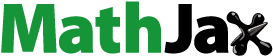
ABSTRACT
Purpose
Supply chain integration (SCI) has been considered an antecedent of sustainability performance (SP) by some studies; however, empirical findings on the nexus between SCI and SP in the existing literature are inconsistent. The study aims to provide an extensive literature review on the SCI–SP relationship.
Design/methodology/approach
We propose a conceptual research framework to investigate the SCI–SP relationship with consideration of the effects of contextual moderators. A meta-analysis is adopted to analyse the sample of 60 selected empirical papers focusing on this relationship.
Findings
The results provide evidence of a positive relationship between SCI and SP, which is affected by the two types of moderating variables: operationalisation of SP construct and control variables (industry sector, economic development, and the country's logistics capabilities). We find that SCI has the greatest impact on economic SP and the lowest impact on social SP. The SCI–SP relationship is more robust in the service industry than in the manufacturing industry. When a country's logistics capabilities increase, SCI's influence on SP becomes weaker.
Limitations/implications
The results of this study are drawn from extant empirical research, and thus the validity and reliability of these findings rely on the validity and reliability of the original studies.
Originality/value
This study is the first meta-analysis to examine the vital relationship between SCI and SP and adopted a country's logistics capabilities as a moderator. The results offer managerial insights regarding integration dimensions that positively affect various measures of SP.
1. Introduction
Sustainability has received much attention from industry and academia in the global economic context (Govindan et al. Citation2020). Pursuing economic growth is no longer the sole focus; seeking environmental and social performance has also become critical (Shen et al. Citation2017). Given the complexity of achieving sustainability performance (SP), both academia and industry have recognised the importance of supply chain integration (SCI) (Chaudhuri, Boer, and Taran Citation2018). In the extant literature, growing evidence implies that SCI can effectively increase a firm's SP in a dynamic environment (Huo, Zhao, and Lai Citation2014; Um and Kim Citation2019). Based on data from 308 Chinese manufacturing companies, Yu, Zhang, and Huo (Citation2019) argued that cooperation between consumer and supplier have positive impacts on environmental performance; similar conclusions are also drawn by other studies (Ateş et al. Citation2012; Carter and Carter Citation1998; Du, Zhang, and Feng Citation2018; Han and Huo Citation2020). In the fashion business, Li et al. (Citation2016) stated that supplier integration has a significantly positive impact on a sustainability performance. Internal integration positively affects sustainability performance (Pakurár et al. Citation2019). Across the world, the importance of SCI has been further heightened by the shock of COVID-19. During the pandemic, transportation was restricted and deliveries inconsistent. Most suppliers were unable to fulfil orders and manufacturers were not able to continue production. Despite the difficult situation, some exceptional companies exhibited great SCI ability; for example, LVMH updated its perfume factories to produce hand sanitiser and Gucci reformed its designer clothing factory to make medical supplies (Bain & Company Citation2020). This rapid business transformation requires a high SCI degree, including fast raw-material sourcing, product development and testing, and efficient logistics and transportation service.
Though many scholars agree that SCI has a positive impact on SP, different opinions have arisen over the years. For instance, Danese and Romano (Citation2011), after analyzing data from 200 global manufacturing plants, argued that customer integration has no positive effect on efficiency in general. Mao, Zhang, and Li (Citation2017) revealed that internal integration is only partially significant in terms of financial performance, while Laari et al. (Citation2016) even argued that internal environmental collaboration affects sustainability performance insignificantly—a result also supported by other studies (Du, Zhang, and Feng Citation2018; Shin, Park, and Park Citation2019).
In addition, several meta-analyses have been conducted investigating the relationship between SCI and sustainability performance (Chang et al. Citation2016; Leuschner, Rogers, and Charvet Citation2013; Mackelprang et al. Citation2014). Of these, only Chang et al. (Citation2016) considered the function of contextual variables in moderating the relationship between SCI and performance. Context refers to external environmental contingencies and internal contingencies (Danese, Molinaro, and Romano Citation2020). In this paper, we study the moderating effect of three contextual variables: the economic development level of the sample country, industry type, and the country's logistics capabilities, as suggested by Danese, Molinaro, and Romano (Citation2020).
Driven by these historically mixed findings on the relationship between SCI and SP and the lack of research on the role of contextual variables, we conduct a meta-analytical study to aggregate all the related empirical research to uncover the generalised interaction between SCI and SP. Sustainability performance may contain environmental, social, and economic performance. Through a comprehensive literature review, we identified 60 articles published between 1998 and 2021 that surveyed a total of 15,332 companies. Our study differs from the most recent meta-analyses (Ataseven and Nair Citation2017) in two ways. First, our study focuses on the relationship between SCI and SP, rather than being confined to economic or operational aspects. Second, we carefully adopt a country's logistics capabilities as a moderator.
The remainder of the paper is structured as follows. Section 2 includes literature review, coding process, research framework development and hypotheses proposition. Section 3 focuses on the details of the research method; publication bias and heterogeneity issues are discussed and resolved. Section 4 presents the meta-analytical results, and Section 5 contains further discussion.
2. Literature review and research framework
2.1. Sampling and literature review
In this research, a meta-analysis approach is adopted to reveal the relation between SCI and SP. Meta-analysis has been prevalently conducted for analysing the quantitative findings of prior published research. Based on the comprehensive evaluation, the research results’ validity can be examined to give an overview of the existing research and propose insights on both theory and practice for future research. Our study focuses on the empirical study in which supply chain integration indicators have functioned as an independent variable, and those of supply chain SP are dependent variables. All the procedures follow the instructions and procedures of Borenstein et al. (Citation2009) and Hunter and Schmidt (Citation2014).
Researches published by peer-reviewed journals in English from 1987 until December 2021 are our primary focuses, in line with the fact that sustainability was initially proposed by the UN Brundtland Commission in 1987. The selected databases are ABI/INFORM Complete, Business Source Complete (EBSCO), Emerald, Scopus, and Web of Science. We link the search strings to ‘supply chain,’ ‘integration,’ ‘sustainability,’ and ‘performance,’ consistent with prior literature (Chen et al. Citation2017). ‘Supply chain,’ ‘supplier,’ and ‘customer’ are commonly used key search terms for the supply chain. The keywords for ‘integration’ include ‘integration,’ ‘collaboration,’ and ‘cooperation.’ For sustainability, we adopt ‘green,’ ‘sustainable*,’ ‘corporate social responsibility,’ and ‘environment*’ as search terms, consistent with prior research on sustainable supply chain management (Carter and Rogers Citation2008). The search terms used for performance are ‘performance,’ ‘benefit,’ ‘return,’ ‘profit*,’ ‘firm performance,’ ‘green performance,’ and ‘sustainability performance.’
The search terms above were combined to collect titles and keywords of articles from the databases. Further sifting allowed the removal of duplicate and irrelevant articles. An initial sample of 2,823 journals was obtained. Next, we identified valid samples via three selection criteria. First, the articles should use an empirical methodology in the study of SCI's effect on SP. Second, the articles should report Pearson's correlation coefficient between SCI and SP. If such coefficient was not provided, other statistics such as the F-statistic should be reported to obtain the corrected correlation coefficient via the calculation procedures shown in Appendix A. Third, the articles should have distinct data sets. If several papers used the same data set, we selected only one of them. After applying these three criteria, 60 papers remained. Finally, records of effect size were extracted from these 60 papers. The distribution of the selected papers is shown in .
Table 1. The distribution of selected papers.
In , the theoretical lens adopted in the sampled articles is summarised. 26 papers (43%) (did not specify the applied theory; in the remainder, a variety of theories are evident, including the resource-based view (RBV), the natural resource-based view (NRBV), resource dependence theory (RDT), the knowledge-based view (KBV), and social capital theory, with the RBV (17%) and the NRBV (8%) the most widely applied. also summarises the analysis methods of the sampled articles. Most of the reviewed papers adopted either structural equation modelling (SEM) (60%) or regression analysis (32%) for data analysis.
Table 2. Distribution of theoretical lens and analysis methods.
2.2. Coding
2.2.1. Coding process
Prior research includes a variety of constructs for SCI and SP. To reach an agreement, two authors independently collected essential information (e.g. effect size, sample size) from the selected papers. If there was any disagreement, the two authors reviewed the paper and had an in-depth discussion until they reached an agreement. Otherwise, a third expert was invited to give their opinion on the disputed point. To ensure that the concepts had been accepted and applied in prior research, this research followed a strict coding process (Wang, Lai, and Shou Citation2018; Govindan et al. Citation2020). The inter-coder reliability was 100%, implying our coding results, as shown in , did not lose consistency or continuity.
Table 3. Coding results.
2.2.2. Independent variable
In this research, SCI was chosen as our independent variable. In prior research, numerous definitions of SCI have been given. Some define SCI as seamless interaction built with suppliers, manufacturers, and customers for strategic alignment and global rather than local optimisation (Devaraj, Krajewski, and Wei Citation2007), which implies that SCI is a strategically external integrated process or practice among supply chain parties. In this study, SCI is defined as ‘the degree to which a manufacturer strategically collaborates with its supply chain partners and collaboratively manages intra – and inter-organisation processes’ (Flynn, Huo, and Zhao Citation2010, 58). Following Ataseven and Nair (Citation2017), we characterise SCI into three types: 1. Customer integration, 2. supplier integration, and 3. internal integration, defined as follows:
Customer integration (CI) refers to the ‘close collaboration and information sharing activities with key customers that provide the firm with strategic insights into market expectations and opportunities, ultimately enabling a more efficient and effective response to customer needs’ (Schoenherr and Swink Citation2012). Famiyeh et al. (Citation2018) pointed out that manufacturing companies have begun implementing supply chain management in response to customer demand for environmentally sustainable products and services, with the potential to improve business performance. CI helps a firm arrange its internal operations in close alignment with market demands (Ataseven and Nair Citation2017). The coordination of inventory control, demand forecasting, and delivery tracking between a firm and its customers is the sustainable example of CI (Wong, Lai, and Cheng Citation2011b).
Supplier integration (SI) represents the ‘coordination and information sharing activities with key suppliers that provide the firm with insights into suppliers’ processes, capabilities and constraints, ultimately enabling more effective planning and forecasting, product and process design, and transaction management’ (Schoenherr and Swink Citation2012). The greenness of manufacturing product depends directly on raw materials provided by suppliers (Pan et al. Citation2020). At the same time, on the basis of cooperation with suppliers, manufacturing enterprises promote the application of green technology innovation and improve the sustainable environmental performance of enterprises (Pan et al. Citation2020). Critical SI practices include joint product development, joint inventory management and quality control, and the long-term sustainable buyer–supplier relationship (Li et al. Citation2016).
Internal integration (II) is the ‘degree to which a firm can structure its organisational practices, procedures and behaviours into collaborative, synchronised and manageable processes in order to fulfil customer requirements’ (Zhao et al. Citation2011). II provides a platform for sharing information within internal functions and provides capabilities for processing, absorbing, analyzing, and implementing information gathered from supplier and customer integration (Flynn, Huo, and Zhao Citation2010). II enables better intra-firm alignment and utilisation of each department's strengths and competencies to pursue excellence (Schoenherr and Swink Citation2012), and includes data and information sharing, strategic collaboration for logistics, continuous process improvement and joint new product development (Feng et al. Citation2017; Wong, Lai, and Cheng Citation2011b).
Several studies on the relationship between SCI and SP have identified the impact of CI, SI, and II. For example, Cheng, Chaudhuri, and Farooq (Citation2016) argued that II is not significantly related to performance, but that the relationship is mediated by external integration. Lai, Wong, and Venus Lun (Citation2014) revealed that higher CI would help a company achieve better market performance. In our sample, 18 of 60 records focus on internal SCI practices, while the remainder are related to CI (19 papers) and SI (23 papers).
2.2.3. Dependent variable
In this meta-analysis, SP is the dependent variable. According to the definition of sustainability, essential aspects of SP include the expectations of the environment, society, and stakeholders in the long term. Elkington (Citation1998) defined SP in terms of the ‘triple bottom line’ (TBL) of economic, environmental, and social performance; this was also empirically applied in Shen’s (Citation2014) work. The editorial by Bentahar and Benzidia (Citation2018) and the literature review conducted by Chen et al. (Citation2017) also suggested measuring SP in terms of these three aspects (economic, environmental, and social):
Economic performance refers to profitability, efficiency, and productivity in general and serves as the most critical reason for integration practices in the supply chain (e.g. Abdallah, Abdallah, and Saleh Citation2017; Chavez et al. Citation2015; Cheng, Chaudhuri, and Farooq Citation2016; Kim Citation2009). It is possible for firms to pursue new economic objectives when they have strong connections, which generates positive externalities, which are conducive to improving economic performance (Lee and Kim Citation2011). The closer the connection between the enterprise and the supplier, the greater the likelihood of cooperation between the two parties. Measurement of economic performance in the reviewed literature includes growth in sales, profits, market share, and return on investment (Flynn, Huo, and Zhao Citation2010).
In terms of environmental performance, companies limit and maintain control over environmental cooperation activities with suppliers through the use of contracts, risk sharing arrangements, and management systems to reduce environment hazards (Hassan, Abindin, and Nordin Citation2018). At the same time, these companies and suppliers have developed consistent cultures and goals through the exchange and sharing of environmental knowledge (Zhang et al. Citation2020), promoting a willingness on both sides to share business in partnerships to better understand shared values (Du, Zhang, and Feng Citation2018; Yu, Zhang, and Huo Citation2019).
Social performance is related to the evaluation of an organisation's effects on employees, the local community, and the whole society in terms of creating social welfare, including ethical programmes, employee education and training, health and safety, and human resource management (e.g. Alfalla-Luque, Marin-Garcia, and Medina-Lopez Citation2015; Delic, Eyers, and Mikulic Citation2019; Gimenez, Sierra, and Rodon Citation2012; Longoni, Golini, and Cagliano Citation2014; Mandal Citation2017). Businesses can improve their social relationships by integrating various green practices to strengthen collaboration (Longoni, Golini, and Cagliano Citation2014). With frequent interactions, mutual trust and interdependence increase, social networks gradually take shape, facilitating access to more valuable resources for both parties (Delic, Eyers, and Mikulic Citation2019; Gimenez, Sierra, and Rodon Citation2012).
2.2.4. Control variables
Control variables in a meta-analysis are those variables that the primary relationship is conditional on; these can change the direction or magnitude of the main effect (Wang, Lai, and Shou Citation2018). Consistent with prior research (Graham and Potter Citation2015; He et al. Citation2014; Liu et al. Citation2018), we extracted industry type (manufacturing or service) and the country's economic development level (developed or developing) as control variables in our meta-analysis. For instance, Liu et al. (Citation2018) found a difference between China and Western firms in the automobile industry, such that, in the Chinese context, the relationship between internal SI and successful implementation of an environmental strategy is significant, but not in Europe. Schoenherr and Swink (Citation2012) and Singh and Power (Citation2014) found that II can contribute to SP in the manufacturing industry. However, Mao, Zhang, and Li (Citation2017) and Laari et al. (Citation2016) failed to prove the significance of the impact of II on SP in the same industry. In the selected articles, the service industry (9 articles), including exporting, hotels, hospitals, logistics, and retailing, received less attention than manufacturing sectors (45 articles). We identified three types of industry: manufacturing, service, and mixed. When multiple industries are investigated, the research is recognised as ‘mixed,’ such as Panahifar et al. (Citation2018) and Yuen and Thai (Citation2017). A country's economic development level is coded as developed, developing or both; ‘mixed’ refers to international surveys in both developing and developed regions. To clarify economic region, we use criterion from World Bank high-income economies to separate the regions into the group of developed or developing.
In addition to economic development level and industry type, we also introduced a country's logistics capabilities as a control variable. In general, efficient and effective logistics operation, as a dynamic capability, is of great importance to achieve competitive advantage (Sandberg and Abrahamsson Citation2011). However, based on the observation by Danese, Molinaro, and Romano (Citation2020), few articles have investigated the moderating effect of such capabilities on the relationship between SCI and SP, especially from the perspective of country. Thus, thus study also included country's logistics capabilities into control variables. The aggregated logistics performance index (LPI) from the World Bank was adapted to measure each country's logistics capabilities. LPI is also used in Wiengarten et al. (Citation2014) to explore logistics capabilities in external SCI. In terms of aggregated LPI ranking, Germany has the highest score (4.19 on a scale of 1–5) among the 167 countries, while Somalia has the lowest (2.0). To see each country's logistics capabilities intuitively, we define this capability as high if the score is more than 3.5, average if it is less than 3.5 but larger than 3.0, and below average if less than 3.0.
2.3. Research framework and hypotheses
2.3.1. The SCI–SP relationship
RDT is adopted as the theoretical lens to develop the research hypotheses in our study. RDT argues that external and internal resources are key to companies to gaining and sustaining competitive advantages (Esfahbodi, Zhang, and Watson Citation2016). RDT helps to understand how and to what extent SCI creates mutual benefits among players in the chain (Shin, Park, and Park Citation2019). Jointly possessed or controlled resources among supply chain partners are often viewed as the focal firm's source of capability development. In a collaborative context, suppliers and customers share mutual goals with the focal firm, which creates a collaborative atmosphere for knowledge and information sharing. With joint planning and decision-making, the focal firm can align with partners, adopt unified standards, share advanced technologies, and obtain the newly added competitive advantages (Vachon and Klassen Citation2008). Within the firm, integrating multiple departments (e.g. logistics, production, marketing, and R&D) can attain strategic alliance of product/service design (Braunscheidel and Suresh Citation2009) and maintain and enhance competitive capability (Kim Citation2006), which has been proved to positively affect sustainability performance (Flynn, Huo, and Zhao Citation2010; Liu and Lee Citation2018; Zhao et al. Citation2011). As demonstrated by empirical tests, cross-functional coordination and supplier involvement may also contribute to eco-innovation of new product design and environmental logistics, subsequently reflected in improved profits, efficiency, competitiveness, market share, and environmental brand image; namely, excellent economic, environmental, and social performance (Mitra and Datta Citation2014). Therefore, we propose the following hypothesis:
H1: SCI is positively associated with SP.
2.3.2. Constructs of SCI
In our study, SCI is categorised into the CI, SI, and II. It has been a research area of active interest to study supplier and customer relationships as well as internal integration and sustainability performance in recent years and a large amount of literature emphasises the importance of the SCI concept (Flynn, Huo, and Zhao Citation2010). There is evidence that customer collaboration may be more effective than supplier collaboration in improving environmental performance (Ralston et al. Citation2015). Three SCI dimensions (CI, SI, and II) have been shown to produce different environmental, cost, and economic benefits (Chavez et al. Citation2015; Cheng, Chaudhuri, and Farooq Citation2016; Kim Citation2009). Meanwhile, the association between SCI and sustainability tends to differ depending on whether internal or external integration is being considered (Braunscheidel and Suresh Citation2009). For instance, Zhao et al. (Citation2011) found that II is positively related to business and operational performance, while CI is only directly associated with operational performance, and SI is not a direct contributor to either. Cheng, Chaudhuri, and Farooq (Citation2016) argued that external integration significantly affects operational performance of a networked plant, whereas II does not. Mao, Zhang, and Li (Citation2017) argued that internal environmental integration improves environmental performance but hinders a firm's financial performance, whereas external green integration improves both environmental performance and financial performance. Consequently, both internal and external integration may be beneficial for SP, but RDT theory implies that external (customer or supplier) relationships lead to tight interconnection with other partners, which also reflect the superior status of the cross-functional relationship. Therefore, we propose the following hypotheses:
H2a: Each type of integration—customer, supplier, and internal—is positively associated with SP.
H2b: The effect of external (customer or supplier) integration is stronger than that of II on SP.
2.3.3. Operationalisation of SP
SP is a multidimensional construct and has multiple measurements. Most studies focus on SCI's effect on economic performance by measuring operational performance, cost performance, quality performance, business performance, and market performance (Flynn, Huo, and Zhao Citation2010; Thai and Jie Citation2018; Wong, Lai, and Cheng Citation2011b). Some have emphasised SCI's green performance (Ateş et al. Citation2012; Liu et al. Citation2018; Yu, Zhang, and Huo Citation2019), but seldom measure social performance in the relationship between SCI and SP (Gimenez, Sierra, and Rodon Citation2012; Hassan, Abindin, and Nordin Citation2018). Gimenez, Sierra, and Rodon (Citation2012) argued that implementing collaborative practices with supply chain partners has a statistically significant impact on the TBL. However, internal social initiatives contribute to better social and environmental performance, but not to economic benefits regarding unit manufacturing cost. However, some findings suggest that enhanced environmental performance can increase economic returns (Liu et al. Citation2018). Hence, measurements of SP lead to differing conclusions on the SCI–SP relationship. Therefore, we propose the following:
H3: The SCI–SP relationship varies with the measurements of SP.
2.3.4. Control variables
In the reviewed literature, control variables are added to ensure reliability and help explore the main relationship between key variables. Our sampled research collected data from different industrial backgrounds across various economic regions. The influence of control variables in terms of industry type and the country's economic development level and logistics capabilities conceivably affect SCI implementation and performance (Devaraj, Krajewski, and Wei Citation2007; Kim Citation2006; Mao, Zhang, and Li Citation2017; Yu, Zhang, and Huo Citation2019). Hence, we consider industry type, economic development level, and a country's logistics capabilities as contextual factors in the relationship between SCI and SP.
Firm activities are strongly related to industry type, and different industries are subject to different market or regulatory pressures regarding sustainability issues. Because of the different regulations and standards, firms may conduct various proactive or reactive SCI schemes, leading to different sustainability outcomes (Mao, Zhang, and Li Citation2017). Except for institutional pressure from authorities and other stakeholders, industry-related characteristics, such as product and market complexity (Wong, Lai, and Bernroider Citation2015), foreign investment (Bardy, Drew, and Kennedy Citation2012), and pollution level (Golgeci et al., Citation2018), also affect firms’ collaboration practices and, consequently, performance. Most extant studies on SCI and SP are based on manufacturing industries (Errassafi, Abbar, and Benabbou Citation2019; He et al. Citation2014; Liu and Lee Citation2018); much less consideration has been given to service sectors, such as logistics (Laari et al. Citation2016; Liu and Lee Citation2018; Yuen and Thai Citation2017) and hospitals (Abdallah, Abdallah, and Saleh Citation2017; Cho et al. Citation2019; Mandal Citation2017). In light of the diversity in the industries in our sample and the different characteristics from the cross-industry perspective, we have:
H4: The SCI–SP relationship varies with industry type.
H5: The SCI–SP relationship varies with economic development level.
H6: The SCI–SI relationship is stronger when the level of a country's logistics capabilities is lower.
3. Research method
3.1. Meta-analysis process
In this study, the meta-analytic approach is adopted to analyse the effect of SCI practice on SP. As mentioned in Hunter and Schmidt (Citation2014), meta-analysis provides a comprehensive and generalised conclusion of extant empirical studies considering the differentiation across individual research projects. With limited available resources and accessible sample size, each research project can only focus on segmented fields, and fails to generalise the findings (Rosenbusch, Brinckmann, and Bausch Citation2011). Meta-analysis can calculate the population effect size, based on the correlation coefficient reported in each sampled empirical study. In our case, if the correlation was not given, we collected the values of Student's ,
-ratios, Chi-square, and Cohen's
, which can be converted into effect size via the formula given by Hunter and Schmidt (Citation2014). The population effect size captures the pooled sampled studies’ mean effect size and reflects the generalised correlation between independent variables and dependent variables.
We first obtained the sampled studies via the procedures discussed in Section 2 and present the coding results in . Comprehensive Meta-Analysis (CMA) version 3.0 was used to obtain the aggregated effect size and conduct the sample tests for publication bias and heterogeneity based on the coding results.
3.2. Publication bias
In our study, confidence intervals are chosen since they are used in a frequentist confidence interval prior to data collection. Credibility intervals in a Bayesian credible interval are used after data is collected (Hunter and Schmidt Citation2014). Furthermore, z-scores were calculated in order to determine whether the effect sizes within the different groups were statistically significant.
Publication bias occurs in the academic publishing arena as a result of supporting rather than rejecting hypotheses contributing to the probability of acceptance for publication; this tendency implies that the resulting published studies then fail to truly represent the research field's overall characteristics (Borenstein et al. Citation2009). To examine the possibility of publication bias, we estimated the sampled studies before meta-analysis by the fail-safe (Borenstein et al. Citation2009). The fail-safe
(
) is calculated to identify the required number of studies with zero-effect size to achieve an insignificant
-value; it can be obtained via the formula:
(Orwin Citation1983), where N is the number of selected studies,
is the average effect size, and
the criterion value. To maintain consistency with Wang, Lai, and Shou (Citation2018), we adopted a threshold of
at the 95% confidence level:
, where
should be larger than the threshold. In this research, the fail-safe
is 1,5532
, which significantly exceeds 310 (
). The results of the fail-safe N imply that publication bias is under control in our sample.
3.3. Heterogeneity
Heterogeneity represents the significance of difference among sampled studies in our research. We conduct heterogeneity analysis with the two most frequently used methods—the -test and the
index—to statistically detect the ‘significance and proportion of observed variance in effect size attributed to systematic cross-samples variability’ (Fang and Zhang Citation2018, 1073). As claimed by Huedo-Medina et al. (Citation2006), the
-test can check the existence of heterogeneity, while the
index can point out directly the degree of heterogeneity. If the
-value is significant, heterogeneity in the sample can affect the results. According to Higgins and Thompson (Citation2002), the
index can be classified into three levels—25%, 50%, and 75%—reflecting a low, moderate, and high level of heterogeneity. According to Borenstein et al. (Citation2009), the fixed-effect model should be applied on identifying studies with similar conditions. If high heterogeneity is detected, the random-effect model should be applied (Borenstein et al. Citation2009).
presents the results of the heterogeneity analysis. The -value 1,327.64 is significant at the confidence level of 0.05, which implies that the results of the meta-analysis would be affected by the sampled studies’ heterogeneity. Further, the corresponding
value is 94.26%, which quantitively confirms the heterogeneity in our sample. Hence, a random-effect model is applied in this meta-analysis, considering the significant systematic difference between the sample articles.
Table 4. The overall meta-analysis results.
4. Results
4.1. The SP effect of SCI
The meta-analysis results on the correlation between SCI and SP are shown in . We evaluated the correlation effect using the guidelines given by Cohen et al. (Citation2003). A weak correlation is obtained with an effect size less than 0.10, while the moderate correlation is 0.10–0.30, and a strong correlation is higher than 0.30.
Our meta-analysis results show that the overall SCI–SP relationship is positive, strong (r = 0.44), and significant (Z = 8.99, P < 0.001) at the 95% confidence level. The corresponding confidence interval of (0.32, 0.50) does not include 0, which supports SCI's overall positive effect on firm SP; therefore, hypothesis H1 is accepted.
The effects of customer (r = 0.32, P < 0.001), supplier (r = 0.34, P < 0.001) and internal (r = 0.54, P < 0.001) integration are all positive and significant. All three confidence intervals exclude 0, which confirms the positive impact of CI, SI, and II on SP; therefore, H2a is supported. However, our results show that II has a stronger impact on SP than either external integration component (customer or supplier); hence, H2b is rejected.
4.2. Moderating effects
4.2.1. Operationalisation of SP
In our study, moderating effects are investigated via three control variables; namely, the measurement of SP, industry type, economic development level, and the country's logistics capabilities, measured by the LPI. The meta-analysis results for the control variables are presented in . SP is measured in terms of three categories (economic, environmental, and social) with three effect sizes (r = 0.54; r = 0.45; r = 0.36) respectively. The results are significant at the 95% confidence level (Z = 7.25, P < 0.001; Z = 8.13, P < 0.001; Z = 7.56, P < 0.001). SCI has strong effects on all three aspects of SP, among which the effect on economic performance is the strongest, followed by environmental and social performance. Therefore, hypothesis H3 is supported; the SCI–SP relationship does vary with the measurement of SP.
Table 5. Meta-analytic results of the moderating results.
4.2.2. Control variables
Three subgroups in terms of industry type were examined to provide evidence for hypothesis H4. Results for the manufacturing sector (r = 0.36, P < 0.001), service sector (r = 0.46, P < 0.001), and mixed industries (r = 0.73, P = 0.003) show that industry type positively moderates the SCI–SP relationship. In investigations of the manufacturing sector, SCI's effect on SP is comparatively weaker than for the service or mixed industry. The strongest correlation is captured in the mixed industry, followed by the service industry and then the manufacturing industry. These statistical results confirm that the relationship between SCI and SP varies by industry; therefore, H4 is supported.
In addition, in the three subgroups of economic region (developing, developed, and mixed), the statistics show that the effects of SCI on SP are positive and significant (r = 0.5, P < 0.001; r = 0.44, P < 0.001; r = 0.29, P < 0.001) at the 95% confidence level. Based on these statistics, the SCI–SP relationship is the strongest in developing countries (r = 0.50) than in developed countries (r = 0.44), but the difference is not large. In mixed regions (developed and developing), the effect of SCI on SP is rather modest (r = 0.29). It can be said that the SCI–SP relationship varies with the country's economic development levels; hence, H5 is accepted.
Last, we present the moderating effect of the country's logistics capabilities on the SCI–SP relationship. As discussed in Section 2.2, logistics capabilities are categorised as high, average, and below average. In , we can see that the SCI–SP relationship is strongest in countries with logistics capabilities below average (r = 0.65, P < 0.001), less strong in countries with average logistics capabilities (r = 0.48, P < 0.001), and weakest in countries with a high level of logistics capabilities (r = 0.39, P < 0.001). In general, the SCI–SP relationship is stronger when a country's logistics capabilities are lower; therefore, H6 is supported.
5. Discussion
5.1 Theoretical contributions
Reviewing the prior research, findings on the effect of SCI on SP are inconsistent and even contradictory. This meta-analytical study selects all the extant empirical findings to provide a synthesis, integration, and interpretation of SCI and SP's nexus. Besides, the moderating effects of operationalisation and control variables on the relationship have also been considered in our research.
First, the meta-analysis confirms the significant and positive relationship between SCI and SP, consistent with some previous conclusions (Ataseven and Nair Citation2017; Chen et al. Citation2017; Geng, Mansouri, and Aktas Citation2017). Our results refute several studies’ conclusions (Laari et al. Citation2016; Mao, Zhang, and Li Citation2017; Willis, Genchev, and Chen Citation2016). Willis, Genchev, and Chen (Citation2016) analyzed survey data from 92 Indian companies and found that II has an insignificant effect on firms’ flexibility performance, which can be classified as economic performance (Killick Citation1995). Mao, Zhang, and Li (Citation2017) proposed that the effects of internal low-carbon integration is only partially significant for economic performance. Their findings are refuted by our results, which confirm the differential effect of SCI's three constructs. Both internal and external (customer or supplier) integration are positively and significantly associated with SP. We even found that II has a more substantial average correlation with SP than CI or SI. One possible reason is that a company should integrate internally before seeking external integration (Ataseven and Nair Citation2017). Consequently, the influence of II on SP should be stronger than CI or SI.
Second, our meta results confirm that SCI has overall strong positive influences on all three facets of SP and that the SCI–SP relationship varies with SP measurement. The positive relationship between SCI and the TBL has been examined by Gimenez, Sierra, and Rodon (Citation2012). The nature of SCI as a goal-achieving tool for mutual strategic schemes may explain this. SCI focuses on global optimisation rather than local optimisation within one department or firm. With different strategic foci, SCI enables focal firms to promote different facets of SP. Consistent with RDT, both cross-departmental alliances and supply chain partnerships can break boundaries of information, knowledge, and resources to gain sustainable competitive advantages. Darmawan, Wong, and Thorstenson (Citation2021), for example, emphasised the importance of supply chain design with coordinated inventory control. Shou et al. (Citation2021) also argued that matching traceability and supply chain collaboration can help firms achieve superior operational performance. Therefore, a solid upstream or downstream integration fosters relational resources and capability, which are hard to imitate or replace (Chang et al. Citation2016). II also yields optimal utilisation of resources among different departments. Hence, the outcome of SCI initiatives (e.g. resource complementarity, coordination of production process, information sharing and knowledge creation, and mutual compliance) contribute to shared strategic goals (e.g. sustainability). As shown in our results, SCI has a stronger effect on economic SP than environmental or social SP. This finding emphasises the economic aspect of SCI. However, the reliability of this conclusion remains to be examined due to the limited sample of articles focusing on environmental performance and social performance.
The context of SCI implementation may affect the consequent SP. In the sampled articles, industry and economic development levels are often adopted as control variables, explaining the variance in the SCI–SP relationship. As for industry, the results confirm that the SCI–SP relationship is stronger in the mixed industry sector than in the service or manufacturing industries. The different effects of SCI may be related to industry characteristics, such as average market margins, inventory levels, concentration ratio of the industry and competition intensity (Geng, Mansouri, and Aktas Citation2017) or the different nature of product/service process characteristics (Ataseven and Nair Citation2017). It would be worth examining why the service rather than the manufacturing industry is more likely to gain SP from SCI practices. Yuen and Thai (Citation2017) compared SCI's impact on operational performance between manufacturing and service supply chains and found that service supply chain receives more influences from external SCI than manufacturing supply chains, but the difference among internal SCI is not significant. Our results partially support their first finding but refute their second finding.
SCI practices in varying economic environments are found to have differentiated impacts on SP. In our findings, SCI implementation in a global business environment (mixed regions) shows weak SP relative to a single economic region (developing or developed region). However, mixed regions represent multiple data sources, which would provide a more comprehensive understanding for scholars on the effects of SCI implementation. Moreover, country-level differences, such as national culture (Chang et al. Citation2016) and logistics capabilities (Wiengarten et al. Citation2014), would affect SCI implementation and the corresponding outcomes. That may be the reason why the SCI–SP relationship is stronger in developing than in developed countries.
The most innovative part of our study is the examining of the moderating effect of a country's logistics capabilities, measured by LPI. We find that the SCI–SP relationship is relatively weak (r = 0.39, P < 0.001) in countries with a high LPI, but relatively high (r = 0.65, P < 0.001) in countries with below-average LPI. The relationship becomes weaker when the LPI level increases. This finding may be surprising, but is consistent with those of the study by Wiengarten et al. (Citation2014). As stated in Wiengarten et country's (Citation2014) research, strong logistics capabilities reduce the resources for supply chain partners to coordinate, but the situation does not necessarily mean that high logistics capabilities would not benefit the SCI–SP relationship. Indeed, those reduced resources can be used on other more idiosyncratic elements of the relationship to create a truly innovative SCI.
5.2. Managerial implications
Our meta-analysis results also yield several practical implications for participants in collaborative practices. First, it is beneficial for managers to adopt SCI practices because SCI practices positively influence SP. In summary, the SCI–SP relationship varies with economic development level and industry type. However, the SCI–SI relationship is stronger when the level of a country's logistics capabilities is lower. However, it might be better to build II before pursuing external integration, as we found that II has a stronger effect on SP than CI or SI. Nevertheless, II is an inside-out concept that is instrumental for external cooperation, which requires more resources at a higher implementation cost. It is not guaranteed that adopting SCI ensures better SP, which depends on problem-solving in light of uncertainty and contextual factors.
Second, this study provides managers with insights into different effects on SP from each aspect of SCI. Our results show a strong correlation between environmental and economic integration and SP. Therefore, companies that adopt a sustainability strategy are encouraged to conduct green integration initiatives, in addition to regular collaboration based on efficiency and profitability. Social integration, such as monitoring suppliers’ ethical compliance, would not hinder the general sustainable benefits, though it seems directly costly and non-financially beneficial. As for measurement of SP, our results imply that SCI activities yield better influence on environmental SP than on social aspects. In this regard, SCI is an efficient tool for green supply chain performance (e.g. waste and pollution reduction, water utilisation). The multiple measurements of SP empower companies to understand and evaluate the significance and advantages of SCI implementation.
Third, managers may usefully put more effort into developing SCI when their companies do business in both the manufacturing and service sectors, where SCI has the most effective influence. Manufacturing industry could adopt the servitization strategy. Compared with the manufacturing industry, managers of companies in the service industry could contribute to companies’ SP after developing SCI, as the effect of SCI on SP is stronger in the service industry than in the manufacturing industry. In addition, managers should also consider the country's logistics capabilities (measured by LPI) when implementing SCI. For the companies in low LPI countries, managers should put more effort into SCI than companies in high LPI countries, because the SCI–SP relationship is the most profound in countries with low LPI. However, managers do not need to balance SCI effort when their companies are located either in developing or developed countries, as the effect of SCI on SP is significantly high in both such regions. Therefore, no matter where firms are located, managers should plan their logistics and transportation properly. According to McKinsey & Company’s (Citation2020) strategic report, one of the actions firms should take to combat COVID-19 is to identify and secure logistics capacity.
6. Conclusion
With 60 reviewed empirical studies, we conducted a meta-analysis of the relationship between SCI and SP. In the subgroup analysis, the differentiated effects of internal and external (customer or supplier) integration are confirmed. The moderating effects of control variables (i.e. industry, economic development level, and country's logistics capabilities) and operationalisation of SP are also examined. Our meta-analysis results support all our hypotheses except for H2b. Our study extends Ataseven and Nair (Citation2017) by examining the moderating effect of control variables. One of the novelties is the investigation of the SCI–SP relationship considering logistics capabilities; to our best knowledge, this is the first meta-analytic research paper that adopted a country's logistics capabilities as a moderator.
Despite the contributions there remain limitations and research opportunities. First, we examined the linear relationship between SCI and SP, but there may be nonlinear relationships still to be explored. Second, although we included social performance in the analysis, we have not gone far in this field. In extant studies, few papers have explored the nexus between SCI and social SP considering ethical issues (e.g. labour, fairness, and safety). The trend of supply chain integration and sustainability performance study could focus on specific domain and green practices. Our future work could identify socially sustainable initiatives through SCI and explore the measurement of social outcomes. Last, we only verified the moderating effects of selected contextual variables (industry type, economic development level, and a country's logistics capabilities), but other control variables could be further explored, including firm size, firm age, and product customisation level. We advocate future research to examine the mediating and moderating effects of these variables, since the existing research on such mechanisms is far from comprehensive or conclusive.
Acknowledgements
The authors would like to thank the editors and anonymous reviewers for their valuable and constructive comments.
Data availability statement
The authors confirm that the data supporting the findings of this study are available within the article.
Disclosure statement
No potential conflict of interest was reported by the author(s).
Additional information
Funding
References
- Abdallah, A. B., M. I. Abdallah, and F. I. M. Saleh. 2017. “The Effect of Trust with Suppliers on Hospital Supply Chain Performance.” Benchmarking: An International Journal 24 (3): 694–715.
- Afum, E., V. Osei-Ahenkan, Y. Agyabeng-Mensah, J. A. Owusu, L. Kusi, and J. Ankomah. 2020. “Green Manufacturing Practices and Sustainable Performance among Ghanaian Manufacturing SMEs: The Explanatory Link of Green Supply Chain Integration.” Management of Environmental Quality: An International Journal. ahead-of-print.
- Agyabeng-Mensah, Y., E. Ahenkorah, E. Afum, A. Agyemang, C. Agnikpe, and F. Rogers. 2020. “Examing the Influence of Internal Green Supply Chain Practices, Green Human Resource Management and Supply Chain Environmental Cooperation on Firm Performance.” Supply Chain Management: An International Journal. ahead-of-print.
- Ahmed, W., M. S. Ashraf, and S. A. Khan, et al. 2020. “Analyzing the Impact of Environmental Collaboration among Supply Chain Stakeholders on a Firm's Sustainable Performance.” Operations Management Research 13: 4–21.
- Alfalla-Luque, R., J. A. Marin-Garcia, and C. Medina-Lopez. 2015. “An Analysis of the Direct and Mediated Effects of Employee Commitment and Supply Chain Integration on Organisational Performance.” International Journal of Production Economics 162: 242–257.
- Ataseven, C., and A. Nair. 2017. “Assessment of Supply Chain Integration and Performance Relationships: A Meta-Analytic Investigation of the Literature.” International Journal of Production Economics 185: 252–265.
- Ateş, M. A., J. Bloemhof, E. M. van Raaij, and F. Wynstra. 2012. “Proactive Environmental Strategy in a Supply Chain Context: The Mediating Role of Investments.” International Journal of Production Research 50: 1079–1095.
- Bain & Company. 2020. Supply Chain Lessons from Covid-19: Time to Refocus on Resilience, https://www.bain.com/insights/supply-chain-lessons-from-covid-19/, accessed date: 2020-12-26.
- Bardy, R., S. Drew, and T. F. Kennedy. 2012. “Foreign Investment and Ethics: How to Contribute to Social Responsibility by Doing Business in Less-Developed Countries.” Journal of Business Ethics 106 (3): 267–282.
- Bentahar, O., and S. Benzidia. 2018. “Sustainable Supply Chain Management: Trends and Challenges.” Transportation Research Part E: Logistics and Transportation Review 119: 202–204.
- Borenstein, M., L. Hedges, J. Higgins, and H. Rothstein. 2009. An Introduction to Meta-Analysis. West Sussex, UK: John Wiley & Sons.
- Braunscheidel, M. J., and N. C. Suresh. 2009. “The Organizational Antecedents of a Firm’s Supply Chain Agility for Risk Mitigation and Response.” Journal of Operations Management 27: 119–140.
- Carter, C. R., and J. R. Carter. 1998. “Interorganizational Determinants of Environmental Purchasing: Initial Evidence from the Consumer Products Industries.” Decision Sciences 29: 659–684.
- Carter, C. R., and D. S. Rogers. 2008. “A Framework of Sustainable Supply Chain Management: Moving Toward new Theory.” International Journal of Physical Distribution & Logistics Management 38: 360–387.
- Chang, W., A. E. Ellinger, K. Kim, and G. R. Franke. 2016. “Supply Chain Integration and Firm Financial Performance: A Meta-Analysis of Positional Advantage Mediation and Moderating Factors.” European Management Journal 34: 282–295.
- Chaudhuri, A., H. Boer, and Y. Taran. 2018. “Supply Chain Integration, Risk Management and Manufacturing Flexibility.” International Journal of Operations & Production Management 38 (3): 690–712.
- Chavez, R., W. Yu, C. Gimenez, B. Fynes, and F. Wiengarten. 2015. “Customer Integration and Operational Performance: The Mediating Role of Information Quality.” Decision Support Systems 80: 83–95.
- Chen, L., X. Zhao, O. Tang, L. Price, S. Zhang, and W. Zhu. 2017. “Supply Chain Collaboration for Sustainability: A Literature Review and Future Research Agenda.” International Journal of Production Economics 194: 73–87.
- Cheng, Y., A. Chaudhuri, and S. Farooq. 2016. “Interplant Coordination, Supply Chain Integration, and Operational Performance of a Plant in a Manufacturing Network: A Mediation Analysis.” Supply Chain Management: An International Journal 21: 550–568.
- Cho, M., M. A. Bonn, L. Giunipero, and J. Divers. 2019. “Restaurant Purchasing Skills and the Impacts upon Strategic Purchasing and Performance: The Roles of Supplier Integration.” International Journal of Hospitality Management 78: 293–303.
- Cohen, J., P. Cohen, S. G. West, and L. S. Aiken. 2003. Applied Multiple Regression/Correlation Analysis for the Behavioral Sciences. 3rd ed. Mahwah, NJ: Lawrence Erlbaum Associates.
- Danese, P. 2013. “Supplier Integration and Company Performance: A Configurational View.” Omega 41: 1029–1041.
- Danese, P., M. Molinaro, and P. Romano. 2020. “Investigating Fit In Supply Chain Integration: A Systematic Literature Review on Context, Practices, Performance Links.” Journal of Purchasing and Supply Management 100634.
- Danese, P., and P. Romano. 2011. “Supply Chain Integration and Efficiency Performance: A Study on the Interactions between Customer and Supplier Integration.” Supply Chain Management: An International Journal 16: 220–230.
- Darmawan, A., H. Wong, and A. Thorstenson. 2021. “Supply Chain Network Design with Coordinated Inventory Control.” Transportation Research Part E: Logistics and Transportation Review 145: 102168.
- Delic, M., D. R. Eyers, and J. Mikulic. 2019. “Additive Manufacturing: Empirical Evidence for Supply Chain Integration and Performance from the Automotive Industry.” Supply Chain Management: An International Journal 24: 604–621.
- Devaraj, S., L. Krajewski, and J. C. Wei. 2007. “Impact of Ebusiness Technologies on Operational Performance: The Role of Production Information Integration in the Supply Chain.” Journal of Operations Management 25: 1199–1216.
- Droge, C., J. Jayaram, and S. K. Vickery. 2004. “The Effects of Internal Versus External Integration Practices on Time-Based Performance and Overall Firm Performance.” Journal of Operations Management 22: 557–573.
- Du, L., Z. Zhang, and T. Feng. 2018. “Linking Green Customer and Supplier Integration with Green Innovation Performance: The Role of Internal Integration.” Business Strategy and the Environment 27: 1583–1595.
- Elkington, J. 1998. Cannibals with Forks: The Triple Bottom Line of 21st Century Business. Gabriola Island, Stony Creek: New Society Publishers.
- Eltantawy, R. A., L. Giunipero, and G. L. Fox. 2009. “A Strategic Skill Based Model of Supplier Integration and its Effect on Supply Management Performance.” Industrial Marketing Management 38: 925–936.
- Errassafi, M., H. Abbar, and Z. Benabbou. 2019. “The Mediating Effect of Internal Integration on the Relationship Between Supply Chain Integration and Operational Performance: Evidence from Moroccan Manufacturing Companies.” Journal of Industrial Engineering and Management 12: 254.
- Esfahbodi, A., Y. Zhang, and G. Watson. 2016. “Sustainable Supply Chain Management in Emerging Economies: Trade-Offs Between Environmental and Cost Performance.” International Journal of Production Economics 181: 350–366.
- Famiyeh, S., A. Kwarteng, D. Asante-Darko, and S. A. Dadzie. 2018. “Green Supply Chain Management Initiatives and Operational Competitive Performance.” Benchmarking: An International Journal 25 (2): 607–631.
- Fang, C., and J. Zhang. 2018. “Performance of Green Supply Chain Management: A Systematic Review and Meta Analysis.” Journal of Cleaner Production 183: 1064–1081.
- Feng, M., W. Yu, R. Chavez, J. Mangan, and X. Zhang. 2017. “Guanxi and Operational Performance: The Mediating Role of Supply Chain Integration.” Industrial Management & Data Systems 117: 1650–1668.
- Flynn, B. B., B. Huo, and X. Zhao. 2010. “The Impact of Supply Chain Integration on Performance: A Contingency and Configuration Approach.” Journal of Operations Management 28: 58–71.
- Geng, R., S. A. Mansouri, and E. Aktas. 2017. “The Relationship between Green Supply Chain Management and Performance: A Meta-Analysis of Empirical Evidences in Asian Emerging Economies.” International Journal of Production Economics 183: 245–258.
- Gimenez, C., V. Sierra, and J. Rodon. 2012. “Sustainable Operations: Their Impact on the Triple Bottom Line.” International Journal of Production Economics 140: 149–159.
- Golgeci, I., D. Gligor, E. Tatoglum, and O. Ayaz. 2018. “A Relational View of Environmental Performance: What Role do Environmental Collaboration and Cross-Functional Alignment Play?” Journal of Business Research 96: 35–46.
- Govindan, K., A. Rajeev, S. S. Padhi, and R. K. Pati. 2020. “Supply Chain Sustainability and Performance of Firms: A Meta-Analysis of the Literature.” Transportation Research Part E: Logistics and Transportation Review 137: 101923.
- Graham, S., and A. Potter. 2015. “Environmental Operations Management and its Links with Proactivity and Performance: A Study of the UK Food Industry.” International Journal of Production Economics 170: 146–159.
- Han, Z., and B. Huo. 2020. “The Impact of Green Supply Chain Integration on Sustainable Performance.” Industrial Management & Data Systems 120: 657–674.
- Hassan, M. G., R. Abindin, and N. Nordin. 2018. “The Impact of Customer Integration on Manufacturing Firms Sustainable Performance.” International Journal of Engineering & Technology 7: 119.
- He, Y., K. Keung Lai, H. Sun, and Y. Chen. 2014. “The Impact of Supplier Integration on Customer Integration and New Product Performance: The Mediating Role of Manufacturing Flexibility under Trust Theory.” International Journal of Production Economics 147: 260–270.
- Higgins, J. P. T., and S. G. Thompson. 2002. “Quantifying Heterogeneity in a Meta-Analysis.” Statistics in Medicine 21: 1539–1558.
- Huedo-Medina, T. B., J. Sánchez-Meca, F. Marín-Martínez, and J. Botella. 2006. “Assessing Heterogeneity in Meta-Analysis: Q Statistic or I² Index?” Psychological Methods 11: 193–206.
- Hunter, J. E., and F. L. Schmidt. 2014. Methods of Meta-Analysis: Correcting Error and Bias in Research Findings. Russell Sage Foundation, New York.
- Huo, B., Z. Han, X. Zhao, H. Zhou, C. H. Wood, and X. Zhai. 2013. “The Impact of Institutional Pressures on Supplier Integration and Financial Performance: Evidence from China.” International Journal of Production Economics 146: 82–94.
- Huo, B., X. Zhao, and F. Lai. 2014. “Supply Chain Quality Integration: Antecedents and Consequences.” IEEE Transactions on Engineering Management 61: 38–51.
- Jayaram, J., and K. Xu. 2013. “The Relative Influence of External Versus Internal Integration on Plant Performance in China.” International Journal of Production Economics 146: 59–69.
- Killick, T. 1995. “Flexibility and Economic Progress.” World Development 23 (5): 721–734.
- Kim, S. W. 2006. “Effects of Supply Chain Management Practices, Integration and Competition Capability on Performance.” Supply Chain Management: An International Journal 11: 241–248.
- Kim, S. W. 2009. “An Investigation on the Direct and Indirect Effect of Supply Chain Integration on Firm Performance.” International Journal of Production Economics 119: 328–346.
- Laari, S., T. Solakivi, J. Töyli, and L. Ojala. 2016. “Performance Outcomes of Environmental Collaboration.” Baltic Journal of Management 11: 430–451.
- Lai, K., C. W. Y. Wong, and Y. H. Venus Lun. 2014. “The Role of Customer Integration in Extended Producer Responsibility: A Study of Chinese Export Manufacturers.” International Journal of Production Economics 147: 284–293.
- Lee, K. H., and J. W. Kim. 2011. “Integrating Suppliers into Green Product Innovation Development: An Empirical Case Study in the Semiconductor Industry.” Business Strategy and the Environment 20 (8): 527–538.
- Leuschner, R., D. S. Rogers, and F. F. Charvet. 2013. “A Meta-Analysis of Supply Chain Integration and Firm Performance.” Journal of Supply Chain Management 49: 34–57.
- Li, W.-Y., P.-S. Chow, T.-M. Choi, and H.-L. Chan. 2016. “Supplier Integration, Green Sustainability Programs, and Financial Performance of Fashion Enterprises Under Global Financial Crisis.” Journal of Cleaner Production 135: 57–70.
- Li, E. L., L. Zhou, and A. Wu. 2017. “The Supply-Side of Environmental Sustainability and Export Performance: The Role of Knowledge Integration and International Buyer Involvement.” International Business Review 26: 724–735.
- Liu, Y., C. Blome, J. Sanderson, and A. Paulraj. 2018. “Supply Chain Integration Capabilities, Green Design Strategy and Performance: A Comparative Study in the Auto Industry.” Supply Chain Management: An International Journal 23: 431–443.
- Liu, C.-L., and M.-Y. Lee. 2018. “Integration, Supply Chain Resilience, and Service Performance in Third-Party Logistics Providers.” The International Journal of Logistics Management 29: 5–21.
- Longoni, A., R. Golini, and R. Cagliano. 2014. “The Role of new Forms of Work Organization in Developing Sustainability Strategies in Operations.” International Journal of Production Economics 147: 147–160.
- Mackelprang, A. W., J. L. Robinson, E. Bernardes, and G. S. Webb. 2014. “The Relationship between Strategic Supply Chain Integration and Performance: A Meta-Analytic Evaluation and Implications for Supply Chain Management Research.” Journal of Business Logistics 35: 71–96.
- Mandal, S. 2017. “The Influence of Dynamic Capabilities on Hospital-Supplier Collaboration and Hospital Supply Chain Performance.” International Journal of Operations & Production Management 37: 664–684.
- Mao, Z., S. Zhang, and X. Li. 2017. “Low Carbon Supply Chain Firm Integration and Firm Performance in China.” Journal of Cleaner Production 153: 354–361.
- McKinsey & Company. 2020. Risk, Resilience, and Rebalancing in Global Value Chains, https://www.mckinsey.com/business-functions/operations/our-insights/risk-resilience-and-rebalancing-in-global-value-chains, accessed date: 2020-12-27.
- Mitra, S., and P. P. Datta. 2014. “Adoption of Green Supply Chain Management Practices and Their Impact on Performance: An Exploratory Study of Indian Manufacturing Firms.” International Journal of Production Research 52: 2085–2107.
- Munir, M., M. S. S. Jajja, K. Chatha, and S. Farooq. 2020. “Supply Chain Risk Management and Operational Performance: The Enabling Role of Supply Chain Integration.” International Journal of Production Economics 227: 107667.
- Orwin, R. G. 1983. “A Fail-Safe N for Effect Size in Meta-Analysis.” Journal of Educational Statistics 8: 157–159.
- Pakurár, M., H. Haddad, J. Nagy, J. Popp, and J. Oláh. 2019. “The Impact of Supply Chain Integration and Internal Control on Financial Performance in the Jordanian Banking Sector.” Sustainability 11: 1248.
- Pan, X., X. Pan, M. Song, and R. Guo. 2020. “The Influence of Green Supply Chain Management on Manufacturing Enterprise Performance: Moderating Effect of Collaborative Communication.” Production Planning & Control 31 (2-3): 245–258.
- Panahifar, F., P. J. Byrne, M. A. Salam, and C. Heavey. 2018. “Supply Chain Collaboration and Firm’s Performance.” Journal of Enterprise Information Management 31: 358–379.
- Rajaguru, R., and M. J. Matanda. 2019. “Role of Compatibility and Supply Chain Process Integration in Facilitating Supply Chain Capabilities and Organizational Performance.” Supply Chain Management: An International Journal 24: 301–316.
- Ralston, P. M., J. Blackhurst, D. E. Cantor, and M. R. Crum. 2015. “A Structure-Conduct-Performance Perspective of How Strategic Supply Chain Integration Affects Firm Performance.” Supply Chain Management: An International Journal 51: 47–64.
- Rehman, S. U., A. Bhatti, S. Kraus, and J. J. M. Ferreira. 2021. “The Role of Environmental Management Control Systems for Ecological Sustainability and Sustainable Performance.” Management Decision 59 (9): 2217–2237.
- Rosenbusch, N., J. Brinckmann, and A. Bausch. 2011. “Is Innovation Always Beneficial? A Meta-Analysis of the Relationship between Innovation and Performance in SMEs.” Journal of Business Venturing 26: 441–457.
- Sandberg, E., and M. Abrahamsson. 2011. “Logistics Capabilities for Sustainable Competitive Advantage.” International Journal of Logistics Research and Applications 14: 61–75.
- Schoenherr, T., and M. Swink. 2012. “Revisiting the Arcs of Integration: Cross-Validations and Extensions.” Journal of Operations Management 30: 99–115.
- Shen, B. 2014. “Sustainable Fashion Supply Chain: Lessons from H&M.” Sustainability 6: 6236–6249.
- Shen, B., Q. Li, C. Dong, and P. Perry. 2017. “Sustainability Issues in Textile and Apparel Supply Chains.” Sustainability 9 (9): 1592.
- Shen, B., X. Xu, and S. Guo. 2019. “The Impacts of Logistics Services on Short Life Cycle Products in a Global Supply Chain.” Transportation Research Part E: Logistics and Transportation Review 131: 153–167.
- Shin, N., S. Park, and S. Park. 2019. “Partnership-based Supply Chain Collaboration: Impact on Commitment, Innovation, and Firm Performance.” Sustainability 11: 449.
- Shou, Y., X. Zhao, J. Dai, and D. Xu. 2021. “Matching Traceability and Supply Chain Coordination: Achieving Operational Innovation for Superior Performance.” Transportation Research Part E: Logistics and Transportation Review 145: 102181.
- Singh, P. J., and D. Power. 2014. “Innovative Knowledge Sharing, Supply Chain Integration and Firm Performance of Australian Manufacturing Firms.” International Journal of Production Research 52: 6416–6433.
- Song, Y., T. Feng, and W. Jiang. 2017. “The Influence of Green External Integration on Firm Performance: Does Firm Size Matter?” Sustainability 9: 1328.
- Sundram, V. P. K., V. Chandran, and M. Awais Bhatti. 2016. “Supply Chain Practices and Performance: The Indirect Effects of Supply Chain Integration.” Benchmarking: An International Journal 23: 1445–1471.
- Swink, M., R. Narasimhan, and S. W. Kim. 2005. “Manufacturing Practices and Strategy Integration: Effects on Cost Efficiency, Flexibility, and Market-Based Performance.” Decision Sciences 36: 427–457.
- Terjesen, S., P. C. Patel, and N. R. Sanders. 2012. “Managing Differentiation-Integration Duality in Supply Chain Integration.” Decision Sciences 43: 303–339.
- Thai, V., and F. Jie. 2018. “The Impact of Total Quality Management and Supply Chain Integration on Firm Performance of Container Shipping Companies in Singapore.” Asia Pacific Journal of Marketing and Logistics 30: 605–626.
- Thomas, E. 2013. “Supplier Integration in new Product Development: Computer Mediated Communication, Knowledge Exchange and Buyer Performance.” Industrial Marketing Management 42: 890–899.
- Um, K.-H., and S.-M. Kim. 2019. “The Effects of Supply Chain Collaboration on Performance and Transaction Cost Advantage: The Moderation and Nonlinear Effects of Governance Mechanisms.” International Journal of Production Economics 217: 97–111.
- Vachon, S., and R. D. Klassen. 2008. “Environmental Management and Manufacturing Performance: The Role of Collaboration in the Supply Chain.” International Journal of Production Economics 111: 299–315.
- Vereecke, A., and S. Muylle. 2006. “Performance Improvement Through Supply Chain Collaboration in Europe.” International Journal of Operations & Production Management 26: 1176–1198.
- Wang, W., K.-H. Lai, and Y. Shou. 2018. “The Impact of Servitization on Firm Performance: A Meta-Analysis.” International Journal of Operations & Production Management 38: 1562–1588.
- Wiengarten, F., H. Li, P. J. Singh, and B. Fynes. 2019. “Re-evaluating Supply Chain Integration and Firm Performance: Linking Operations Strategy to Supply Chain Strategy.” Supply Chain Management: An International Journal 24: 540–559.
- Wiengarten, F., M. Pagell, M. U. Ahmed, and C. Gimenez. 2014. “Do a Country’s Logistical Capabilities Moderate the External Integration Performance Relationship?” Journal of Operations Management 32: 51–63.
- Willis, G., S. E. Genchev, and H. Chen. 2016. “Supply Chain Learning, Integration, and Flexibility Performance: An Empirical Study in India.” International Journal of Logistics Management 27: 755–769.
- Wong, C. Y., S. Boon-Itt, and C. W. Y. Wong. 2011a. “The Contingency Effects of Environmental Uncertainty on the Relationship Between Supply Chain Integration and Operational Performance.” Journal of Operations Management 29: 604–615.
- Wong, C. W. Y., K. Lai, and T. C. E. Cheng. 2011b. “Value of Information Integration to Supply Chain Management: Roles of Internal and External Contingencies.” Journal of Management Information Systems 28: 161–200.
- Wong, C. W. Y., K. H. Lai, and E. W. N. Bernroider. 2015. “The Performance of Contingencies of Supply Chain Information Integration: The Roles of Product and Market Complexity.” International Journal of Production Economics 165: 1–11.
- Wong, C. Y., C. Wong, and S. Boon-Itt. 2020. “Effect of Green Supply Chain Integration and Green Innovation on Environmental and Cost Performance.” International Journal of Production Research 58: 1–21.
- Yang, C.-L., S.-P. Lin, Y. Chan, and C. Sheu. 2010. “Mediated Effect of Environmental Management on Manufacturing Competitiveness: An Empirical Study.” International Journal of Production Economics 123: 210–220.
- Yu, Y., M. Zhang, and B. Huo. 2019. “The Impact of Supply Chain Quality Integration on Green Supply Chain Management and Environmental Performance.” Total Quality Management & Business Excellence 30: 1110–1125.
- Yuen, K. F., and V. V. Thai. 2017. “The Influence of Supply Chain Integration on Operational Performance: A Comparison Between Product and Service Supply Chains.” International Journal of Logistics Management 28: 444–463.
- Zhang, Q., J. Pan, Y. Jiang, and T. Feng. 2020. “The Impact of Green Supplier Integration on Firm Performance: The Mediating Role of Social Capital Accumulation.” Journal of Purchasing and Supply Management 26: 100579.
- Zhao, X., B. Huo, W. Selen, and J. H. Y. Yeung. 2011. “The Impact of Internal Integration and Relationship Commitment on External Integration.” Journal of Operations Management 29: 17–32.