinger, G., O. Renn, C. Begg, and C. Kuhlicke. 2013. “The Risk Perception Paradox: Implications for Governance and Communication of Natural Hazards.” Risk Analysis 33 (6): 1049–1065. https://doi.org/10.1111/j.1539-6924.2012.01942.x.
- Wegwarth, O., U. Mansmann, F. Zepp, D. Lühmann, R. Hertwig, and M. Scherer. 2023. “Vaccination Intention following Receipt of Vaccine Information through Interactive Simulation vs Text among COVID-19 Vaccine-Hesitant Adults during the Omicron Wave in Germany.” JAMA Network Open 6 (2): E 2256208. https://doi.org/10.1001/jamanetworkopen.2022.56208.
- Weinstein, N. D. 1982. “Unrealistic Optimism about Susceptibility to Health Problems.” Journal of Behavioral Medicine 5 (4): 441–460. https://doi.org/10.1007/BF00845372.
Appendix
Questionnaire items: original German wording and English translation
Our questionnaires were distributed in German; here we provide translations for all items analyzed in the main text. Note that the wording of some questions (e.g. infection status) changed across waves; this is indicated below.
Risk judgment
Infection status
Response categories for both questions:
This variable was recoded from the following original categories: (1) The responses ‘Confirmed infection’ and ‘Suspected infection’ were combined into ‘yes’; (2) ‘No evidence of infection’ was coded ‘no’; (3) The remaining category ‘Does not apply/don’t know’ was left as ‘not applicable’. These questions were asked separately for six levels of social proximity. The relevant part (e.g. Have you been diagnosed …? Has some in your family been diagnosed …?) was replaced in each of the six questions. In a second step the six questions were recoded into 4 groups used in the analysis: ‘direct experience’ (1), ‘family experience’ (2), ‘network experience’ (3–6). In the case the question was answered with ‘No evidence of infection’ the fourth category ‘no experience’ was used.
Fear
Results of multilevel modeling
Additional analysis
Fear of a life-threatening disease
The responses ranging from 0 to 100 were divided by 10 to make comparison with the other measures easier.
Figure A1. Fear of a life-threatening disease by age group. Error bars represent bootstrapped 95% CIs.

Table A1. Demographic characteristics of participants (N = 15,015).
Table A2. Number of participants per wave.
Table A3. Multilevel model predicting risk judgment by experience of infection (two-way split: direct or no experience).
Table A4. Multilevel model predicting risk judgment by experience of infection (four-way split: direct, family, network, or no experience).
Table A5. Multilevel model predicting risk judgments by media mentions and google searches.